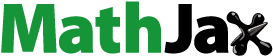
Abstract
Agriculture plays an indispensable role in the economic system of all countries, and the issue of agricultural carbon emissions has become a challenge to the sustainable development of the global economy. Against this backdrop, based on the data of 30 provinces, municipalities directly under the central government and autonomous regions (excluding Hong Kong, Macau and Tibet) in China from 2005 to 2019, this current study comprehensively uses the two-way fixed effect panel model and panel vector autoregressive (PVAR) model to explore the static and dynamic relationships between agricultural mechanization, large-scale operation and agricultural carbon emissions. Analysis show that agricultural mechanization and large-scale operation promote the agricultural carbon emissions, and increasing the level of urbanization will help curb the increase of agricultural carbon emissions. However, in a dynamic perspective, there is a two-way granger causality relationship between agricultural mechanization and agricultural carbon emissions. Agricultural mechanization is an important cause of agricultural carbon emissions and its promoting effect is sustainable, while the short-term effect of large-scale operation on agricultural carbon emissions is positive, and in the long run it helps reduce agricultural carbon emissions. Accordingly, the study recommends that the government should make the following efforts: firstly, speed up the research and development of agricultural machinery driven by clean energy; secondly, guide the effective transfer of land and encourage farmers to reduce the amount of agricultural chemical materials input while improving the use efficiency of pesticide chemical materials; thirdly, uphold the concept of ecological civilization in the process of promoting urbanization.
PUBLIC INTEREST STATEMENT
Agriculture plays an important role in many countries around the world, and agriculture is both a source of greenhouse gas emissions and a huge carbon sink system. Therefore, agricultural production is closely related to climate change caused by carbon dioxide emissions, and it is necessary to pay attention to the carbon emissions of the agricultural industry. Based on the two major trends of agricultural development, namely mechanization and large-scale management, this paper proposes the relationship between agricultural mechanization, large-scale management and agricultural carbon emissions.
1. Introduction
With the continuous intensification of greenhouse effect, global warming has triggered unprecedented extreme weather events all over the world, and countries around the world have begun to seek cooperation to cope with the coming crisis. It is generally accepted that rapid industrial development at the cost of high level of carbon dioxide emissions is one of the important reasons for environmental degradation (X. Liu & Bae, Citation2018). Different from industry, agricultural production is not only one of the most sensitive areas to climate change, but also one of the important sources of global anthropogenic greenhouse gas emissions. At the same time, agriculture is both a source of greenhouse gas emissions and a huge carbon sink system. Tomato production, for example, often requires input of a certain amount of fertilizer and pesticides during tomato planting, which will generate carbon emissions. However, the photosynthesis of tomato growth is a carbon sink process, and the subsequent picking, storage, transport and sale will also generate a certain amount of carbon emissions (Gokmenoglu & Taspinar, Citation2018; Ntim-Amo et al., Citation2022). Since agriculture plays an important part in the economy of many countries, and agricultural production is closely related to climate change caused by carbon dioxide emissions (Rehman et al., Citation2021). Therefore, the current agricultural carbon emissions need to be paid attention to, reducing agricultural carbon emissions and creating low-carbon agriculture are the future direction of the global society (Fais et al., Citation2016).
Agricultural mechanization and large-scale operation are two major agricultural development trends in many economies in the current period. Among them, as far as the former is concerned, agricultural mechanization refers to the process of using advanced agricultural machinery to equip agriculture and improve agricultural production and operation conditions, thus continuously improving agricultural economic and ecological benefits. So, agricultural mechanization is one of the manifestations of technological progress in the field of agriculture. According to the endogenous growth theory, technological progress can improve the efficiency of natural resource utilization and promote the effective recycling of resources, thus reducing energy consumption and environmental pollution at the same time. Agricultural technology is the main factor that directly affects the energy consumption of agricultural land. Agricultural technology progress can directly and indirectly affect carbon dioxide emissions, specifically: on the one hand, agricultural technology progress not only directly reduces carbon emissions per unit of energy consumption by improving traditional energy utilization efficiency, but also directly reduces carbon dioxide emissions by optimizing carbon dioxide capture and storage technology. On the other hand, the progress of agricultural technology indirectly optimizes the structure of agricultural energy consumption and increases the use of new renewable energy sources, thereby reducing the proportion of high-energy technologies in agricultural production. It is worth noting that the increase in total agricultural carbon emissions may also form a reverse force mechanism for the development of agricultural technology, forcing agricultural technology to progress. In theory, agricultural mechanization can become one of the important tools to promote agricultural emission reduction. However, it is undeniable that when agricultural mechanization does not achieve agricultural mechanization in the sense of technological progress, for example, the use of diesel as the driving energy of agricultural machinery, then this agricultural mechanization may only liberate rural productivity to a certain extent, and it is difficult to help energy conservation and emission reduction. Therefore, the impact of agricultural mechanization on agricultural carbon emissions is doubtful.
In terms of large-scale operation, large-scale operation mainly benefits from the outflow of rural labor force from agricultural production. In order to maintain the level of agricultural output, excessive chemical energy input such as fertilizer and agricultural film may be generated, thus contributing to the increase of agricultural carbon emissions (Kalantari et al., Citation2017; Rehman et al., Citation2017). Therefore, under the above background, it is of great practical significance to analyze the influence of agricultural mechanization and large-scale operation on agricultural carbon emissions. At the same time, development of agricultural industry also leads to the acceleration of urbanization. However, it is undeniable that in the process of urbanization, the use of fuels such as electricity, oil, gas and coal has also increased significantly, which leads to the increase of greenhouse gas emissions (Brown et al., Citation2012; Kurniawan & Managi, Citation2018; Shunsuke et al., Citation2008). Therefore, effects of other factors (such as urbanization level, infrastructure quality and industrial upgrading) brought by agricultural sector on agricultural carbon emissions should also be paid attention to.
There has been a lot of discussion in academic circles about agricultural mechanization and scale management. Among them, studies on agricultural mechanization mostly focus on the impact of agricultural mechanization on rural income growth (Z. Zhou et al., Citation2016), labor transfer (G. C. Li et al., Citation2018), grain production growth (Rajkhowa et al., Citation2020) and labor efficiency improvement (Belton et al., Citation2021). Research on scale management mostly focuses on analyzing the impact of scale management on the development of geographical indication agriculture (D. L. Li, Citation2022) and the demand for agricultural socialization services (D. P. Liu et al., Citation2019). The main research methods are simultaneous equation model and binary discrete model. It can be found that existing studies rarely focus on the impact of agricultural mechanization and scale management on agricultural carbon emissions, and rarely involve the interaction between these three. The innovation of this paper is mainly reflected in the following two points: Firstly, in the research content, the three factors of agricultural mechanization, scale management and agricultural carbon emission are put in the same system, and then the change of agricultural carbon emission is analyzed under the development trend of agricultural mechanization and large-scale operation. Secondly, the two-way fixed effect model and panel vector autoregressive (PVAR) model are comprehensively used to reveal the static and dynamic relationship between agricultural mechanization, scale management and agricultural carbon emissions.
Based on the above analysis, this paper comprehensively uses the two-way fixed effect panel model and PVAR model to explore the correlation between agricultural mechanization, large-scale operation and agricultural carbon emissions, and then tries to put forward policy suggestions for reducing agricultural carbon emissions. The main contents of the remaining part of this paper include: the second part is the literature review, systematically combing and summarizing the relevant literature; The third part is the methods and study data, briefly explaining the variable, data and model used in this paper. The fourth part is the study results and discussion, which analyzes and discusses the empirical results. The fifth part is the conclusion and recommendations.
2. Literature review
The topic of low-carbon development has been around for a long time. Low-carbon development is a sustainable development mode characterized by low energy consumption, low environmental pollution and low carbon emission, which is of great significance to the sustainable development of economy and society. There has been a wide discussion in the academic community about the calculation method of carbon emissions and the drivers of carbon emissions. Agricultural carbon emissions accelerate global warming. The promotion of low-carbon agriculture is an important strategy to relieve the pressure of world resources and protect the world ecological environment. It also promotes the organic unity of sustainable agricultural development and ecological environmental protection. As the only sector in the national economy that creates carbon sinks, more and more scholars pay attention to agricultural carbon emissions (Bilandžija et al., Citation2016; Lal, Citation2015). The following paper reviews relevant literature from two aspects: calculation methods of carbon emissions and driving factors of carbon emissions.
2.1. Calculation methods of carbon emissions
Carbon dioxide is the main greenhouse gas responsible for global warming and is therefore considered a major contributor to climate change (Jahanger et al., Citation2022; Rej et al., Citation2022). Therefore, studies related to environmental change mostly use carbon emissions as a key variable to measure environmental quality (Adewale Alola et al., Citation2021). In specific studies, a variety of indicators are used to measure carbon emissions. For example, Xu et al. considered the fossil fuels used in agricultural production activities, measured agricultural carbon emissions from the perspective of energy consumption, and selected six energy sources including coal, gasoline, kerosene, diesel, fuel oil and natural gas for estimation (Xu et al., Citation2021). Ramanathan applied the data envelopment analysis method to synthesize all relevant indicators such as energy consumption, economic activities and carbon emissions into an overall indicator and then carried out the follow-up analysis (Ramanathan, Citation2002). Zhou further constructed carbon emission performance indicators from the perspective of total factor production based on different DEA models, so as to dynamically reflect the total factor carbon emission performance of 18 major carbon emitting countries from 1997 to 2004 (P. Zhou et al., Citation2008). Mielnik and Goldemberg assessed climate evolution patterns in developing countries using a “carbonization index”, which refers to carbon emissions per unit of energy consumed (Mielnik & Goldemberg, Citation1999). Ang also used an energy intensity index with a similar connotation to “carbonization index” to study climate change (Ang, Citation1999). Some indicators, such as carbon emission intensity and per capita carbon emission, have been used in many studies related to carbon emission (Sun, Citation2005; Tol et al., Citation2006).
2.2. Drivers of carbon emissions
With regard to the influencing factors of carbon emissions, scholars have carried out a wealth of studies using data from different countries, mainly focusing on financial development, technological progress and agricultural development. There are mainly three views on the impact of financial development on carbon emissions. The first view is that financial development promotes the increase of carbon emissions (Sadorsky, Citation2010; Shahbaz et al., Citation2016). For example, Sadorsky found that financial development can ease credit constraints faced by household and business sectors, thereby stimulating increased consumption and investment, which will lead to increased energy consumption and further lead to increased carbon emissions (Sadorsky, Citation2010). In the study of developing and developed countries, Kim et al. found that financial development would hinder the development and application of green technologies, thus leading to the increase of energy consumption and carbon emissions, however, this negative impact would be mitigated by improvement of the quality of political institutions (Kim et al., Citation2021). The second view is that financial development inhibits the increase of carbon emissions (Acheampong et al., Citation2020; Bekhet et al., Citation2017; Lanoie et al., Citation1998). For example, Kumbaroglu et al (Kumbaroğlu et al., Citation2008) found that financial development can reduce carbon emissions and environmental pollution by promoting technological innovation in the energy sector. Tamazian and Rao, Zeqiraj et al. and Kim et al. also pointed out that financial development can guide financial resources to environmentally friendly investment projects, thus restricting environmental pollution behavior to a certain extent (Kim et al., Citation2020; Tamazian & Bhaskara Rao, Citation2010; Zeqiraj et al., Citation2020). The third view holds that there is no inevitable relationship between financial development and carbon emissions (Omri et al., Citation2015; Ozturk & Acaravci, Citation2013). Regarding the impact of technological progress on carbon emissions, green technology innovation contributes to reducing raw material waste and improving energy efficiency (Gerlagh & Lise, Citation2005; Grossman & Krueger, Citation1995; Porter & van der Linde, Citation1995).
Scholars have also conducted a series of studies on the impact of agricultural development on carbon emissions. For example, Li et al. took China as an example and built an agricultural modernization index based on data such as the use of agricultural mechanization tools, irrigation rate, fertilizer application, pesticide application, grain output per unit of land and grain output per capita (S. Li et al., Citation2019). The research results showed that improvement of agricultural modernization promoted the increase of China’s carbon emissions. Jiang et al. selected data of China and found that there are two main factors leading to the increase of carbon emissions (Jiang et al., Citation2021). One is the increase in food demand caused by the increase in rural population, and the other is the increase in per capita cultivated land area. Amin et al. analyzed the long-term and short-term effects of agricultural modernization on carbon emissions by using data from Nepal, Bangladesh, Pakistan, India and other countries, results showed that although agricultural modernization would restrain the increase of carbon emissions in the short term, in the long term, this trend would reverse (Amin et al., Citation2022). Balsalobre-Lorente et al. analyzed the relationship between carbon emissions and agriculture in BRICS countries by using the share of agricultural added value, and concluded that agricultural production in BRICS countries accelerated carbon emissions (Balsalobre-Lorente et al., Citation2019). Wang et al. analyzed the carbon emissions of G7 countries, and concluded that agricultural added value has a negative impact on environment (Wang et al., Citation2020). However, Dar and Asif, using data from South Asian countries, found that countries with a higher share of total agricultural output have lower carbon emissions (Dar & Asif, Citation2019). Rafiq et al., using data from 53 countries including high-, middle- and low-income countries, also found that increasing the share of agricultural output contributes to reducing carbon emissions (Rafiq et al., Citation2016). Manufacturing and agriculture are the pillars of Indonesia’s national economy and have a strong potential for environmental damage. Slamet et al. also concluded that manufacturing, agriculture and urbanization are all causes for the increase of carbon emissions in Indonesia (Slamet et al., Citation2020). Agriculture also plays an indispensable role in promoting Nepal’s socio-economic development. Abdul et al. showed that the decrease of agricultural land area and the increase of fertilizer application level contribute to the increase of carbon emissions in Nepal (Abdul Jabbar et al., Citation2022).
In summary, existing literature has fully discussed the relationship between agricultural development and carbon emissions, which provides a valuable reference for this current study. However, the impact of specific changes in agricultural production (such as agricultural mechanization and large-scale operation) and closely related factors (such as urbanization level, infrastructure construction and industrial upgrading) on agricultural carbon emissions is still unclear, and the dynamic relationship between agricultural mechanization, large-scale operation and agricultural carbon emissions needs to be supplemented. Therefore, this paper attempts to use panel data of 30 provinces (excluding Hong Kong, Macau and Tibet) in China as an example to comprehensively analyze the relationship between agricultural mechanization, large-scale operation and agricultural carbon emissions.
3. Methods and study data
3.1. Data source and processing
This study analyzes the data of 30 provinces in China (which is limited to data availability, not including Hong Kong, Macau and Tibet, and the same below) from 2005 to 2019, and the data is mainly derived from the Annual Chinese Statistics Yearbook and the Chinese Macro Statistics. For some missing data values, the linear interpolation method is used in this paper. Finally, the total sample size of this paper is 450.
3.2. Empirical models
3.2.1. Two-way fixed effect model
In order to study the direct impact of agricultural mechanization and large-scale operation on agricultural carbon emissions, and effectively control the individual effect and time effect, this paper adopts the two-way fixed effect panel model and constructs the following equation:
Where, represents province,
represents year,
represents explained variable, namely agricultural carbon emissions.
represents agricultural mechanization.
represents large-scale operation.
represents a set of control variables affecting agricultural carbon emissions.
and
represent individual effect and time effect in the model, respectively.
represents the disturbance.
3.2.2. Panel Vector Autoregressive (PVAR) model
There may be a bi-directional feedback mechanism between agricultural mechanization, large-scale operation and agricultural carbon emissions, and the influence is in the dynamic evolution process. Therefore, based on data availability and integrity, this study uses PVAR model to systematically identify the relationship among agricultural mechanization, large-scale operation and agricultural carbon emissions. PVAR model is generally used to analyze the dynamic influence relationship between variables. It has the dual advantages of both time series and panel data, which is conducive to the screening of the dynamic relationship between different variables in panel data that are endogenous variables to each other, and can also effectively reveal the two-way causal relationship between variables. The PVAR model constructed in this paper includes model estimation (generalized moment estimation GMM), impulse response, Granger causality test and variance decomposition. Among them, GMM preliminarily reports the relationship between variables and the influence between variables and the lag period. Impulse response further measures the effect of random disturbance of one variable on other variables. Granger causality test identifies the causal relationship between variables; Variance decomposition reflects the contribution of one variable to other variables. This paper sets the following PVAR model:
Where, represents the province,
represents the year,
represents the lag order of endogenous variables,
is the lag term coefficient matrix to be estimated,
is the individual fixed effect vector,
is the time fixed effect vector, and
is the random disturbance term.
3.3. Description of variables
3.3.1. The explained variable: carbon (agricultural carbon emissions)
Agricultural carbon emissions refer to greenhouse gas emissions (mainly carbon dioxide) caused directly or indirectly by the application of chemical fertilizers and pesticides, energy consumption and land ploughing and irrigation in the process of agricultural production. The agricultural carbon emission evaluation system mainly includes the following two aspects: First, the carbon emission generated by the input of agricultural materials in the process of agricultural production, including agricultural fertilizers, pesticides, agricultural films and agricultural diesel; The second is the carbon emissions caused by farmland changes in the process of agricultural production, including farmland tilling and farmland irrigation. Therefore, agricultural carbon sources are identified as six aspects, namely pesticides, irrigation, agricultural film, fertilizer, diesel oil and ploughing. The corresponding carbon emission coefficients and reference sources of different carbon sources are shown in Table . The carbon emission coefficient refers to the amount of carbon emissions generated per unit of energy in the process of burning or using each energy source. Taking pesticides as an example, the carbon emission coefficient of the use of pesticides is 4.9341 kg/kg, which means that every 1 kg of pesticides will produce 4.9341 kg of carbon emissions. The total agricultural carbon emission of each province is equal to the sum of the product of the conversion amount of chemical fertilizer applied, the amount of pesticide used, the amount of agricultural plastic film used, the amount of agricultural diesel used, the total sown area and the effective irrigated area of the corresponding province and the carbon emission coefficient respectively.
Table 1. Carbon sources, coefficient and reference sources of agricultural carbon emissions
Based on the calculation method of agricultural carbon emissions, Figures show the agricultural carbon emission information of 30 provinces in China in 2005, 2010, 2015 and 2019. As can be seen from the figure, the agricultural carbon emission of Shandong Province was 11.26 million tons in 2005, ranking the first. In 2010, the agricultural carbon emission of Shandong Province rose to 11.31 million tons, ranking the second. The agricultural carbon emission of Henan province ranked first in 2010, 2015 and 2019, with 12.49 million tons, 13.35 million tons and 12.61 million tons respectively, showing a trend of gradual decline. It is not difficult to find that, from a horizontal perspective, different provinces have different agricultural carbon emissions. Vertically, agricultural carbon emissions in different provinces are a dynamic changing process over time. Such differences and dynamic changes are determined by a variety of factors, such as differences in urbanization levels, agricultural mechanization levels, large-scale operations, infrastructure construction and industrial upgrading in different provinces or in the same province in different years. In the fourth part of this paper, econometric models are used to analyze the impact of agricultural mechanization and large-scale operation on agricultural carbon emissions, and the impact of infrastructure quality, industrial upgrading and urbanization level on agricultural carbon emissions is also investigated. On this basis, the dynamic correlation between agricultural mechanization, large-scale operation and agricultural carbon emissions is further discussed.
3.3.2. Core explanatory variables
One of the cores explanatory variables of this paper is agricultural mechanization, which is measured by the total power of agricultural machinery in each province.
Another core explanatory variable that this paper focuses on is large-scale operation. The geographical condition of China is large population and small land, and thus forms a dispersed small-scale peasant production mode, which is characterized by small-scale, fragmented and dispersed. China implements the household contract responsibility system, and most areas distribute land equally according to population or labor force, forming a decentralized small-scale production mode. Therefore, in this context, academics usually define large-scale operation in terms of the area of land cultivated by population or labor. Referring to the studies of Zhu and Wang (Citation2023) and Chen and Wang (Citation2022), the ratio of the total sown area of crops in each province to the corresponding number of employees in the primary industry is taken as the proxy variable of large-scale operation.
3.3.3. Control variables
In order to eliminate the possible adverse effects of other factors on the measurement results, this paper added the variables of industrial upgrading (AGR), infrastructure quality (ROAD) and urbanization level (URB) into the empirical model. The above variables are added for the following reasons: First, agricultural carbon emissions are mainly derived from production links, and the development of industrial structure is a key factor affecting agricultural carbon emissions (X. Li, Citation2014; Mohareb & Kennedy, Citation2012), therefore, The variable AGR is added in this paper; Second, infrastructure quality is the leading capital of rural social development, which drives the rectification and reconstruction of projects such as rural biogas digesters and hydropower pipe networks, thus affecting agricultural non-point source pollution and agricultural carbon emissions (Lin et al., Citation2020). Therefore, the variable of ROAD is added in this paper. Third, the increase of urbanization leads to the reduction of rural labor force, which encourages farmers to pay attention to large-scale and intensive management, thus helping to save resources and reduce agricultural carbon emissions (Hou & Yao, Citation2018). At the same time, the status of labor transferred to cities has changed from producers of agricultural products to consumers of agricultural products, increasing the demand for the quality of agricultural products, which encourages farmers to adopt green and low-carbon agricultural technologies, reduce pesticide and fertilizer input, and thus reduce agricultural carbon emissions (J. H. Zhou et al., Citation2015). Therefore, the variable of URB is included in this paper.
In concrete measures, the industrial upgrading variable is measured by the proportion of the gross output value of the secondary and tertiary industries in GDP. The infrastructure quality is measured by the grade highway mileage. The urbanization level variable is measured by the proportion of urban population and rural population. In order to overcome the potential heteroscedasticity problem in the econometric model, this paper takes logarithm of the corresponding real index. The descriptive statistics of the indicators used in this paper are shown in Table .
Table 2. Variable description and descriptive statistics
4. Study results and discussion
4.1. Two-way fixed effect model
In this part, the two-way fixed effect model is adopted to empirically analyze the impact of agricultural mechanization and large-scale operation on agricultural carbon emissions, and the regression results are shown in Table . In the first column of Table , only MACH and SCALE are added. In column 2–5, AGR, URB, ROAD and lag phase 1 agricultural carbon emissions (L.CARBON) are gradually added. As can be seen from the estimation results, with the continuous increase of control variables, R2 gradually increases, and the R2 in column 5 reaches 0.9563, which indicates that the regression model in this paper has a good fitting effect. In addition, no matter how the control variables are adjusted, the estimated coefficients of MACH and SCALE are both significantly positive, indicating that overall, the development of mechanization and large-scale operation in the current agricultural sector contributes to the increase of agricultural carbon emissions. Based on the data of 13 provinces in China’s major grain-producing areas from 1998 to 2019, He et al. (Citation2023) constructed the environmental Kuznets model and quantile regression model to analyze the impact of agricultural mechanization on agricultural carbon emissions, and also concluded that agricultural mechanization has a positive promoting effect on agricultural carbon emissions.
Table 3. Estimation results of two-way fixed effect model
From the perspective of control variables, the regression coefficient of URB variable is significantly negative, indicating that urbanization level has a significant negative impact on agricultural carbon emissions, which means that promoting the improvement of urbanization level is conducive to curbing agricultural carbon emissions and promoting the green development of agriculture. This is because the level of urbanization reflects the level of regional economic development. With the improvement of the level of urbanization, the urban lifestyle and resource utilization mode have changed, thus reducing the consumption of agricultural resources and agricultural carbon emissions to a certain extent. In addition, higher levels of urbanization may promote the flow of land to other non-agricultural entities, which make more rational and scientific use of organic agricultural inputs and low-carbon agricultural machinery. And higher levels of urbanization may also or promote farmers to farm more traditional food crops rather than cash crops, thus reducing agricultural carbon emissions. Wei et al. (Citation2023) also concluded that urbanization level has a significant negative impact on agricultural carbon emissions.
4.2. Panel Vector Autoregressive (PVAR) model
4.2.1. Unit root check of panel data
Before estimating the model, it is necessary to conduct the stationarity test of each variable to judge the stationarity of panel data, so as to ensure that the model can be accurately estimated and the pseudo-regression can be avoided. In this paper, three methods, LLC test, IPS test and ADF-Fisher test, are used to conduct stationary test on the data. If the test results all reject the null hypothesis, it indicates that the sequence is stable; otherwise, it indicates that the sequence is unstable. Panel unit root test is carried out after first-order difference processing of each variable, and the test results are shown in Table . The results show that all the test methods reject the null hypothesis of the existence of unit root at the significance level of 1%. Therefore, it is determined that all variables are stable in this paper, which indicates that six variables are first-order monolithic processes, and the PVAR model can be preliminarily constructed.
Table 4. Unit root check of panel data
4.2.2. Co-integration test
The results of co-integration test are shown in Table . According to the test results, there is a long-term co-integration relationship between agricultural mechanization, large-scale operation and agricultural carbon emissions, which provides strong support for the following analysis.
Table 5. Results of co-integration test
4.2.3. Judgment of the optimal lag order
An important part of PVAR model estimation is to determine the optimal lag order. Determining the optimal lag order can improve the accuracy of PVAR model estimation results. The optimal lag order is determined by AIC, BIC and HQIC criteria. It can be seen from Table that the optimal lag period of PVAR model is 2.
Table 6. Lag order of the model
As shown in Figure , data stationarity test results show that all feature roots are distributed in the unit circle, indicating that the PVAR model meets the stability condition.
4.2.4. Generalized Moment (GMM) estimation of PVAR model
The estimation results obtained by GMM are shown in Table . It can be found from the estimated results that:
Taking h_DCARBON as the explained variable, the estimated coefficients of L.h_DCARBON, L.h_DMACH, L.h_DURB and L.h_DROAD, which lag one stage, all pass the significance test. Among them, the estimated coefficients of L. h_CARBON and L.h_DMACH are significantly positive at the 5% level, the estimated coefficient of L.h_DURB is significantly positive at the 10% level, and the estimated coefficient of L.h_DROAD is significantly positive at the 1% level. The regression coefficients of L.h_DSCALE and L.h_DAGR, which lag one stage, are both positive, but fail to pass the significance test. The above results indicate that in the initial stage of the transformation from traditional agriculture to modern agriculture, the improvement of agricultural mechanization level and urbanization level are likely to be the main factors promoting the increase of agricultural carbon emissions.
Taking h_MACH as the explained variable, the estimated coefficients of L.h_DCARBON, L.h_DMACH and L.h_DAGR with a lag of one stage are all positive, where, the estimated coefficient of L.h_DCARBON is significantly positive at the level of 1%, the estimated coefficients of L.h_DSCALE, L.h_DURB and L.h_DROAD with a lag of one stage are all negative. The above results indicate that agricultural mechanization is affected by many complex factors, and the increase of agricultural carbon emission forces the improvement of agricultural mechanization level.
Taking h_DSCALE as the explained variable, the estimated coefficients of L.h_DCARBON and L.h_DAGR, which lag one stage behind, are both negative. However, the estimated coefficients of L.h_DMACH, L.h_DSCALE, L.h_DURB and L.h_DROAD, which lag one stage behind, are all positive. The above results indicate that there is no effective incentive mechanism to promote large-scale operation at present.
Taking L.h_DAGR as the explained variable, the estimated coefficients of L.h_DCARBON, L.h_DMACH and L.h_DSCALE with a lag of one stage are all negative. The estimated coefficients of L.h_DAGR, L.h_DURB and L.h_DROAD, which lag one stage behind, are all positive. Among them, the estimated coefficients of L.h_DAGR and L.h_DROAD are significantly positive at the level of 5%, and the estimated coefficient of L.h_DURB is significantly positive at the level of 10%. The above results show that industrial upgrading itself has formed a good inertia of development, and improving the quality of infrastructure and the level of urbanization can effectively promote industrial upgrading.
Taking h_DURB as the explained variable, the estimated coefficients of L.h_DCARBON, L.h_DMACH, L.h_DAGR, L.h_DURB and L.h_DROAD with a lag of one stage are all negative. The estimated coefficient of L.h_DSCALE with a lag of one stage is positive. The above results may be due to the fact that the current urbanization is still dependent on the macro-control of the government. The government can directly affect the level of urbanization by adjusting the structure of fiscal revenue and expenditure, adopting fiscal tax means and enriching financing methods.
Taking h_DROAD as the explained variable, the estimated coefficients of L.h_DCARBON, L.h_DMACH, L.h_DSCALE, L.h_DAGR and L.h_DROAD with a lag of one stage are all positive. The estimated coefficient of L.h_DURB with one stage lag is negative. Among them, the estimated coefficients of L.h_DCARBON and L.h_DMACH are significantly positive at the level of 5%. The estimated coefficient of L.h_DROAD is significantly positive at the 1% level. The above results may be attributed to the urgent need to enhance agricultural mechanization to replace the insufficient supply of labor force in order to achieve large-scale operation. The misplacement of agricultural production cycle in different provinces and the improvement of the utilization efficiency of agricultural machinery promote the development of cross-regional operation of agricultural machinery, which raises the requirements for the infrastructure quality. The infrastructure quality itself has formed a good inertia of development.
Table 7. GMM estimation results of PVAR model
4.2.5. Granger causality test
Granger causality test can determine whether the lag term of one variable has a significant impact on other variables, and is used to investigate whether the explanatory variable has the predictive ability of the explained variable, so as to prove whether there is a temporal causal relationship between the two variables. If a variable can predict another variable to a certain extent, it can be determined that the former variable is the Granger reason of the latter variable. In this part, under the PVAR framework, Granger causality test of each variable with a lag of 2 periods is carried out respectively, and the test results are shown in Table . The following conclusions can be drawn from the test results: DCARBON and DMACH are granger reasons of each other at the significance level of 5%. DROAD and DCARBON are each other’s Granger reasons at the significance level of 10%. DSCALE and DMACH, DAGR and DSCALE, DAGR and DROAD, DROAD and DMACH all show significant one-way Granger causality relationship. Among them, DMACH is DSCALE’s Granger reason with a significance level of 10%, DSCALE is DAGR’s Granger reason with a significance level of 1%, DROAD is DAGR’s Granger reason with a significance level of 5%, DMACH is DROAD’s Granger reason with a significance level of 5%. Generally speaking, industrial upgrading is conducive to promoting carbon emission reduction and is the cause of carbon emission reduction. However, as the established technology level and industrial upgrading process are limited by economic laws and practical development, the carbon emission reduction effect of industrial upgrading is affected (Niu & Jiang, Citation2013).
Table 8. Granger causality test results
4.2.6. Pulse response analysis
Impulse response is used to judge the dynamic impact of one standard deviation shock on a variable on other variables, which cannot be captured by the ordinary panel regression model. In this part, the impulse response is used to test the short- and long-term interdependence of the relationship among agricultural mechanization, large-scale operation and agricultural carbon emissions. The impulse response diagram is obtained by Monte-Carlo simulation for 200 times (as shown in Figure ). In Figure , the horizontal axis (S-axis) represents the lag order of impact, and the upper and lower curves contain 95% confidence intervals. The middle curve is the IRF response curve, and the vertical axis is the response intensity.
The first row of Figure shows the responses of agricultural carbon emissions to the impact on related variables. When agricultural mechanization is hit by an impact, it has a positive effect on agricultural carbon emissions, which reaches the maximum value at the end of the second period, and then gradually weakens. This indicates that agricultural mechanization is an important cause of agricultural carbon emissions, and the positive effect of agricultural mechanization on agricultural carbon emissions is sustainable. When scale operation is hit by an impact, it has a positive effect on agricultural carbon emissions in the first period. This effect reaches a peak at the end of the first period, then begins to decline, reaches a maximum negative effect at the end of the second period, and begins to converge to zero in the fourth period. It can be seen that when there is a short-term impact on large-scale operation, agricultural operators have less knowledge of new fertilization and application technologies, leading to extensive investment in agricultural chemicals and an increase in agricultural carbon emissions. However, in the long run, large-scale operation is conducive to promoting agricultural operators to adjust agricultural production inputs, such as adopting efficient fertilization technology, so as to improve the use efficiency of chemical inputs and reduce agricultural carbon emissions. When industrial upgrading is hit by an impact, the effect on agricultural carbon emissions is positive, and the positive effect reaches the maximum at the end of the second period, and then begins to decline, but increases slightly in the fourth period, and then continues to decline. When urbanization level is hit by an impact, it has a positive effect on agricultural carbon emissions, which reaches its maximum value at the end of the first period and then begins to decline, and in the long run, this effect tends to converge to zero. The impact on infrastructure quality in the current period has a positive effect on agricultural carbon emissions. This effect reaches the maximum at the end of the first period and then begins to decline, and then gradually declines after a slight increase in the third period. Therefore, it is not difficult to find that industrial upgrading and infrastructure quality have a sustained impact on agricultural carbon emissions.
The second row of Figure shows the responses of agricultural mechanization to the impact on related variables. When agricultural carbon emission is hit by an impact, it has a promoting effect on agricultural mechanization, which reaches the peak value at the end of the first period and gradually decreases. It shows that agricultural carbon emission has a sustainable promoting effect on agricultural mechanization. When large-scale operation is impacted, the effect on agricultural mechanization is negative in the current period, reaches the minimum value at the end of the first period, then increases, and begins to converge in the fifth period. Thus, in the short term, scale operation hinders agricultural mechanization. This may be because China’s agricultural development still has the characteristics of more people and less land, so the agricultural field has not fully formed the conditions conducive to agricultural mechanization operation. When industrial upgrading is hit by an impact, it has a positive effect on agricultural mechanization, and this promoting effect reaches a peak at the end of the third period and then begins to decline. Therefore, the promoting effect of industrial upgrading on agricultural mechanization is sustainable. When infrastructure quality is impacted, it has a negative effect on agricultural mechanization in the first period, then a positive effect from the second period, and then a positive effect all the time, indicating that although infrastructure quality is not conducive to agricultural mechanization in the short term, it will continue to promote the agricultural mechanization in the long term.
The third row of Figure shows the responses of large-scale operation to the impact on related variables. The effect of agricultural mechanization change on large-scale operation shows a decreasing trend and converges to zero from the fourth period. The reason for this phenomenon may be the slowing down of economic development and industrial transformation (especially the development of agricultural machinery service outsourcing market in recent years has diversified agricultural machinery operation methods), which leads to the fluctuation of large-scale operation level. When large-scale operation is impacted, its impact on itself reaches a positive maximum during the impact period, and then begins to decline until it begins to converge to zero at the end of the third period. Therefore, it can be seen that large-scale operation is unsustainable. In the case of the impact on urbanization level and infrastructure quality, the former has a negative effect on large-scale operation, and the latter has a positive effect on large-scale operation, and both of these effects begin to converge at zero from the fourth period.
The fourth row of Figure describes the responses of industrial upgrading to the impact on related variables. When agricultural carbon emission is impacted, its effect on industrial upgrading is positive in the current period, and then decreases. It becomes negative at the beginning of the second period, and then maintains negative effect all the time, and converges to zero from the sixth period. When agricultural mechanization is impacted, the negative effect on industrial upgrading reaches its peak in the current period, and with the passing of time, the negative effect begins to decline, and begins to converge to zero at the end of the second period. When large-scale operation is impacted, its effect on industrial upgrading is negative in the current period, then increases, and reaches the peak of positive effect at the end of the second period and then begins to decline, which indicates that short-term large-scale operation is conducive to promoting industrial upgrading, and in the long run, this effect tends to converge to zero. When industrial upgrading is impacted, its effect on itself reaches a peak in the current period, and then decreases continuously until it begins to converge to zero at the end of the fourth period, indicating that industrial upgrading is not sustainable. In the case of the impact on urbanization level and infrastructure quality, both of them have a positive effect on industrial upgrading, and in the long run, they converge to zero. It is not difficult to find that none of agricultural carbon emissions, agricultural mechanization, large-scale operation, industrial upgrading, urbanization level and infrastructure quality has a sustained effect on industrial upgrading.
The fifth row of Figure shows the responses of urbanization level to the impact on related variables. When agricultural carbon emission, agricultural mechanization, industrial upgrading and infrastructure quality are impacted, they all have a negative effect on the urbanization level. Among them, the negative effect of agricultural carbon emissions on the urbanization level reaches the peak at the end of the first period, and the negative effects of agricultural mechanization, industrial upgrading and infrastructure quality on the urbanization level all reach the peak at the end of the second period. When large-scale operation is impacted, its effect on urbanization level decreases after reaching the maximum in the current period, and continues to decline after recovering slightly in the second period. In the long run, all of these effects have a tendency to converge to zero. It follows that none of agricultural carbon emissions, agricultural mechanization, large-scale operation, industrial upgrading, urbanization level and infrastructure quality has a sustained effect on urbanization level.
The sixth row of Figure shows the responses of infrastructure quality to the impact on related variables. When agricultural carbon emission is impacted, it has a positive effect on infrastructure quality, which reaches a peak at the end of the first period and then begins to decline. When agricultural mechanization is hit, the effect on infrastructure quality is always positive. In terms of phases, this positive effect shows a downward trend in the first phase, begins to rise at the beginning of the second phase, reaches a peak at the end of the second phase and then declines, and this positive effect converged to zero in the long run. Thus, the effect of agricultural mechanization on the infrastructure quality is sustainable. A impulse on industrial upgrading has a similar effect on infrastructure quality as an impulse on agricultural mechanization. However, in terms of the impact on large-scale operation and urbanization level, both of them have a negative effect on the infrastructure quality, and both of these negative effects begin to converge at zero in phase six. It can be seen that although changes in large-scale operation and urbanization level in the short term have an inhibitory effect on the infrastructure quality, this inhibitory effect will gradually weaken with the passing of time.
4.2.7. Variance decomposition
Variance decomposition gives the contribution degree of variance to the mutual interpretation of different variables. Table shows the variance decomposition results of lag period 1, 5 and 10.
Variance decomposition of agricultural carbon emissions (DCARBON): In the first phase, the variance contribution rate came from agricultural carbon emission itself. Since then, agricultural carbon emission has always had a great impact on itself, which is consistent with the above result of impulse response. Although the variance contribution rate of agricultural carbon emissions decreased gradually, it still maintained absolute advantages. For example, in the 10th phase, the variance contribution rate of agricultural carbon emissions still reached 80.2%. DMACH is the factor with the largest variance contribution rate to agricultural carbon emission except agricultural carbon emission itself, and the variance contribution rate of agricultural mechanization in the 5th and 10th phases reaches 7.1% and 8.4%, respectively. The variance contribution rate of DSCALE to agricultural carbon emissions is not obvious, basically stable at 0.2%, which is basically consistent with the result of impulse response. The contribution of DAGR and DROAD increased with the extension of the forecast period, staying at 1.9% and 4.3% respectively in the fifth phase, and rising to 2.4% and 5.1% respectively in the 10th phase. In the long run, the infrastructure quality is another minor factor affecting agricultural carbon emissions.
Variance decomposition of agricultural carbon emissions (DMACH): The variance contribution rate of the first phase mainly came from the agricultural mechanization itself, and the variance contribution rate of agricultural mechanization was 99.7%. The variance contribution of DCARBON in the first phase was 0.3%. With the extension of the prediction period, the variance contribution rate of agricultural carbon emissions increased, which was 7.9% and 9.4% in the 5th and 10th periods, respectively. DURB is another secondary factor affecting agricultural mechanization, and the variance contribution rate remained above 7% in the later period. In the long run, agricultural mechanization is most important to itself and remains firmly dominant.
Variance decomposition of large-scale operation (DSCALE): The variance contribution rate mainly came from the large-scale operation itself. Although the large-scale operation’s contribution rate decreased with the lag period, it remained at the variance contribution rate of 93.4% in the late period. However, the contribution of DCARBON, DMACH, DAGR, DURB and DROAD were all small and remained unchanged in the later period, which were 4.0%, 0.9%, 0.7%, 0.7% and 0.2%, respectively. It can be seen that agricultural carbon emission is the factor that has the biggest impact on large-scale operation besides large-scale operation itself.
Variance decomposition of industrial upgrading (DAGR): The variance contribution rate of the first period mainly came from the industrial upgrading, then the DCARBON, DMACH and DSCALE, which contributed to 93.2%, 1.9% and 4.7% respectively. The variance contribution rates of DURB and DROAD were all not obvious. In the later period, the contribution of agricultural mechanization to industrial upgrading remained at 4.1%, while the contribution of urbanization level and infrastructure quality gradually increased and remained at 7.0% and 4.8%, respectively. It can be seen that, agricultural carbon emissions, large-scale operation, urbanization level and infrastructure quality all have gradually increased explanatory power for industrial upgrading, that is, long-term effect is obvious. Relatively speaking, agricultural mechanization has stronger explanatory power for industrial upgrading in the short term.
Variance decomposition of urbanization level (DURB): The variance contribution rate mainly came from the urbanization level itself. Although the contribution rate of urbanization level decreased with the passing of lag period, the variance contribution rate remained above 94%. The variance contribution rate of DSCALE remained stable. The contribution of DCARBON, DMACH, DAGR and DROAD were all small, but they all tended to increase over time. Among them, the contribution of large-scale operation was relatively highest, which may be because scale operation enables more and more rural population to gather in cities, promotes the concentration of demand, division of labor and specialization, promotes the improvement of structural efficiency and economies of scale, and thus promotes the construction of urbanization.
Variance decomposition of infrastructure quality (DROAD): In the first phase, variance contribution was mainly from infrastructure quality itself, followed by DMACH. The contribution of DURB was not significant, and the impacts of DCARBON, DSCALE and DAGR on infrastructure quality were all not significant. Subsequently, the contribution rate of infrastructure quality gradually decreased to 74.6% in the 10th phase. It is noteworthy that with the passing of lag period, the contribution degree of agricultural carbon emission and agricultural mechanization gradually increased, among which, the contribution degree of agricultural carbon emission increased from 7.1% in the 5th period to 10.2% in the 10th period, and the contribution degree of agricultural mechanization increased from 9.2% in the 5th period to 9.9% in the 10th period. In other words, the explanatory power of agricultural carbon emissions and agricultural mechanization on infrastructure quality has gradually increased, showing obvious long-term effects. These results further echo the impulse response analyses above.
Table 9. Results of PVAR variance decomposition
5. Conclusion and recommendations
Based on the data of 30 provinces and municipalities in China from 2005 to 2019, this paper analyzes the relationship between agricultural mechanization, large-scale operation and agricultural carbon emissions by using the two-way fixed-effect model and PVAR model. The main conclusions are as follows: First, agricultural mechanization and large-scale operation have both promoted the increase of agricultural carbon emissions, which indicates that the intelligent and green level of agricultural machinery and equipment at this stage still needs to be improved, and agricultural large-scale operation is still a traditional extensive and low level of development. Therefore, it is urgent to promote the transformation and upgrading of agricultural mechanization and large-scale operation. Improving the level of urbanization can help restrain the increase of agricultural carbon emissions, which means that promoting the urbanization process is one of the feasible ways to reduce agricultural carbon emissions. Second, there is interaction between agricultural mechanization and agricultural carbon emissions. Agricultural mechanization is an important reason to promote the increase of agricultural carbon emissions, and this promotion effect is sustainable in time. However, the increase in agricultural carbon emissions will in turn force the transformation and upgrading of agricultural mechanization. Third, the impact of large-scale operation on agricultural carbon emissions varies over time. Large-scale operation will not reduce carbon emissions in a short period of time, but positively promote the increase of agricultural carbon emissions. Only when large-scale operation reaches a certain level in the time dimension will it play a role in restraining the increase of agricultural carbon emissions.
According to the above research conclusions, this paper puts forward the following three suggestions: First, given that agricultural mechanization is an important factor affecting agricultural carbon emissions, it is necessary to accelerate the research and development and application of agricultural machinery driven by clean energy, so as to reduce the carbon emissions of agricultural machinery from the energy port. The traditional agricultural machinery powered by diesel has a high carbon emission. Therefore, the application of technological innovation in agricultural mechanization and new energy development should be strengthened, and the innovation of new energy agricultural machinery should be developed, so as to develop a low-carbon agricultural mechanization development path. Second, in view of the fact that scale operation can inhibit the increase of agricultural carbon emissions in the long run, it is suggested to improve the land transfer management system and guide the land transfer effectively to promote the appropriate scale operation of agriculture. At the same time, farmers should be guided by various ways to actively apply advanced information technology and ecological agriculture technology, so as to reduce the input of agricultural chemical materials in the process of agricultural scale operation and improve the use efficiency of agricultural chemical materials. These measures include accelerating the establishment of agricultural technology production, university and research systems, effectively promoting ecological compensation systems and facilities, and increasing government subsidies for agricultural technology. When it comes to specific production levels, for example, farmers are encouraged to choose new environmentally friendly fertilizers and pesticides, scientifically combine organic and inorganic fertilizers, use degradable agricultural film, and use water-saving irrigation methods such as sprinkler irrigation or drip irrigation, so as to improve the use efficiency of chemical materials and agricultural green production level, and reduce direct carbon emissions from agriculture. When it comes to specific production levels, for example, farmers can be encouraged to choose new environmentally friendly fertilizers and pesticides, scientifically combine organic and inorganic fertilizers, use degradable agricultural film, and use water-saving irrigation methods such as sprinkler irrigation or drip irrigation, so as to improve the efficiency of chemical materials use and reduce direct agricultural carbon emissions. Third, in view of the fact that improving the level of urbanization is a feasible way to curb agricultural carbon emissions at this stage, it is suggested to further promote urbanization construction by adhering to the concept of ecological civilization, so as to comprehensively improve the resource utilization efficiency in the process of urbanization construction. At the same time, all aspects of construction and management in the process of urbanization should be promoted to meet the green and low-carbon requirements, so as to comprehensively improve the green and low-carbon ecological environment and give full play to the agricultural carbon emission reduction effect of urbanization.
Disclosure statement
No potential conflict of interest was reported by the author(s).
Additional information
Notes on contributors
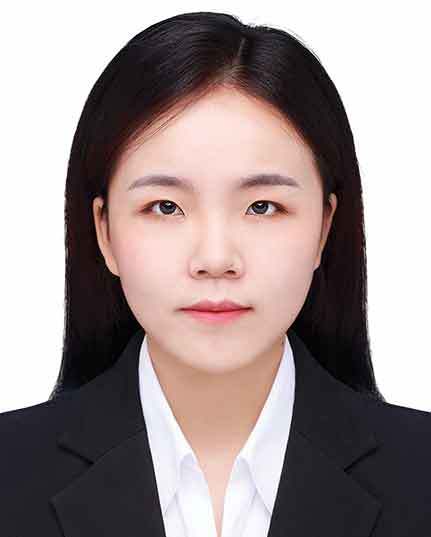
Ningning Guan
Ningning Guan is a professional teacher at Nanjing XiaoZhuang University. Her dissertation focuses on agricultural carbon emissions, discussing the static and dynamic relationships between agricultural mechanization, large-scale operation, and agricultural carbon emissions. Her bachelor's degree, master's degree and doctorate are all in finance. She has been working on agricultural development issues since her master's degree. Her doctoral thesis thoroughly analyzed topics related to agricultural mechanization and large-scale management. After completing her PhD in 2022, she began to focus on the relationship between agricultural development and climate change.
Lingyun Liu
Lingyun Liu is a professional teacher at NanJing XiaoZhuang University.
Kai Dong
Kai Dong is an associate professor at NanJing XiaoZhuang University.
Meng Xie
Meng Xie is a doctoral student at Nanjing Agricultural University.
Yingjie Du
Yingjie Du is an associate professor at Tianjin University of Science and Technology.
References
- Abdul Jabbar, S., Tul Qadar, L., Ghafoor, S., Rasheed, L., Sarfraz, Z., Sarfraz, A., Sarfraz, M., Felix, M., & Cherrez-Ojeda, I. (2022). Air quality, pollution and sustainability trends in South Asia: A Population-Based Study. International Journal of Environmental Research and Public Health, 19(12), 7534. https://doi.org/10.3390/ijerph19127534
- Acheampong, A. O., Amponsah, M., & Boateng, E. (2020). Does financial development mitigate carbon emissions? Evidence from heterogeneous financial economies. Energy Economics, 88, 104768. https://doi.org/10.1016/j.eneco.2020.104768
- Adewale Alola, A., Ozturk, I., & Bekun, F. V. (2021). Is clean energy prosperity and technological innovation rapidly mitigating sustainable energy-development deficit in selected sub-Saharan Africa? A myth or reality. Energy Policy, 158, 112520. https://doi.org/10.1016/j.enpol.2021.112520
- Amin, N., Song, H., & Khan, Z. A. (2022). Dynamic linkages of financial inclusion, modernization, and environmental sustainability in South Asia: A panel data analysis. Environmental Science and Pollution Research, 29(11), 16588–23. https://doi.org/10.1007/s11356-021-16648-7
- Ang, B. W. (1999). Is the energy intensity a less useful indicator than the carbon factor in the study of climate change? Energy Policy, 27(15), 943–946. https://doi.org/10.1016/S0301-4215(99)00084-1
- Balsalobre-Lorente, D., Driha, O. M., Bekun, F. V., & Osundina, O. A. (2019). Do agricultural activities induce carbon emissions? The BRICS experience. Environmental Science and Pollution Research, 26(24), 25218–25234. https://doi.org/10.1007/s11356-019-05737-3
- Bekhet, H. A., Matar, A., & Yasmin, T. (2017). CO2 emissions, energy consumption, economic growth, and financial development in GCC countries: Dynamic simultaneous equation models. Renewable and Sustainable Energy Reviews, 70, 117–132. https://doi.org/10.1016/j.rser.2016.11.089
- Belton, B., Win, M. T., Zhang, X., & Filipski, M. (2021). The rapid rise of agricultural mechanization in Myanmar. Food Policy, 9, 102095. https://doi.org/10.1016/j.foodpol.2021.102095
- Bilandžija, D., Zgorelec, Ž., & Kisić, I. (2016). Influence of tillage practices and crop type on soil CO2 emissions, sustainability. Sustainability, 8(1), 90. https://doi.org/10.3390/su8010090
- Brown, A., Dayal, A., & Rumbaitis Del Rio, C. (2012). From practice to theory: Emerging lessons from Asia for building urban climate change resilience. Environment and Urbanization, 24(2), 531–556. https://doi.org/10.1177/0956247812456490
- Chen, Y. B., & Wang, S. (2022). Rural labor outflow, agricultural scale management, and agricultural carbon emissions. Economy and Management, 3(6), 43–49. https://kns.cnki.net/kcms/detail/13.1032.F.20221019.2140.008.html
- Dar, J. A., & Asif, M. (2019). Do agriculture-based economies mitigate CO 2 emissions? International Journal of Energy Sector Management, 14(3), 638–652. https://doi.org/10.1108/IJESM-01-2019-0011
- Dubey, A., & Lal, R. (2009). Carbon footprint and sustainability of agricultural production systems in Punjab, India, and Ohio, USA. Journal of Crop Improvement, 23(4), 332–350. https://doi.org/10.1080/15427520902969906
- Fais, B., Sabio, N., & Strachan, N. (2016). The critical role of the industrial sector in reaching long-term emission reduction, energy efficiency and renewable targets. Applied Energy, 162, 699–712. https://doi.org/10.1016/j.apenergy.2015.10.112
- Gerlagh, R., & Lise, W. (2005). Carbon taxes: A drop in the ocean, or a drop that erodes the stone? The effect of carbon taxes on technological change. Ecological Economics, 54(2–3), 241–260. https://doi.org/10.1016/j.ecolecon.2004.12.037
- Gokmenoglu, K. K., & Taspinar, N. (2018). Testing the agriculture-induced EKC hypothesis: The case of Pakistan. Environmental Science and Pollution Research, 25(23), 22829–22841. https://doi.org/10.1007/s11356-018-2330-6
- Grossman, G. M., & Krueger, A. B. (1995). Economic growth and the environment*. The Quarterly Journal of Economics, 110(2), 353–377. https://doi.org/10.2307/2118443
- He, Q., Zhang, J. B., & Zhang, H. (2023). The impact of agricultural mechanization on agricultural carbon emissions: Evidence from major grain-producing areas. Statistics & Decision, 39(1), 88–92. https://doi.org/10.13546/j.cnki.tjyjc.2023.01.016
- Hou, M. Y., & Yao, S. B. (2018). Measurement and temporal-spatial dynamic evolution of urban eco-efficiency in China. China Population, Resources & Environment, 28(3), 13–21. https://kns.cnki.net/kcms2/article/abstract?v=ClyI0LvUrM8vMp7sz8bBmCiYdVyPG5rty8nb0QnWROerJrSp1z97bCiwQaeEcJrFmV8imD6YtlZhgoW3EcYB32CKMT7ZJIwy5fvpwqmdhU3pLPWgKuz9k3XONE2jVz24PBms7pR4WGo=&uniplatform=NZKPT&language=CHS
- Jahanger, A., Usman, M., Murshed, M., Mahmood, H., & Balsalobre-Lorente, D. (2022). The linkages between natural resources, human capital, globalization, economic growth, financial development, and ecological footprint: The moderating role of technological innovations. Resources Policy, 76, 102569. https://doi.org/10.1016/j.resourpol.2022.102569
- Jiang, J., Zhao, T., & Wang, J. (2021). Decoupling analysis and scenario prediction of agricultural CO2 emissions: An empirical analysis of 30 provinces in China. Journal of Cleaner Production, 320, 128798. https://doi.org/10.1016/j.jclepro.2021.128798
- Kalantari, F., Mohd Tahir, O., Mahmoudi Lahijani, A., & Kalantari, S. (2017). A review of vertical farming technology: A guide for implementation of building integrated agriculture in Cities. Advanced Engineering Forum, 24, 76–91. https://doi.org/10.4028/www.scientific.net/AEF.24.76
- Kim, D.-H., Hsieh, J., & Lin, S.-C. (2021). Financial liberalization, political institutions, and income inequality. Empirical Economics, 60(3), 1245–1281. https://doi.org/10.1007/s00181-019-01808-z
- Kim, D.-H., Wu, Y.-C., & Lin, S.-C. (2020). Carbon dioxide emissions and the finance curse. Energy Economics, 88, 104788. https://doi.org/10.1016/j.eneco.2020.104788
- Kumbaroğlu, G., Karali, N., & Arıkan, Y. (2008). CO2, GDP and RET: An aggregate economic equilibrium analysis for Turkey. Energy Policy, 36(7), 2694–2708. https://doi.org/10.1016/j.enpol.2008.03.026
- Kurniawan, R., & Managi, S. (2018). Coal consumption, urbanization, and trade openness linkage in Indonesia. Energy Policy, 121, 576–583. https://doi.org/10.1016/j.enpol.2018.07.023
- Lal, R. (2015). Restoring soil quality to mitigate soil degradation. Sustainability, 7(5), 5875–5895. https://doi.org/10.3390/su7055875
- Lanoie, P., Laplante, B. T., & Roy, M. (1998). Can capital markets create incentives for pollution control? Ecological Economics, 26(1), 31–41. https://doi.org/10.1016/S0921-8009(97)00057-8
- Li, D. L. (2022). Research on the impact of scale operation of farmland on the development of geographical indication agriculture: Based on the survey of six counties in Shaanxi province and Shandong province. China Land Science, 36(1), 78–87. https://kns.cnki.net/kcms2/article/abstract?v=ClyI0LvUrM_JZqoC_KIPxA045GXmJC0w4FLrjpf47jvupTMt6i3dm1cKIx8SvvT-4VgxM5GTmR0TTAZoaDMWKeOAUDM59Neb7HLJOFm8yx7vQwwwYLa96Z7QvnSEgF8xlLag47g6ies=&uniplatform=NZKPT&language=CHS
- Li, X. (2014). Analysis and countermeasures on the relation of China’s carbon emission and industrial structure. Journal of Industrial Technological Economics, 33(1), 154–160. https://kns.cnki.net/kcms2/article/abstract?v=ClyI0LvUrM9yn7AiL6gsuvO2y340d1mWkhr3jmooEL6Yy6zdW0-VOTPfucf6WLfmu1EwoqDSTX6qgyw2WDGX2z_V1he0a3K9sBiKr_3im44hVxPXsgcL94z15HyVGrVv&uniplatform=NZKPT&language=CHS
- Li, G. C., Li, Y. Y., & Zhou, X. S. (2018). Agricultural mechanization, labor transfer and farmers’ income growth: The cause and the effect. Chinese Rural Economy, 407(11), 112–127. https://kns.cnki.net/kcms2/article/abstract?v=ClyI0LvUrM_UkWGNxSJ6Lgh2XX8du_hKNu8h893aacBM6XFS5TtpXELMh-V0X8o05JVbNwtnQnkftKS94S9PHl2IpnTKe_zD414Uala7fBLTQPHQGu3ZUmyeU4iAsXZ5ChVK8phlI4Q=&uniplatform=NZKPT&language=CHS
- Lin, L. F., Li, R., & Chen, C. B. (2020). From pollution “refuge” to green “main battlefield”: 70 years of rural environmental governance in China. Journal of Arid Land Resources and Environment, 34(7), 30–36. https://doi.org/10.13448/j.cnki.jalre.2020.180
- Liu, X., & Bae, J. (2018). Urbanization and industrialization impact of CO2 emissions in China. Journal of Cleaner Production, 172, 178–186. https://doi.org/10.1016/j.jclepro.2017.10.156
- Liu, D. P., Liu, Y., & Chen, S. (2019). Analysis of the influence of land transfer and scale management on the agricultural social service demand-Based on the survey of large rice grower in jianghan plain. Chinese Journal of Agricultural Resources and Regional Planning, 40(1), 170–176. https://kns.cnki.net/kcms2/article/abstract?v=ClyI0LvUrM9WetxkBp84f-bqLqQzSROnvWEQ9C-PNVpv65KLjP8SUQmZ_Qjk-zmm3U4UXFrHCMTMfuMcO9bxkHQUs1Q1V533anblPLh3qrgRF__XhCtRh7QHscHXrMz7QJkMtkz0Hho=&uniplatform=NZKPT&language=CHS
- Li, S., Zhou, C., & Wang, S. (2019). Does modernization affect carbon dioxide emissions? A panel data analysis. Science of the Total Environment, 663, 426–435. https://doi.org/10.1016/j.scitotenv.2019.01.373
- Mielnik, O., & Goldemberg, J. (1999). Communication the evolution of the “carbonization index” in developing countries. Energy Policy, 27(5), 307–308. https://doi.org/10.1016/S0301-4215(99)00018-X
- Mohareb, E., & Kennedy, C. (2012). Greenhouse gas emission scenario modeling for cities using the PURGE model. Journal of Industrial Ecology, 16(6), 875–888. https://doi.org/10.1111/j.1530-9290.2012.00563.x
- Niu, H. L., & Jiang, K. S. (2013). Carbon emission effect of industrial structure adjustment in China: Empirical research based on extended STIRPAT model and spatial panel data. Journal of Technology Economics, 32(8), 53–62. https://kns.cnki.net/kcms2/article/abstract?v=ClyI0LvUrM_Xs-t67tBx4Qgs1sU7R2NO9W78ZM_iKoNyxaMMykfjm4IZsdi8RG4Sgh0KYn7I2N8_sbA6sW5rSkqrKR7y_yBIna94uC-69TEKIV8EkbTF8V2nCRI9Cn1k&uniplatform=NZKPT&language=CHS
- Ntim-Amo, G., Qi, Y., Ankrah-Kwarko, E., Ankrah Twumasi, M., Ansah, S., Boateng Kissiwa, L., & Ruiping, R. (2022). Investigating the validity of the agricultural-induced environmental Kuznets curve (EKC) hypothesis for Ghana: Evidence from an autoregressive distributed lag (ARDL) approach with a structural break. Management of Environmental Quality: An International Journal, 33(2), 494–526. https://doi.org/10.1108/MEQ-05-2021-0109
- Omri, A., Daly, S., Rault, C., & Chaibi, A. (2015). Financial development, environmental quality, trade and economic growth: What causes what in MENA countries. Energy Economics, 48, 242–252. https://doi.org/10.1016/j.eneco.2015.01.008
- Ozturk, I., & Acaravci, A. (2013). The long-run and causal analysis of energy, growth, openness and financial development on carbon emissions in Turkey. Energy Economics, 36, 262–267. https://doi.org/10.1016/j.eneco.2012.08.025
- Porter, M. E., & van der Linde, C. (1995). Toward a new conception of the environment-competitiveness relationship. J Journal of Economic Perspectives, 9(4), 97–118. https://doi.org/10.1257/jep.9.4.97
- Rafiq, S., Salim, R., & Apergis, N. (2016). Agriculture, trade openness and emissions: An empirical analysis and policy options. Australian Journal of Agricultural and Resource Economics, 60(3), 348–365. https://doi.org/10.1111/1467-8489.12131
- Rajkhowa, A., Barman, I., Das, P. K., Deka, S. D., & Sonowal, A. (2020). An analysis of extent of farm mechanization in north bank plains agro-climatic zone of Assam. Asian Journal of Agricultural Extension, Economics & Sociology, 11, 81–90. https://doi.org/10.9734/ajaees/2020/v38i1130455
- Ramanathan, R. (2002). Combining indicators of energy consumption and CO2 emissions: A cross-country comparison. International Journal of Global Energy Issues, 17, 214–227. https://doi.org/10.1504/IJGEI.2002.000941
- Rehman, A., Luan, J., Khatoon, R., Hussain, I., & Iqbal, M. S. J. L. S. J. (2017). Modern agricultural technology adoption its importance, role and usage for the improvement of agriculture. 14(2), 70–74. https://doi.org/10.7537/marslsj140217.10
- Rehman, A., Ma, H., Ahmad, M., Ozturk, I., & Chishti, M. Z. (2021). How do climatic change, cereal crops and livestock production interact with carbon emissions? Updated evidence from China. Environmental Science and Pollution Research, 28(24), 30702–30713. https://doi.org/10.1007/s11356-021-12948-0
- Rej, S., Bandyopadhyay, A., Mahmood, H., Murshed, M., & Mahmud, S. (2022). The role of liquefied petroleum gas in decarbonizing India: Fresh evidence from wavelet–partial wavelet coherence approach. Environmental Science and Pollution Research, 29(24), 35862–35883. https://doi.org/10.1007/s11356-021-17471-w
- Sadorsky, P. (2010). The impact of financial development on energy consumption in emerging economies. Energy Policy, 38(5), 2528–2535. https://doi.org/10.1016/j.enpol.2009.12.048
- Shahbaz, M., Shahzad, S. J. H., Ahmad, N., & Alam, S. (2016). Financial development and environmental quality: The way forward. Energy Policy, 98, 353–364. https://doi.org/10.1016/j.enpol.2016.09.002
- Shunsuke, M., Akira, H., & Tetsuya, T. (2008). Does trade liberalization reduce pollution emissions? Discussion paper, Research Institute of Economy, Trade and Industry (RIETI), 08013. https://ideas.repec.org/p/eti/dpaper/08013.html
- Slamet, N., Dargusch, P., Wadley, D., & Aziz, A. J. J. O. E. I. L. (2020). Carbon emission from dredging activities in land reclamation developments: The case of Jakarta Bay. Journal of Environmental Informatics Letters, 3(2), 59–69. https://doi.org/10.3808/jeil.202000034
- Sun, J. W. (2005). The decrease of CO2 emission intensity is decarbonization at national and global levels. Energy Policy, 33(8), 975–978. https://doi.org/10.1016/j.enpol.2003.10.023
- Tamazian, A., & Bhaskara Rao, B. (2010). Do economic, financial and institutional developments matter for environmental degradation? Evidence from transitional economies. Energy Economics, 32(1), 137–145. https://doi.org/10.1016/j.eneco.2009.04.004
- Tol, R. S., Pacala, S. W., & Socolow, R. (2006). Understanding long-term energy use and carbon dioxide emissions in the USA. SSRN Electronic Journal. https://doi.org/10.2139/ssrn.927741
- Wang, L., Vo, X. V., Shahbaz, M., & Ak, A. (2020). Globalization and carbon emissions: Is there any role of agriculture value-added, financial development, and natural resource rent in the aftermath of COP21? Journal of Environmental Management, 268, 110712. https://doi.org/10.1016/j.jenvman.2020.110712
- Wei, M. S., Yan, T. W., & Luo, S. X. (2023). The impacts of scale management and technological progress on green and low-carbon development of agriculture: A quasi-natural experiment based on the establishment of major grain-producing areas. Chinese Rural Economy, 458(2), 41–65. https://doi.org/10.20077/j.cnki.11-1262/f.2023.02.003
- Xu, L., Fan, M., Yang, L., & Shao, S. (2021). Heterogeneous green innovations and carbon emission performance: Evidence at China’s city level. Energy Economics, 99, 105269. https://doi.org/10.1016/j.eneco.2021.105269
- Zeqiraj, V., Sohag, K., & Soytas, U. (2020). Stock market development and low-carbon economy: The role of innovation and renewable energy. Energy Economics, 91, 104908. https://doi.org/10.1016/j.eneco.2020.104908
- Zhou, P., Ang, B. W., & Poh, K. L. (2008). Measuring environmental performance under different environmental DEA technologies. Energy Economics, 30(1), 1–14. https://doi.org/10.1016/j.eneco.2006.05.001
- Zhou, J. H., Tang, L. Q., & Li, K. (2015). Research progress on adapting agricultural production transformation to climate change. China Rural Survey, 123(3), 74–86+97. https://kns.cnki.net/kcms2/article/abstract?v=ClyI0LvUrM8sStnbuF8anVCPV64iw2vAXI0VEuF4jRUIGbBlFuQL3s8PvnSVkqJn1pin1q_hYJ3O4m9CtqYTpd1bWoqQrPOVY9ls7hGT86vWlnZ3Ttn9ZcLY8rrZ9-jRO9lgIxJC4b8=&uniplatform=NZKPT&language=CHS
- Zhou, Z., Zhang, C., Peng, C., & Kong, X. Z. (2016). Agricultural mechanization and farmers’ income: Evidence from subsidy policies for purchasing farm machinery and tools. Chinese Rural Economy, 374(2), 68–82. https://kns.cnki.net/kcms2/article/abstract?v=ClyI0LvUrM8ol2dFNl-GqwGXu-JlTyUdRTe6P9t03kYpVqm2fqbg0pUtHxPN6iUWPcuNqBs-c9crkikk57N7E2u1Y3VrpJjh4n0w1XbUMFLmwgfeflq16X0vVjSCo0EDo7tK6XZef4k=&uniplatform=NZKPT&language=CHS
- Zhu, W., & Wang, R. M. (2023). Study on the impact of technological progress and operation scale on agricultural carbon emissions. Agricultural Economy, 2(12), 13–15. https://doi.org/10.3390/en15124464