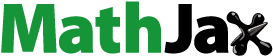
Abstract
Large-scale farming is an important way for sustainable development of pig industry. In this regard, the digital economy has an important influence on the industrial structure and economic development, but whether it is conducive to large-scale pig farming (LSPF) needs further study. This study assesses the development level of the digital economy in 31 provinces of China and examines the transmission mechanism of the digital economy in LSPF. The results demonstrate that the digital economy can contribute both directly and indirectly to the LSPF through improved technological innovation, enhanced merchandise sales, and reduced labor costs of raising pigs. More importantly, the digital economy has a significant negative spatial spillover effect on LSPF; that is, it is not conducive to large-scale farming in neighboring localities. The results of the heterogeneity analysis further show that the digital economy is more significant for regions that have been identified as LSPF key and potential growth areas. The findings provide a reference for policymakers and professionals in emerging economies to facilitate the scale-up and sustainability of the pig industry.
1. Introduction
Pork is the most consumed meat in the world (Research & Markets, Citation2020; Lin-Schilstra et al., Citation2022). While rising incomes and urbanization drive global pork consumption (Milford et al., Citation2019), better farm management practices and efficient logistics provide the impetus for higher quantity of pork production. Although the consumption of pork in Europe and North America is the highest it has ever been, half of the world’s pork is produced and consumed in Asia, especially in China (Qiao et al., Citation2016; Yao et al., Citation2022). The United States Department of Agriculture’s Livestock and Poultry: World Market and TradeFootnote1 report states that in 2021 the global pork production was 107.77 million tons, while China’s pork production was 47.50 million tons, which accounted for about 44.07% of the total production. China’s pork imports have ranked first in the world for three consecutive years, reaching 4.33 million tons in 2021, that is, 37.22% of the world’s pork imports (approximately 11.63 million tons). Undoubtedly, China has become one of the most important players in the world’s pork-related industries.
At present, small and medium-scale farmers produce about 90% of China’s farms pigs within the country (Yao et al., Citation2022). However, these farmers are yet to catch up with the technical, land, and feed management of large-scale farmers. This discrepancy hinders the competitiveness and sustainability of China’s pig farming industry, and highlights the industry’s vulnerability and lack of sustainability under competitive pressures from external risks (Xu et al., Citation2022). For example, the spread of African swine fever in China in late 2018 led directly to the mandatory culling of 1.2 million pigs in 2019, with even heavier losses for small-scale pig farmers (Yao et al., Citation2022; Zhao et al., Citation2019). So, how does one improve the level of large-scale farming in China’s pig industry and enhance sustainable development?
The digital economy is a series of economic activities, with information technology at its core and modern networks as its carrier, that aims to efficiently apply digital technology to improve the economic structure (Pan et al., Citation2022; Paradise, Citation2019). The advancement of the digital economy has had a great influence on the structure of pork production globally (Groher et al., Citation2020). The integration of the digital economy and production activities has already begun to transform the pig farming industry in terms of redistribution of production factors, improvement of production efficiency, and optimization of operational models (Neethirajan & Kemp, Citation2021). Ultimately, it has provided new options for transforming and upgrading the pig farming industry in China. The application of the digital economy in the pig farming industry entails rational data planning and effective use of data in pig production (Bao & Xie, Citation2022), or put simply, to use resources rationally and match resources effectively for each link in the production cycle (Tzanidakis et al., Citation2021). At the same time, new-generation digital technologies such as mobile Internet, Internet of Things, cloud computing, and big data are also improving animal welfare and promoting sustainable development in the pig industry (Bao & Xie, Citation2022; Collins & Smith, Citation2022; Tzanidakis et al., Citation2021).
Within academia, most literature focuses on the effect of the digital economy on macroeconomic development (Ding et al., Citation2021), carbon emissions (Zhang et al., Citation2022), technology innovation (Sorescu & Schreier, Citation2021), tourism (Hojeghan & Esfangareh, Citation2011), urban development (Zhu & Chen, Citation2022), productive efficiency (Pan et al., Citation2022; Watanabe et al., Citation2018), which greatly drives the boundaries of digital economy research. Existing studies on the digital economy and farming industry have focused on the application of digital technologies in the farming industry. The rapid growth of the world population has increased the demand for higher quantities of food, and the traditional breeding model can hardly guarantee the human demand for meat (Neethirajan & Kemp, Citation2021). With the arrival of Industry 4.0, the digital economy has taken the world stage, and digital technology is widely used in agricultural production (Williams, Citation2021). The development of the digital economy has dramatically changed the breeding model (Neethirajan, Citation2017) and the commodity transaction methods (Knudsen et al., Citation2021). In this context, big data can help farmers monitor large populations of animals for health and welfare, detect issues with individual animals promptly, and use data analysis to anticipate issues even before they occur (Benjamin & Yik, Citation2019). For example, data monitoring systems can detect cow behavior around the clock, detect pig screams, monitor coughs in multiple species to identify animal respiratory diseases, and identify pregnant cows through body temperature changes (Neethirajan, Citation2017); these technological advancements have already improved animal health and welfare. The development of the digital economy has dramatically changed the commodity transaction methods and business operation modes (Knudsen et al., Citation2021). The e-commerce model, for instance, has enabled more farmers to use the Internet to provide electronic economic transactions, including products, services, and interactions with partners and customers (Hojeghan & Esfangareh, Citation2011; Kablana & Chhikara, Citation2013). The application of these technologies is critical to the scale-up and sustainability of the farming industry.
Although there is scholarly interest in the application of digital technologies in the farming industry, there are limited studies that quantify the effect of the digital economy on the development of large-scale farming. At present, as most countries transform their traditional economies into digital economies, exploring the relationship between the digital economy and the farming industry has become a valuable research topic (Miao, Citation2021).
To explore the impact of the digital economy on the LSPF, this study first uses the entropy method to measure the level of development of the digital economy in each province of China. It employs the ordinary least squares (OLS) method to examine the linear impact of the digital economy on large-scale pig farming (LSPF). Secondly, with the development of the digital economy, the free flow of production factors makes inter-regional industrial development correlated, thus creating a “spatial spillover effect.” Whether the digital economy has a spatial spillover effect on the LSPF? To test the digital economy’s spatial impact on the pig industry’s development, we also adopt the spatial Durbin model (SDM) to analyze the spatial spillover effect of the digital economy on LSPF. Thirdly, to make the model estimates more accurate, we used the robustness tests and endogeneity analysis to explain whether the model is reliable. Finally, the mediation role of merchandise sales, labor costs, and technological innovation between the digital economy and LSPF was assessed, and the effect of the digital economy on LSPF in each of the four types of pig farming regions in China was investigated. This analytical strategy can explain the contribution of the digital economy to the development of LSPF more clearly, and the conclusions drawn may be a valuable reference for the digital transformation and development of the breeding industry in China and other countries around the world.
The major contributions of this study are as follows: (1) Research topic. Differing from existing research, this study provides new empirical evidence for investigations related to the digital economy and farming industry from the perspective of the digital economy empowering the development of LSPF. The findings not only fill the research gaps in this area of study, but also provide useful references for policymakers and emerging economies. (2) This study makes full use of 21 newly recorded data from the National Bureau of Statistics of China, Peking University, and Ali Research Institute to construct an evaluation system of China’s digital economy. It makes our calculated results of China’s provincial-level digital economy development more accurate. (3) Finally, this study provides multi-level empirical analysis perspectives and improves the robustness of the research results. It not only discusses the linear effect of the digital economy on LSPF, but also analyzes its mechanism, spatial spillover effect, and heterogeneity. Furthermore, we innovatively use the length of fiber optic cable lines as an instrumental variable for the robustness test, and thus, ensure the robustness of the regression results.
2. Research hypothesis
We demonstrate the direct and indirect effects and spatial spillover effects of the digital economy on LSPF. Figure graphically represents our research framework and hypotheses.
2.1. The influence of the digital economy on LSPF
The digital economy affects all aspects of society, including the breeding industry (Kuznetsov et al., Citation2018). The development of LSPF is closely related to the digital economy (Groher et al., Citation2020) in that it equips agricultural producers with new high-performance equipment and modern management tools, computer monitoring, satellite navigation tools, fuel consumption control, and load optimization (Kuznetsov et al., Citation2018). This can effectively improve the efficiency with which farms are managed. Moreover, the digital economy can help modern farms optimize the economic contribution per animal, reduce the drudgery of repetitive tasks, and overcome fewer effective problems to increase the scale-up potential of farms (Neethirajan & Kemp, Citation2021).
The development of the digital economy is conducive to eliminating the obstacles to communication between various industries and departments, helping the breeding industry to collaborate with other industries, reducing development costs and expanding the scale of the breeding industry (C. R. Eastwood et al., Citation2021). Without highly sophisticated t equipment and the means to quickly process big data, it is hard to imagine how much the efficiency of agricultural production in these areas would be reduced (Vorotnikov et al., Citation2020). Therefore, we hypothesize:
Hypothesis 1 (H1):
The digital economy can promote the development of LSPF.
2.2. Spatial spillover effect of the digital economy on LSPF
An important feature of the digital economy is that it compresses the space-time distance and enhances the breadth and depth of inter-regional economic activities through efficient information transmission (Zhang et al., Citation2022), affecting the surrounding areas (Mossberger et al., Citation2022). The digital economy breaks the limitations of geographical distance (J. Li et al., Citation2020; Sorescu & Schreier, Citation2021), and promotes information flow and interactions across time and space between cities and regions, which can generate spatial spillover effects (Ma & Zhu, Citation2022). Moreover, information and communication technologies can rapidly expand to different industries (Giusti et al., Citation2020), and effectively guide the division of labor and cooperation across regions (Blut et al., Citation2021), thereby raising the speed of capital, technology, and labor mobility, which can influence the development of pig farming in neighboring regions. This fact leads to the following hypothesis.
Hypothesis 2 (H2):
The impact of the digital economy on LSPF has a spatial spillover effect.
2.3. Mediation effect of the digital economy on LSPF
In addition to directly affecting LSPF, the digital economy can also indirectly affect LSPF by promoting technology innovation, raising merchandise sales, and reducing labor costs.
Technology innovation. The development of the digital economy is conducive to the dissemination of knowledge; it improves the technological innovation capabilities of enterprises (Tang et al., Citation2021), promotes the optimal allocation of resources and industrial structure updation (Ren et al., Citation2021), and reduces barriers to large-scale production. It has brought global agriculture into the era of precision farming (C. Eastwood et al., Citation2017). Blockchain technology, for example, secures and guarantees the traceability of animal products from farm to table and the application of technologies such as big data, while detectors can monitor disease outbreaks and prevent related economic losses and food-related health pandemics (Neethirajan & Kemp, Citation2021; Rosendal & Olesen, Citation2022). These technologies can improve breeding efficiency and drive the large-scale development of the livestock industry.
Merchandise sales. The digital economy has changed the products and modes of regional trade (K. J. Li et al., Citation2020; Pradhan et al., Citation2019), and enhanced the market transaction efficiency (Y. Chen, Citation2020). For example, e-commerce platforms utilize advanced big data management, artificial intelligence, and product push systems to quickly analyze consumer consumption preferences and optimize product marketing strategies, thereby augmenting the purchase probability and stimulating commodity transactions (J. Li et al., Citation2020), especially for basic household goods and food consumption. The increase in sales and demand for these commodities will further stimulate companies to expand their production scale. Likewise, with the widespread application of the digital economy to the whole industry chain of pig breeding, pork processing, pork product promotion, and pork consumption, new business models have further raised the demand and consumption of pork products, and promoted the expansion of the pig breeding industry.
Labor costs. ICTs are aimed at minimizing resource consumption, which is manifested by the possibility of saving production resources, including labor, and more accurate control over the flow of material and technical resources (Neethirajan & Kemp, Citation2021). Driven by big data, artificial intelligence, and other digital technologies, the digital economy can improve the operational efficiency of pig farms and reduce labor costs, including information acquisition costs (Chen, Citation2022). The reduction of labor costs is conducive to the expansion of pig farms and the strengthening of their competitive advantage (Vorotnikov et al., Citation2020). Based on these aspects, the following hypothesis is proposed:
Hypothesis
3 (H3): The digital economy can have a positive effect on LSPF by improving technology innovation, raising merchandise sales, and reducing labor costs.
3. Variables selection and methodology
3.1. Variables selection
3.1.1. Explained variables
According to the records and definitions in the China Animal Husbandry and Veterinary Statistical Yearbook (https://data.cnki.net/yearBook/single?id=N2023030190), the standard for LSPF is a farm that raises more than 500 pigs per year. Therefore, this study uses the ratio of the number of farms raising more than 500 pigs to all pig farms, to represent the development level of LSPF. The data are mainly from the China Animal Husbandry and Veterinary Statistical Yearbook of each year in China (2011–2018).
3.1.2. Explanatory variable
Owing to the resource endowment and data availability limitations of the study area, it was difficult to form a uniform standard for the measurement of the digital economy (Zhang et al., Citation2022; Zou & Deng, Citation2022). Some scholars measure the level of digital economy development through Internet development, digital financial inclusion, and digital economy infrastructure (Z. Li & Wang, Citation2022; J. Li et al., Citation2022; Pan et al., Citation2022; Zhang et al., Citation2022). However, the indicators of different dimensions of digital economy development should contain useful information on the development of the digital economy, considering only one or a certain level of indicators can lead to a lopsided understanding of the digital economy (Pan et al., Citation2021). The measurement of the digital economy should not only reflect the integration of ICT, enterprise, and social development, but also focus on the construction of digital economy infrastructure and the level of governance. Therefore, this study measures the level of digital economy development through the dimensions of digital economy infrastructure, digital industrialization, industry digitization, and digital governance (Pan et al., Citation2021).
The digital economy infrastructure is a pre-condition for the development of the digital economy, and it includes the number of Internet domain names, the Internet broadband access port, the mobile phone penetration rate, the length of fiber optic cable line, the mobile telephone switching system capacity, the number of computer users, and the Internet broadband access users.
Digital industrialization refers to the added value of the information industry characterized by digital technology, including digital technology innovation and digital industrial production. It mainly includes the electronic information manufacturing industry, ICT industry, software service industry, and Internet-related industries (Pan et al., Citation2021). This study uses the total amount of telecommunication services, the output value of information services, number of people in the information service industry, and software business revenue to measure digital industrialization.
Industry digitization is the result of the convergence of industry and digitization technologies, which encompasses the number of corporate-owned websites, number of couriers, digital financial inclusion index, and e-commerce transaction volume.
Digital governance originates from data governance, which is an important guarantee for the stable and orderly development of the digital economy. The level of digital governance is measured by selecting indicators such as the years of education per capita, number of patents granted, technology market turnover, number of R&D projects in industrial enterprises above the designated size, R&D expenditures of industrial enterprises above the designated size, and the full-time equivalent of R&D staff in industrial enterprises above the designated size.
The digital financial index measure is the Peking University Digital Financial Inclusion Index of China (PKU_DFIIC), maintained by the Institute of Digital Finance at Peking University (IDF-PKU).Footnote2 The rest of the data are obtained from the China Statistical Yearbook for each year (2011–2018), and any individual vacant data were filled by the linear interpolation method. The digital economic index system is established as shown in Table .
Table 1. The digital economic index system
The entropy weight method is a branch of information theory (J. Li et al., Citation2022). Entropy weight can indicate useful information provided by the index, and it is a relatively objective measurement method (H. Xu et al., Citation2018). This study mainly adopts the entropy weight method to calculate the digital economy level of 31 provinces in China. We calculate the entropy weight method based on J. Li et al. (Citation2022), Sahoo et al. (Citation2017), H. Xu et al. (Citation2018) and the measure result of digital economy in Appendix A1.
3.1.3. Mediating variables
Based on the hypothesis mentioned in section 2, this study selects merchandise sales, labor costs, and technology innovation as the mediating variable. The merchandise sales are denoted by the total retail sales of social commodities, labor costs are denoted by the average labor cost per pig, and technological innovation is represented by the number of patents granted per capita in the region (Tang et al., Citation2021). We mainly use the 31 Chinese provinces statistic data from 2011 to 2018, and the data on total retail sales of social commodities and patents granted per capita are obtained from the National Bureau of Statistics of China (National Bureau of Statistics of China, Citation2022), and the average labor cost per pig is obtained from the Compilation of National Agricultural Cost-Benefit Information (https://data.cnki.net/yearBook/single?id=N2022100031).
3.1.4. Control variables
Other variables also have a greater effect on LSPF. Among them, the endowment of breeding resources and economic development conditions have a major effect on large-scale breeding (Shi et al., Citation2022; Sun et al., Citation2022; Zhen et al., Citation2020). Hence, the control variables are analyzed from two aspects: pig breeding resource endowment and economic development level. Pig breeding resource endowment includes feed sources (annual production of corn and soybeans), total road mileage, financial payments for agriculture, and the proportion of people with higher education (Sun et al., Citation2022; Zhen et al., Citation2020). Economic development level incorporates urbanization rate, industrial structure, number of banking institutions, livestock economic development level, and urban and rural residents’ consumption level (Mikayilov et al., Citation2018; Zhen et al., Citation2020). The data are mainly from the National Bureau of Statistics of China (https://data.stats.gov.cn/english/) and CNRD databases (https://www.cnrds.com/) from 2011 to 2018. Table presents the descriptive statistics.
Table 2. Descriptive statistics of variables
4. Methodology
4.1. Static panel model
To examine the effect of the digital economy on LSPF (H1), this study establishes the following static panel regression model:
where is the development level of pig farming on a large scale;
is the development level of the digital economy in province i at t year;
represents a series of control variables;
is the province fixed effect;
is time fixed effect;
is the random error term, i is province, t is time.
4.2. Spatial effect
To meet the spatial econometric needs of the panel data and ensure that the spatial parameter estimations reveal the dynamic transfer and path conduction for the digital economy spillover effect on LSPF over time, it is necessary to construct the spatial weight matrix (Dubé et al., Citation2014).
4.2.1. Spatial weight matrix
The spatial weight matrix is the basis for measuring horizontal digital economy spillover relationships among regions (Liu et al., Citation2022). According to the first law of geography, all things are related to each other, and those things that are close to each other are more closely related (Tobler, Citation1970). Therefore, the digital economy spillover is influenced by the distance between two regional administrative centers and is inversely related, which means that the closer the two places are, the stronger the spatial association, and the further the two places are, the weaker the association. This study constructs the spatial weight matrix (2), based on the geographical distance relationship, using a geographical inverse distance method, after which the matrix was subjected to random row normalization (3).
where denotes each element of the geographic distance weight matrix representing the spatial interactions between provinces,
denotes the distance between province i and province j, calculated from the latitude and longitude coordinates of the corresponding provincial capital city.
4.2.2. Spatial correlation test
The spatial effect is a long-term process (P. Chen et al., Citation2021), and the effect of the development of the digital economy on LSPF may also have a certain time lag in space, but the spatial weight matrix based on geographic distance cannot effectively distinguish spatial correlations over time. Moran’s index is usually used to measure the spatial spillover effect period changes (Ping et al., Citation2004). Therefore, we use Moran’s index to test the spatial correction between the digital economy and LSPF with Equationequation (4)(4)
(4) :
where is the global Moran’s index,
and
are the level of LSPF and digital economy in province i and province j,
is the average level of LSPF or digital economy,
represent the elements in row i and column j of the spatial weight matrix, n is the number of provinces included in this research samples,
is the sample variance. The value range of Moran’s index is [−1, 1], and when its value is [0,1], it indicates that the variables are positively correlated in space, and there is a phenomenon of spatial aggregation. Similarly, the value [−1,0] indicate that the variable has a negative spatial correlation, and when the value is close to 0, the variable is randomly distributed.
4.2.3. Spatial panel model
As an extended model, the SDM, with the spatial lag model (SLM) and spatial error model (SEM), considers both the characteristics of variables’ interdependence among regions and the spatial spillover effects and, therefore, should be given priority in empirical analysis (Pace & LeSage, Citation2009). In the same vein, like Pace and LeSage (Citation2009) and X. Chen et al. (Citation2022), we construct the following model:
where the is the spatial panel weight matrix;
and
are the spatial autoregression coefficient and the spatial autocorrelation coefficient, respectively;
denotes control variables;
is the spatial error autocorrelation term; θ is the random perturbation term; when
is not zero, but θ and δ are zero, the model is SLM; when ρ and
are zero, but δ is not zero, the model is SEM; when ρ and θ are not zero, but δ is zero, the model is SDM.
The existence of spatial lag, for both the explained and explanatory variables in the SDM, can bias the coefficient estimates. Pace and LeSage (Citation2009), therefore, treated the SDM to partial differentiation. The partial differential matrix of the explained variable (Y) with respect to the explanatory variable (Xr) at a given moment can be transformed as in Equationequation (6)(6)
(6) :
The mean of the diagonal elements of the matrix at the far right of the formula is the direct effect, thereby indicating the effect of the explanatory variable on the explained variable in the region. The mean of the non-diagonal elements is the indirect effect.
4.3. Mediation effect model
To examine H3, we reference J. Xu and Yu (Citation2022) to establish the mediation effect analysis framework. To investigate the effect of the digital economy on LSPF, the following mediation effect test model was established:
where is a mediating variable,
is the development level of digital economy;
represents a series of control variables;
is the province fixed effect;
is time fixed effect;
is the random error term, i is province, and t is time.
5. Results and discussion
5.1. Baseline estimation results
In this study, after conducting the Hausman test, the results show p-value less than 0.05, thus indicating that the model rejects the hypothesis of the random effect and therefore the fixed effects model is chosen for estimation.
Table presents the OLS estimation results, where columns (1) and (2) are the OLS estimation results of the two-way fixed effects before and after adding the control variables, respectively, and column (3) is the OLS estimation of the two-way fixed effects with the mediating variables. All the results establish that the digital economy has a significant positive effect on LSPF, and the R-squared values also increase with the addition of control variables; this finding is consistent with Hypothesis 1. From columns (3) of Table , we can see that for each unit increase in the digital economy, the proportion of LSPF will increase by 0.033. Pig farming is not only a critical Chinese agricultural production sector, but it is also one of the most consumed meat products by the population. Likewise, China is actively developing its digital economy. According to the results of the study, the development of the digital economy can significantly increase the rate of scale development in China’s pig farming industry, which has important implications for the development of China’s pig farming industry.
Table 3. Fixed effect regression results
It is worth mentioning that after adding the mediating variables, the number of patents granted per capita, and the total retail sales of social commodities have a positive effect on LSPF, and the average labor cost per pig has a significantly negative effect on LSPF, which provides a regression basis for the mediation effects test.
5.2. Robustness test and endogeneity test
5.2.1. Robustness check
Robustness tests are conducted to ensure the reliability of the baseline regression results.
First, province-year interaction effects are introduced to alleviate the possible changes brought about by the extensive development of the digital economy (Zhang et al., Citation2022). The results in Column (1) of Table reveal that the promotion effect of the digital economy on LSPF has passed the significance test of 1%.
Secondly, this study uses the proportion of farms with a breeding scale of more than 1,000 (LSPF (>1,000)) and 3,000 pigs (LSPF (>3,000)) to replace the proportion of farms with farm sizes greater than 500 pigs, to test whether the digital economy still has a facilitating effect on LSPF. Columns (2) and (3) of Table shows that the digital economy contributes significantly to LSPF.
Table 4. Robustness test
The information service industry, including the information transmission service, IT service, software industry (Matsuno et al., Citation2017), is very closely related to the digital economy and is the basis for the sustainable development of the digital economy (Garifova, Citation2015). Therefore, this study adopts the information service industry output value, instead of the digital economy development level, to examine the robustness of the model. We consider the logarithmic output value of information service industry (Lnisiov) and the data from the China National Bureau of Statistics (National Bureau of Statistics of China, Citation2022) to examine robustness. Columns (4) and (5) in Table suggest that the proxy variables (Lnisiov) are still significant, thus revealing that the digital economy significantly promotes the development of LSPF, further supporting Hypothesis 1.
5.2.2. Endogenous analysis
The endogeneity of variables is an important problem that needs to be resolved in empirical studies. If the model has endogeneity, the result of the empirical analysis will be biased. Control of the fixed effect can mitigate endogeneity (Ma & Zhu, Citation2022). Hence, this study adopts the two-way fixed effects in the baseline regression (Table ), and the result shows that digital economy can drive LSPF. However, considering that the model may have missing variables that could also cause endogeneity problems, we adopt the instrument variable method to alleviate endogeneity. Based on two basic conditions of the instrument variable (IV), the variable relevant to the digital economy is determined, without affecting LSPF (Zou & Deng, Citation2022). The development of the digital economy is closely related to communication devices, such as optical cables. In China, fiber optic cable is mostly used for national defense and military constructions, and for residents’ information communication devices. It is constructed by enterprises directly managed by the Chinese central government, such as China Telecom and China Unicom. The fiber optic cable construction is not directly related to the local pig farms, but the digital economy requires fiber optic cables as a support equipment for technology. Therefore, the logarithm of the fiber optic cable construction length (Lnoccl) is used as an instrumental variable.
The two stage least square method (2SLS) is adopted for the estimation. From the 2SLS regression results shown in Table , the Kleibergen-Paap rk Wald F statistic is larger than 10 (12.665), thus indicating that the model passes the endogenous test. It further confirms that the digital economy has a significant promotional effect on LSPF. The estimates after using the instrumental variables method taught that the fixed effects model estimates rose by 0.127, that the OLS regression caused approximately 0.127 of the estimated bias, and that the coefficient estimate of 0.18 corrected using the 2SLS regression was closer to the causal effect of the digital economy on LSPF.
Table 5. 2SLS Estimate
5.3. Spatial spillover effects analysis
To examine the impact of the spatial effect of the digital economy on LSPF, the study first tests the spatially correlation between LSPF and digital economy. From Table , all Moran’s Index of digital economy and LSPF from 2011 to 2018 are significant, thereby suggesting that the LSPF and De have obvious spatial correlation in China.
Table 6. Spatial correlation test results of the digital economy on LSPF
The Hausman test results in Table show that the random effect model is rejected, and hence, we select the fixed effect model. In addition, the LR-Test and Wald-Test results reject both SDM degradation to spatial automatic regress model (SAR) and spatial error model (SEM), so this study chooses the SDM for analysis.
Table 7. Selection of spatial panel model
As point estimation testing of spatial spillover effect may lead to model estimation bias, it is necessary to decompose the total direct and indirect effects to more accurately explainable spatial spillover effects (Pace & LeSage, Citation2009). Table confirms that the direct effects in the SDM and SAR are significantly positive, but the indirect effects in the SDM and SAR are negatively influenced, thus showing that the digital economy has a negative spatial spillover effect on LSPF. A possible explanation for this could be that LSPF requires large amounts of capital, technology, labor, and a stable source of feed. Although the development of the digital economy can facilitate the flow and efficiency of use of these necessary resources, the regions with higher levels of digital economy would also absorb capital, labor, and other elements of production from the surrounding areas for economic prosperity. This situation is not conducive to the development of LSPF in the neighborhood.
Table 8. Spatial regression results
5.4. Mediation effects analysis
We use the mediation effect model to further explain the internal transmission mechanism of the digital economy on LSPF. In addition, this study includes mediating variables during baseline regression analysis (Table ). Columns (1)-(6) of Table establishes that the digital economy can significantly promote the rate of LSPF by enhance the technology innovation and merchandise sales, and reduce the average labor cost per pig, thus Hypothesis 3 was verified. Specifically, we look at the transmission mechanism of this mediating effect. First, the development of the digital economy promotes technological innovation and merchandise sales in each Chinese province, reducing the average labor cost per pig; also, the results show a relatively significant effect of the digital economy on merchandise sales, with each unit increase in the digital economy leading to a rise in merchandise sales, while technological innovation increases by 0.064%. Further, an increase in technology innovation and merchandise sales can also facilitate the rate of LSPF, with each 1% increase in technology innovation and merchandise sales increasing the LSPF by 0.214 and 0.011 units, respectively, and each 1% increase in the average labor cost per pig decreasing the LSPF by 0.029 units. Our findings reveal that technological innovation, merchandise sales and labor costs are effective mediating variables and help us further understanding of how the digital economy can increase the proportion of LSPF.
Table 9. Mediation effect test
5.5. Heterogeneity analysis
LSPF is also greatly related to regional resource endowment and economic development positioning. The Chinese government categorizes the development of pig farms in four areas, key development area, potential growth area, constrained development area and moderate development area, in accordance with the endowment of livestock production and economic development position in each region.Footnote3 Therefore, we further analyze the role of the digital economy on LSPF in different regions of China.
Table shows that the digital economy in the key development areas and potential growth areas provide significant promotion for LSPF, primarily because these areas have better resource endowment for pig farming and have relative advantages in the availability of land for farming, government subsidies and agricultural development. So, the development of the digital economy allows these regions to integrate resources to develop LSPF. Constrained development areas include areas with high levels of economic development, such as Beijing, Shanghai, Jiangsu, and Guangdong. These areas have a higher level of economic development and their industrial structure is mainly based on the service industry and high-tech industry, and it is, therefore, difficult to carry out LSPF in these regions. As the level of development of the digital economy increases, it becomes tougher for these regions to support the development of LSPF, but they have the resources to develop high-tech industries and services with higher added value. The digital economy in the moderate development area has a certain promotion effect on the development of LSPF, but the results do not pass the significance test. Moderate development areas are Tibet, Xinjiang, Shaanxi, Shanxi, and Gansu, which have vast land areas and good endowment of livestock breeding resources, and the growth of the digital economy can promote the improvement of breeding resource utilization efficiency.
Table 10. Heterogeneity analysis
6. Conclusions
This study adopts the entropy weight method to measure the development level of the digital economy in 31 provinces in China from 2011 to 2018 and further analyzes the relationship between the digital economy and LSPF. The main findings of this study are as follows: First, the development of the digital economy not only directly contribute to LSPF but also indirectly boosts to the proportion of large-scale pig farms through improved technological innovation, enhanced merchandise sales, and reduced average labor cost per pig. Second, the digital economy has a negative spatial spillover effect on LSPF, which means that the development of the digital economy in one area does not facilitate LSPF in the neighboring areas. There are many reasons for this, but the most likely one is that the areas with highly developed digital economies will absorb labor and capital from the neighboring areas, which may hinder the development of the large-scale pig breeding industry in the surrounding areas. Finally, the results of the heterogeneity analysis show that the digital economy in the key development areas and potential growth areas of pig breeding has a significant promotional role for LSPF. However, in the constrained development areas of pig breeding, such as Beijing and Shanghai, the development of the digital economy is not conducive to LSPF.
Based on the above conclusions, we propose the following policy recommendations. First, we found that the development of the digital economy can promote LSPF. That is, the Chinese government needs to properly guide the development of the digital economy in each province, and encourage the adoption of digital technologies in the pig breeding industry to improve production efficiency, reduce production costs, and facilitate a shift from traditional backyard farming to large-scale farming. While improving the scale of pig breeding, local governments should also promote the intensive, standardized, automated and intelligent development of the whole industrial chain of production and operation, such as breeding, slaughtering, processing, and cold chain logistics. Second, the digital economy can have a negative spatial spillover effect on LSPF, which is not beneficial for the development of LSPF in low-level digital economy areas. This requires the Chinese government to increase its support to low-level digital economy regions, especially large pig-breeding provinces such as Sichuan, Henan, and Yunnan. Provincial governments should also introduce relevant policies to support the development of the local pig farming industry and promote the transformation and upgradation of the province’s pig farming industry. Meanwhile, major pig breeding provinces such as Sichuan and Henan, provinces with a stronger digital economy such as Shanghai and Beijing, must cooperate further to improve the application of digital economy in the pig breeding industry. Third, regional differences play a key role in the relationship between the digital economy and LSPF. Therefore, the Chinese government should consider local conditions and customize preferences for pig breeding farms in key development areas, potential growth areas, and moderate development areas, and establish policies to minimize the overflow of capital and labor from these areas to highly developed economic areas.
7. Research limitations and future research
Although this study empirically analyzes the role of the digital economy on the LSPF, there are a few limitations. First, although it attempts to construct an indicator system for the development level of China’s provincial digital economy using 21 types of data. But because of the complexity of the digital economy, the indicator system cannot accurately reflect the development level of the digital economy. Second, the impact of uncertain factors that may affect the LSPF, such as environmental regulation, policy changes, and resident consumption preference, are not considered.
Therefore, future research can focus on the following aspects: (1) employ more scientific and rational methods to measure the development of the digital economy comprehensively and precisely; and (2) emphasize the impact of external shocks on LSPF, including environmental regulations, policy changes, COVID-19, and other factors.
Abbreviations
CAICT | = | China Academy of Information and Communication Technology |
LSPF | = | large-scale pig farming |
SAR | = | spatial automatic regress model |
SDM | = | spatial Durbin model |
SEM | = | spatial error model |
ICT | = | information and communication technology |
OLS | = | ordinary least squares. |
NBS | = | National Bureau of Statistics of China. |
Geolocation information
The main area of the study is mainland China.
Correction
This article has been corrected with minor changes. These changes do not impact the academic content of the article.
Disclosure statement
No potential conflict of interest was reported by the author(s).
Data availability statement
The datasets analyzed during the current study are available from the corresponding author on reasonable request or can be collected from https://data.cnki.net/.
Additional information
Funding
Notes on contributors
Kun Zhou
Kun Zhou Ph.D., is currently studying in the College of Management, Sichuan Agricultural University. He has been majored in the digital economy, agriculture economy, and environmental economy.
Huan Wang
Huan Wang is a Ph.D., graduated from China Agricultural University. He is a lecturer and teaches at Sichuan Agricultural University. He has been majoring in the animal husbandry economy.
Jin Wu
Jin Wu Master, graduated from Jilin Agricultural University. He is a professor at the Biogas Institute of the Ministry of Agriculture and Rural Affairs. He has been focuses on rural energy, agricultural resource utilization, and circular economy policy.
Jianqiang Li
Jianqiang Li Ph.D., graduated from Nanjing Agricultural University. He is a professor and a doctoral supervisor at the College of Management, Sichuan Agricultural University. He has been mainly engaged in the teaching and scientific research of land resources management and livestock economy.
Notes
1. The data on pork production and trade are from the United States Department of Agriculture: https://apps.fas.usda.gov/psdonline/circulars/livestock_poultry.pdf.
2. The digital inclusive finance data are from https://idf.pku.edu.cn/docs/20210421101507614920.pdf. It is worth noting that the digital financial inclusion data are measured by the Institute of Digital Finance Peking University based on billions of data from Ali Research Institute. The digital financial inclusion consists of three first-level indicators, twelve second-level indicators, and 33 third-level indicators, including coverage breadth, usage depth, and degree of digital level.
3. China’s Ministry of Agriculture and Rural Affairs released the National Pig Production Development Plan (2016–2020) in 2016, a document that takes into account factors such as the environmental carrying capacity of pig breeding, resource endowment, consumption preferences and slaughter and processing, and divides China’s 31 provinces into key development areas (including seven provinces or cities such as Hebei, Shandong and Henan), constrained development areas (including 11 provinces or cities such as Beijing, Shanghai and Tianjin), potential growth areas (including six provinces such as Liaoning and Jilin) and moderate development areas (including seven provinces such as Shaanxi and Shanxi). Information from:http://www.moa.gov.cn/nybgb/2016/diwuqi/201711/t20171127_5920859.htm.
References
- Bao, J., & Xie, Q. (2022). Artificial intelligence in animal farming: A systematic literature review. Journal of Cleaner Production, 331, 129956. https://doi.org/10.1016/j.jclepro.2021.129956
- Benjamin, M., & Yik, S. (2019). Precision livestock farming in swine welfare: A review for swine practitioners. Animals, 9(4), 133. https://doi.org/10.3390/ani9040133
- Blut, M., Wang, C., Wünderlich, N. V., & Brock, C. (2021). Understanding anthropomorphism in service provision: A meta-analysis of physical robots, chatbots, and other AI. Journal of the Academy of Marketing Science, 49(4), 632–25. https://doi.org/10.1007/s11747-020-00762-y
- Chen, Y. (2020). Improving market performance in the digital economy. China Economic Review, 62, 101482. https://doi.org/10.1016/j.chieco.2020.101482
- Chen, X., Teng, L., & Chen, W. (2022). How does FinTech affect the development of the digital economy? Evidence from China. The North American Journal of Economics & Finance, 61, 101697. https://doi.org/10.1016/j.najef.2022.101697
- Chen, P., Xie, R., Lu, M., & Huang, Z. (2021). The impact of the spatio-temporal neighborhood effect on urban eco-efficiency in China. Journal of Cleaner Production, 285, 124860. https://doi.org/10.1016/j.jclepro.2020.124860
- Collins, L. M., & Smith, L. M. (2022). Review: Smart agri-systems for the pig industry. Animal, 100518, 100518. https://doi.org/10.1016/j.animal.2022.100518
- Ding, Y., Zhang, H., & Tang, S. (2021). How does the digital economy affect the domestic value-added rate of chinese exports? Journal of Global Information Management, 29(5), 71–85. https://doi.org/10.4018/JGIM.20210901.oa5
- Dubé, J., Legros, D., Thériault, M., & Des Rosiers, F. (2014). A spatial difference-in-differences estimator to evaluate the effect of change in public mass transit systems on house prices. Transportation Research Part B: Methodological, 64, 24–40. https://doi.org/10.1016/j.trb.2014.02.007
- Eastwood, C. R., Edwards, J. P., & Turner, J. A. (2021). Review: Anticipating alternative trajectories for responsible Agriculture 4.0 innovation in livestock systems. Animal, 15, 100296. https://doi.org/10.1016/j.animal.2021.100296
- Eastwood, C., Klerkx, L., & Nettle, R. (2017). Dynamics and distribution of public and private research and extension roles for technological innovation and diffusion: Case studies of the implementation and adaptation of precision farming technologies. Journal of Rural Studies, 49, 1–12. https://doi.org/10.1016/j.jrurstud.2016.11.008
- Garifova, L. F. (2015). Infonomics and the value of information in the digital economy. Procedia Economics and Finance, 23, 738–743. https://doi.org/10.1016/S2212-5671(15)00423-2
- Giusti, J. D., Alberti, F. G., & Belfanti, F. (2020). Makers and clusters. Knowledge leaks in open innovation networks. Journal of Innovation & Knowledge, 5(1), 20–28. https://doi.org/10.1016/j.jik.2018.04.001
- Groher, T., Heitkämper, K., & Umstätter, C. (2020). Digital technology adoption in livestock production with a special focus on ruminant farming. Animal, 14(11), 2404–2413. https://doi.org/10.1017/S1751731120001391
- Hojeghan, S. B., & Esfangareh, A. N. (2011). Digital economy and tourism impacts, influences and challenges. Procedia – Social and Behavioral Science, 19, 308–316. https://doi.org/10.1016/j.sbspro.2011.05.136
- Kablana, A. S. K., & Chhikara, K. S. (2013). A theoretical and quantitative analysis of financial inclusion and economic growth. Management and Labour Studies, 38(1–2), 103–133. https://doi.org/10.1177/0258042X13498009
- Knudsen, E. S., Lien, L. B., Timmermans, B., Belik, I., & Pandey, S. (2021). Stability in turbulent times? The effect of digitalization on the sustainability of competitive advantage. Journal of Business Research, 128, 360–369. https://doi.org/10.1016/j.jbusres.2021.02.008
- Kuznetsov, N. I., Ukolova, N. V., Monakhov, S. V., & Shikhanova, J. A. (2018). Development of the digital economy in modern agriculture of Russia: Opportunities, drivers and trends. Scientific Papers Series Management, Economic Engineering in Agriculture & Rural Development, 18(1), 219–226. Retrieved April 14, 2022 http://managementjournal.usamv.ro/pdf/vol.18_1/Art28.pdf
- Li, J., Chen, L., Chen, Y., & He, J. (2022). Digital economy, technological innovation, and green economic efficiency—Empirical evidence from 277 cities in China. Managerial and Decision Economics, 43(3), 616–629. https://doi.org/10.1002/mde.3406
- Lin-Schilstra, L., Backus, G., Snoek, H., & Mörlein, D. (2022). Consumers’ view on pork: Consumption motives and production preferences in ten European Union and four non-European Union countries. Meat Science, 187, 108736. https://doi.org/10.1016/j.meatsci.2022.108736
- Liu, Y., Liu, S., Shao, X., & He, Y. (2022). Policy spillover effect and action mechanism for environmental rights trading on green innovation: Evidence from China’s carbon emissions trading policy. Renewable and Sustainable Energy Reviews, 153, 111779. https://doi.org/10.1016/j.rser.2021.111779
- Li, Z., & Wang, J. (2022). The dynamic impact of digital economy on carbon emission reduction: Evidence city-level empirical data in China. Journal of Cleaner Production, 351, 131570. https://doi.org/10.1016/j.jclepro.2022.131570
- Li, J., Wu, Y., & Xiao, J. J. (2020). The impact of digital finance on household consumption: Evidence from China. Economic Modelling, 86, 317–326. https://doi.org/10.1016/j.econmod.2019.09.027
- Matsuno, S., Uchida, Y., Ito, T., & Ito, T. (2017). A survival analysis of the Japanese information service industry. Procedia computer science, 121, 291–296. https://doi.org/10.1016/j.procs.2017.11.040
- Ma, D., & Zhu, Q. (2022). Innovation in emerging economies: Research on the digital economy driving high-quality green development. Journal of Business Research, 145, 801–813. https://doi.org/10.1016/j.jbusres.2022.03.041
- Miao, Z. (2021). Digital economy value chain: Concept, model structure, and mechanism. Applied Economics, 53(37), 4342–4357. https://doi.org/10.1080/00036846.2021.1899121
- Mikayilov, J. I., Galeotti, M., & Hasanov, F. (2018). The impact of economic growth on CO2 emissions in Azerbaijan. Journal of Cleaner Production, 197(10), 1558–1572. https://doi.org/10.1016/j.jclepro.2018.06.269
- Milford, A. B., Le Mouël, C., Bodirsky, B. L., & Rolinski, S. (2019). Drivers of meat consumption. Appetite, 141, 104313. https://doi.org/10.1016/j.appet.2019.06.005
- Mossberger, K., LaCombe, S., & Tolbert, C. J. (2022). A new measure of digital economic activity and its impact on local opportunity. Telecommunications Policy, 46(1), 102231. https://doi.org/10.1016/j.telpol.2021.102231
- National Bureau of Statistics of China. 2022. Regional data (Annual by province,2011-2018). Retrieved January 24, 2022. https://data.stats.gov.cn/easyquery.htm?cn=E0103.
- Neethirajan, S. (2017). Recent advances in wearable sensors for animal health management. Sensing and Bio-Sensing Research, 12, 15–29. https://doi.org/10.1016/j.sbsr.2016.11.004
- Neethirajan, S., & Kemp, B. (2021). Digital livestock farming. Sensing and Bio-Sensing Research, 32, 100408. https://doi.org/10.1016/j.sbsr.2021.100408
- Pace, R. K., & LeSage, J. P. (2009). A sampling approach to estimate the log determinant used in spatial likelihood problems. Journal of Geographical Systems, 11(3), 209–225. https://doi.org/10.1007/s10109-009-0087-7
- Pan, W., He, Z., & Pan, H. (2021). Research on spatiotemporal evolution and distribution dynamics of digital economy development in China. China Soft Science, 10, 137–147. https://kns.cnki.net/kcms/detail/detail.aspx?dbcode=CJFD&dbname=CJFDLAST2021&filename=ZGRK202110014&uniplatform=NZKPT&v=3a01D728ebOpZ_a6y3UFucMFK7ebpM-lh0X-7WFATwtdxy8lag-cvyJqcrrCnr23
- Pan, W., Xie, T., Wang, Z., & Ma, L. (2022). Digital economy: An innovation driver for total factor productivity. Journal of Business Research, 139, 303–311. https://doi.org/10.1016/j.jbusres.2021.09.061
- Paradise, J. F. (2019). China’s quest for global economic governance reform. Journal of Chinese Political Science, 24(3), 471–493. https://doi.org/10.1007/s11366-019-09610-5
- Ping, J. L., Green, C. J., Zartman, R. E., & Bronson, K. F. (2004). Exploring spatial dependence of cotton yield using global and local autocorrelation statistics. Field Crops Research, 89(2–3), 219–236. https://doi.org/10.1016/j.fcr.2004.02.009
- Pradhan, R. P., Arvin, M. B., Nair, M., Bennett, S. E., & Bahmani, S. (2019). Short-term and long-term dynamics of venture capital and economic growth in a digital economy: A study of European countries. Technology in Society, 57, 125–134. https://doi.org/10.1016/j.techsoc.2018.11.002
- Qiao, F., Huang, J., Wang, D., Liu, H., & Lohmar, B. (2016). China’s hog production: From backyard to large-scale. China Economic Review, 38, 199–208. https://doi.org/10.1016/j.chieco.2016.02.003
- Ren, S., Hao, Y., Xu, L., Wu, H., & Ba, N. (2021). Digitalization and energy: How does internet development affect China’s energy consumption? Energy Economics, 98, 105220. https://doi.org/10.1016/j.eneco.2021.105220
- Research & markets. 2020. Global pork market forecast (2018 to 2026)—by production, consumption, import, export & company. Retrieved May 1, 2022. https://www.globenewswire.com/news-release/2020/05/12/2031727/0/en/Global-Pork-Market-Forecast-2018-to-2026-By-Production-Consumption-Import-Export-Company.html.
- Rosendal, G. K., & Olesen, I. (2022). Overcoming barriers to breeding for increased lice resistance in farmed Atlantic salmon: A case study from Norway. Aquaculture, 548(1), 737574. https://doi.org/10.1016/j.aquaculture.2021.737574
- Sahoo, M. M., Patra, K. C., Swain, J. B., & Khatua, K. K. (2017). Evaluation of water quality with application of Bayes’ rule and entropy weight method. European Journal of Environmental and Civil Engineering, 21(6), 730–752. https://doi.org/10.1080/19648189.2016.1150895
- Shi, B., Wang, S., Jiao, J., Li, G., & Yin, C. (2022). Recognition on characteristics and applicability of typical modes for manure & sewage management in pig farming: A case study in Hebei, China. Waste Management, 148, 83–97. https://doi.org/10.1016/j.wasman.2022.05.018
- Sorescu, A., & Schreier, M. (2021). Innovation in the digital economy: A broader view of its scope, antecedents, and consequences. Journal of the Academy of Marketing Science, 49(4), 627–631. https://doi.org/10.1007/s11747-021-00793-z
- Sun, R., Liu, X., Li, D., Zhuang, J., Qi, S., & Meng, B., Liu, M. (2022). Optimization of China’s pig production system to reduce environmental impacts based on a data envelopment and life cycle analysis model. Resources, Conservation and Recycling, 185, 106483. https://doi.org/10.1016/j.resconrec.2022.106483
- Tang, C., Xu, Y., Hao, Y., Wu, H., & Xue, Y. (2021). What is the role of telecommunications infrastructure construction in green technology innovation? A firm-level analysis for China. Energy Economics, 103, 105576. https://doi.org/10.1016/j.eneco.2021.105576
- Tobler, W. R. (1970). A computer movie simulating urban growth in the Detroit Region. Economic Geography, 46, 234. https://doi.org/10.2307/143141
- Tzanidakis, C., Simitzis, P., Arvanitis, K., & Panagakis, P. (2021). An overview of the current trends in precision pig farming technologies. Livestock Science, 249, 104530. https://doi.org/10.1016/j.livsci.2021.104530
- Vorotnikov, I. L., Ukolova, N. V., Monakhov, S. V., Shikhanova, J. A., & Neyfeld, V. V. (2020). Economic aspects of the development of the “digital agriculture” system. Scientific Papers Series Management, Economic Engineering in Agriculture & Rural Development, 20(1), 633–638. Accessed April 14,2022. http://managementjournal.usamv.ro/index.php/scientific-papers/85-vol-20-issue-1/2174-economic-aspects-of-the-development-of-the-digital-agriculture-system
- Watanabe, C., Naveed, K., Tou, Y., & Neittaanmäki, P. (2018). Measuring GDP in the digital economy: Increasing dependence on uncaptured GDP. Technological Forecasting & Social Change, 137, 226–240. https://doi.org/10.1016/j.techfore.2018.07.053
- Williams, L. D. (2021). Concepts of digital economy and industry 4.0 in intelligent and information systems. International Journal of Intelligent Networks, 2, 122–129. https://doi.org/10.1016/j.ijin.2021.09.002
- Xu, H., Ma, C., Lian, J., Xu, K., & Chaima, E. (2018). Urban flooding risk assessment based on an integrated k-means cluster algorithm and improved entropy weight method in the region of Haikou, China. Journal of Hydrology, 563, 975–986. https://doi.org/10.1016/j.jhydrol.2018.06.060
- Xu, G., Sarkar, A., Qian, L., Shuxia, Z., Rahman, M. A., & Yongfeng, T. (2022). The impact of the epidemic experience on the recovery of production of pig farmers after the outbreak-Evidence from the impact of African swine fever (ASF) in Chinese pig farming. Preventive Veterinary Medicine, 199, 105568. https://doi.org/10.1016/j.prevetmed.2022.105568
- Xu, J., & Yu, W. (2022). Information and communication technology and carbon emissions in China: The rebound effect of energy intensive industry. Sustain Prod Consum, 12. https://doi.org/10.1016/j.spc.2022.06.004
- Yao, H., Zang, C., Zuo, X., Xian, Y., Lu, Y., Huang, Y., & Li, X. (2022). Tradeoff analysis of the pork supply and food security under the influence of African swine fever and the COVID-19 outbreak in China. Geography and Sustainability, 3(1), 32–43. https://doi.org/10.1016/j.geosus.2022.01.005
- Zhang, W., Liu, X., Wang, D., & Zhou, J. (2022). Digital economy and carbon emission performance: Evidence at China’s city level. Energy Policy, 165, 112927. https://doi.org/10.1016/j.enpol.2022.112927
- Zhao, J., Bai, Z., & Ma, L. (2019). China needs long-term solutions for African Swine Fever. Sci Bull, 3(20), 1469–1471. https://doi.org/10.1016/j.scib.2019.08.015
- Zhen, H., Cheng, H., & Chun, Y. (2020). Spatial econometric analysis of environmental total factor productivity of ranimal husbandry and its influencing factors in China during 2001–2017. The Science of the Total Environment, 723, 137726. https://doi.org/10.1016/j.scitotenv.2020.137726
- Zhu, W., & Chen, J. (2022). The spatial analysis of digital economy and urban development: A case study in Hangzhou, China. Cities, 123, 103563. https://doi.org/10.1016/j.cities.2022.103563
- Zou, J., & Deng, X. (2022). To inhibit or to promote: How does the digital economy affect urban migrant integration in China? Technol. Technological Forecasting & Social Change, 179, 121647. https://doi.org/10.1016/j.techfore.2022.121647