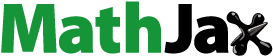
Abstract
Over the past decades, intensive reclamation has caused significant land degradation, threatening both ecological and food security. The Chinese government has taken a series of novel Conservation Tillage (CT) measures according to regional climate and soil conditions to increase agriculture’s multifunctional and resilience. Previous studies have reported CT in northeast China can improve soil organic carbon and reduce ammonia emissions in northeast China. However, whether the regionally adapted CT can avoid its inherent limitations in seed emergence and crop growth is still lacking comprehensive understanding and investigation. By using high-resolution and time-series Unmanned Aerial Vehicle (UAV) observations, our study investigated and evaluated the performance of different advanced and regional adapted CT treatments in crop emergence and growth compared with conventional tillage during the whole growing season. Based on the field measurements, we found that although the residues can impact the seed emergence, there was no significant difference between CT treatments and conventional tillage, with some CT treatments can even raise the emergence rate. We also found that the high residues covered CT treatments obstructed the maize grown in the early growing season including the V3 and V6 period, while the crop growth in these treatments caught up with the conventional tillage in the following growing periods. Our study suggested that well-adjusted CT treatments in Northeast China have the potential to maintain crop production accompanied by enhancing the ecosystem’s multifunctionality. The results from this study provided observational evidence of crop growth impact from CT, which will have great implications in convincing the farmers and beneficial to the promotion of conservation tillage.
1. Introduction
In light of the growing global population and associated food demand, Conservation Agriculture (CA) has become an effective approach to ensuring future food security and supporting sustainable agricultural development (Eeswaran et al., Citation2021; Landers et al., Citation2021; Pittelkow et al., Citation2015; Xu et al., Citation2022). Generally, CA is a crop production system based on three main principles: minimum mechanical soil disturbance, permanent degrees of residues retention, and crop rotation/intercropping (FAO, Citation2014; Kassam et al., Citation2019). CA could generate substantial environmental benefits and has been extensively studied in North and South America, Australia, Europe, South Africa, and Asia (Kassam et al., Citation2022; Liu et al., Citation2019; Rusinamhodzi et al., Citation2011; TerAvest et al., Citation2015; Thierfelder & Mhlanga, Citation2022). With less disruption to soil’s structure and more crop residues, CA can promote ecosystem multifunctionality by raising carbon sequestration, retaining soil moisture, enhancing soil biodiversity, preventing soil erosion, enhancing resilience to climate change/extremes, and improving water quality (Holland, Citation2004; Komarek et al., Citation2021; Petito et al., Citation2022; Wittwer et al., Citation2021). Returning the crop residues to the field instead of burning them can also reduce the risk of air pollution. Conservation Tillage (CT) has been broadly defined as a soil management practice in which most of the surface is undisturbed compared to conventional tillage and leaves sufficient crop residues in place to cover the soil surface after planting (Holland, Citation2004). Adopting CT reduced the intensity of soil cultivation and thereby decreased the consumption of fossil fuels (Phillips et al., Citation1980), CO2 emissions, and production costs (Palm et al., Citation2014).
In compliance with Sustainable Development Goals (SDGs) proposed by the United Nations, CA is designed to ensure long-term, sustainable agricultural production while improving crop resilience to climate change and protecting the soil, atmosphere, and even water environment (Nouri et al., Citation2021; Su et al., Citation2021; Y. J. Zhang et al., Citation2021). Furthermore, with residues retained on the surface, CA can also influence the surface energy budget. CA increased the surface albedo when the soil was covered with crop residues and decreased the surface solar radiation absorption and made the soils cooler than the conventionally tilled soils, mainly for the beginning of the growing season (Drury et al., Citation1999; Fabrizzi et al., Citation2005; Ogle et al., Citation2012). The potential for soil waterlogging and lower soil temperature in certain regions may constrain root growth and further affect yield (Alvarez & Steinbach, Citation2009; Van den Putte et al., Citation2010).
Although having so many environmental advantages, how CA alters crop yield is still uncertain, depending on the soil types, climate conditions, and crop types, which strongly influenced the farmer’s acceptability and promotion of CA (Mupangwa et al., Citation2021; Pittelkow et al., Citation2015; Soane et al., Citation2012; Thierfelder et al., Citation2015). For instance, CA has considerable potential in south Asia to increase rice-wheat productivity by up to 38% (T. N. Zhang et al., Citation2022). Rusinamhodzi et al. (Citation2011) found long-term zero tillage treatment can help improve the rainfed maize yield in semi-arid and sub-humid regions. Yield benefits of CA were recorded almost immediately in some regions of Africa, where water is limited (Thierfelder et al., Citation2013). Mulch cover in high rainfall areas is investigated to decrease yields in high rainfall areas due to waterlogging, whereas increase yields in semi-arid regions due to moisture retention (Rusinamhodzi et al., Citation2011). In Eastern and Southern Africa, maize-common bean systems under CA produced the most stable maize yields (Mupangwa et al., Citation2021). These studies highlight to achieve the sustainable goal (both environment multifunction and yields) of CA, CA should be targeted and adapted to local specific biophysical conditions and socio-economic factors.
As one of the top three black soil regions in the Northern Hemisphere, Northeast China produces one-third of the country’s commercial grain (L. Yu et al., Citation2022). However, intensive reclamation has caused significant degradation including soil erosions, threatening both ecological and food security. In the past decades, the Chinese government has taken a series of novel CT measures according to regional climate and soil conditions to increase agriculture’s multifunctional and resilience. Previous studies have reported CT in northeast China can improve soil organic carbon (Y. Zhang et al., Citation2022). Combined with the appropriate application of livestock manure and straw return, CT can reduce ammonia emissions and increase crop productivity in northeast China (Xu et al., Citation2022). However, whether the regionally adapted CT can avoid its inherent limitations in seed emergence and crop growth is still lacking comprehensive understanding and investigation.
Remote sensing technologies have been widely applied in investigating land surface environmental changes in response to climate change and human management (Liang et al., Citation2010; L. X. Yu & Liu, Citation2019; L. Yu et al., Citation2020; L. X. Yu et al., Citation2017). For example, remote sensing can help detect crop residues based on high-resolution satellite imagery in CT practices (Bouwman et al., Citation2021), which also provide effective solutions to evaluate the impact of different tillage treatments on crop growth at different scales. The development of unmanned aerial vehicles (UAVs) and novel image processing techniques made the crop growth impact comparison between each tillage can be achieved at finer scales, for example, centimeter or millimeter resolution. This made quantifying the seed emergence rate at the plant scale possible when the growing season starts. Therefore, at the local scale, the new UAV technologies provide an efficient tool to evaluate the performance in crop growth of CT, which can help inform agricultural policymakers and convince farmers in adopting and expanding the use of CT in the near future.
Therefore, our major objective of this study was to explore the effects of different tillage treatments on seed emergence and crop growth during the growing season. First, super-high UAV observations with 7.9 mm resolution were used to investigate spatial distributions of crop residues, soil, and maize at the beginning of the growing season. Second, based on crop-count algorithms and time-series UAV NDVI observations, the seed emergence rate and the following crop growth at each growing stage for each treatment are compared and analyzed. Finally, we discussed the driving factors influencing maize emergence and crop growth. Our results provide a direct evaluation of CT performance during the crop growth process, which can help convince indecisive farmers to adopt CT in their future cultivation, and finally achieve short-term gains and longer-term sustainability.
2. Method and materials
2.1. Study area
The conservation tillage field experiment of Maize was established at Chenjiadian (~44.076°N,125.133°E), in Nong’an County, Jilin Province, China (Figure ). The Chenjiadian demonstration plot was supported by the Science and Technology Project for Black Soil Granary, Chinese Academy of Sciences, and was mainly used to display new conservation tillage techniques and made sustainable agriculture possible. The climate here belongs to a temperate continental monsoon climate, with the annual mean temperature and annual total precipitation being 6.3 °C and 580 mm, respectively. The growing season here begins in May and ends in late September, the accumulated temperature above 10 °C was approximately 2890 °C (2011 °C 2020 10-year average) and the precipitation was concentrated in the summer seasons. The climate conditions here benefit the growth of spring maize, with a single growing season. In addition, with high wind speed, the area was more likely to experience wind erosion and making here an ideal region for adopting conservation tillage.
Figure 1. The geophysical location and experiment field of our study area. The polygons with black outlines are two transects for super high-resolution UAV observations. A. Straw mulching ridge tillage (SMRT), B. Straw mulching strip-till+no-till (SMSTNT), C. Straw mulching wide-narrow row strip-till (SMWRST), D. Straw returning mulch tillage (SRMT), E. Straw mulching wide-narrow row no-till (SMWRNT), F. Straw mulching-deep plowing returning to the field in turn (SMPR) respectively.
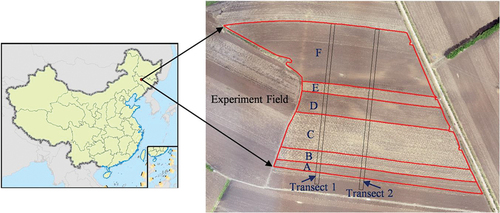
2.2. Field treatments
Among the three principles of CA, residues retention instead of tillage approaches has been reported to play a more critical role in achieving sustainable agriculture or land use (Xiao et al., Citation2021). In our study, we conducted one conventional tillage and five conservation tillage experiments across our study area in 2022 (Figure ): (A) conventional ridge tillage, which was the control experiment (B) straw mulching strip-till+no-till, (C) straw mulching wide-narrow row strip-till, (D) straw returning mulch tillage, (E) straw mulching wide-narrow row no-till, (F) straw mulching-deep plowing returning to the field in turn. For each conservation tillage, 100% of the maize residues were returned to the field after harvest in the former year. The detailed information for each tillage is shown in Table . In all six tillages, the pre-crop was maize and fertilization and pesticide applications with same formula were used in these experiments. Maize was sowed at the beginning of May, with SMWRNT a little later due to the low elevations. Previous studies reported good field management is the first step for evaluating tillage effects on crop productivity (Baudron et al., Citation2012), which was guaranteed by the experienced agronomist and advanced agricultural instruments.
Figure 2. Six tillage experiments and associated photographs in 2022 (The photographs were taken on 27 May 2022).
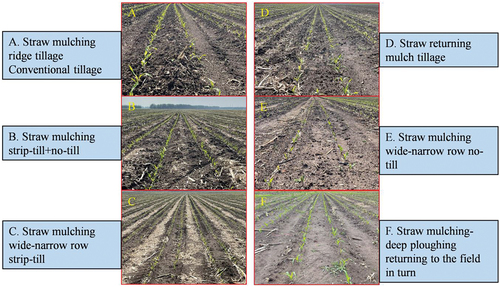
Table 1. The detailed information for six tillage experiments
2.3. UAV and field observations
We conducted a series of UAV observations after the maize sowing in the Chenjiadian demonstration plot. Before the seed emergence, the optical characteristics of the surface reflected the properties of the soil. On 12 May, visible and multispectral were obtained to obtain the soil spectral characteristics. At this time, the surface can be divided into two components including bare soil and maize straws. After the seeding stage, we conducted multispectral observations based on UAVs three times on 27 May (V2), 7 June (V3), and 17 June (V4), with plant height accounts for 10 cm, 20 cm, and 35 cm, respectively. In addition, we also conducted a series of UAV field experiments during the whole growing stages including third leaf collar (V3, 7 June), sixth leaf collar (V6, 27 June), tasseling (VT, 18 July), silking (R1, 02 August), blister (R2, 24 August), and dough (R4, 16 September) stages. In addition, we conducted simultaneous measurements of the plant height at each growth stage. Once the maize reached maturity, we selected 60 samples across our field, each with an area of 10 m × 10 m, to measure the maize yield. Note that the height-adapted approach was used to investigate crop growth during different growing stages. At the beginning of the growing season (e.g. seedling stage), the vegetation coverage was very small compared with the soil. Therefore, super-high spatial resolution is required for monitoring maize emergence at the seedling stage. In this study, we used the DJ Phantom 4-multispectral to detect the maize emergence and the flight height was set as 15 m. Then, the surface resolution can reach 0.79 cm. Because our experimental field covered ~20 ha area, it is not feasible to acquire the super high-resolution UAV data for the whole field. Therefore, we designed three belt transects (10 m in width and 480 m in length) for each flight experiment when monitoring the seed emergence. In other growing season periods, as our objective was to investigate the influence of CT on crop growth, the flight height was set as 100 m and the spatial resolution was equal to 5.29 cm.
With an integrated multispectral imaging system, DJ Phantom 4-multispectral integrates one visible light camera and five multispectral cameras (blue band, green band, red band, red edge, and near-infrared, the detailed information can be found in Table ), which are, respectively, responsible for visible light imaging and multispectral imaging. It should be noted that all cameras have a 2 million pixel resolution and are equipped with a global shutter. The imaging system is installed on the three-axis PTZ, ensuring clear and stable imaging. Generally, DJ Phantom 4-multispectral can obtain DN values and can auto-calculate the vegetation indexes, including NDVI, LCI, and so on. To obtain the reflectivity value of each band, we used 25%, 50%, and 75% reflectance calibration plates to perform radiometric correction before the vegetation indexes are calculated.
Table 2. The detailed band information for DJ Phantom 4-multispectral
2.4. Method and analysis
The object-oriented method was used to classify the land covers, including vegetation, soil, and straw. The reflectance of the first five bands shown in Table was used for scale segmentation, in which the best segmentation scale, shape factor, and compactness factor were set to 1, 0.3, and 0.7, respectively. Training samples were selected based on both the RGB composite and NIR-R-G composite images. Brightness means, the standard deviation of blue, green, red, red edge, and near-infrared bands, length/width ratio, shape index, Visible-band Difference Vegetation Index (VDVI), and Green-band Difference Vegetation Index (GDVI, subtraction green band reflectance from the red band) were selected as the classification characteristics. By using the support vector machine (SVM) algorithm, the mentioned three land cover types can be classified.
Maize counts identification and analysis
In this study, we used the ENVI Crop Science toolbox to extract the maize plants based on ENVI 5.4. One of the most important functions of this precision agriculture toolkit is to count crops, which requires high-resolution satellite or UAV images, with a resolution usually less than 0.5 m. Crop monitoring generally involves five parts: vegetation index calculating, mask threshold selection, single parcel crop extraction, crop vector output, healthy analysis, and batch crop extraction. First, VDVI was used to detect each maize plant. We used VDVI instead of NDVI because it has advantages in distinguishing vegetation and associated shadows. VDVI can be expressed as:
in which, ,
, and
are reflectivity for the red, green, and blue bands. The mask threshold was selected by trying different raster color slices. As the multispectral images were taken at different growing periods, the threshold was varied based on the maize growing status. Then, different regions of interest (ROIs) were used to count the maize plants. After that, the count vector can be output into a vector format and further merged into the final results.
We used ten 1 m × 1 m quadrats randomly distributed on each transects (total 10 × 3 belts, ranges from i to j) to evaluate the capacity of low altitude UAV images in distinguishing maize plants at different monitoring time. The identification accuracy (IA) of period t can be expressed as:
In which, represents the identified maize counts,
represents the actual maize counts, which were derived from manual visual identification. Through comparison of the IAs at different periods t, the optimal monitoring time for the maize count identification can be obtained. Based on the intelligent identification of maize plants, combined with the experts’ identification, the actual number of maize counts can be extracted. Then, the maize emergence rate (MER) for each tillage can be expressed as:
In which, is the actual maize count for tillage experiment i,
and
are the maize spacing and the number of rows for experiment i. To assess the uniformity of the seedlings, we computed the coefficient of variation (CV) in diameter extracted from the ROIs for each treatment.
In evaluating the performance of different treatments on crop growth, we first used the UAV observed zonal mean NDVI represent the crop growth status at each stage during the growing season. Then, we derived the maize height based on Gradient Boosted Regression Tree (GBRT), by training the observed maize height and corresponding UAV observed vegetation index. Similar procedures are also applied to simulate the maize yield at the field scale. GBRT is a machine learning algorithm that makes predictions by combining multiple decision trees and uses gradient descent optimization to improve the predictions (Elith et al., Citation2008; Friedman, Citation2001). In addition, we calculated the coefficient of variation of NDVI and plant height for each experiment to represent the growth homogeneously for each treatment. The frame diagram of this study was illustrated in Figure .
3. Results
3.1. Maize residues pattern across the experiment field
Based on object-oriented classification in combined with a support vector machine algorithm, maize residues cover was extracted based on the visible images across the experiment field (Figure ). We found the maize residues cover showed significant spatial heterogeneity across the field but great homogeneity among each treatment. The treatments SMWRST (C), SMSTNT (B), and SMWRNT(E) showed high residues covers, followed by SMRT (A), SRMT (D), and SMPR (F) with relatively low residues covers. This can be explained by the specialized processing for each treatment. In SMWRST, 57.02% of the surface was covered by the maize residues, which was 50.60%, and 38.84% for SMSTNT, and SMWRNT, respectively. In contrast, the straw percentage for SRMT and SMPR is 17.67% and 3.42%, respectively. The control treatment shared a 19.25% straw cover percentage. Although all of the maize residues were returned to the field, different conservation tillage technologies made the straw patterns quite different. This provides us an opportunity to investigate the impact of straw mulch on maize emergence rate and crop growth.
3.2. Effect of conservation tillage on seedling emergence
We count the maize plants based on the precision agriculture toolkit at three growth periods. A schematic diagram displaying the identification result was illustrated in Figure . In general, the first two periods (27 May and 7 June) showed high precision in identifying the maize plant, while the last period when the leaves of more than five missed several plants due to the overlapping between each plant. This suggested the size of the plant can remarkably affect the maize counting. The quadrats statistical results shown in Table also demonstrated the above description. It should also be noted that counting maize when the plant was too little, was not fair for late-emerging plants, which can be seen in Figures . Therefore, we selected the growing stage when the majority of plants have three leaf collars to monitor the maize emergence rate.
Table 3. The identified accuracy obtained from quadrats statistical results
With manual inspection and careful proofreading, the emerging maize was finally counted and calculated for each tillage technology. Generally, our results showed the emergence rates for all six tillage treatments are higher than 93% (Figure ). The treatment SRMT shared the highest emergence rate, which was 96.47%, followed by SMPR (96.46%), SMWRNT (94.74%), SMRT (94.17%), SMSTNT (93.47%), and SMWRST (93.03%). In comparison with the control experiment, we found the conservation tillage including SRMT and SMPR significantly improved the seeding emergence by ~2.5% (p < 0.05), while the other treatments including SMWRST, SMSTNT, and SMWRNT may slightly decrease the maize emergence; however, which was not significant based on the variance analysis. Combined with the characteristics of each conservation tillage, we found seed emergence rate was negatively correlated with the straw cover percentage, with the pearson’s R equals to −0.86, which was significantly at p = 0.027. These results provide evidence that maize residues can affect the maize emergence rate, and the conservation tillages with higher straw covers have no advantage in seedling emergence.
To evaluate the consistency of the seedlings, we analyzed the extracted ROIs for each treatment and calculated the mean, standard deviation (STD), and CV of the plant diameter (Table ). Our results indicate that the treatment including SMPR and SRMT had the highest plant size, with a mean diameter larger than the other treatments. In comparison, the no-tillage treatments including SMSTNT and SMWRNT, and SMWRST showed smaller plant sizes. We used the CV to represent the seedling uniformity, and our results showed that the CV in SMWRNT was the lowest, followed by SMSTNT and SMWRST. We found that the CV was largest in SMPR, followed by SMWRNT and SMRT, indicating that the seedlings in these treatments were not as uniform as those in the other three treatments.
Table 4. The crop diameter and uniformity for different tillages
3.3. Effect of conservation tillage on crop growth and maize yield
Based on time-series UAV NDVI observations at V3, V6, tasseling, silking, blister, and dough stages during the growing season, we evaluated the crop growth differences between different treatments based on zonal statistics (Figure ). At the V3 stage, the mean NDVI for all treatments was below 0.3, and the associated standard deviations for NDVI were the largest among the growing stages. In this stage, the maize plant was small, and the residues and soil are the dominant components of the land surface. The treatments with high percentage residues including SMSTNT, SMWRST, and SMWRNT shared a relatively low NDVI, whereas the treatment with low residues covered including SMPR, SMRT, and SRMT shared a relatively high NDVI, which was consistent with the seed emergence results described in section 3.2. The crop growth in the V6 stage preserved and expanded the NDVI differences between the six treatments in the prior stage to the greatest during the whole growing season. These results implied the influence of CT on crop growth mainly concentrated at the initial vegetative stages.
Figure 7. Mean and standard deviations in NDVI (a, b) and plant height (c, d) for each treatment during the growing season. A-F represent the six tillage experiments including SMRT, SMSTNT, SMWRST, SRMT, SMWRNT, and SMPR respectively. When calculating the mean and std values, the values of waterlogging areas was masked with the threshold of NDVImean-0.5NDVIstd in 24 August.
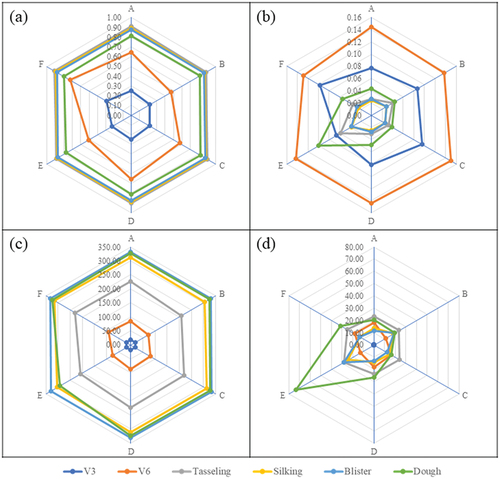
From the tasseling stage, the crop growth differences between the six treatments decreased to the minimum in the silking and blister stages. In comparison with the conventional SMRT, the maize grown in the treatment of SMPR has advantages at the beginning of the growing season (V3 and V6 periods), while no advantages were observed in the following periods. Note that although the crop growth in SMWRST was slower at the beginning of two growth periods, which caught up with the other treatments in the following stages. The NDVI in SMWRST was the highest among these treatments in the dough stage. The crop growth in SMSTNT shared a similar growth trajectory with SMWRST, with NDVI values a little smaller. Interestingly, both SMWRNT and SMWRST treatments are experimented based on wide-narrow row cultivation, crop growth differences were observed from the V6 to dough periods, with higher NDVIs in SMWRST than SMWRNT, indicating the strip-till improved from no-till has superiority for enhancing crop growth, particularly for stages from reproductive to maturity. In addition, with lower NDVI standard deviations, we found the spatial distribution of NDVI in SMRT, SMSTNT, SMWRST, SRMT, and SMPR was more homogenous than in SMWRNT, especially since tasseling.
We observed from the NDVI results that the crop’s NDVI reached its peak during the tasseling period, indicating saturation of NDVI since then. To isolate this influence, we further investigated the differences in plant height between tillages using both field measurements and UAV images. Using the GBRT machine learning approach, we generated data on plant height during six growing stages for each treatment. The zonal statistical results are also presented in Figures . Our findings demonstrate that plant height increased from V3 and reached its peak at the blister stage, followed by a slight decrease during the dough stage. This changing pattern in plant height, unlike the NDVI, reflects the actual crop growth process from the perspective of plant form. At the V3 stage, the plant heights across six different treatments are similar, averaging around 17 cm, with the standard deviations being negligible. Since the plants are still small at this stage, differences between the treatments are not yet apparent. However, significant differences in plant height become noticeable at the V6 stage, with SMPR, SRMT and SMRT treatments showing higher heights compared to SMSTNT and SMWRNT treatments. Starting from the tasseling stage, there was a noticeable increase in the difference of plant height between the SMWRNT treatment and the other treatments. This was further accentuated by the highest Standard Deviation (STD) observed in the SMWRNT treatment, indicating a greater degree of nonuniformity. It is worth noting that despite a lower initial plant height in the SMWRST treatment compared to SMPR, the former managed to catch up with the latter by the dough stage.
Based on the time series UAV observed vegetation indices and crop phenology metrics, we reconstructed the spatial pattern of maize yield across six tillage (Figure ) by using the GBRT machine learning regression approach. Before the regional statistics, we masked the yield simulated in the waterlogging areas by using the threshold of NDVImean-0.5NDVIstd in 24 August. Our findings revealed that the field mean yield was 101.55 × 102 kg/ha. The highest maize yield was observed in SMRT, which was 102.89 × 102 kg/ha, followed by SMWRST (102.79 × 102 kg/ha), SMSTNT (101.98 × 102 kg/ha), SRMT (101.21 × 102 kg/ha), SMPR (101.17 × 102 kg/ha), and SMWRNT (98.54 × 102 kg/ha). This suggests that the maize yield from some CT are comparable to the conventional ridge tillage. Specifically, the yield gap between SMWRST and SMRT was only 0.10 × 102 kg/ha, accounting for 0.10% of the yield obtained from SMRT, whereas for SMSTNT it was 0.88%. The yield gap was even larger for other treatments, representing 1.62%, 1.67%, and 4.22% for SRMT, SMPR, and SMWRNT, respectively.
4. Discussions
4.1. High-resolution UAV observations for precise agriculture monitoring
In this study, we monitored maize growth throughout the entire growing season using UAVs, from seed emergence to harvest, at the field scale, which provides an excellent case of UAV application in smart agriculture. Our results indicated the UAV observations presented great potential in monitoring the crop growth status with super high spatial resolution and high time flexibility. Compared to conventional surveys, using UAVs to collect field data is effortless, rapid, and cost-effective (Mogili & Deepak, Citation2018; Yang et al., Citation2018; C. Zhang & Kovacs, Citation2012), which was well confirmed by the seed emergence monitoring in our study. The emergence rate is a critical factor in plant growth and can significantly impact crop yield and overall plant health. In general, seed emergence rate is obtained from manual counts, which are time-consuming and may produce imprecise results if the samples are not large enough. Moreover, investigating the seed emergence rate using satellite measurements is difficult because, at the beginning of the growing season, the crop percentage is much smaller than the soil, making it impossible to distinguish the maize plant from the background. However, with flexible flight height and temporal resolution from UAVs, we could distinguish maize seedlings clearly with low-altitude flights at 15 m.
With the aid of GIS tools, we not only detected the maize emergence rate at the field scale but also identified the best time to monitor seed emergence rate. Our results detected the V3 as the best time to monitor the maize emergence rate when the flight height was set to 15 m. This optimal monitoring time may vary depending on the spatial resolution of multispectral images from DJ Phantom 4-multispectral. Our results can be helpful in field management and provide references for investigation and remedy seedlings accordingly. Note that our study used the commonly used NDVI and plant height derived from multispectral UAV images to represent the crop growth process during the growing season. In the future, more UAV payloads including Lidar can be incorporated to capture and generate more crop growth characteristics including a clearer description of crop structure.
4.2. Impact of conservational tillage on seed emergence and crop growth
An increase in environmental benefits tends to be coupled with a decrease in productivity, which can be called the productivity—environmental protection dilemma (Wittwer et al., Citation2021). Instead of evaluating the ecosystem’s multifunctionality, our study investigated how CT influences crop productivity from seed emergence to crop harvest based on high-resolution time-series UAV field measurements during the growing season from May to September. Some previous studies and farmers worried that crop residues can inhibit maize emergence due to low temperatures (Drury et al., Citation1999; Fabrizzi et al., Citation2005; Ogle et al., Citation2012). Our results found the seed emergence in all treatments including CT and conventional tillage is higher than 93%, the emergence rate in two CT treatments with fewer straw covers including SRMT and SMPR are significantly higher than conventional tillage-SMRT, while other treatments showed no significant differences with SMRT. These results indicated some regional adapted CT practices enhanced the emergence rate rather than lowering it. Our analysis confirmed the effect of straws on seed emergence as seed emergence rates are negatively correlated with straw percentages.
Previous studies documented crop yields highly depended on crop growth in the late instead of the beginning of the growing season (Jia et al., Citation2011). Based on the time-series UAV NDVI observations and derived plant height during the growing season, the residues impact on crop growth is mainly concentrated in the early growing season, including the seeding and V6 period. The CT treatments have no significant difference in affecting crop growth for the normal vegetative and reproductive growth stages with conventional tillage. Particularly, the crop growth in SMWRST treatment goes beyond conventional tillage in the dough stage. Our result revealed the maize yield from some CT are comparable to the conventional ridge tillage, with the yield gap less than 1%. However, the yield gap still existed, particularly for SMWRNT. In 2022, Jilin province experienced heavy and frequent rainfall, as a result, waterlogging occurred in some areas. The heterogeneous and relatively small NDVI and plant height observed in the western part of SRMT and SMWRNT can be attributed to the water-logging, which can be detected by the UAV RGB and NDVI images Figures . Of course, we cannot simply attribute the water-logging to the impact of applying CT. Through comparing with the digital surface model (DSM) data observed from UAVs at the seeding stage (Figure ), we found the low-lying areas are the dominant regions where flooding occurred. This water-logging event suggests drainage facilities and measurements should be established and priority should be given to areas with high flood risk. In addition, as our experiments are only conducted for one year, and the influences from CA systems on water logging or crop yield usually takes several years to show up. Continued experiments are needed to evaluate the long-term benefits from CA.
4.3. Implications and uncerntaintities
Soil temperature and moisture are considered the dominant driving factors influencing seed emergence and crop growth in CT. In mid-high latitude Northeast China, crop growth was highly affected by temperatures. With fully covered residues, more solar radiation was reflected from the surface instead of absorbed by the sheltered soil, which cools the soil. Considering the regional climate characteristics, the improved conservation tillages such as SMWRST (the residues were pulled to both sides of the seedbed by the strip plow) can effectively reduce the impact of low temperatures, and the residues can help retain soil moisture.
CT has been documented to increase soil resilience in climate extremes and increase yield stability (Nouri et al., Citation2021). In particular, CT can retain greater soil moisture than conventional tillage and can mitigate the impacts of droughts (Eeswaran et al., Citation2021). Rusinamhodzi et al. (Citation2011) documented that CT with mulch could have a negative effect over the long term due to the increased occurrence of water-logging conditions. These studies suggest that CT with mulch may enhance crop growth in water-limited regions or drought years, while it may limit crop growth or yield in humid regions or humid years. Therefore, it would be unfair to evaluate the CT performance on crop growth only based on this humid year. In addition, some studies documented that yield increases from CA usually cannot be expected in the initial years of practicing CA, and the benefits of CA become more apparent with time (TerAvest et al., Citation2015; Thierfelder & Mhlanga, Citation2022; Thierfelder et al., Citation2013, Citation2015). Therefore, multi-year time series observations are needed to comprehensively evaluate how conservation tillage influences long-term crop growth and yields.
Our study investigated how CT influences crop growth based on a whole growing season of UAV observations. As a result, the CT treatments in this study only involved two principles of CA, named less disturbance and residues coverage. It should be noted that maize-soybean rotation and maize-soybean intercropping were also applied in our experiments field, which was in the south of treatment A. Some studies documented that CA stands a high chance of outperforming CT in non-tropical regions, where with well-managed field fertilization and integrated weed and pest control (Su et al., Citation2021). Therefore, additional analysis should be conducted for these treatments when multi-year measurements are available to better evaluate the benefits of applying CA instead of CT in enhancing green agriculture and food security.
5. Conclusions
By using high-resolution and time-series UAV observations, our study investigated and evaluated the performance of different advanced and regional adapted CT treatments in crop emergence and growth compared with conventional tillage during the whole growing season. Based on the field measurements, we conclude that although the residues can impact the seed emergence, there was no significant difference between CT treatments and conventional tillage, with some CT treatments including SRMT and SMPR can even raise the emergence rate. This means improved and region-adjusted CT treatments effectively overcome the seed emergence limitations. We also conclude that the high residues-covered CT treatments obstructed the maize grown in the early growing season including the V3 and V6 period, while the crop growth in these treatments caught up with the conventional tillage in the following growing periods, in which SMWRST showed the best performance during the dough stage. Our study suggested that well-adjusted CT treatments in Northeast China have the potential to maintain increase crop production accompanied by enhancing the ecosystem’s multifunctionality. In the future, multi-year observations across different experiments are required to evaluate the long-term gains from both productivity and environmental benefits.
Acknowledgments
This study was supported by the Strategic Priority Research Program (A) of the Chinese Academy of Sciences (XDA28080503), the National Natural Science Foundation of China (42071025), the Youth Innovation Promotion Association of Chinese Academy of Sciences (2023240) and the Scientific and Technological Development Program of Jilin Province (20220101154JC). We thank Aizhen Liang, Dandan Huang, Yan Zhang, and Xuewen Chen from the Northeast Institute of Geography and Agroecology, Chinese Academy of Sciences, for their guidance of detailed information about each conservational tillage technology in this study.
Disclosure statement
The authors declare that they have no known competing financial interests or personal relationships that could have appeared to influence the work reported in this paper.
Additional information
Funding
References
- Alvarez, R., & Steinbach, H. S. (2009). A review of the effects of tillage systems on some soil physical properties, water content, nitrate availability and crops yield in the Argentine Pampas. Soil and Tillage Research, 104(1), 1–17. https://doi.org/10.1016/j.still.2009.02.005
- Baudron, F., Tittonell, P., Corbeels, M., Letourmy, P., & Giller, K. E. (2012). Comparative performance of conservation agriculture and current smallholder farming practices in semi-arid Zimbabwe. Field Crops Research, 132, 117–128. https://doi.org/10.1016/j.fcr.2011.09.008
- Bouwman, T. I., Andersson, J. A., & Giller, K. E. (2021). Adapting yet not adopting? Conservation agriculture in central Malawi. Agriculture, Ecosystems & Environment, 307, 107224. https://doi.org/10.1016/j.agee.2020.107224
- Drury, C. F., Tan, C. S., Welacky, T. W., Oloya, T. O., Hamill, A. S., & Weaver, S. E. (1999). Red clover and tillage influence on soil temperature, water content, and corn emergence. Agronomy Journal, 91(1), 101–108. https://doi.org/10.2134/agronj1999.00021962009100010016x
- Eeswaran, R., Nejadhashemi, A. P., Kpodo, J., Curtis, Z. K., Adhikari, U., Liao, H. S., Li, S. G., Hernandez-Suarez, J. S., Alves, F. C., Raschke, A., & Jha, P. K. (2021). Quantification of resilience metrics as affected by conservation agriculture at a watershed scale. Agriculture, Ecosystems & Environment, 320, 107612. https://doi.org/10.1016/j.agee.2021.107612
- Elith, J., Leathwick, J. R., & Hastie, T. (2008). A working guide to boosted regression trees. Journal of Animal Ecology, 77(4), 802–813. https://doi.org/10.1111/j.1365-2656.2008.01390.x
- Fabrizzi, K. P., Garcia, F. O., Costa, J. L., & Picone, L. I. (2005). Soil water dynamics, physical properties and corn and wheat responses to minimum and no-tillage systems in the southern Pampas of Argentina. Soil and Tillage Research, 81(1), 57–69. https://doi.org/10.1016/j.still.2004.05.001
- FAO. (2014). What is conservation agriculture? FAO CA website. http://www.fao.org/ag/ca/1a.html.
- Friedman, J. H. (2001). Greedy function approximation: A gradient boosting machine. The Annals of Statistics, 29(5), 1189–1232. https://doi.org/10.1214/aos/1013203451
- Holland, J. M. (2004). The environmental consequences of adopting conservation tillage in Europe: Reviewing the evidence. Agriculture, Ecosystems & Environment, 103(1), 1–25. https://doi.org/10.1016/j.agee.2003.12.018
- Jia, K., Wu, B., Tian, Y., Li, Q., & Du, X. (2011). An effective biophysical indicator for opium yield estimation. Computers and Electronics in Agriculture, 75(2), 272–277. https://doi.org/10.1016/j.compag.2010.12.003
- Kassam, A., Friedrich, T., & Derpsch, R. (2019). Global spread of conservation agriculture. International Journal of Environmental Studies, 76(1), 29–51. https://doi.org/10.1080/00207233.2018.1494927
- Kassam, A., Friedrich, T., & Derpsch, R. (2022). Successful experiences and lessons from conservation agriculture worldwide. Agronomy-Basel, 12(4), 769. https://doi.org/10.3390/agronomy12040769
- Komarek, A. M., Thierfelder, C., & Steward, P. R. (2021). Conservation agriculture improves adaptive capacity of cropping systems to climate stress in Malawi. Agricultural Systems, 190, 103117. https://doi.org/10.1016/j.agsy.2021.103117
- Landers, J. N., de Freitas, P. L., de Oliveira, M. C., Neto, S. P. D., Ralisch, R., & Kueneman, E. A. (2021). Next steps for conservation agriculture. Agronomy-Basel, 11(12), 2496. https://doi.org/10.3390/agronomy11122496
- Liang, S. L., Kustas, W., Schaepman-Strub, G., & Li, X. W. (2010). Impacts of climate change and land use changes on land surface radiation and energy budgets. IEEE Journal of Selected Topics in Applied Earth Observations and Remote Sensing, 3(3), 219–224. https://doi.org/10.1109/JSTARS.2010.2053570
- Liu, P., He, J., Li, H., Wang, Q., Lu, C., Zheng, K., Liu, W., Zhao, H., & Lou, S. (2019). Effect of straw retention on crop yield, soil properties, water use efficiency and greenhouse gas emission in China: A meta-analysis. International Journal of Plant Production, 13(4), 347–367. https://doi.org/10.1007/s42106-019-00060-w
- Mogili, U. M. R., & Deepak, B. B. V. L. (2018). Review on application of drone systems in precision agriculture. Procedia Computer Science, 133, 502–509. https://doi.org/10.1016/j.procs.2018.07.063
- Mupangwa, W., Nyagumbo, I., Liben, F., Chipindu, L., Craufurd, P., & Mkuhlani, S. (2021). Maize yields from rotation and intercropping systems with different legumes under conservation agriculture in contrasting agro-ecologies. Agriculture, Ecosystems & Environment, 306, 107170. https://doi.org/10.1016/j.agee.2020.107170
- Nouri, A., Yoder, D. C., Raji, M., Ceylan, S., Jagadamma, S., Lee, J., Walker, F. R., Yin, X. H., Fitzpatrick, J., Trexler, B., Arelli, P., & Saxton, A. M. (2021). Conservation agriculture increases the soil resilience and cotton yield stability in climate extremes of the southeast US. Communications Earth & Environment, 2(1). https://doi.org/10.1038/s43247-021-00223-6
- Ogle, S. M., Swan, A., & Paustian, K. (2012). No-till management impacts on crop productivity, carbon input and soil carbon sequestration. Agriculture, Ecosystems & Environment, 149, 37–49. https://doi.org/10.1016/j.agee.2011.12.010
- Palm, C., Blanco-Canqui, H., DeClerck, F., Gatere, L., & Grace, P. (2014). Conservation agriculture and ecosystem services: An overview. Agriculture, Ecosystems & Environment, 187, 87–105. https://doi.org/10.1016/j.agee.2013.10.010
- Petito, M., Cantalamessa, S., Pagnani, G., Degiorgio, F., Parisse, B., & Pisante, M. (2022). Impact of conservation agriculture on soil erosion in the annual cropland of the Apulia Region (Southern Italy) based on the RUSLE-GIS-GEE framework. Agronomy-Basel, 12(2), 281. https://doi.org/10.3390/agronomy12020281
- Phillips, R. E., Blevins, R. L., Thomas, G. W., Frye, W. W., & Phillips, S. H. (1980). No-tillage agriculture. Science: Advanced Materials and Devices, 208(4448), 1108–1113. https://doi.org/10.1126/science.208.4448.1108
- Pittelkow, C. M., Linquist, B. A., Lundy, M. E., Liang, X. Q., van Groenigen, K. J., Lee, J., van Gestel, N., Six, J., Venterea, R. T., & van Kessel, C. (2015). When does no-till yield more? A global meta-analysis. Field Crops Research, 183, 156–168. https://doi.org/10.1016/j.fcr.2015.07.020
- Rusinamhodzi, L., Corbeels, M., van Wijk, M. T., Rufino, M. C., Nyamangara, J., & Giller, K. E. (2011). A meta-analysis of long-term effects of conservation agriculture on maize grain yield under rain-fed conditions. Agronomy for Sustainable Development, 31(4), 657–673. https://doi.org/10.1007/s13593-011-0040-2
- Soane, B. D., Ball, B. C., Arvidsson, J., Basch, G., Moreno, F., & Roger-Estrade, J. (2012). No-till in northern, western and south-western Europe: A review of problems and opportunities for crop production and the environment. Soil and Tillage Research, 118, 66–87. https://doi.org/10.1016/j.still.2011.10.015
- Su, Y., Gabrielle, B., & Makowski, D. (2021). The impact of climate change on the productivity of conservation agriculture. Nature Climate Change, 11(7), 628–633. https://doi.org/10.1038/s41558-021-01075-w
- TerAvest, D., Carpenter-Boggs, L., Thierfelder, C., & Reganold, J. P. (2015). Crop production and soil water management in conservation agriculture, no-till, and conventional tillage systems in Malawi. Agriculture, Ecosystems & Environment, 212, 285–296. https://doi.org/10.1016/j.agee.2015.07.011
- Thierfelder, C., Chisui, J. L., Gama, M., Cheesman, S., Jere, Z. D., Bunderson, W. T., Eash, N. S., & Rusinamhodzi, L. (2013). Maize-based conservation agriculture systems in Malawi: Long-term trends in productivity. Field Crops Research, 142, 47–57. https://doi.org/10.1016/j.fcr.2012.11.010
- Thierfelder, C., & Mhlanga, B. (2022). Short-term yield gains or long-term sustainability? – a synthesis of conservation agriculture long-term experiments in Southern Africa. Agriculture Ecosystems & Environment, 326, 107812. https://doi.org/10.1016/j.agee.2021.107812
- Thierfelder, C., Rusinamhodzi, L., Ngwira, A. R., Mupangwa, W., Nyagumbo, I., Kassie, G. T., & Cairns, J. E. (2015). Conservation agriculture in Southern Africa: Advances in knowledge. Renewable Agriculture and Food Systems, 30(4), 328–348. https://doi.org/10.1017/S1742170513000550
- Van den Putte, A., Govers, G., Diels, J., Gillijns, K., & Demuzere, M. (2010). Assessing the effect of soil tillage on crop growth: A meta-regression analysis on European crop yields under conservation agriculture. The European Journal of Agronomy, 33(3), 231–241. https://doi.org/10.1016/j.eja.2010.05.008
- Wittwer, R. A., Bender, S. F., Hartman, K., Hydbom, S., Lima, R. A. A., Loaiza, V., Nemecek, T., Oehl, F., Olsson, P. A., Petchey, O., Prechsl, U. E., Schlaeppi, K., Scholten, T., Seitz, S., Six, J., & van der Heijden, M. G. A. (2021). Organic and conservation agriculture promote ecosystem multifunctionality. Science Advances, 7(34). https://doi.org/10.1126/sciadv.abg6995
- Xiao, L. G., Kuhn, N. J., Zhao, R. Q., & Cao, L. H. (2021). Net effects of conservation agriculture principles on sustainable land use: A synthesis. Global Change Biology, 27(24), 6321–6330. https://doi.org/10.1111/gcb.15906
- Xu, P., Li, G., Houlton, B. Z., Ma, L., Ai, D., Zhu, L., Luan, B., Zhai, S. Q., Hu, S. Y., Chen, A. P., & Zheng, Y. (2022). Role of organic and conservation agriculture in ammonia emissions and crop productivity in China. Environmental Science & Technology, 56(5), 2977–2989. https://doi.org/10.1021/acs.est.1c07518
- Yang, S., Yang, X., & Mo, J. (2018). The application of unmanned aircraft systems to plant protection in China. Precision Agriculture, 19(2), 278–292. https://doi.org/10.1007/s11119-017-9516-7
- Yu, L., Liu, Y., Liu, T., & Yan, F. (2020). Impact of recent vegetation greening on temperature and precipitation over China. Agricultural and Forest Meteorology, 295, 108197. https://doi.org/10.1016/j.agrformet.2020.108197
- Yu, L., Liu, Y., Liu, T., Yu, E., Bu, K., Jia, Q., Shen, L., Zheng, X., & Zhang, S. (2022). Coupling localized Noah-MP-Crop model with the WRF model improved dynamic crop growth simulation across Northeast China. Computers and Electronics in Agriculture, 201, 107323. https://doi.org/10.1016/j.compag.2022.107323
- Yu, L. X., & Liu, T. X. (2019). The impact of artificial wetland expansion on local temperature in the growing season—the case study of the Sanjiang Plain, China. Remote Sensing, 11(24), 2915. https://doi.org/10.3390/rs11242915
- Yu, L. X., Liu, T. X., Bu, K., Yan, F. Q., Yang, J. C., Chang, L. P., & Zhang, S. W. (2017). Monitoring the long term vegetation phenology change in Northeast China from 1982 to 2015. Scientific Reports, 7(1). https://doi.org/10.1038/s41598-017-14918-4
- Zhang, C., & Kovacs, J. M. (2012). The application of small unmanned aerial systems for precision agriculture: A review. Precision Agriculture, 13(6), 693–712. https://doi.org/10.1007/s11119-012-9274-5
- Zhang, T. N., Xiong, W., Sapkota, T. B., Jat, M. L., Montes, C., Krupnik, T. J., Jat, R. K., Karki, S., Nayak, H., Al Faisal, A., & Jat, H. S. (2022). The optimization of conservation agriculture practices requires attention to location-specific performance: Evidence from large scale gridded simulations across South Asia. Field Crops Research, 282, 282. https://doi.org/10.1016/j.fcr.2022.108508
- Zhang, Y., Gao, Y., Zhang, Y., Huang, D. D., Li, X. J., Gregorich, E., McLaughlin, N., Zhang, X. P., Chen, X. W., Zhang, S. X., Liang, A. Z., & Xiang, Y. (2022). Effect of long-term tillage and cropping system on portion of fungal and bacterial necromass carbon in soil organic carbon. Soil and Tillage Research, 218, 105307. https://doi.org/10.1016/j.still.2021.105307
- Zhang, Y. J., Tan, C. J., Wang, R., Li, J., & Wang, X. L. (2021). Conservation tillage rotation enhanced soil structure and soil nutrients in long-term dryland agriculture. The European Journal of Agronomy, 131, 126379. https://doi.org/10.1016/j.eja.2021.126379