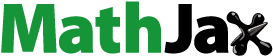
Abstract
Processed beans are rich in nutrients and aid in improving household diets as they contain nutritional elements like protein, dietary fibre, and minerals while saving time and money. However, the consumption frequency for processed beans is still low among households in Machakos County. This study aims to determine the factors influencing consumption frequency for precooked bean products in Machakos County, Kenya. A sample of 385 respondents was obtained using a multistage sampling procedure. Results from the zero-inflated negative binomial regression model revealed that age, presence of children under 5 years in the household, household health, and nutrition knowledge significantly and positively influenced the frequency of consumption of precooked bean products. Further, the results revealed that the frequency of consumption of precooked bean products was significantly and negatively influenced by household size and employment status as well as the colour of these bean products. Given the positive and significant influences of nutrition knowledge on consumption frequency, an emphasis on the economic and health benefits of precooked bean products is needed to increase the consumption frequency for these products. Additionally, investing in formal education could increase the consumption frequency for precooked bean products over time.
1. Introduction
Many developing nations struggle with protein-calorie malnutrition (Kadam et al., Citation2009; Semba, Citation2016), which is primarily caused by insufficient intake of energy, protein, and micronutrients (Ahmed et al., Citation2020). Consumption of traditional protein sources like the common bean is essential to improve nutrition and enhance protein supply for the expanding population. According to Kotue et al. (Citation2018), common bean is a source of food with the highest nutrition value. It is a staple food crop that is significant for both economic and nutritional reasons. As the price of meat, fruits, and vegetables continue to rise, it is projected that the common bean will contribute more to human nutrition (Deshpande et al., Citation2009). Moloto et al. (Citation2018), notes that the common bean has historically contributed significantly to the diets of many individuals in several countries throughout Asia, Africa, the Middle East, and South America and has been recognized to be a “poor man’s meat”.
Consumption of recommended levels of legume crop, mostly common bean, is heralded as critical in addressing obesity pandemic in developing countries (Thompson et al., Citation2017; Tucker, Citation2020). According to Winham et al. (Citation2008) and Messina (Citation2014), beans have a nutritional profile that is suitable for all ages because they are rich in protein and carbohydrates and have a low fat content. Children who consume beans derive significant amounts of fibre, magnesium, and potassium than those who do not consume (Winham et al., Citation2008). In addition, teenagers who consume beans weigh significantly less and have smaller waist measurements than those who do not consume (Fulgoni et al., Citation2006). Moreover, adults who want to reduce the amount of fat and cholesterol in their diet may replace meat with beans as a healthy alternative (Winham & Hutchins, Citation2007). Studies have established a connection between frequent bean consumption and improved health, as well as the prevention and management of chronic diseases such as cancer and coronary heart disease (Ganesan & Xu, Citation2017; Winham et al., Citation2016). Consuming diets high in beans lowers low-density lipoprotein cholesterol, improves metabolic syndrome risk factors, and lowers the risk of developing diabetes and ischemic heart disease (Messina, Citation2014). According to Nyau (Citation2015), the numerous phytochemicals in beans, such as polyphenols, which have both anticarcinogenic and antioxidant characteristics, could be the cause of the physiological effects
Health organizations have included information regarding beans in their nutritional guidelines, including suggestions designed specifically to help prevent cancer and heart disease. According to a report by the World Cancer Research Fund and the American Institute for Cancer Research (1997), all individuals should adopt a diet that is predominantly plant-based and plentiful in a variety of vegetables and fruits. The report specifically mentioned legumes like beans as being crucial to the diet. Compared to the 540 g per week recommended in the Dietary Guidelines for Americans from 2005, the 2010 guideline suggested consuming 270 g of beans per week to reap these potential health benefits. Additionally, the World Health Organization (WHO) (Citation2019) recommends a consumption level of 160 g of beans per day among adults.
Despite the nutritional and health benefits of common beans, their consumption has been limited by their prolonged cooking times and high energy and water requirements (Ugen et al., Citation2017). According to Syanda (Citation2019), the preparation of common beans for consumption is limited by their hardness caused by the permeability of the seed coat, which leads to prolonged cooking times and high fuel costs. To reduce the cooking time, the dried beans are subjected to high-pressure hydration during processing without compromising the bean quality. Dry beans with suitable industrial characteristics are selected for processing. The processed beans are quick to cook, tasty, and nutritious (Nakitto et al., Citation2015), and they also generate less flatulenceFootnote1 (Rose et al., Citation2021). Dalberg (Citation2019) states that processed beans provide all the nutrition of common beans while saving households with over 1 hour and 40 min of cooking time and USD 0.48 per kilogram in fuel expenses.
Precooked bean (PCB) varieties are processed from selected dry beans which are from red mottled sugar and yellow bean families. Dry beans undergo hydration, cooking and sterilization, and dehydration to produce PCBs. The dry bean varieties were selected for production and industrial precooking based on their cooking time, colour, micronutrient content, and ease of growing (IDRC. CRDI, Citation2019). Some of the PCB grains are packed in airtight sachets, and the remaining is further processed to produce three other products: bean flour, bean noodles, and bean snack. Packed PCB grains can be reheated in 15 min before consumption, compared to 2 hours required for raw beans. According to Ugen et al. (Citation2021), introduction of the PCB products has led to a significant shift from the use of firewood to gas and charcoal. The aforementioned study found that 68% of the consumers used charcoal, 14% firewood, and 17% gas to cook non-processed dry beans. For PCB products, however, 42% used charcoal and 46% used gas. Thus, the use of clean energy, gas, instead of wood-based fuel is becoming more prevalent when PCB products are being consumed. Furthermore, when PCB products are prepared households saves 75% water relative to cooking dry beans. Additionally, Aseete et al. (Citation2018), in their study found that consumers obtain high value from a PCB product due to reduced cooking time, and hence water and fuel savings. Furthermore, the demand for PCB products is driven by the desire for cost savings and convenience. However, Ugen et al. (Citation2021), notes that the consumption of PCB products is still relatively low despite these apparent benefits.
Previous studies have examined the consumption frequency and heterogeneity in the frequency of common beans (Medard, Citation2017; Winham et al., Citation2019). They have also investigated the frequency of bean consumption for canned beans and bio-fortified common beans (Mwangwela et al., Citation2021; Winham et al., Citation2019). However, information on the frequency of PCB products has not been documented. The current study is intended to fill the highlighted gap by evaluating factors influencing consumption frequency for PCB products in Kenya. From the findings of this study, policy-makers could use the information to urge individuals to adopt healthier diets, such as PCB products.
2. Materials and Methods
This study was conducted in Machakos Town Sub County, in Machakos County, Kenya. Machakos County spans 6208.2 km2, with an estimated population of 1,098,584 people (KNBS, Citation2019). It is located between longitudes 36°45′ East and 37°45′ East and latitudes 0°45′ South and 1°31′ South. Machakos County has a tiny peri-urban area between it and Nairobi County, which is quickly urbanizing. The majority of residents of Machakos County rely on wood for their cooking energy, which has led to deforestation, which in turn has led to low supply of both food and water (MoALF, Citation2017). As a result, the water cycle has been disrupted lowering the region’s crop productivity.
This study sought to determine the factors influencing consumption frequency for PCB products. The study interviewed both consumer and non-consumer households of PCB products. It is aimed at households that used these products in 2020, thus, relied on recall information from consumer households. The PCB products examined were packed beans, bean flour, bean noodles, and bean snacks. Multistage sampling was used to obtain this sample of responders. The first stage involved purposive selection of Machakos County because processing of PCB products is mainly done in this county. Machakos Sub County was then selected because it has benefited from bean research intervention by KALRO. In the third stage, a systematic sampling technique was used to select 193 consumers of PCB products from a list of consumers obtained from KALRO. Finally, to choose 192 non-consumers of PCB products, a systematic sample technique was used, and respondents in every fourth household were interviewed. As part of this, it was first determined whether the respondent was a non-consumer, and if not, interviews were conducted with respondents from the immediate household. Using Cochran’s (Citation1977) sample size approach, data from 385 respondents was collected for the study. The respondents were distributed in the seven wards of Machakos town sub county (Table ) according to data from KNBS (Citation2019).
Table 1. Distribution of sample size in the wards in Machakos town sub county, Kenya
Each participant completed a consent form, and the permission notice mentioned maintaining the responders’ anonymity. A semi-structured questionnaire was used to collect both quantitative and qualitative data. The survey collected information on PCB product markets, product components, socioeconomic variables, and household consumption patterns. Trained enumerators conducted in-person interviews to collect the study’s data.
The number of times a household consumed PCB products in a typical month was the dependent variable in this study. Therefore, the outcome variable for this purpose was a count greater than zero if a household used PCB products and zero otherwise. The study adopted the Poisson probability distribution model for the analysis because of its suitability for a count data outcome than Ordinary Least Squares (OLS) regression. The parameter estimate using OLS would be inaccurate and ineffective if the resulting variable was not negative (Mwololo et al., Citation2019).
The positive and discrete aspect of the count outcome variable is captured by the Poisson distribution, which also permits inference based on the likelihood of occurrence, according to Mwololo et al. (Citation2019). The equal dispersion assumption in the model also allows for skewness in count data. The probability density function of the Poisson regression model can be defined as;
where is the random Poisson variable,
is the number of times a household consumed a given product,
is a vector of explanatory variables, and
is the expected mean parameter of the probability function.
The Poisson model assumes equal data dispersion, that is, the variance function is equal to the conditional mean of the data. However, in most real-world data, non-consumption results in extra zeros and over-dispersion (Bekalo & Kebede, Citation2021). According to Tüzen and S (Citation2017), there are two categories of excess zeroes: sampling zeroes (imperfect zeroes) and structural zeroes (perfect zeroes). Perfect zeros come from a latent class for which zeros are the only possible outcome, while imperfect zeros originate from a latent class where zeros are only feasible due to the sampling process. The non-consumers or consumers who chose not to utilize these products during the study period are responsible for the extra zeros in the data used for this study. That is, the non-consumers are responsible for imperfect zeros, while consumers who did not consume PCB products during the study reference period were responsible for the perfect zeros.
In count data with excess zeros, the usual Poisson model yields consistent but inefficient estimates, which overstate or invalidate standard errors and result in incorrect inference (Mahama et al., Citation2020). To overcome this, a Negative Binomial Regression (NBR) model could be utilized (Mwololo et al., Citation2019; Olawuyi & Mushunje, Citation2019). The NBR model incorporates a dispersion parameter to account for unexplained variations in the count data, hence addressing over-dispersion (Tüzen & S, Citation2017). The probability mass function for the NBR is;
where is the probability parameter of success and
is the inverse of the dispersion parameter.
The NBR distribution may account for the over-dispersion due to the additional dispersion parameter, which lets the variance exceed the mean. NBR predicts more zeros than the standard Poisson model when low-mean events are considered. In some circumstances, the predominance of zero counts is a significant source of over-dispersion, and the ensuing over-dispersion is not sufficiently captured by the NBR model (Bekalo & Kebede, Citation2021). In these circumstances, zero-inflated Poisson (ZIP) or zero-inflated negative binomial (ZINB) models can be used to fit the data.
The ZIP model assumes that data is produced by two distinct processes, one of which only generates perfect zero counts and the other generates both zero and non-zero counts from the not-always-zero group (Tüzen & S, Citation2017). Therefore, ZIP integrates the two modeling approaches: logistic regression component forecasts the existence or absence of outcome resulting from structured zeros, and Poisson regression component forecasts the magnitude of the count outcome, which may also include zero counts. Similar to how ZIP and the traditional Poisson model relate, the Zero Inflated Negative Binomial (ZINB) is an expansion of the NBR model. ZINB combines NBR model and logistic regression model. The logistic regression model predicts the extra zeros, while the NBR model estimates positive counts and random zeros. The zero-inflated models are therefore represented as follows;
Where is the uncertainty parameter, which is the mixing proportion,
is the expected mean parameter and
stands for the count distribution.
To determine factors that influence consumption frequency for PCB products, ZINB model was used in this study. Previous studies (Gido et al., Citation2017; Pittman et al., Citation2018; Sheu et al., Citation2004; Tüzen & S, Citation2017) have used the ZINB model in their analysis. The choice of variables used in this study (Figure ) was based on previous literature (Aseete et al., Citation2018; Jolly et al., Citation2008; Liu et al., Citation2020; Saghaian & Mohammadi, Citation2018) and variables of interest in this study. These factors have been classified into three groups: institution and market factors, product-related attributes, and socioeconomic characteristics of households.
3. Results and discussion
3.1. Descriptive results
3.1.1. Socioeconomic characteristics of respondents in Machakos County
Age
The study results revealed that the mean age of decision makers in consumer households was approximately 41 years, while that of those in non-consuming households was averagely 42 years (Table ). This suggests that the high nutritional value of bean-based products promotes healthy ageing, thus, appealing to middle-aged respondents. These results corroborate with those of Lutomia et al. (Citation2021) who found that the average age of the net bean consumers in Machakos County was 42. However, two-tailed t-test results indicate that age was statistically insignificant implying that both consumer and non-consumer households’ decision makers did not have significant differences in age.
Education
On average, decision makers in consumer households of PCB products had 12 years of education, while that of the non-consumer households was 11 years. Two-tailed t-test results indicate that education is statistically significant at 1% implying that decision makers in consumer households had spent more years in school compared to those in non-consuming households. Individuals with higher education levels are associated with consuming healthier diets as compared to those with low education levels who are associated with consuming diets high in carbohydrates and low in fibre (Fard et al., Citation2021).
Household size
The mean household size was approximately three members for consumer households of PCB products and approximately six members for non-consumer households (Table ). Two tailed t-test results indicate that household size was statistically significant at 1% implying that household size determines the choice of bean products to consume among the consumers. PCB products are perceived to be expensive (Mukamugema et al., Citation2019) than the common dry beans, implying that larger households would spend more on a prepared PCB meal. Consistent with this finding are those of Aseete et al. (Citation2018) who found that PCB enthusiasts had smaller household sizes.
Children below 5 years
The mean number of children below the age of five in consumer households of PCB products was 2, while that in non-consumer households was 1. Two tailed t-test results indicate that children below the age of five were statistically significant at 5% implying that consumer households had more children below 5 years compared to non-consumer households. The PCB products have abundant proteins, vitamins, and minerals which are essential for a child’s growth and development.
Monthly income
The mean income for decision makers in consumer households was approximately 18,666 Kshs and 12,246 Kshs for those in non-consuming households. Two tailed t-test results indicate that monthly income was statistically significant at 1% implying that decision makers in consumer households had higher monthly income compared to those in non-consuming households. This implies that households with higher income levels have a higher purchasing power compared to those with lower income levels. These findings support those of Dominguez-Viera et al. (Citation2022), who found that higher-income consumers have a higher willingness to pay for healthy varieties of processed foods compared to low-income consumers.
Market distance
The mean distance from home to the nearest market for consumer households was 1.3 km while that for non-consumer households was 2.5 km. Two tailed t-test results indicate that market distance was statistically significant at 1% implying that consumer households of PCB products have their homesteads located near a market compared to non-consumer households. This suggests that a large market distance could limit consumption of PCB products.
Health status
The mean number of household members living with a medical condition in consumer households was 1. The number of individuals living with a medical condition was used as a proxy measure for household health status. Two tailed t-test results indicate that health status was statistically significant at 1% implying that consumer households had more family members with medical conditions than non-consumer households. Bean-based products are packed with nutrients and are a healthy substitute for animal protein.
Gender
Females (53%) make up the majority of decision makers in consumer households of PCB products, whereas males are the majority in non-consumer households (Table ). Chi-square results indicate that gender is statistically significant at 10% implying that there are more female decision makers in consumer households of PCB products. In most households, women prepare the majority of the meals (Schaeffer, Citation2019). Thus, the use of PCB products liberates women from spending a significant amount of time on meals preparation. This suggests that women can use their free time to complete other household tasks and engage in other productive activities.
Table 2. Continuous characteristics of respondents in Machakos County, Kenya
Employment status
The proportion of decision makers in consumer households who are in employment was 77% and those in non-consuming households was 51%. Chi-square results show that employment was statistically significant at 1% indicating that more decision makers in consumer households of PCB products were in employment. Possible explanation could be that individuals who are in employment might have little time to prepare meals, so majority of them prefer PCB products to dry beans.
Group membership
The proportion of decision makers in consumer households who are in social groups was 76% and those in non-consuming households was 75%. Social groups have an impact on individual’s purchasing decisions and are a continuous, active source of information about various consumption values (Niosi, Citation2021). However, chi-square results reveal that group membership was statistically insignificant, indicating that there were no significant differences between decision makers who belong to social groups in consumer and non-consumer households.
3.2. Consumption frequency for PCB products in Machakos County, Kenya
Descriptive statistics (Table ) reveal that packed beans are the most consumed PCB product, that is 4.15 times per month, followed by bean flour 3.12 times on average. Further interrogation revealed that the packed PCBs are utilized in the preparation of githeri, a staple meal in Machakos County made from a mixture of beans and maize. Additionally, the respondents disclosed that they prepare bean stew, which is served as an accompaniment to main meals such as rice and stiff porridge (ugali), which explains why they are consumed more frequently than other PCB products.
Table 3. Categorical characteristics of respondents in Machakos County, Kenya
3.3. Factors influencing consumption frequency for PCB products
The goodness-of-fit of the four-count data models was compared and evaluated using the likelihood ratio test (LR), the Vuong test, the ZIP option test, the Akaike information criterion (AIC), and the Bayesian information criterion (BIC). Poisson model’s deviation and Pearson goodness of fit were first calculated. Both categories of goodness of fit were significant revealing that there was over dispersion of data. To evaluate the degree of over-dispersion of data, the two categories of goodness of fit were divided by degrees of freedom. The ratios were noticeably higher than one, which showed that the data was excessively distributed. As an additional proof of over-dispersion, the NBR model was performed, which demonstrated that there was over-dispersion of data because alpha was greater than zero. NBR would have been a better model fit, as evidenced by the fact that its AIC and BIC results were much lower than those for Poisson. The zero-inflated model was then run. Significant results from the z-test for Vuong demonstrated that ZINB performs better than NBR model in the underlying data-generating process, demonstrating that both perfect and imperfect states for excess zeros were certainly in operation. Because ZINB had a significant LR test alpha of zero, it was more accurate at fitting data than ZIP. ZINB was utilized in the current study to determine the factors influencing consumption frequency for PCB products. Due to the heteroscedasticity issue with the data, the ZINB model was run with the robust option to provide robust standard errors.
Table shows the ZINB model results of factors influencing consumption frequency for PCB products. The NBR model’s findings, which are presented in the first section of the table, highlight the factors influencing the likelihood of households to be in imperfect state. The study results showed that there is a positive and significant relationship between the age of the decision-maker and the consumption frequency for PCB products. Older respondents are more likely to often consume PCB products than their younger counterparts. Older people are more cognizant of the significance of including beans in their diets to promote healthy aging (Doma et al., Citation2019). The nutritional value of beans was a crucial factor that the study found to be prevalent among older bean consumers. Similarly, Wuenstel et al. (Citation2016), reported the same results where teenagers between the ages of 13 and 18 were found to have lower mean bean intake values and lower bean consumption frequencies.
Table 4. Monthly consumption frequencies for PCB products
As shown in Table , educational background significantly and positively influenced consumption frequency for PCB products. Individuals with more years of education were more likely to consume PCB products frequently than those with fewer years of schooling. This is because, compared to people with lower levels of education, they were found to be more aware of the nutritional and health benefits of including beans in their meals, hence, expected to make healthier eating decisions. According to Bellisle (Citation2003), education aid in understanding a food product’s dietary information and effects on health. Additionally, those with higher education levels adopt new products faster than those with lower education levels, which may impact the effectiveness of interventions connected to health (Worsley et al., Citation2004). These results conform with those of Fard et al. (Citation2021), who found a positive relationship between higher educational attainment and a healthier diet.
Table 5. ZINB results on consumption frequency for PCB products
Household size had a significant, negative impact on how often PCB products were consumed. According to Tiffany (Citation2020), PCB products are expensive than the common beans; thus, a large household will use more of these goods to prepare a meal, which results in higher food expenses. This finding corroborates the finding of Aseete et al. (Citation2018), who found that PCB enthusiasts had small households, demonstrating a significant negative relationship between household size and PCB product consumption frequency.
The presence of children under the age of five in a family had a significant impact on how frequently PCB products were consumed. Children need a well-balanced diet full of all the vital vitamins, minerals, and other nutrients for healthy growth. Families can provide a balanced diet for their children and other household members by including PCB products in their meals. According to Saito et al. (Citation2018), Japanese married women’s intake of various minerals and macronutrients is influenced by the presence of young children in the home. These findings support those of Nambiar and Florkowski (Citation2013), who found that having children in the home significantly and positively affects how often people consume peanut butter paste with other foods in Uganda.
The health status of a household was also found to positively and significantly influence the consumption frequency for PCB products. A household with high number of members with health condition is more likely to consume PCB products often. Beans are a significant protein source and are high in various crucial minerals, including potassium, magnesium, folate, iron, and zinc (Messina, Citation2014). Moreover, Messina (Citation2014) asserts that including beans in one’s diet can lower levels of low-density lipoprotein cholesterol, have a positive impact on risk factors for metabolic syndrome, and lower one’s chance of developing diabetes and ischemic heart disease. These results concur with those of Henn et al. (Citation2022), who discovered that the health advantages of pulses are among the important factors influencing consumption frequencies in the five European countries investigated.
A household whose decision-maker is in formal employment was found to significantly and negatively influence how often households consumed PCB products. In-depth probing revealed that those in employment have limited time to balance between work and household chores. According to Caswell and Yaktine (Citation2013), individuals in formal employment often get food in ways that are unsuitable for maintaining a balanced household diet, such as ordering takeout, and dining out. This means that households whose decision-maker is in formal employment are more likely not to frequently consume PCB products in a typical month. These results are in odds with those of Blekking et al. (Citation2020) who found that informally employed households had significantly lower food consumption frequency. The current study focused on the frequency of consumption of PCB products in the rural cites in Kenya, whereas Blekking et al. (Citation2020) focused on urban food security in Zambia, which explains the disparity in findings.
Nutritional information on PCB products was found to have a significant and positive impact on consumption frequency for these products. The PCB products are a good source of protein, carbohydrates, and minerals, hence, will likely be used more frequently by consumers who are aware of their nutritional benefits than by consumers who are unaware. According to Gámbaro et al. (Citation2011), nutritional awareness had a significant positive influence on the frequency of consumption of fruits, vegetables, and low-fat items, thus, supporting this study’s findings.
The study found that the colour of PCB products had a significant negative influence on the consumption frequency for the aforementioned products. These findings were consistent with those of Wahyudi et al. (Citation2019), who found that the colour of rice had a significant negative influence on the frequency of consumption of locally produced rice in Indonesia. However, Winham et al. (Citation2019), are in odds with these findings, as they found that respondents selected the common dry bean types based on the colour of the beans (p = 0.001), which affected how frequently they consumed bean meals. The variation in the findings can be attributed to the fact that Winham et al. (Citation2019) examined the frequency of dry bean consumption among Hispanic and non-Hispanic women, whereas this study examined the frequency of PCB product consumption among households in Machakos County, Kenya.
Results from the logistic regression are included in the last part of Table , along with factors that indicate the possibility that a household will be in a perfect state. The likelihood of consumption frequency for PCB products being positive was significantly and negatively influenced by household size. A large family is more likely to spend more money on food, so it is expected that they will consume common beans, a substitute for PCB products, to lower the cost of their meal. Hence, a large household was probably a true non-consumer of PCB products. A small household will probably consume PCB products, therefore lowering the likelihood of a count being a true zero. Monthly income was found to have a significant negative influence on the likelihood of a count being zero for how often PCBs are consumed. Households with high incomes are more likely to consume PCB products, reducing the likelihood that a count is truly zero.
Education level was also found to significantly and negatively influence the likelihood of consumption frequency for PCB products being positive. Households with higher levels of education are more likely to consume PCB products because they were found to be aware of their nutritional benefits, which reduces the possibility of consumption frequency being positive. Similarly, Gido et al. (Citation2017) found that education level decreased the likelihood of consumption intensity of leafy African Indigenous Vegetables (AIVs) being positive. Finally, the price perception of PCB products had a significant negative influence on the likelihood of a positive consumption frequency. Respondents who perceive PCB products affordable are more likely to consume these products, thus, decreasing the likelihood of a positive consumption frequency for the aforementioned products.
4. Conclusion
The findings of this study indicate that having knowledge on the nutritional composition of PCB products drives consumption frequency of these bean products. Additionally, education level positively affects how regularly a household consumes these products, probably as a result of knowledge of the health benefits associated with regular consumption of bean-based products. Further, the presence of a child as proxied by the number of children below 5 years in a household and the health status of the household motivates regular consumption of PCB products. Children, especially those below the age of five, require protein and various vitamins and minerals for development and growth which they can reap from regular intake of these bean products. Additionally, PCB products are a healthy food choice for individuals with medical conditions because they need calories, vitamins, minerals, and proteins to help with healing and disease management. However, the findings of this study indicate that regular consumption of PCB products is negatively affected by household size, probably due to the costs associated with meal preparation made from these products. In addition, colour negatively impacts regular consumption of PCB products. Surprisingly, employment status of the household head also negatively affects regular consumption of PCB products, likely as a result of limited time to balance between work and other household tasks. This study was conducted within a short duration of time. Future research on PCB products could consider studying household purchase behaviour for these products over a longer period of time.
This study recommends that nutritional education programs could consider offering PCB products to consumers as an accompaniment to main meals when developing policy and program strategies. In addition, these programs should emphasize on the health benefits of frequently consuming PCB products. Lastly, investing in formal education could improve the consumption frequency for PCB products over time.
Correction
This article has been corrected with minor changes. These changes do not impact the academic content of the article.
Disclosure statement
No potential conflict of interest was reported by the author(s).
Additional information
Funding
Notes on contributors
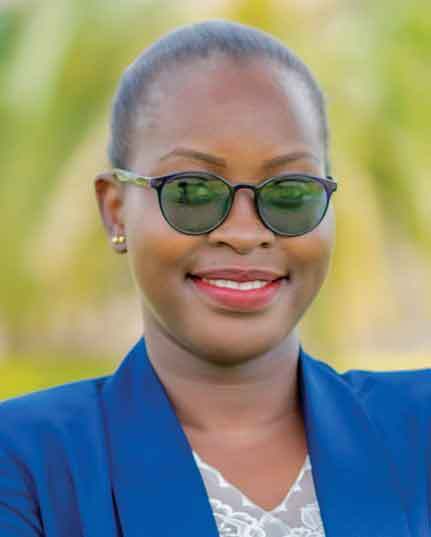
Judy Gatwiri Mutegi
Judy Gatwiri Mutegi is a master’s candidate in the Department of Agricultural Economics and Agribusiness Management, Egerton University. She received her Bsc. degree in Agricultural Economics from Laikipia University in 2017. Her research interest is in Food and Nutrition Security. The current research contributes to achieving sustainable development goal number 2 which advocates for ending hunger and achieving Food and Nutrition Security.
Notes
1. Flatulence is the condition of having gas buildup in the digestive tract.
References
- Ahmed, T. M., Hossain, I., Islam, M., Ahmed, A. M., Afroze, F., & Chisti, J. M. (2020). 143 - protein-energy malnutrition in children hunter’s tropical medicine and emerging infectious diseases (Tenth ed.). https://doi.org/10.1016/B978-0-323-55512-8.00143-5
- Aseete, P., Katungi, E., Bonabana-Wabbi, J., Birachi, E., & Ugen, M. (2018). Consumer demand heterogeneity and valuation of value-added pulse products: A case of precooked beans in Uganda. Agriculture & Food Security, 7(1). https://doi.org/10.1186/s40066-018-0203-3
- Bekalo, D. B., & Kebede, D. T. (2021). Zero-inflated models for count data: An application to number of antenatal care service visits. Annals of Data Science, 8(4), 683–17. https://doi.org/10.1007/s40745-021-00328-x
- Bellisle, F. (2003). Why should we study human food intake behaviour? Nutrition, Metabolism, and Cardiovascular Diseases, 13(4), 189–193. https://doi.org/10.1016/s0939-4753(03)80010-8
- Blekking, J., Waldman, K., Tuholske, C., & Evans, T. (2020). Formal/Informal employment and urban Food Security in sub-Saharan Africa. Applied Geography, 114, 102131. https://doi.org/10.1016/j.apgeog.2019.102131
- Caswell, J. A., & Yaktine, A. L. (2013). Committee on examination of the adequacy of food resources and SNAP allotments, food and nutrition board, committee on National Statistics. In Institute of Medicine, & National Research Council (Eds.), Supplemental Nutrition Assistance Program: Examining the Evidence to Define Benefit Adequacy. National Academies Press (US). PMID: 24901188.
- Cochran, W. (1977). Sampling Techniques (3rd ed.). John Willey & Sons. https://www.wiley.com/en-us/Sampling+Techniques%2C+3rd+Edition-p-9780471162407
- Dalberg. (2019). Commercialization assessment: High Iron beans in Kenya- Final report for gain and Harvest Plus (p. 37). https://nutritionconnect.org/sites/default/files/2020-01/191213_Kenya_Iron%20Beans_Report_vFINAL.pdf
- Deshpande, S. S., Shridhar, K., Sathe, D. K., Salunkhe, R., & Rackis, J. J. (2009). Dry beans of Phaseolus: A review. Part 3. C R C Critical Reviews in Food Science and Nutrition, 21(2), 137–195. https://doi.org/10.1080/10408398409527399
- Doma, K. M., Farrell, E. L., Leith-Bailey, E. R., Soucier, V. D., & Duncan, A. M. (2019). Motivators, Barriers and other factors Related to bean consumption in Older adults. Journal of Nutrition in Gerontologoy & Geriatrics, 38(4), 397–413. https://doi.org/10.1080/21551197.2019.1646690
- Dominguez-Viera, M. E., Berg, M., Donovan, J., Perez-Luna, M. E., Ospina-Rojas, D., & Handgraaf, M. (2022). Demand for healthier and higher-priced processed foods in low-income communities: Experimental evidence from Mexico city. Food Quality and Preference, 95, 104362. https://doi.org/10.1016/j.foodqual.2021.104362
- Fard, N., Morales, G., Mejova, Y., & Schifanella, R. (2021). On the interplay between educational attainment and nutrition: A spatially-aware perspective. EPJ Data Science, 10(1). https://doi.org/10.1140/epjds/s13688-021-00273-y
- Fulgoni, V. L., Papanikolaou, Y., Fulgoni, S. A., Kelly, R. M., & Rose, S. F. (2006). Bean consumption by children is associated with better nutrient intake and lower body weights and waist circumferences. American Society for Nutrition, 20(4), 621–621. https://doi.org/10.1096/fasebj.20.4.A621
- Gámbaro, A., Raggio, L., Dauber, C., Claudia Ellis, A., & Toribio, Z. (2011). Nutritional knowledge and consumption frequency of foods–a case study]. Archivos latinoamericanos de nutricion, 61(3), 308–315.
- Ganesan, K., & Xu, B. (2017). Polyphenol-rich dry common beans (Phaseolus vulgaris l.) and their health benefits. International Journal of Molecular Sciences, 18(11), 2331. https://doi.org/10.3390/ijms18112331
- Gido, O. E., Ayuya, O. I., George, O., & Bokelmann, W. (2017). Consumption intensity of Leafy African Indigenous vegetables: Towards Enhancing Nutritional Security in Rural and urban Dwellers in Kenya. Agricultural and Food Economics, 5(14). https://doi.org/10.1186/s40100-017-0082-0
- Henn, K., Olsen, S. B., Goddyn, H., Wender, L., & Bredie, P. (2022). Willingness to replace animal-based products with pulses among consumers in different European countries. Food Research International, 157, 111403. https://doi.org/10.1016/j.foodres.2022.111403
- IDRC. CRDI. (2019). Precooked beans for food, nutrition and income in Kenya and Uganda. https://idrc-crdi.ca/en/research-in-action/precooked-beans-food-nutrition-and-income-kenya-and-uganda
- Jolly, C., Awuah, R., Fialor, S., Agyemang, K., Kagochi, J., & Binns, A. (2008). Groundnut consumption frequency in Ghana. International Journal of Consumer Studies, 32(6), 675–686. https://doi.org/10.1111/j.1470-6431.2008.00697.x
- Kadam, S. S., Salunkhe, D. K., & Luh, B. S. (2009). Winged bean in human nutrition. C R C Critical Reviews in Food Science and Nutrition, 21(1), 1–40. https://doi.org/10.1080/10408398409527395
- KNBS. (2019). Kenya population and housing census volume I: Population by County and sub-County—kenya. National Bureau of Statistics. https://www.knbs.or.ke/?wpdmpro=2019-kenya-population-and-housing-census-volume-i-population-by-county-and-sub-county
- Kotue, T. C., Marlyne, J. M., & Wirba, L. Y. (2018). Nutritional properties and nutrients chemical analysis of common beans seed. MOJ Biology & Medicine, 3(2), 41–47. https://doi.org/10.15406/mojbm.2018.03.00074
- Liu, A., Li, J., & Gómez, M. (2020). Factors influencing consumption of edible insects for Chinese consumers. Insects, 11(1), 10. https://doi.org/10.3390/insects11010010
- Lutomia, C. K., Karanja, D., Nchanji, E. B., Induli, I., Mutuku, R., Gichangi, A., Mutuli, W., & Birachi, E. (2021). Consumer intentions to buy nutrient-rich precooked bean snacks: Does sensory evaluation matter? African Journal of Food Agriculture Nutrition & Development, 21(2), 17621–17642. https://doi.org/10.18697/ajfand.97.20210
- Mahama, A., Awuni, J. A., Mabe, F. N., & Azumah, S. B. (2020). Modelling adoption intensity of improved soybean production technologies in Ghana - a Generalized Poisson approach. Heliyon, 6(3), e03543. https://doi.org/10.1016/j.heliyon.2020.e03543
- Medard, E. (2017). Factors underlying common Beans’ (Phaseolus vulgaris L.) consumption decisions in Dar es Salaam, Tanzania. [ Master of Science Thesis], Submitted to Sokoine University of Agriculture. http://www.suaire.sua.ac.tz/handle/123456789/2113
- Messina, V. (2014). Nutritional and health benefits of dried beans. The American Journal of Clinical Nutrition, 100(1), 437–442. https://doi.org/10.3945/ajcn.113.071472
- MoALF. (2017). Climate risk profile for Machakos County. Kenya County Climate risk profile series. the Ministry of Agriculture, Livestock and Fisheries (MoALF). Cgiar. https://cgspace.cgiar.org/bitstream/handle/10568/96283/Machakosf_Climate_Risk_Profile_Final.pdf
- Moloto, M. R., Moremi, H., Soundy, P., & Maseko, S. T. (2018). Biofortification of common bean as a complementary approach to addressing zinc deficiency in South Africans. Acta Agriculturae Scandinavica, Section B — Soil & Plant Science, 68(7), 575–584. https://doi.org/10.1080/09064710.2018.1454507
- Mukamugema, A., Mshenga, P., & Birachi, E. (2019). Barriers to institutional adoption of new products innovation: a case of precooked beans among schools in Rwanda. Journal of Agribusiness and Rural Development, 52(2), 139–146. https://doi.org/10.17306/J.JARD.2019.01199
- Mwangwela, A., Mwachumu, M., & Banda, I. (2021). Cooking characteristics and consumer acceptability of bio-fortified beans. International Institute of Tropical Agriculture.
- Mwololo, H., Nzuma, J., Ritho, C., & Aseeta, A. (2019). Is the type of agricultural extension services a determinant of farm diversity?. https://www.tandfonline.com/doi/full/10.1080/21665095.2019.1580596
- Nakitto, M. A., Muyonga, J. H., & Nakimbugwe, D. (2015). Effects of Combined traditional processing Methods on the Nutritional Quality of beans. Food Science, 3(3), 233–241. https://doi.org/10.1002/fsn3.209
- Nambiar, P., & Florkowski, W. (2013). Peanut Paste/Butter consumption frequency in the Republic of Uganda: Count data model approach. Presentation, Southern Agricultural Economics Association Annual Meeting (SAEA), Orlando, FL.
- Niosi, A. (2021). Introduction to consumer behaviour. BCcampus. https://opentextbc.ca/introconsumerb
- Nyau, V. (2015). Nutraceutical perspectives and utilization of common beans (Phaseolus vulgaris L.): A review. African Journal of Food, Agriculture and Development, 14, 7. https://doi.org/10.18697/ajfand.66.12990
- Olawuyi, S. O., & Mushunje, A. (2019). Social Capital and Adoption of Alternative Conservation Agricultural Practices in South-Western Nigeria. Sustainability, 11(3), 716. https://doi.org/10.3390/su11030716
- Pittman, B., Eugenia, B., Sarin, S., O ‘Malley, S. S., Liss, T., & Gueorguieva, R. (2018). Models for Analyzing zero-inflated and over Dispersed count data: An Application to Cigarette and Marijuana use. Nicotine & Tobacco Research, 22(8), 1390–1398. https://doi.org/10.1093/ntr/nty072
- Rose, D. J., Poudel, R., Mallory, J., Haute, V., Yang, Q., Wang, L., Singh, M., & Liu, S. (2021). Pulse processing affects gas production by Gut Bacteria during in vitro Fecal Fermentation. Food Research International Journal, 14, 11045. https://doi.org/10.1016/j.foodres.2021.110453
- Saghaian, S., & Mohammadi, H. (2018). Factors affecting frequency of fast food consumption. Journal of Food Distribution Research, 49(1). https://doi.org/10.22004/ag.econ.274600
- Saito, A., Matsumoto, M., Hyakutake, A., Saito, M., & Okamoto, N. (2018). The presence of children in households was associated with dietary intake among Japanese married women: The Potato study. Journal of Nutritional Science, 7, e16. https://doi.org/10.1017/jns.2018.9
- Schaeffer, K. (2019). Among U.S. couples, women do more cooking and grocery shopping than men. https://www.pewresearch.org/fact-tank/2019/09/24/among-u-s-couples-women-do-more-cooking-and-grocery-shopping-than-men/
- Semba, R. (2016). The Rise and Fall of protein Malnutrition in Global health. Annals of Nutrition & Medicine, 69(2), 79–88. https://doi.org/10.1159/000449175
- Sheu, M., Hu, T., Theodore, E., Keeler, O., Michael, O., & Sung, H. (2004). The Effect of a Major Cigarette price change on Smoking Behavior in California: A zero‐inflated negative Binomial model. Health Economics, 13(8), 781–791. https://doi.org/10.1002/hec.849
- Syanda, J. S. (2019). The effects of physical properties of common bean (Phaseolus vulgaris L.) varieties on soaking and cooking time. [ Master of Science Thesis], submitted to South Eastern Kenya University. http://repository.seku.ac.ke/handle/123456789/4462
- Thompson, H. J., McGinley, J. N., Neil, E. S., & Brick, M. A. (2017). Beneficial effects of common bean on Adiposity and Lipid Metabolism. Nutrients, 9(9), 998. https://doi.org/10.3390/nu9090998
- Tiffany. (2020). Cooked beans or dry beans, which is cheaper? Don’t Waste the Crumbs. https://dontwastethecrumbs.com/cooked-beans-dry-beans-which-is-cheaper/.
- Tucker, L. A. (2020). Bean consumption Accounts for Differences in body fat and waist Circumference: A cross-Sectional study of 246 women. Journal of Nutrition and Metabolism, 20, 1–9. https://doi.org/10.1155/2020/9140907
- Tüzen, F. M., & S, E. (2017). A comparison of count data models with an application to daily cigarette consumption of young persons. Communications in Statistics- Theory and Methods, 47(23), 5825–5844. https://doi.org/10.1080/03610926.2017.1402050
- Ugen, M., Karanja, D., Birachi, E., Katabalwa, C., Ouma, J., & Mutuku, R. (2017). Precooked beans for food, nutrition, and income in Kenya and Uganda. https://www.idrc.ca/en/research-in-action/precooked-beans-food-nutrition-and-income-kenya-and-uganda
- Wahyudi, A., Kuwornu, J. K., Gunawan, E., Datta, A., & Nguyen, L. T. (2019). Factors influencing the frequency of consumers’ Purchases of Locally-produced rice in Indonesia: A Poisson regression analysis. Agriculture, 9(6), 117. https://doi.org/10.3390/agriculture9060117
- Winham, D., Florian, T., Thompson, S., & Brock, G. (2016). Low-income US women under-informed of the specific health benefits of consuming beans. PLOS ONE, 11(1), e0147592. https://doi.org/10.1371/journal.pone.0147592
- Winham, D. M., & Hutchins, A. M. (2007). Baked bean consumption reduces serum cholestrol in hypercholesterolemic adults. Nutrition Research, 27(7), 380–386. https://doi.org/10.1016/j.nutres.2007.04.017
- Winham, D., Tisue, M., Palmer, S., Cichy, K., & Shelley, M. (2019). Dry bean Preferences and Attitudes among Midwest Hispanic and non-Hispanic White women. Nutrients, 11(1), 178. https://doi.org/10.3390/nu11010178
- Winham, D., Webb, D., & Barr, A. (2008). Beans and Good health. Nutrition Today, 43(5), 201–209. https://doi.org/10.1097/01.NT.0000303354.21347.45
- World Health Organization (WHO). (2019). Healthy diet. Who.int. https://www.who.int/news-room/fact-sheets/detail/healthy-diet
- Worsley, A., Blaschea, R., Ball, K., & Crawford, D. (2004). The relationship between education and food consumption in the 1995 Australian National Nutrition Survey. Public Health Nutrition, 7(5), 649–663. https://doi.org/10.1079/phn2003577
- Wuenstel, J., Wądołowska, L., Słowińska, M., Niedźwiedzka, E., Kowalkowska, J., & Kurp, L. (2016). Intake of dietary fibre and Its Sources Related to Adolescents’ age and Gender, but not to their weight. Central European Journal of Public Health, 24(3), 211–216. https://doi.org/10.21101/cejph.a4331
Appendix 1
Table A1. Poisson model results on consumption frequency for PCB products
Appendix 2
Table A2. NBR results on consumption frequency for PCB products
Appendix 3
Table A3. Results from ZIP model on consumption frequency for PCB products