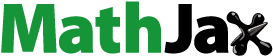
Abstract
Bundling the adoption of different adaptation strategies is useful in reducing multiple agricultural risks, building the resilience and supporting food security. This study analyses the effect of bundled adoption of conservation tillage, improved crop variety, and crop diversification on household food security in Ethiopia. In this study, a quantitative survey approach was used to gather household data using structured questionnaires. The data were gathered from randomly selected drought-prone districts of the Wolaita zone (southwest Ethiopia), which is often characterized by severe soil erosion, frequent rainfall variability, fragmented landholding, and poor plot tenure system, all of which threaten household food security. Household interview data were analyzed by the means of a multinomial endogenous switching model. Using the principal component analysis, the adoption of different adaptation strategies was bundled into three key components: conservation tillage, improved crop varieties, and crop diversification. The results showed that greater food security outcomes were achieved with the bundled adoption of conservation tillage, improved crop varieties, and crop-legume production compared to either of the single adoption. Adopters of the three bundles were 32.29% more food secure in terms of household food consumption score and 24.46% more food secure based on the scores of dietary diversity. The adoption of the three bundled climate change adaptation strategies was significantly influenced by the gender of the household head, farm size, and value of productive farm assets, implying that socioeconomic conditions are important factors affecting the adoption of bundled strategies and household food security. To promote bundling of adaptation strategies, frequent provision of agricultural extension services, active participation in farmer/female peers, and empowerment of women should be maintained through continuous education and gendered training.
1. Introduction
Most agricultural systems in sub-Saharan Africa (SSA) face challenges in improving food production as a result of ongoing climate change, resource depletion, and biodiversity loss. Although agricultural systems are key drivers for enhancing food availability and affordability, they are frequently affected by climate change through rising water scarcity, heat wave intensity, drought, and flood frequency, which cause drastic declines in productivity (Sova et al., Citation2018). Such adverse climate change impacts are expected to become more pronounced over the next century. As a result, the climate change-driven food insecurity has critically remained a challenge for smallholders, who particularly depend on rainfed systems for their livelihood. For instance, with its weak adaptive capacity due to poverty, internal conflict, and malnutrition (Wassmann et al., Citation2019), Ethiopia is particularly hit hard by climate change-driven food insecurity (Myers et al., Citation2017; van Wesenbeeck & Lammers, Citation2020).
As the climate change effects are striking in rainfed agriculture, it is not surprising that the adoption of bundled adaptation strategies is considered a leading candidate for ensuring food security. However, evidence to date appears rather mixed in approaching the intersection between food security and climate change adaptation depending on the productivity- and yield-oriented approach (Wekesa et al., Citation2018). Although such a lens can play a pivotal role in meeting food demand at scale, it does not take into account wider socio-economic contexts of adaptation, which determines not only productivity but also food production (Chichongue et al., Citation2020). In the context of food security, for example, lack of access to food is not the only driver influencing food insecurity. While ensuring food security and building the resilience of farmers, reducing the risks of production while increasing yield per capita, calorie intake, and dietary diversity is critical. Moreover, increased production, either by intensifying production or expansion of agricultural land, is generally assumed to exacerbate climate change risks. This can create a potentially false dichotomy where food security and adaptation are assumed as competing “‘system goals’” that must always involve trade-offs against each other (Myers et al., Citation2017; Tambo & Mockshell, Citation2018).
Although farmers adopt several interrelated strategies, such as conservation tillage including the use of different crop and grass mulches as well as the use of animal manure, along with improved crop varieties and types and crop diversification, previous studies do not attempt to combine these strategies at a specific plot. Conservation tillage is defined as use of cover crops (Mango et al., Citation2017), whereas conservation agriculture is the combination of minimum/zero tillage, crop cover, and crop diversification to enhance productivity and reduce climate change risks (Hadgu et al., Citation2019; Teklewold et al., Citation2017). However, there is a lively debate regarding which combinations of conservation tillage, improved crop varieties, and crop diversification are most appropriate to ensure food security (Prasad et al., Citation2016; Sova et al., Citation2018). While some consider low-cost input practices, such as conservation tillage as one of the most important adaptation strategies (Thornton et al., Citation2018), other studies propose models of input intensification including the use of crop varieties (Webb et al., Citation2021; Zougmor et al., Citation2021). Such strategies can reduce the application of chemical fertilizers, pesticides, and herbicides by promoting locally available productivity-enhancing practices. Advocates of low-cost input practices argue that the use of conservation tillage and crop diversification may help farmers optimize soil nutrients and improve organic matter in the long run. This can occur only when household food availability and affordability are ensured (Mango et al., Citation2017). Rather, in a context where farmers have insufficient food, the use of improved crop varieties is largely suggested by the advocates of high-cost agricultural technologies (Aryal et al., Citation2018).
Moreover, there is no “one-size-fits-all” climate change adaptation strategy that can be applied universally, nor are the available strategies always successful in buffering food security (Thornton et al., Citation2018). Given the diverse heterogeneity of SSA farming systems, livelihoods, agro-ecology, and institutional settings, there is no silver-bullet approach that can fit all systems (Mango et al., Citation2017). This shows that farming systems in Ethiopia are very dynamic, consisting of divergent agro-ecological zones ranging from semiarid lowlands to cool humid highlands, varying from extensive pastoral to intensive crop-livestock systems (Wainaina et al., Citation2016). As a result, specific type of climate change adaptation strategies may provide multiple outcomes in one setting, but it may also generate trade-offs in other settings, unless a combined set of adaptations is promoted. For example, the scale-up of conservation tillage practices in farming and agro-pastoral livelihood systems may create competition for crop residues unless improved fodders are introduced (Branca & Perelli, Citation2020; Webb et al., Citation2021).
To address the gaps in knowledge, this study assesses the food security impacts of bundled adaptation strategies in Ethiopia. Such assessments provide critical information for understanding how much agriculture should adapt to climate change and the cost of offsetting food insecurity (Wekesa et al., Citation2018). A rigorous analysis of adoption of climate change adaptation strategies and household food security interfaces is important for informing plans and programs targeting food security and social protection. Given the negative impacts of climate change on food security in Ethiopia, helping smallholder farmers better adapt to this variability is a central concern. Several studies have argued that promoting adaptation strategies is one of the effective mechanisms to address the challenges of food insecurity while reducing climate-related risks (Amadu et al., Citation2020; Hussain et al., Citation2023; Mekonnen et al., Citation2021). Such strategies include the use of mixed cropping of legumes with cereal crops, improved crop varieties, crop diversification, irrigation use, soil and water conservation practices, which maximize productivity, income, and food availability while reducing climate change risks. Depending on the capacity of each adaptation strategies to ameliorate multiple risks, most farmers and agencies can more readily recognize climate change risks and are thus more likely to engage in building knowledge and institutional resilience (Hussain et al., Citation2023).
To address these issues, this study applied a multi-method research design that combines qualitative and quantitative techniques to increase the scientific rigor of each method (Mwongera et al., Citation2017). Data on socioeconomic factors, institutional systems, historical climate, conservation tillage, crop diversification, use of improved crop varieties, food consumption, and dietary diversity were collected from Ethiopia through interviews and discussion. The data were analyzed using principal component analysis, which groups the heterogeneous principal CSI clusters into a common package. To account for endogeneity arising from farmers’ self-selection biases, this study used a recently developed Multinomial Endogenous Switching Regression (Issahaku & Abdulai, Citation2020).
This study contributes to the growing literature on adaptation and food security in the context of ongoing climate change. Previous adaptation studies have evaluated the impacts of adaptation adoption on food security, usually from the consumption expenditure perspective (Aryal et al., Citation2018; Thornton et al., Citation2018; Wassie & Pauline, Citation2018). In doing so, most adaptation-driven food security studies collect data on the amount of food physically available and the money needed to purchase the food at the household level (Mango et al., Citation2017). However, such a perspective might underestimate or overestimate the true adoption impact of food security by ignoring the amount and diversity of food consumed at the household level (Wekesa et al., Citation2018).
Moreover, this study contributes to the understanding of the interrelationship among the adoption of multiple adaptation strategies to simultaneously address household food security and climate change risks. Previous studies have independently investigated the impact of a specific adaptation strategy on food security outcomes independently (Teklewold et al., Citation2017; Thornton et al., Citation2018; Wekesa et al., Citation2018). Such studies have not considered the interdependent nature of adaptation strategies to deal with multiple socio-economic and ecological stresses, overlooking important synergies. This is due to the fact that the effect of climate change does not only manifest itself through climatic factors like temperature and rainfall variability but also economic and social impacts, so does the use of the specific strategies to deal with climate change risks. According to Mango et al. (Citation2017), the use of one adaptation strategy can be conditional on its rate of adoption and other socio-economic and biophysical factors at the local scale. Consequently, a focus on specific adaptation strategies can either underestimate or overestimate the true adaptation impacts of food security (Hadgu et al., Citation2019).
An additional contribution of this study is the investigation of the specific factors that can largely influence the adaptation-driven food security status of smallholder farmers subjected to multiple climatic and socioeconomic risks. Being generally qualitative in nature, the majority of the studies conducted so far (Branca & Perelli, Citation2020; Zougmor et al., Citation2021) have examined the limits and barriers of adopting adaptation and mitigation strategies without placing emphasis on their impacts. Given the fact that not all adaptation strategies are equally capable of driving food security due to variations in farm and livelihood systems (Falco, Citation2014), analyzing location-specific adoption is an essential precursor to understanding what locally enabling conditions are available to promote or hinder the adoption of adaptation for food security. Bridging this research gap could provide useful information for developing successful food security policies within the context of climate change that build household resilience and agricultural sustainability in SSA (Chichongue et al., Citation2020).
Consequently, this study analyzes the adoption of multiple adaptation strategies within the context of food security outcomes, including household food consumption and household dietary diversity. It then examines the degree of bundling between different sets of adaptation strategies driving food security. Next, several social and institutional factors that influence the adoption of bundled sets of adaptation strategies while driving household food security were analyzed. Finally, this study evaluated the effect of adopting adaptation strategies on household food security. The results of this study are directly relevant to research and policy options addressing food security challenges by promoting bundled adoption of adaptation strategies to support future food security plans. The findings of the study are relevant to inform policymakers and scientific community by generating an integrated knowledge and evidence supporting food security.
2. Methodology
2.1. The study site
The study was conducted in the Wolaita zone, which is located in the southern parts of Ethiopia. This zone stretches from 6° N to 7° northern latitude and 37° E to 38° eastern longitude, with an elevation between 500 and 3200 m above sea level. For this zone, the mean annual temperatures show a minimum value of 15°C and maximum value of 32°C, whereas the annual precipitation is 900 mm National Meteorological Agency (NMA, Citation2015). Bimodal seasonal rainfall patterns are prominent while extending from months of March to September. The period between March and May is the short rainy season, whereas the period between June and September is the long rainy season. The land area covers 4100 km2, of which 65% is cultivated or fertile cultivable (Bagnara, Citation2017). In the Wolaita zone, particularly in dryland areas, which are mainly exposed to frequent droughts and incidents of food insecurity, climate change has unprecedented negative effects on livelihood systems.
2.2. Sampling strategies and data
Using a mixed-method research design, this study employed household surveys and focus-group discussions. For the household survey, sample selection was performed using a cluster sampling technique that divided the study units into smaller samples in four stages. First, two districts, Abela Abaya and Kindo Koysha, which are highly prone to drought, soil moisture stress, and erosion and are subjected to rapid soil degradation and frequent food crises, were selected purposively from the Wolaita zone through consultations with extension workers. Both districts are located within the Great Rift Valley, which is home to diverse flora and fona, but prone to frequent droughts and dry spells, as well as facing serious problems of soil degradation and deforestation that threaten food security. To deal with extreme events, farmers are expected to adopt several types of soil water conservation practices, irrigation practices, conservation tillage, crop diversification, and drought-resistant maize varieties. Second, while obtaining the lists of rural kebeles, which is the lowest administrative unit in Ethiopia, from the local administrator, the sampling frame was developed. Using a simple random sampling technique, two villages were selected from each cluster. Third, the final sample size was determined using the formula suggested by Kothari (Citation2004) in EquationEquation (1)(1)
(1) :
where n represents the required size to predict the population N; z indicates the confidence level based on the 95% (z = 1.96); p denotes the distribution of population attributes (p = 0.5); q is 1– p; and e represents the desired level of precision (e = 5%). A p value of 50% was assumed to increase the level of precision for the sample size and reduce the variability of population attributes. This was based on the logic that the more heterogeneous the population is, the larger the sample size required in order to obtaining a given level of precision.
In the final stage, to select samples proportionally from the study villages, a probability proportional to the size sampling technique was applied. Accordingly, a total of 340 heads of households were selected for interview. The data covered detailed information on demographic and socioeconomic characteristics, and institutional systems. Data on landholdings, climate risks, agricultural inputs, crop production, type of adaptation used, and dietary diversity were collected. The data were entered into a statistical package for social science (SPSS) v. 20 for coding and decoding and Stata v. 11 for final regression analysis.
2.3. Analytical framework
2.3.1. Principal component analysis
The last cropping season was used as a reference for selection of adaptation strategies. First, different categories of conservation tillage, improved crop variety, and crop diversification practices were identified. This was followed by grouping of heterogeneous principal clusters using principal component analysis. Orthogonal rotation (varimax method) was used to rotate the components (Hyland et al., Citation2015). For the sake of easy interpretation of the results, those highly and similarly correlated practices were placed in each component. The result of the rotation was three principal components from a possible 10 extracted with eigenvalues >1 following the criterion (Deressa, Citation2010). Such analysis is useful in decreasing the issues of dimensionality without leaving the context of the information. This method is more convincing than the use of conventional method, which is difficult to draw arguments about a group. The analysis was performed with careful iterations and rotation using the varimax method (Moroda, Citation2018), as shown below:
Where = principal components that are uncorrelated,
= coefficient of the correlation, and
= factors influencing the choice of a particular strategy bundle. The adaptation strategies identified and grouped through a principal component analysis are presented in Table .
Table 1. Adoption of conservation tillage, improved crop varieties, and crop diversification strategies
2.3.2. Stage 1: selection model (Multinomial)
At this stage, a multinomial logit (Greene, Citation2012) was used to model the determinants of the choice of bundles of conservation tillage (T), improved crop varieties (I), and crop diversification (D). Smallholder farmers are expected to maximize their food security outcomes, by comparing the economic benefits obtained from four (L) alternative strategies. The requirement for farmer i to choose any strategy j over other alternatives L is that
L ≠ j, that is, j provides higher expected food security than any other strategy.
is a latent variable representing the expected food security status and is influenced by observed independent factors and unobserved characteristics (Greene, Citation2012), which can be expressed using Equation (2):
where captures the observed explanatory variables, and the error term
captures unobserved terms. The covariate vector
is assumed to be uncorrelated with the unobserved feature
, that is,
= 0, whereby the error terms
are assumed to be evenly distributed and independent, that is, based on the hypothesis of the independent irrelevant alternatives (Makate et al., Citation2019). Selection model (2) can be translated into a multinomial logit model in such a way that the likelihood of adopting a specific strategy
is
2.3.3. Stage 2: multinomial endogenous switching regression
2.3.3.1. Model
To predict the effect of T, I, and D bundles on food security outcomes, an endogenous switching regression (ESR) was used by including a selection bias correction model. Farm households face five categories, with category j = 1 being the reference (non-responsive). For the food security, equation of each possible category is defined as
where represents food security status,
indicates a set of different explanatory variables, and the ith farmer in the first j and the error terms
are distributed with E
= 0 and var
.
is observed if and only if the selected strategy j is used, which occurs when
; if the error terms in equation (3) and equation (4) are not independent, the OLS estimates for equation (4) are biased. A consistent estimation of
requires the inclusion of the selection correction terms for the alternative choices in equation (3). MESR assumes the following linearity:
. By construction, the correlation between the error terms in Equation (3) and Equation (4) was zero.
Based on this assumption, equation (3) can be expressed as follows:
is the covariance between
and μ, and j is the inverse Mills ratio computed from the estimated probabilities in Equation (5), as follows:
where shows the coefficients of the correlation ɛ and μ, and
are error factors with an expected zero mean value and unitary standard deviation. In the context of the multinomial choice, there were
selection correction terms, one for each alternative strategy. In order to account for heteroscedasticity arising from the generated repressors given by
, the standard errors in equation (5) were bootstrapped.
2.4. Estimation of average treatment effects
To analyse the ATT while comparing the expected outcome values of adoption and non-adoption for a particular strategy, a counterfactual analysis was performed (Mango et al., Citation2017). The ATT in the actual and counterfactual scenarios was calculated based on Equation (7) and Equation (8):
2.4.1. Food security status with adoption
2.4.2. Food security status without adoption (counterfactual)
The Average Effect of the Treatment on the Treated (ATT) can be indicated as the difference between (7a) and (8a), which is given by equation (9):
where ATT indicates the expected difference in adopters’ mean food security status if adopter characteristics had the same outcome as non-adopters, while is the selection term that captures all potential effects of differences in unobserved variables.
2.4.3. Summary of model variables
An innovation adoption and impact assessment approach has been applied to simultaneously estimate the adoption of strategy bundles and their food security impacts (Issahaku & Abdulai, Citation2020). The innovation adoption approach allows for a full investigation of the relationship between farmers’ “adoption” and “no adoption” of combinations of conservation tillage, crop diversification, and use of improved crop varieties. This approach provides an understanding of the critical pathways and impacts driven by the adoption of combinations of CSIs (Thornton & Herrero, Citation2015). Such relationships can be captured by estimating the effects of several explanatory variables on the combinations of strategy bundles and food security effects. As dependent variable, the adoption of improved crop varieties included the use of drought-tolerant maize varieties, high yielding pigeon peas, and organic fertilizers. Potential practices that combined crop residue, grasses, and animal manure as soil mulches were categorized under conservation tillage. Such strategies can be combined with the diversification of crops with legumes and other crop varieties that provide higher yields while optimizing productivity. Table shows the variables used to estimate the effect of strategy bundle adoption on household food security.
Table 2. Model variables
2.5. Measuring food security outcomes
For measuring food security, the Household Food Consumption Score (HFCS) and household dietary diversity score were used as proxies. The HFCS of a household was calculated by multiplying the frequency of foods consumed within 7 days with the weighting of each food group. The HDDS considers the food items consumed within the last 24 h. These two indicators measure food diversity, which is strongly correlated with dietary quality and adequacy (Wekesa et al., Citation2018).
3. Results and discussion
3.1. Principal component analysis (PCA)
The results of the principal components (PCs) and coefficients of linear combinations, called loadings, are presented in ). Accordingly, three principal components explained 76.821% of the total variability in the dataset (Comp3), which is a good fit, indicating that the PCA results highly explained the data. The first component explained 52.092% of the variance (Comp1) and was correlated with the use of crop (maize, barley, wheat, and sorghum) residue as soil cover, reduced tillage with soil cover, use of organic manure for soil, and use of grasses as mulches, all of which had positive effects (factor loadings). Thus, these sub-components were combined into the principal components of conservation tillage.
The second component explains 58.841% of the variance in the data. This variation is largely due to the bundled use of drought tolerant crop varieties and the use of extra-early maturing crop varieties, which are categorized under the improved crop variety principal component (Comp2). Each component has both positive and negative effects, implying variation in the direction and magnitude of the effects across strategies. The adoption of legumes in crop rotation, mixed adoption of crops with non-legumes, diversification of crop species grown in sequences, and crop varietal diversity within crop species are categorized under the principal component of crop diversification. Such sub-components largely explain the principal component but with high negative effects at varying degrees. In Table , communality shows more than 60% of the variability in the data, which is valid for fulfilling the minimum criteria.
Table 3. Loadings of the three components
3.2. Descriptive statistics
For the 340 respondents surveyed, the average age of the household head was 41.43 with a standard deviation of 13.67. Among the sample respondents, 75% were male and the remaining 25% were female. The average education level of the respondents in terms of school year was 5.34 with a standard deviation of 3.41. The majority of farmers participate in non-farm activities, such as petty trading, animal bartering, seasonal migration, daily labor work, cereal crop bartering, carpentry, and poetry. The total land size in hectare is 0.34 with a standard deviation of 0.69. About 69% of the respondents were contacted by extension agents, who provided advice on crop and animal production, as well as natural resource management.
Crop diversification was the most preferred component, with 46.32% of farmers using at least one unit (Table ). This component includes the use of legumes in crop rotation, mixed use of crops with non-legumes, diversification of crop species in sequences, and crop varietal diversity within crop species and rotating crop species. The second most used component was improved crop variety to enhance productivity and reduce risks. This component was used by 39.45% of households across the study districts. The use of improved crop varieties entailed the adoption of drought-tolerant crop varieties and extra-early maturing crop varieties.
Table 4. List of conservation tillage, improved crop varieties, and crop diversification strategies
Conservation tillage measures were used by 29.84% of farming households. The practices in this component include the adoption of crop residue as soil cover to enhance nutrient recycling, use of grasses as mulches to protect soil erosion along with the reduced tillage with soil cover and use of organic manure. Such conservation tillage practices do not only serve as a source of nutrient for succeeding crops but also enhance soil moisture and air quality.
3.3. Econometric results
Conservation tillage, improved crop varieties, and crop diversification can be adopted by smallholder farmers in various combinations. This combination results in a varying set of adoption bundles that can enhance agricultural productivity while reducing climate change risk. Further, such adoption bundles, in turn, have significant implications for achieving household food security outcomes, including improved consumption and dietary diversity. Given the set of available adoption bundles, understanding the factors influencing the adoption or dis-adoption of a specific strategy bundle is critical for the adaptation planning.
Table depicts a combination of various packages, whereby eight out of nine possible combinations were implemented by farming households. Few farmers (4.4%) were non-adopters of any CSI package. About 19.7% of farmers used package T1I0D1. This package comprised conservation tillage and crop diversification only. Another 4.4% used package T0I1D0 that had only used improved crop varieties. Further, 9.4% of farmers used package T1I1D0 that contained conservation tillage and improved crop varieties. Another 8.3% of farmers used package T0I1D1 that contained the use of improved crop varieties and crop diversification strategies. Approximately 1.7% of farmers used packages of T1I1D1 with all the four groups of CSI.
Table 5. Specification of conservation tillage, improved crop varieties, and crop diversification bundles
The majority of households (24.4%) adopt T0I1D1, which can raise the adoption of improved crop varieties and crop diversification. This shows that achieving household food production depends on the adoption of a combination of packages, despite the soil degradation challenges. This finding is in line with the results of (Wekesa et al., Citation2018), who indicated that farmers in the area implement several responsive strategies for survival amidst climate change risks. A close observation to Table indicates that all the adopters (96.4% of all farmers) implement adaptation packages that included at least crop diversification strategies. This result reflects the need for farmers to meet their basic crop production requirements for food generation.
3.4. Determinants of choice of bundled adaptation strategies
In this subsection, the main socio-economic and biophysical factors influencing the adoption of adaptation strategy packages are first elucidated by means of a multinomial selection model. This was followed by modelling the effect of each strategy bundle on household food security. This impact was modelled using a multinomial endogenous switching regression (MESR) following a two-stage regression analysis. The first stage of MESR is the multinomial logit model, which determines the factors that influence the choice of strategy bundles. This is an important stage, as it guides the necessary interventions to improve the adoption of strategy bundles. In the second stage, the impact of adopting strategy bundles on household food security is determined. Table reports the marginal effects from the MNL model that measured the expected change in the probability of a particular choice being made with respect to a unit change in an independent variable.
Table 6. Marginal effects estimates for the determinants CSA packages by MNL
The non-adoption of adaptation strategy bundles (T0I0D0) was the reference category compared with the other four bundles. Results show that four parameter estimates: one for each mutually exclusive bundle was adopted by farmers. The Wald test, in which all regression coefficients are jointly equal to zero, was rejected [χ2 (339) = 375.76; p = .000]. Thus, the results show that the estimated coefficients differ substantially across the alternative bundles.
The household age has a negative effect on the choice of T1I0D0 and a positive effect on the adoption of T1I1D1 at 10% and 1% significance levels, respectively. An increase in the age by 1 year is likely to reduce the likelihood of adopting T1I0D0 bundles by 0.19%, while increasing the likelihood of adopting T1I1D1 by 0.22%. This indicates that, as age increases, farmers shift from a small number of bundles to larger ones. Older farmers may be more experienced in production technologies and may have accumulated more physical and social capital to afford larger and better bundles. In contrast, in Ethiopia, the study of Tesfaye et al. (Citation2020) revealed that the age of the household head had a negative effect on the bundled adoption of conservation tillage and crop diversification. This shows that a rise in age of the household is likely to reduce the probability of the joint adoption of conservation tillage and crop diversification. In addition, older farmers may not be aware of recent innovations compared with younger farmers in a specific farm and livelihood context (Wekesa et al., Citation2018).
With regard to gender, male-headed households were 2.5% more likely to use bundles of T1I1D1 combined with conservation tillage, improved crop varieties, and crop diversification only at the 5% significance level. This relationship is based on the relative non-use of any bundle compared to female-headed households. This may explain the negative relationship between strategy bundle adoptions in this study. Female-headed households generally face frequent limitations in terms of having resources, opportunities, and taking household decisions effectively, so that they are less likely to adopt strategy bundles that enhance food security. This is due to the fact that women have less access than men to agricultural inputs, formal credit services, and extension services, all of which support adoption of strategy bundles. This result is consistent with the findings of Wekesa et al. (Citation2018), who showed that the likelihood of farmers adopting improved and new agricultural practices is significantly affected by the gender of the household head in Kenya.
Farmers with more years of education are less likely to adopt T0I1D0 strategy bundles, which include drought-resistant crop varieties and early maturing crop varieties. An additional year of education increased the probability of adopting the strategy bundle by 7.3% at the 5% significance level. This is partly because educated farmers have a higher capacity to access and analyse information on new technologies and innovations, which offers higher yields while promoting investment in strategy bundles. These categories of farmers improve productivity and minimize the risk of adopting strategy bundles by managing and diversifying knowledge sources. In line with this finding, Alemu and Mengistu (Citation2019) indicated that farmers with higher levels of education tend to build their knowledge and improve their skills and ability to assess and fight risks.
Farmers with a greater value of productive assets were more likely to use larger bundles of T1I1D1 than those who did not adopt any strategy bundles. Access to productive farm assets significantly and negatively influenced the adoption of T0I1D0 bundles. Specifically, the likelihood of adopting this strategy bundle has increased by 0.14% for farmers with larger sets of productive assets. Such farmers can adopt several strategy bundles, including drought-tolerant crop varieties and early maturing varieties. This finding is consistent with that of Mengistu and Assefa (Citation2020), who noted that limited access to productive assets may hinder the adoption of new conservation practices that require huge investments.
Farmers with a larger farm size per hectare are highly likely to adopt T1I0D0 and T1I1D1 strategy bundles. This implies that an increase in the size of land by 1 acre (0.40 ha) increased the probability of adopting T1I0D0 by 7.3% while increasing the likelihood of using T1I1D1 by 8.1%. This finding implies that having a larger farm size is likely to increase the opportunity to diversify cropping and, as a result, raises the likelihood of adopting strategy bundles against non-adoption of strategy bundles. This finding agrees with the results of Wassie and Pauline (Citation2018), who stated that large farms maintain economies of scale and thereby provide greater opportunities to diversify production.
Farmers who perceive a greater soil fertility status are highly likely to adopt T1I0D1 and T1I1D1 strategy bundles. The likelihood of adopting T1I0D1 and T1I1D1 bundles decreased by 3.1% and 11.3%, respectively, compared to the likelihood of not adopting any strategy bundles. This implies that farmers who perceived the fertility status of their farm soil were less likely to adopt strategy bundles than farmers who did not perceive the fertility of the soil. This means that soils with higher nutrients, containing sufficient organic matter and bearing greater soil moisture, do not require further investment in strategy bundles. This result is consistent with the findings of Tekeste (Citation2021) in Ethiopia, who indicated that farmers with higher fertility plots have less propensity to use conservation practices that do not add further fertility value to the farm.
Farmers who experience frequent erratic seasonal rainfall during the cropping period are more likely to adopt more strategy bundles to enhance productivity and reduce climate risks. Past experience with variability in seasonal rainfall positively affects the bundled adoption of T1I0D1 and T1I1D1. The probability of choosing these bundles increased by approximately 0.71% and 22.3% for farmers who had experienced frequent rainfall variability in terms of late start and early end in the recent past, respectively. Such farmers are likely to implement a strategy bundle that improves soil moisture, copes with heat stress and dry spells, and adapts to climate change through sustainable diversification and intensification. This finding agrees with the results of Araya et al. (Citation2011), who indicated that the erratic nature of seasonal rainfall, which poses a threat to stable cropping, is the main driver for farmers to adopt a combination of conservation practices.
Farmers with membership status in local farmer/women groups are more likely to adopt sustainable conservation practices and improve crop varieties. Membership in farmer groups positively and significantly influences the adoption of T1I1D1 and T1I1D0. Compared with the probability of not adopting any strategy bundles, membership in a farmer group increased the propensity to adopt these strategy bundles by 12.3% and 9.05%, respectively. Membership in farmer and/or women groups provides an opportunity for households to facilitate the smooth flow of information and experience sharing on new innovations, insurance, and extension services, thereby increasing the probability of adopting a broad set of strategy bundles. Additionally, through group networks, members can potentially exchange new ideas and innovations, handle farm demonstrations, and connect to the dissemination of important research findings. This result is in line with the findings of Wekesa et al. (Citation2018), who indicate that the exchange of new information and innovations through involvement in peers significantly increases the probability of technology adoption. This is due to the fact that practical demonstration of experimental results through peers can provide greater trust among members as they share several common values, beliefs, and cultures that bring them into a sense of belongingness.
Farmers with frequent contact with extension agents and obtaining advice on sustainable crop production and conservation practices are more likely to adopt T1I1D0 and less likely to adopt the T1I0D0 strategy bundle. A one unit increase in the frequency of extension contact with farmers agents augments the probability of adopting T1I1D0 by 32.0%, but simultaneously reduces the probability of adopting T1I0D1 by 1.3%. The provision of agricultural extension information and technology can play a significant role in adopting larger strategy bundles. However, a reduction in the probability of adopting T1I0D1 suggests that the goal of promoting strategy bundles using extension agents had divergent effects. For example, farmers who adopt T1I0D1 are cynical about the authenticity of the extension information and its effect on improving productivity and reducing climate risk. Thus, they are likely to opt to avoid adopting strategy bundles. This result is consistent with the findings of Chichongue et al. (Citation2020), who indicated that farmers who frequently receive advice from extension agents are highly likely to adopt sustainable agricultural conservation practices, improved crop varieties, and climate risk reduction strategies.
3.5. Average adoption treatment effects for the strategy bundles
In this subsection, treatment effects are determined to determine the effect of strategy bundle adoption on household food security outcomes. These treatment effects vary widely across adoption groups, depending on the characteristics of farmers and farming conditions. This is the difference between HFCS and HDDS for the adoption and non-adoption of a specific set of strategy bundles. The ordinary least squares regression of the HFCS and HDDS indicates the significant and differing effects of each strategy bundle on food security status. This occurs by including the (selection) bias correction terms obtained from the results of the multinomial selection model.
The food categories for HFCS and HDDS indicate that there is variation in food security status across adopters and non-adopters of the strategy bundles. These categories were used to measure and compare the status of household food security within the context of the adoption and non-adoption of strategy bundles in both actual and counterfactual conditions. HFCS was used in combination with HDDS, as the latter only captures meals taken within 24 hours, which may not include occasional meals taken on particular days, such as market days within a week. In addition, the HDDS was used to analyze, compare, and validate the sensitivity of the HFCS. The two scores do not strongly correlate (0.45), suggesting the use of both indices to measure the level of household food security across adoption groups. This result is in agreement with the findings of Mango et al. (Citation2017), who applied both HFCS and HDDS to measure the status of food security in the context of adopting sustainable agricultural practices.
The results show varying levels of average adoption effects in terms of HFCS and HDDS under actual and counterfactual conditions (Table ). Farmers who adopted T1I0D0 gained higher HFCS in counterfactual conditions than in treatment conditions. However, farmers who adopted the T1I1D0 strategy bundles gained higher HFCS and HDDS in the treated condition than in the untreated condition. This is due to the fact that the adoption of drought tolerant crop varieties along with conservation tillage is critical to improve productivity, enhance yields, and ensure food security by maintaining soil fertility and soil moisture, especially during the dry season. Farmers adopting T1I1D1 gained both a higher HFCS and greater HDDS under treatment conditions compared to the counterfactual scenario. This result is consistent with the findings of (Mango et al., Citation2017) who indicated that conservation agriculture potentially improve farmers’ yields by improving the efficiency of soil nutrient use.
Table 7. Results of multinomial endogenous switching regression model
Except for adopters T1IOD1, T1I1D0, and T1I1D1, all other farmers adopting other strategy bundles would be better off in the counterfactual scenarios, suggesting the availability of other better strategy bundles. The adoption of T1I0DO, T0I0D1, and T0I1D0 strategy bundles had a limited impact on the HFCS and HDDS. Despite their limitations, farmers who adopted T1IODO and T0I0D1 gained higher HFCS and HDDS scores than those who did not adopt any strategy bundles. This implies that farmers need to adopt specific strategy bundles, depending on the local farm and household context, to support food security and deal with climate change. This result is in agreement with the findings of Wekesa et al. (Citation2018), who revealed that the adoption of any specific climate-smart strategy provides a higher food security status in Kenya
compared to non-adoption. For larger bundles of T1I1D1, T1I0D1, and T1I1DO, there was a higher gain in food security outcomes, that is, both the HFCS and HDDS, compared to farmers who adopted only one strategy. To put into a context, a complete adoption of bundled strategies such as conservation tillage, the adoption of drought tolerant crop varieties and crop diversification had the greatest overall impact of 32.3 and 24.3 scores on HFCS and HDDS, respectively. This implies that farmers who adopted these bundles were 32.3% and 24.3% more food secure than their counterparts who chose not to adopt any of the strategy bundles in the counterfactual scenario. This implies that farmers may be more food-secure if they adopt different strategy bundles in combination rather than separately to increase productivity, reduce climate change risks, and ensure household food security. Such bundles are quite comprehensive, as they cover a wider range of gains, improve soil conditions, increase yields, and mitigate soil degradation.
4. Conclusions and policy implications
This study analyzed the rate of adoption of conservation tillage, improved crop varieties, and crop diversification as adaptation strategies to support food security and reduce climate change risks. The findings show that farmers who adopt conservation tillage improve crop varieties and diversify crops are below average. Conservation tillage was the least preferred and implemented strategy, followed by the use of improved crop varieties. This can perhaps be attributed to the fact that the majority of farmers were resource-constrained and had low productive assets. This result is consistent across the household characteristics of the farmers and farm features of the plot. To this end, it can be concluded that adoption of strategy bundles depends on several limiting factors beyond the adoption of strategy bundles. This suggests the need for proper farmer empowerment to move progressively towards more capital-intensive and sustainable agricultural practices.
This study also assessed the different factors influencing the choice of strategy bundles in the context of improving productivity and reducing climate change risk. Accordingly, the findings show that young and male-headed farmers who attended greater years of education are more likely to adopt conservation tillage and crop diversification strategy bundles. Contrary to the expectations, farmers with greater farm size per hectare and good soil fertility status are less likely to use a combination of conservation tillage and improved crop varieties. However, farmers who have membership status and frequent contact with extension agents to get advice on sustainable agricultural practices are highly likely to adopt conservation tillage, improved crop varieties, and crop diversification. As a result, it can be concluded that the adoption of strategy bundles to improve productivity and reduce climate change risks is largely driven by several social and economic factors, among which individual characteristics and social relationships are important.
This study also evaluated the effect of several strategy bundles on food security outcomes, such as HFCS and HDDS, as proxy measures. The results show that farmers who adopt conservation tillage and improved crop varieties have gained greater benefits in terms of HFCS and HDDS in the treatment condition against the counterfactual scenario. A larger package comprising conservation tillage improved crop varieties, and crop diversification had the greatest impact on household food security. Although the average size of the farm for each household seems very small, which would not allow different combinations of strategy bundles to be applied, the adoption of greater strategy bundles addresses a wider spectrum of both field and soil conditions while also mitigating soil degradation for production stability. Thus, this study concluded that, to gain more food security and environmental conservation benefits, the adoption of a specific strategy bundle should be followed by the simultaneous or sequential adoption of all other strategy bundles, although such adoption depends on different household and farm characteristics. The study suggests that an increase in frequency of extension contacts with farmers provides an oppurtunity to invest them in bundled adaptation practices inorder to reduce risks associated with the climate chnage while at the same time imroving household food security. Second, women’s empowerment and active participation in peers should also be promoted through civic education, continuous training, and engagement in equal decision-making and access to resources. Future research should address more detailed gendered impacts of adaptation on household food security by including broader measures such as food affordability and stability at household level.
Authors’ contribution
The entire author contributed to this manuscript
Availability of data and materials
Data and materials can be accessed on special request with permission from the Department of Rural Development and Agricultural Extension, Wolaita Sodo University, Ethiopia, and the author.
Competing interests
There is no competing interest on the manuscript.
Ethical approval and consent to participate
Ethical approval was obtained from the Research and Ethical Approval Committee of Wolaita Sodo University.
Acknowledgments
Special thanks goes to three anonymous reviewers.
Additional information
Funding
Notes on contributors
Sisay Belay Bedeke
Dr. Sisay Belay Bedeke is working as an Assistant Professor in The Department of Rural Developmen, Wolaita Sodo University, Ethiopia. Bedeke’s Research areas include climate change vulnerability, community reslaince, economics of climate smart innovation, food security, sustainble agriculture, aagricultural extension. He published many research articles in various international peer reviewed journals. Bedeke undetook several consultancy work for different GOs and NGOs in Ethiopia and beyound. He also worked in the capacity of Research and Documentation Officer in Wolaita Sodo University.
References
- Alemu, T., & Mengistu, A. (2019). Impacts of climate change on food security in Ethiopia : Adaptation and mitigation options : A Review : Soil-water-plant nexus impacts of climate change on food security in Ethiopia : Adaptation and mitigation options : A Review. June. https://doi.org/10.1007/978-3-319-75004-0
- Amadu, F. O., McNamara, P. E., & Miller, D. C. (2020). Understanding the adoption of climate-smart agriculture: A farm-level typology with empirical evidence from southern Malawi. World Development, 126, 104692. https://doi.org/10.1016/j.worlddev.2019.104692
- Araya, T., Cornelis, W. M., Nyssen, J., Govaerts, B., Bauer, H., Gebreegziabher, T., Oicha, T., Raes, D., Sayre, K. D., Haile, M., & Deckers, J. (2011). Effects of conservation agriculture on runoff, soil loss and crop yield under rainfed conditions in Tigray, Northern Ethiopia. Soil Use and Management, 27(3), 404–19. https://doi.org/10.1111/j.1475-2743.2011.00347.x
- Aryal, J. P., Jat, M. L., Sapkota, T. B., Khatri-Chhetri, A., Kassie, M., Rahut, D. B., & Maharjan, S. (2018). Adoption of multiple climate-smart agricultural practices in the Gangetic plains of Bihar, India. International Journal of Climate Change Strategies and Management, 10(3), 407–427. https://doi.org/10.1108/IJCCSM-02-2017-0025
- Bagnara, G. L. (2017). Agricultural production and marketing of Wolaita rural area (issue July). https://doi.org/10.13140/RG.2.2.34313.95846
- Branca, G., & Perelli, C. (2020). ‘Clearing the air’: Common drivers of climate-smart smallholder food production in Eastern and southern Africa. Journal of Cleaner Production, 270, 121900. https://doi.org/10.1016/j.jclepro.2020.121900
- Chichongue, O., Pelser, A., Tol, J. V., Du Preez, C., & Ceronio, G. (2020). Factors influencing the adoption of conservation agriculture practices among smallholder farmers in Mozambique. International Journal of Agricultural Extension, 7(3), 277–291. https://doi.org/10.33687/ijae.007.03.3049
- Deressa, T. T. (2010). Assessment of the vulnerability of Ethiopian agriculture to climate change and farmers’ adaptation strategies (issue July). https://doi.org/10.13140/RG.2.1.1090.2889
- Falco, S. (2014). Adaptation to climate change in Sub-Saharan agriculture: Assessing the evidence and rethinking the drivers. European Review of Agricultural Economics, 41(3), 405–430. https://doi.org/10.1093/erae/jbu014
- Greene, W. W. H. (2012). Econometric analysis. Prentice Hall, 97. https://doi.org/10.1198/jasa.2002.s458
- Hadgu, K. M., Bishaw, B., Iiyama, M., & Birhane, E. (2019). Climate-smart agriculture enhancing resilient agricultural (issue July). World Agroforestry Center.
- Hussain, S., Mubeen, M., Nasim, W., Fahad, S., Ali, M., Ehsan, M. A., & Raza, A. (2023). Investigation of irrigation water requirement and evapotranspiration for water resource management in southern Punjab, Pakistan. Sustainability, 15(3), 1768. https://doi.org/10.3390/su15031768
- Hyland, J. J., Jones, D. L., Parkhill, K. A., Barnes, A. P., & Williams, A. P. (2015). Farmers’ perceptions of climate change: Identifying types. Agriculture and Human Values, 33(2), 323–339. https://doi.org/10.1007/s10460-015-9608-9
- Issahaku, G., & Abdulai, A. (2020). Adoption of climate-smart practices and its impact on farm performance and risk exposure among smallholder farmers in Ghana. Australian Journal of Agricultural and Resource Economics, 64(2), 396–420. https://doi.org/10.1111/1467-8489.12357
- Kothari, C. R. (2004). Methods and Techniques (2nd ed.). New Age International Publishers.
- Makate, C., Makate, M., Mango, N., & Siziba, S. (2019). Increasing resilience of smallholder farmers to climate change through multiple adoption of proven climate-smart agriculture innovations. Lessons from southern Africa. Journal of Environmental Management, 231(August 2018), 858–868. https://doi.org/10.1016/j.jenvman.2018.10.069
- Mango, N., Siziba, S., & Makate, C. (2017). The impact of adoption of conservation agriculture on smallholder farmers’ food security in semi-arid zones of southern Africa. Agriculture & Food Security, 6(1), 4–11. https://doi.org/10.1186/s40066-017-0109-5
- Mekonnen, A., Tessema, A., Ganewo, Z., & Haile, A. (2021). Climate change impacts on household food security and adaptation strategies in southern Ethiopia. Food and Energy Security, 10(1), 1–14. https://doi.org/10.1002/fes3.266
- Mengistu, F., & Assefa, E. Enhancing livelihood assets of households through watershed management intervention program: Case of upper Gibe basin, Southwest Ethiopia. (2020). Environment Development and Sustainability, 22(8), 7515–7546. Springer Netherlands. https://doi.org/10.1007/s10668-019-00534-x
- Moroda, G. (2018). Climate variability and households ’ Vulnerability to food insecurity in Ethiopia a case study of Boset District, East Shewa, Doctoral Dissertation, Addis Ababa University, Ethiopia (issue November). Center of Rural Development.
- Mwongera, C., Shikuku, K. M., Twyman, J., Läderach, P., Ampaire, E., Van Asten, P., Twomlow, S., & Winowiecki, L. A. (2017). Climate smart agriculture rapid appraisal (CSA-RA): A tool for prioritizing context-specific climate smart agriculture technologies. Agricultural Systems, 151, 192–203. https://doi.org/10.1016/j.agsy.2016.05.009
- Myers, S. S., Smith, M. R., Guth, S., Golden, C. D., Vaitla, B., Mueller, N. D., Dangour, A. D., & Huybers, P. (2017). Climate change and global food systems: Potential impacts on food security and undernutrition. Annual Review of Public Health, 38(1), 259–277. https://doi.org/10.1146/annurev-publhealth-031816-044356
- NMA. (2015). Weather records: National Metrology Agency (NMA), Addis Ababa, . http://www.ethiomet.gov.et/
- Prasad, P. V. V., Hijmans, R. J., Pierzynski, G. M., & Middendorf, J. B., (2016). Climate smart agriculture and sustainable intensification: Assessment and priority setting for Rwanda. Kansas State University, January, 91. https://www.usaid.gov/sites/default/files/documents/1860/Rwanda-CSA-SI-AssessmentReport.pdf
- Sova, C. A., Grosjean, G., Baedeker, T., Nguyen, T. N., Wallner, M., Nowak, A., Corner-Dolloff, C., Girvetz, E., Laderach, P., & Lizarazo, M. (2018). Bringing the concept of climate-smart agriculture to life. Bringing the Concept of Climate-Smart Agriculture to Life. https://doi.org/10.1596/31064
- Tambo, J. A., & Mockshell, J. (2018). Differential impacts of conservation agriculture technology options on household income in sub-Saharan Africa. Ecological Economics, 151(January), 95–105. https://doi.org/10.1016/j.ecolecon.2018.05.005
- Tekeste, K. (2021). Climate-smart agricultural (CSA) practices and its implications to food security in Siyadebrina Wayu District, Ethiopia. African Journal of Agricultural Research, 17(1), 92–103. https://doi.org/10.5897/ajar2020.15100
- Teklewold, H., Mekonnen, A., Kohlin, G., & Falco S, D. I. (2017). Does adoption of multiple climate-smart practices improve farmers’ climate resilience? Empirical evidence from the Nile Basin of Ethiopia. Climate Change Economics, 8(1), 1750001. https://doi.org/10.1142/S2010007817500014
- Tesfaye, W., Blalock, G., & Tirivayi, N. (2020). Climate-smart innovations and Rural poverty in Ethiopia: Exploring impacts and pathways. American Journal of Agricultural Economics, 103(3), 878–899. https://doi.org/10.1111/ajae.12161
- Thornton, P. K., & Herrero, M. (2015). Adapting to climate change in the mixed crop and livestock farming systems in sub-Saharan Africa. Nature Climate Change, 5(9), 830–836. https://doi.org/10.1038/nclimate2754
- Thornton, P. K., Whitbread, A., Baedeker, T., Cairns, J., Claessens, L., Baethgen, W., Bunn, C., Friedmann, M., Giller, K. E., Herrero, M., Howden, M., Kilcline, K., Nangia, V., Ramirez-Villegas, J., Kumar, S., West, P. C., & Keating, B. (2018). A framework for priority-setting in climate smart agriculture research. Agricultural Systems, 167(September), 161–175. https://doi.org/10.1016/j.agsy.2018.09.009
- van Wesenbeeck, L., & Lammers, E. (2020). Climate smart agriculture for food and nutrition security. Dutch Research Council, WOTRO Science for Global Development.
- Wainaina, P., Tongruksawattana, S., & Qaim, M. (2016). Tradeoffs and complementarities in the adoption of improved seeds, fertilizer, and natural resource management technologies in Kenya. Agricultural Economics (United Kingdom), 47(3), 351–362. https://doi.org/10.1111/agec.12235
- Wassie, A., & Pauline, N. (2018). Evaluating smallholder farmers’ preferences for climate smart agricultural practices in Tehuledere District, northeastern Ethiopia. Singapore Journal of Tropical Geography, 39(2), 300–316. https://doi.org/10.1111/sjtg.12240
- Wassmann, R., Villanueva, J., Khounthavong, M., Okumu, B. O., Vo, T. B. T., Sander, B. O., Rice, I., & Baños, L. (2019). Adaptation, mitigation and food security: Multi-criteria ranking system for climate-smart agriculture technologies illustrated for rainfed rice in Laos. Global Food Security, 23, 33–40. https://doi.org/10.1016/j.gfs.2019.02.003
- Webb, P., Flynn, D. J., Kelly, N. M., & Thomas, S. M. (2021). The transition steps needed to transform our food systems. Agriculture and Food System Nutrition.
- Wekesa, B. M., Ayuya, O. I., & Lagat, J. K. (2018). Effect of climate-smart agricultural practices on household food security in smallholder production systems: Micro-level evidence from Kenya. Agriculture & Food Security, 7(1), 1–14. https://doi.org/10.1186/s40066-018-0230-0
- Zougmor, R. B., Läderach, P., & Campbell, B. M. (2021). Transforming food systems in Africa under climate change pressure: Role of climate-smart agriculture. Sustainability, 13(8), 4305–4317. https://doi.org/10.3390/su13084305