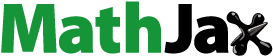
Abstract
There have been rising concerns about the implementation and effective monitoring of the Planting for Food and Jobs (PFJ) programme in Ghana. This paper examines farmers’ perceptions and impact of the PFJ programme on yield and profitability of maize in the Ashanti region of Ghana. Using data from 400 maize producers, we employ perception indices, endogenous regression, propensity score matching and inverse probability weighted regression adjustment approach to evaluate farmers’ perceptions about the programme and investigate the determinants of farmers’ participation in the PFJ programme and its impact on maize yield and profitability. Our results showed that farmers have a positive perception about the PFJ programme. Furthermore, the key factors influencing participation in PFJ are years of formal education, political affiliation, farm size, experience in maize farming, marital status, offfarm income, credit access, political affiliation and distance from farmer location to district capital. In addition, the results show that the PFJ programme has a significant positive impact on maize yield and profitability. For effective participation, the interventions should target educated farmers with large farm sizes- and living farther from the district capital. There is the need for periodic sensitization and education of farmers to encourage participation in the PFJ programme for improved yield and profitability.
Public Interest Statement
The local maize industry in Ghana has experienced several initiatives and interventions aimed at improvement from successive governments and donor partners. However, it is not enough to establish maize processing industries alone, since improvement in maize yield is crucial to reducing reliance on imports. To achieve this, the Ghanaian Government introduced the PFJ programme in 2017, with the goal of improving food security, employment, and reducing imports. However, low participation rates in the programme have been noted due to difficulty in accessing agricultural resources, including improved seed, fertilizer, market, and extension services. The study used data from 400 maize farmers in Ghana to evaluate the impact of the PFJ programme on yield and profitability. The research revealed that participants had higher gross profits compared to non-participants. The causal effect of participation was found to be positive and significantly increased gross profit and yield among programme participants in Ghana.
1. Introduction
Agriculture is still a critical sector of the Ghanaian economy providing employment and the foreign exchange earnings. However, the sector is dominated by smallholder farmers with about 90% of farm households operating on less than 2 hectares and contributing about 80% of the national agricultural output (Food Agriculture Organization FAO, Citation2018). As a consequence, there is a decline in agriculture’s contribution to the national GDP from 31.8% in 2009 to 20.2% in 2015 (Institute of Statistical, Social and Eeconomic Research ISSER, Citation2017). In response to the challenges of the agricultural sector and to boost agricultural productivity, all policies designed by the Governments since independence projected the sector to improve the welfare of major stakeholders of the agricultural value chain. Many governments in Africa are beginning to seek economic transformation through agricultural modernization by prioritizing agriculture in economic planning (African Center for Transformation ACET, Citation2017). The Government of Ghana (GoG) drawing from agricultural policies such as the Comprehensive Africa Agriculture Development Programme (CAADP) and the African Peer Review Mechanism (APRM) has played an essential role in improving the performance of the Ghanaian economy (Zimmerman et al., Citation2009). In 2002, Ghana had its first Food and Agricultural Sector Development Policy (FASDEP I) as a domestic agricultural policy, which was framed to modernize the agricultural sector. Currently, the Planting for Food and Jobs (PFJ) policy is the main flagship programme of the government for the agricultural sector. It is being implemented nationwide through the Ministry of Food and Agriculture (MoFA). It seeks to ensure adequate availability of selected crops and intensification of food crop production, extended support to non-public sector service providers, provide job opportunities for the teeming unemployed youth, and make general awareness for all formal workers to either grow some crops or establish backyard gardens (MoFA, Citation2017). One major and popular crops covered under the PFJ programme nationwide is maize.
The PFJ programme is expected to play a major role in modernizing agriculture and improving productivity to achieve food security and profitability for farmers. However, since its introduction in 2017, there has not been a study on how famers perceive the entire policy to be and how these impacts on land productivity and profitability. In addition, the programme has been faced with some challenges. For instance, it has been argued that the programme amounts to recycling of old policies since it does not offer new solutions and is launched without a comprehensive policy document or implementation plan (IMANI, Citation2017). In addition, the issue of late supply of inputs has been raised by farmers and some agricultural experts as a major concern (Mabe et al., Citation2018; Pauw, Citation2018). The challenge with the recent distribution of farm inputs, cumbersome registration process to become a participant, supply of poor-quality seeds, inadequate fertilizers and limited access to extension services are still commonplace even in the era of the PFJ (Tekuni et al., Citation2021; Awuni, Citation2017). The issue of discrimination of farmers based on political party lines has also been raised (IMANI, Citation2017; Ismaila & Tanko, Citation2021). Even though these speculations abound in the media space, there is no empirical information on how farmers perceive the PFJ programme and how the programme is impacting on crop productivity and profitability. A number of studies have analyzed factors influencing participation (see; Ansah et al., Citation2018; Ismaila & Tanko, Citation2021; Mabe et al., Citation2018) and few studies analyzed the impact of the PFJ programme (see; Lambongang et al., Citation2019; Nurideen, Citation2019Tanko et al., Citation2019). For instance, Ismaila and Tanko (Citation2021) employed Systematic Probit Regression model to analyzed the determinant of participation without analyzing the impact of the PFJ programme. Also, Tekuni et al. (Citation2021) employed linear, logit and probit models to only analyzed factors influencing PFJ participation. However, this paper adopted rigorous methods of impact evaluations including endogenous switching regression, inverse probability weighted regression and propensity score matching.
The paper assesses farmers’ perception and impact of Planting for Food and Jobs programme on maize productivity and profitability in Sekyere Kumawu District of the Ashanti Region. Specifically, it examines maize farmers’ perception of the PFJ programme, identifies the factors that influence maize farmers’ decision to participate in the PFJ programme, evaluates the impact of the PFJ programme on maize farmers’ productivity and profitability and examines the key constraints faced by maize farmers registered under the PFJ programme. In view of this, proper planning, which requires reliable data, encourages decentralization of the PFJ programme by the government makes it imperative for each district to evaluate the impact of the programme in order to strategize for the future. The findings of this research will inform stakeholders to enhance support for the programme as well as identify solutions to any challenges for effective implementation to increase productivity, food security and welfare of smallholder maize farmers. Furthermore, this paper will add to the minimal empirical evidence of the perceptions and impacts of the PFJ programme.
2. Literature review
Maize, also known as Zea mays, has been an important food crop in Ghana for centuries. Maize introduced in the late 16th century has established itself as a staple food crop in the southern region of Ghana. Today, it is the most widely grown staple food crop in sub-Saharan Africa and is cultivated across diverse agroecological zones, accounting for 21% of global production (Mulungu & Ng’ombe, Citation2019). Maize is the third most important cereal crop consumed in the world, after rice and wheat, and is grown in all continents except for Antarctica. In 2019, global maize production reached 1,148 million thousand tonnes (FAO, Citation2022). In Africa, maize is cultivated on an estimated 33 million hectares, covering 17% of cultivated land in sub-Saharan Africa (FAO, Citation2022). Nigeria is the top producer of maize on the continent, accounting for 16% of the total harvest. Maize production in Africa accounts for 7.5% of global maize production, with over 200 million people depending on it for food security in the region (Abate et al., Citation2017).
Smallholder farmers in rural areas produce over 70% of the maize in Ghana, according to MoFA (Citation2017). In 2016, Ghana’s average maize yield was 2 metric tonnes per hectare, which is below the potential yield of 5–6 metric tonnes per hectare, but this increased to 3 metric tonnes per hectare in 2020 (Knoema, Citation2020). With an annual growth rate of 8.8% between 1971 and 2020, Ghana’s maize production as of 2020 is 3 million tonnes (Knoema, Citation2020). Maize accounts for 50–60% of local cereal crop production and contributes 3.3% to total agricultural production value in Ghana (MoFA, Citation2017). This crop provides food for people, feed for livestock and raw materials for agro-based industries. Maize is the largest staple food crop in Ghana, grown by the majority of smallholder farmers across agroecological zones, and plays a critical role in improving the livelihoods of many people. The annual per capita consumption of maize in Ghana is 45 kg per person per year (Bariw et al., Citation2020).
Maize production in Ghana brings significant economic benefits, but the yield on Ghana’s maize farms is one of the lowest in the world. The current yields in Ghana are 1.73 metric tonnes/ha and 1.92 metric tonnes/ha, respectively (ISSER, Citation2017; MoFA, Citation2017). Unfortunately, over 70% of Ghana’s maize production comes from smallholders who lack the necessary resources to increase productivity, resulting in low yields (MoFA, Citation2017). As a result of low yields, Ghana’s maize output falls short of industry demand. Improving productivity for smallholder farmers through increased access to resources and knowledge transfer can help increase yield and meet the demand for maize in Ghana, improving food security and increasing income for farmers.
Over the past decade, successive governments and donor partners have implemented various initiatives to enhance the local maize industry (MOFA, Citation2017). However, the establishment of maize processing industries alone will not suffice if maize yield is not improved, which will lead to continued reliance on maize imports (MoFA, Citation2017). The Government of Ghana launched the PFJ programme to enhance food security, increase employment opportunities and reduce food imports. The programme integrates farming and marketing services to increase productivity of major food crops by providing technical knowledge and farm inputs. Consequently, the programme aims to promote good agronomic practices, fertilizer application, improved seed adoption and marketing of farm outputs. Despite subsidies, the programme has failed to improve smallholder maize farmers’ yield and profitability in Sekyere Kumawu District (IMANI, 2017). The government should evaluate and address the programme’s weaknesses by providing better extension services, access to finance, and market linkages to enhance smallholders’ productivity, competitiveness and welfare.
Farmers’ participation is crucial for the success of government input subsidy programmes, as their input is necessary to ensure the concerns of all stakeholders are addressed. Participation benefits farmers by providing them with information about their needs, priorities and capabilities, mobilizing local resources, improving utilization of facilities and services, obtaining feedback and building capacity of local institutions. Education and farming experience are significant factors that affect farmers’ participation in agricultural programmes (Khoza et al., Citation2019, Tanko et al., Citation2021; Asante et al., Citation2014; Etwire et al., Citation2013). Educated farmers are more hospitable to new ideas and are more likely to innovate, while those with several years of farming experience have extensive knowledge and should be ready to obtain information about government programmes (Khoza et al., Citation2019; Tanko et al., Citation2021). Extension agents also play a role in farmers’ participation as they help identify and distribute inputs to beneficiaries (Eneyew, Citation2012; Etwire et al., Citation2013; Khoza et al., Citation2019). Wealth status is another factor that affects farmers’ participation, with poorer farmers being less likely to receive subsidy vouchers in some countries. Other factors like farm size and distance to programme locations also affect participation, with a positive link between participation and maize farm size reported in some studies (Etwire et al., Citation2013; Motsoari et al., Citation2015). By addressing these factors, government programmes can increase participation and improve smallholder farmers’ welfare.
3. Materials and methods
3.1. Study area
The study was conducted in the Sekyere Kumawu District in Ashanti Region of Ghana, which lies between Latitudes 0° 20 and 1° 20 North and Longitudes 0° 45 and 1° 15 West. The district has a total land area of 1,500.6 square kilometres, which represents 6.2 per cent of the total land of Ashanti Region. The major rainy season is from March to July, whilst the minor rainy season is from mid-September to November, with peaks occurring in July and November, respectively. The district has relatively high humidity due to the forest cover. The population is 64,396 of which females are 52 percent and males 48 percent (GSS, Citation2021). In the district, agriculture is very dominant, which comprises farming and animal husbandry. Mostly, the cultivation of food crops such as; maize, cassava, plantain, cocoyam and others. Similarly, cash crops such as cocoa and oil palm are grown on a small-scale basis with vegetables grown on commercial purposes. Maize is one of the major crops cultivated in this district, and the maize value chain employs a greater workforce in the agricultural sector (MoFA, 2020). Figure presents the map of the study area.
3.2. Sampling technique
The data for this paper were obtained from a sample of 400 maize farmers consisting of 200 from treatmentFootnote1 and 200 from controlFootnote2 groups. The data were collected from primary and supported with secondary information (FAOSTAT, MoFA website, etc.); primary data were obtained from individual interviews using a structured questionnaire. The study used a multi-stage sampling technique to select the farmers used in this study. In the first stage, the Sekyere-Kumawu District of Ghana was purposely selected because agriculture is the most dominant occupation in the district. Also, the district is endowed with abundant arable lands which support different types of cash crops such as cocoa, oil palm, citrus cashew, mango and food crops like cassava, plantain, rice, yam, cocoyam, maize and vegetables in Ghana. Five (5) communities were purposively selected from a list of 16 major maize-producing communities from the district. The selection of these communities was based on their major levels of maize production. A simple random sampling technique was further used to select the number of maize farmers from each settlement area. The farmers were selected across the following communities with the following details: Kumawu (100), Woraso (90), Gyedi (70), Bodomase (80) and Abotanso (60). Finally, 200 of the treatment group from Kumawu, Woraso and Gyedi and few selected from the other two communities and 200 of the control group were randomly selected from Bodomase and Abotanso with few from other three communities. This difference across the communities was due to participants and non-participants’ proportion in each community. The data were collected between March and June 2020.
3.3. Empirical strategy
To achieve the objectives of the study, various methods were employed, including perception indices, the endogenous switching regressions model, propensity score matching and inverse probability weighted regression adjustment. The socioeconomic characteristics of farmers were analyzed using descriptive statistics, and the methods used in the study are further discussed in the next section
3.3.1. Perception indices
The data collected were summarized using the descriptive statistics. A five-point Likert scale (1=strongly disagree, 2=disagree, 3=neutral, 4=agree and 5=strongly agree) was used to measure maize farmers’ perception of the PFJ programme, and the mean score (M.S) was first estimated and explicitly expressed as:
where; fsa = frequency of strongly agree, fa =frequency of agree, fn = frequency of neutral, fd = frequency of disagree, fsd = frequency of strongly disagree, and x = number of maize farmers who responded to the specific perception statement.
The overall perception index (P.I) was calculated as;
where P.I = overall perception index and n = number of perception statements.
3.3.2. Estimation of yield and profitability
The yield was computed using the expression below:
where = yield,
= total output of maize (in kg) and
= land area under maize production (acre).
The profitability was estimated using the expression:
where π = profit, TR= total revenue (quantity of maize in Kg * unit price of maize in Ghanaian cedis), GM=gross margin, TVC=total variable cost, BCR=benefit–cost ratio, TPC = total production cost (all costs of inputs including seeds, fertilizers, herbicides, labour, etc., were summed for an acre of maize produced).
3.3.3. Endogenous switching regression
In this paper, a farmer who decides whether to join a PFJ programme or not, he/she first assesses the benefits associated with participation. Considering the theory of rational decision (Rahm & Huffman, Citation1984), it is expected that the expected benefit of participant () generated from participation exceeds that of non-participant (
), that is
. Therefore, a choice problem is encountered which is analysed using a binary Probit model (Abdulai & Huffman, Citation2014) expressed as:
Xi signifies a vector of explanatory variables that affects farmer’s decision to participate in PFJ programme. To address endogeneity problem, the control function approach was employed (Abdulai & Huffman, Citation2014; Ngwako et al., Citation2021).
Subsequently, separate gross margins and yield models are specified for participants and non-participants of PFJ expressed as:
where Xi signifies a set of factors (socioeconomic and institutional variables). signify vectors of parameters to be estimated, and
are assumed to have a trivariate normal distribution with mean zero and non-singular covariance matrix (Maddala, Citation1986), expressed as:
where = variance of
,
= covariance of
and
,
= covariance of
and
,
= variance of
, and
= variance of
. Consequently, the econometric literature suggests that both the outcomes of participants (Y1) and non-participants (Y0) are not observed simultaneously (Abdulai & Huffman, Citation2014). Hence, the covariance of
and
is not defined with no information regarding the covariance. For this reason, EquationEquation 19
(19)
(19) to EquationEquation 19
(19)
(19) should be estimated simultaneously such that correlation between the error terms is accounted for. In this regard, the ordinary least squares or maximum likelihood estimation approaches cannot be used. Therefore, we employed a more robust estimation approach; thus, a full information maximum likelihood estimation (FIMLE) best suit appropriately (Lokshin & Sajaia, Citation2004). This can be expressed as:
where (.) signifies cumulative normal distribution function,
(.) signifies a normal density distribution function,
signifies optional weight for observation i, and
can be expressed as:
where signifies the correlation term between
and
,
signifies the correlation term between
and
. Therefore, the correlation terms are assumed to have values range from −1 to 1 and
and
are always positive. To achieve this, the FIMLE directly computes estimates
,
, and
, where
is defined as:
We used political affliction and perception about the PFJ programme as instrument variables. We assumed that both political affiliation and perception directly affect decision to participate in the PFJ programme but does not affect the outcome variables (yield and gross profit). Lastly, after the estimation of the parameters in models, with the impact of PFJ programme on maize yield and gross margins, the average treatment effect on the treated (ATT) is estimated as:
Whether the is significantly different or not from zero, standard t-test is used.
3.3.4. Propensity score matching
The average impact of PFJ programme on maize was estimated using the propensity score matching method (PSM). In order to get rid of biases, the PSM is the appropriate technique to control selection as result from the non-randomizing treatment to the PFJ programme. For research works on evaluation of impacts, the majority focus on estimating the average treatment effect (ATE) and the average treatment effect on the treated (ATT) (Abdulai & Huffman, Citation2014; DiFalco et al., Citation2011; Ma & Abdulai, Citation2019; Ngwako et al., Citation2021). The impact of PFJ programme on maize yield and profitability following other studies employed the propensity score matching as robustness check of the endogenous switching regression (ESR) results (see; Ma & Abdulai, Citation2019). The PSM consisted of two stages including a binary model (probit model) and ATT specified as:
where {.} denotes normal cumulative distribution and X denotes vector of pre-treatment characteristics. Subsequently, the PSM considers selection on observables only based on the conditional independence assumption (CIA) that potential outcomes are independent of the participation decision conditional on covariates. After this, ATT is computed as:
3.3.5. Inverse probability weighted regression adjustment
Following Robins et al. (Citation2007) and Wooldridge (Citation2007), the IPWRA as a robustness check for any potential biased estimates of average treatment effect on the treated (ATT) results from the scores of the propensity estimates computed based on the outcome variables. For misspecification of either the treatment or outcome variable, the IPWRA gives consistent estimates because of its doubly robust characteristics (Wooldridge, Citation2003). The study adopted IPWRA estimator as robust check of the ESR model (see, Cattaneo, Citation2010; Tan, Citation2010). For continuous outcome, linear regression model fit on treatment variable computes the inverse probability by calculating the group difference of the adjusted mean treatment group (Lunceford & Davidian, Citation2004). The IPWRA is expressed as:
where t = treatment variable, x = independent variables, and T = outcome variable.
All analyses were conducted using Statistical Package for Social Scientist (SPSS) version 21 and Stata software version 15. The explanatory variables are defined in Table with their a priori signs.
Table 1. Explanatory variables in the models
4. Results and discussion
4.1. Socio-economic characteristics of maize farmers
Table presents the descriptive statistics of the socio-economic characteristics of maize farmers. Participation in PFJ programme among farmers has some variables statistically significant for participants and non-participants. For instance, years of schooling, farm size, marital status, household size, off-farm activities, distance to district capital and distance to nearest agro-shop were statistically significant among the groups. The males were more than females representing 74.3% and 25.8%, respectively. This was not surprising because in most rural farm households in Ghana are male-dominated (MoFA, 2020). Also, the females do not inherit land from a deceased parent but have to farm through the good-heartedness of the husband if married or through father or male family head (Quaye et al., Citation2019). The average mean age of 50 years, hence, presents a generally adult maize farming population. Consequently, more youth must be encouraged into maize production. A typical maize farming household consists of five persons. This was relatively higher than the national average of four (4) members per household (GSS, Citation2021). About 59% of the farmers engaged in off-farm activities, and 72% of respondents are married. The average off-farm income of the respondents was GHS2,626.66 ($445.99). The mean years of schooling among the respondents was 7 years which is higher than the average Ghanaian schooling years of 5.1 (GSS, Citation2021). Farmers who are educated are more adopters to new ways and methods of farming. Consistent with Asante et al. (Citation2019), education enables farmers to access and process information, thus making educated farmers more likely to adopt improved technologies. Farmers had 14.8 years of farming maize, implying that respondents are more experienced in maize farming. However, agriculture sector of Ghana is considered to have higher illiteracy rate among smallholder farmers which is an impediment to yield and yield growth (GSS, Citation2021).
Table 2. Descriptive statistics of socio-economics characteristics of maize farmers
Close to 73.3% of the farmers had no credit. This could be because of the bureaucratic process in accessing credit coupled with the request for collaterals in applying for it as well as the high interest in credits from financial institutions. On average, farmers had contacts with extension agent about four times in a year. This response indicated that farmers receive extension services and advice in order to adhere to good agricultural practices to improve maize yield (Etwire et al., Citation2013). While 42% of the respondents were in favor of the ruling party, close to 62% of the respondents were affiliated to other political parties. 72% were not members of any farmer-based organization (FBO), while 28.0% were members of FBO with average of 2 years of membership with the FBO. This implies that either most respondents were not interested in FBOs due to cumbersome registration, mistrust and unsustainability or there may not be more vibrant FBOs in the community or the FBO concept is quite young in the district (Ismaila & Tanko, Citation2021; Mabe et al., Citation2018). The average distance to district capital and nearest agro-shop was 3.5 km and 1.39 km, respectively.
4.2. Packages for maize farmers under PFJ programme
Table presents packages for maize farmers under PFJ programme. The PFJ programme provides four packages to participants. These includes improved seed, fertilizer, extension service and ready market. The results show that 67% of the respondents used improved seeds, implying that most respondents preferred improved seeds. Also, farmers believed that seeds are the signposts for harvesting more maize, and hence, farmers tend to either save better seeds or improved seeds purchased from the market or from government (MoFA, 2020; Pauw, Citation2022). About 68.5% of the respondents received fertilizers from the PFJ programme, while 97.0% had extension support with 45.0% of the respondents having access to ready market. These findings imply that the PFJ programme is contributing impressively in enhancing maize production (Pauw, Citation2022; Yakubu et al., Citation2019). On average, 7 kgs of improved seeds were obtained by respondents amounting to GHS13.90 ($2.37), all of which were obtained on credit. In addition, respondents on average purchased 5.2 bags (50 kg) of fertilizers which amounted to average of GHS390.00 ($66.41) of fertilizers and paid in full with no outstanding during the 2019 season.
Table 3. Packages under PFJ programme
4.3. Farmers’ perception about PFJ programme
Table presents the results of farmers’ perceptions about PFJ programme. The responses were measured on five-point Likert scale where farmers were given the opportunity to choose among the following options: strongly disagree, disagree, neutral, agree and strongly agree. Perception index was computed to understand the overview of farmer’s perceptions. The timely supply of seeds and fertilizers is very vital in maize productivity. Majority of the respondents (55.5%) strongly agree that fertilizers are supplied on time as compared to seeds of 51.5%. In terms of quality of seeds and fertilizers, 67.0% and 68.5% of the respondents strongly agree. About 51.5% and 50.5% of the respondents strongly agree that adequate seeds and fertilizers are obtained through the PFJ programme, respectively. Respondents also strongly agree that access to seeds and fertilizers have been improved by the PFJ programme comprising of 57.0% and 58.5%, respectively. Extension contacts and improving crop productivity score a mean of 4.8 each representing 71.5% and 80.5%. Grievance handling mechanism scores a mean of 4.4 representing 53.0% of respondents strongly agree.
Table 4. Perception of respondents about PFJ programme
However, the ready market as a PFJ package scores a mean of 3.0 representing 40.0% of respondents who disagrees with this package. Other respondents showed dissatisfaction with ready market (6.5%) as the PFJ programme encourages in the study area. This concurs with Sharma et al. (Citation2016) who reported that farmers who have easy access to markets in a community will still look out for alternative markets with less transaction costs incurred. Overall perception index was 4.4, indicating that respondents had positive perception towards the PFJ programme. These results agree with Jayne et al. (Citation2018), Mabe et al. (Citation2018) and Yawson et al. (Citation2010) who reported that most of the respondents almost agreed that the subsidy programme was very good and there is a need to call for its protraction with necessary corrections.
4.4. Maize productivity and profitability
Table presents the results of profitability of participants and non-participants of PFJ programme during 2019 season (per acre basis). In Ghana, cultivation of maize may not be solely for household consumption. But most farmers may be interested in selling their output to in order to raise income for other household needs. Therefore, it is necessary to analyze farmers’ maize profitability since they invest into farming and expect returns at the end of every season.
Table 5. Maize revenue and costs analysis during 2019 season for participants and non-participants PFJ programme (per acre basis)
Based on Table , it was revealed that total variable cost accounted for an overwhelming 85.4% and 84.5% of the total cost of production for participants and non-participants, respectively. On average, it costs participant and non-participant of GHS2,007.21 ($341.78) and GHS1,793.36 ($305.37), respectively, to cultivate one acre of maize farm in the study area. An average of GHS2,285.05 ($389.09) and GHS1,928.10 ($328.31) per acre was obtained as revenue to participant and non-participant of the programme. Pauw (Citation2022) indicated that the income generated by farmers through maize production was higher, yet it remained lower than what they could have earned in the absence of the PFJ programme.
It was indicated that the net farm profit and gross margin of an average participant were GHS277.84 ($47.31) and GHS569.56 ($96.98) per acre, respectively. The net farm profit and gross margin of an average non-participant were GHS134.64 ($22.93) and GHS412.10 ($70.17) per acre, respectively. BCR of participants and non-participants was 1.14 and 1.08, respectively. This indicates that for every cedi invested into maize production, GHS1.14 and GHS1.08 will be realized as revenue for participants and non-participants. This means that participants and non-participants of the programme will realize 1.14% and 1.08% from maize production per acre. On average, participants and non-participants of the programme generated a yield of 4.1bags (100 kg/bag) and 3.4bags per acre. This indicates that an average participant and non-participant will generate maize output of 4.1bags and 3.4bags per acre from their production, respectively. The finding of Pauw (Citation2022) found relatively higher maize yield (40%) and profitable maize production among participants of PFJ programme in Ghana.
Comparatively, participation in the PFJ programme is found to be profitable than non-participation at 1% significance level (Table ). On average, participants earn additional revenue of GHS356.95 per acre and accrued net profits of GHS143.2 ($24.38) per acre. The study indicated that an average participant spends an additional GHS213.85 ($36.41) to produce an acre of maize. This additional benefit and costs could be linked to the fact that participants are able to intensify their production while expanding their farm size to cultivate more maize since there are available subsidized inputs under the programme (MoFA, 2020; Mabe et al., Citation2018; Tanko et al., Citation2021).
4.5. Endogenous switching regression results for participation and impact of PFJ participation on productivity and profitability
The maximum likelihood estimates of the determinants and impact of participation on gross margins and maize yields are presented in Table . The selection equations denote the determinants of participation in the PFJ programme. The results of the Wald test statistics in the Table are 333.15 and 162.96, respectively, and statistically significant at 1%. This means that the explanatory variables jointly influenced the dependent variable.
Table 6. Endogenous switching regression results for participation and impact of participation on gross margin and yield
The empirical results show that the likelihood of participating in the PFJ programme is generally associated with age and statistically significant at 1%. This implies that older farmers are more likely to participate in agricultural development programmes. Consistent with previous studies (Awotide et al., Citation2015; Baffoe et al., Citation2014; Etwire et al., Citation2013; Nxumale & Oladele, Citation2013), older farmers may dwell on their positive experiences and hence may be more likely to participate in agricultural development programmes. Higher education of farmers represents positive and significant effect; thus, more educated farmers are more likely to participate in the programme. Educated farmers have the notion of easily approving new technologies and practice knowledge acquired (Khoza et al., Citation2019, Tanko et al., Citation2021; Asante et al., Citation2014; Etwire et al., Citation2013). Experience in maize farming was found to be positive and significant, implying that experienced farmers are equipped with knowledge and expertise to decipher the importance of the agricultural programme in nexus to maize production. Similar results were obtained by Tekuni et al. (Citation2021), Ntshangase et al. (Citation2018) and Tarawali et al. (Citation2012) who found a positive relationship between experience in farming and participation. Additionally, the result shows that farmers who are married are more involved to family activities which constrained participation in the programme. Consistent with Oladejo et al. (Citation2011), an inverse relationship between marriage and participation in agricultural programmes was found. They could hence divert the funds which were marked for maize production for other family purposes by participating in the programme in order to obtain these inputs and services on credit or at a relatively lower cost (Nnadi & Akwiwu, Citation2008; Pauw, Citation2022). Also, farm size has a positive significant effect on participation, indicating a higher probability of participation in the programme for farmers with large farm sizes. For instance, additional acre of land is a sign of an expansion of farm from subsistence to commercialization. Kuwornu et al. (Citation2014) asserted that larger farm sizes are associated with smallholder farmers’ diversification into agricultural activities. This result is consistent with Alhassan et al. (Citation2021), Etwire et al. (Citation2013) and Motsoari et al. (Citation2015).
The coefficient of the political affiliation variable is also positive and significant, suggesting that being a member of political affiliation tends to increase the likelihood of participating in the programme. Generally, farmers are more inclined to agricultural programmes associated with their political interest. This result is consistent with Brodie et al. (Citation2009), and Ismaila and Tanko (Citation2021) reported that social and economic groups a person belongs to have an impact on his/her level of participation which often led to less access to resources and practical support making their participation in community development initiatives rather difficult and limited. FBO membership is also positive and significant, indicating that farmers that are of group membership are more likely to participate in the programme. The notion of being a FBO member is to have access to inputs, credits, information flow as well as technical advice which enhance participation in agricultural programmes (Abdallah et al., Citation2021; Mensah & Agbekpornu, Citation2015; Motsoari et al., Citation2015).
Frequent extension visits variable is also positive and significant, suggesting that farmers are more likely to participate in the programme. Extension agent serves as bridging information gap and advocate the benefits about the programme; hence, frequent visits could play a key role to enhance participation. Consistent with Etwire et al. (Citation2013), a positive relationship between access to extension contacts and participation in agricultural projects was found. The distance to district capital and distance to nearest agro-input shop have negative effect and are statistically significant, suggesting that greater distance to the nearest district or agro-shop decreases participation in the programme. Consistent with the findings, there is a negative relationship between distance and participation (Eneyew, Citation2012; Khoza et al., Citation2019).
Other factors that influence participation in PFJ programme include price of maize, fertilizer, seed and labor. The positive effect of the fertilizer subsidy indicates that farmers with access to the fertilizer subsidy are more likely to participate in the programme. Besides the importance of fertilizer in maize production, experiences from participation in the subsidy programme increase the likelihood of participating in the PFJ programme. Also, maize seed subsidy was positive and significant, implying that adequate quantity of maize seeds on time increases farmers likelihood of participation in the programme. Generally, both maize seed and fertilizer subsidies are the ultimate goal of the programme to enhance maize production (MoFA, Citation2017). Further, the price of maize had a positive and significant influence on participation, implying that higher price of maize at the market increases the chance of participation in the programme. Higher maize prices serve as an incentive for farmers to increase production, thereby increasing the likelihood of participating in the programme. Thus, farmers are enthused to produced more maize for better price at the market. The negative effect and significant of labor variable indicate that farmers who have access to lower cost of labor are more likely to participate in the programme.
The impact of participation in PFJ programme on gross margins and yields is presented in Table , for participants and non-participants, respectively. Generally, we estimate the impact of socioeconomic and farm level characteristics on gross margins and maize yields for participants and non-participants of the PFJ programme. In our estimates, the overall perception about the PFJ programme and political affiliation was used as an instrument variable. IV is expected to affect participation in selection equations. However, it is expected not to affect gross margins and maize yields directly considering participants or non-participants.
Obviously, the results show that older farmers are key to explaining higher maize yields and higher gross margins among participants of the programme. Age is positive and significant, implying that older farmers leverage on the benefits of programme to enhance maize yields. Similar studies reported a positive effect of age which measures farmer’s experience on crop productivity (Ngwako et al., Citation2021; Sebatta et al., Citation2014). Education is key to determining the farmers yield and gross margins. For instance, additional year spent in school increased yield and gross margins among participants. Overall, more educated farmers are expected to generate higher maize yields and gross margins because they can intuit the numerous benefits of the programme to boost maize production. Consistent with Kassie et al. (Citation2011), experience in maize farming has a positive and significant impact on both maize yield and gross margins, implying a higher maize yield and gross margins for participants and non-participants, respectively. The result shows a negative effect of household size among participants, suggesting that an increase in household size results in a decrease yield. Contradicting with Abdulai and Huffman (Citation2014) and Ersado et al. (Citation2004) who reported that larger households could probably practice labor intensive farming, off-farm income has a negative and significant effect on yield and gross margins for participants and non-participants. Farmers who engaged in off-farm activities are expected to have higher income and diversify their incomes. However, the finding of this study is similar to other study suggesting that farmers engage in activities other than faming to warrant basic needs (Ngwako et al., Citation2021).
Interestingly, extension visits have differential impacts on both participants and non-participants. The positive and significant coefficients suggest that through extension services we can improve greatly both maize yields and gross margins for participants but greatly improve only the gross margins for non-participants. Participation in agricultural extension services increases farmers access and availability of programme information which could increase the likelihood of participation and impact their incomes (Anang & Ayambila, Citation2020; Ngwako et al., Citation2021; Richard, Citation2017). Also, credit access has a positive and significant impact, implying that credit access enhances higher maize yields for participants. The coefficient of fertilizer subsidy was positive and significant, suggesting higher maize yields recorded among participants but rather reduced maize yields for non-participants. With regard to seed, it was found to be positive and significant, indicating higher maize yield and gross margins for participants. The findings could be attributed to the fact that participants received both seed and fertilizer subsidy and, hence, could decrease farmers’ input costs. Additionally, the price of maize was negative and significant, indicating that lower price reduces maize yields for both groups but tended to increase gross margins among participants. The coefficient of herbicide was positive and significant, suggesting that participants obtained higher maize yields and gross margins than non-participants. Generally, fertilizer, seed and herbicide contribute to participants higher maize yields leading to higher gross margins. These findings are consistent with Abdulai and Huffman (Citation2014).
4.6. Impact of PFJ on respondents’ profitability and productivity
Table presents the results of the impacts of the PFJ programme on gross margin and maize yield. The paper evaluated the impact of PFJ programme on maize farmers’ yield and profitability. The ESR model was applied using the gross margin per acre and yield per acre during the season.
Table 7. Impact of PFJ programme on maize productivity and profitability
The mean of yield per acre of treated and non-treated participants was 8.58 and 3.10 bags per acre. The mean of gross margin per acre of treated and non-treated participants of the programme was GHS668.27 ($113.79) and GHS478.87 ($81.54), respectively. It was indicated that gross margin per acre was statistically significant at 1% level, implying that the casual effect of participation in the programme is GHS189.40 representing 40% increase in gross margins. Consequently, the yield per acre is also significant at 1% level. The causal effect of participation in the programme is 5.48 bags per acre, suggesting about 27.1% increase in productivity per acre. This could be linked to the fact that the PFJ programme in the study area has been a supportive arm to participants, therefore increasing their productivity and profitability as planned by the government. The findings are consistent with Minten and Barrett (Citation2008) who found that agricultural projects can improve farm productivity and household incomes.
4.7. Robustness check
The propensity score matching (PSM) and inverse probability weighted regression adjustment (IPWRA) are used as complement to check the robustness of the ESR model. These robustness check estimators give consistent estimates of the treatment and outcome with either misspecification in one or both variables (Wooldridge, Citation2003). Tables present the propensity score and IPWRA-based results, respectively. In Table , the results show that participation led to 5.38% to 7.01% increase in yield, while gross margin increased from GHS109.89 to GHS257.71. The finding shows that participation in the programme is vital to improve productivity and gross income of farmers in Ghana. Similar finding obtained by found that participation in agricultural technology shows a positive impact on rice yield and farm income.
Table 8. Propensity score matching results
Table 9. Inverse probability weighted regression adjustment (IPWRA)
Figure presents the histogram distribution of the propensity scores among maize farmers who are on support and those not on support. The figure depicts that bottom half of the figure shows the propensity scores distribution for the untreated, while the upper half refers to the treated individuals. Evidently, the common support condition is satisfied as there is an overlap in the distribution of the propensity scores for treated and untreated farmers.
Further, IPWRA results indicated in Table reveal that participation in the programme has a greater potential causal effect on farmers’ yield and gross income. Specifically, the casual effect of yield and gross income increased by 6.88 kg/acre and GHS262.83, respectively. It was evident that participation in agricultural interventions generally improves yield and farm income, similar to the finding with Danso-Abbeam and Baiyegunhi (Citation2018).
5. Conclusions and recommendations
This paper examines the perceptions and impacts of the PFJ on yield and incomes of smallholder maize farmers in the Sekyere Kumawu District of Ghana. The perception indices, endogenous switching regression and inverse probability weighted regression adjustment were employed to assess and analyse the perceptions, factors influencing participation and constraints faced by farmers, respectively. The results revealed that majority of maize farmers (59%) engaged in off-farm activities, whereas relatively women involvement was 25.8% in maize farming. Further, a typical maize farmer is on average 49.5 years of age which indicated an economically productive-active maize growing population age as recommended by FAO. In addition, the result shows that respondents had positive perception about the PFJ programme. Majority of the respondents believed that the PFJ programme has contributed to improvement in crop productivity and access to extension services.
In addition, the study found out that maize production is profitable venture for both participants and non-participants of the PFJ programme with benefit–cost ratio of 1.14 and 1.08, respectively. The gross margin of an average participant and non-participant was GHS569.56 ($96.98) and GHS412.10 ($70.17) per acre, respectively. On average, the yield of participant and non-participant was 4.1 and 3.4 bags (100 kg) per acre, respectively. The programme was found to have a significant positive impact on maize productivity and profitability.
The study further estimates participation and impact of participation in PFJ programme on maize yields and gross margins. The results show that selection of sample would have resulted into biasness without considering the estimates of participation decisions and, thus, selection equation. The participation factors that influence farmers to participate in the PFJ programme revealed that age, years of schooling, political affiliation, farm size, experience in maize farming, married status, off-farm income and credit access all had positive significant influence. However, distance from farmer location to district capital had a negative influence. This implies that the longer distance from farmer location to district capital discourages respondent interest in participating in the programme. The coefficient of years of schooling was positive and has significant influence on participation together with impact of yields and gross margins. This suggests that education is key especially in rural farm settings. Also, household size was negative and has significant impact on both maize yields and gross margins but not significant on participation decision. Our findings also confirm the importance of FBOs and extension services to reduce information uncertainties; thereby, these groups formations could help to have greater access to the programme packages. Also, the findings showed that participants of the programme with smaller farm size capitalize on the programme benefits to expand their farms. Further, the seed and fertilizer subsidies contributed to higher participation in the programme as well as significant impact on maize yields and gross margins for participants.
Consequently, the impact of participation on maize yields and gross margins showed that the casual effect of participation in the programme increases gross margins by about 40% and productivity per acre by 27.1%, indicating that the programme contributes significantly to maize farmers’ productivity and profitability. Also, the positive and significant causal effect implies that potentially the PFJ programme plays a vital role in raising farm productivity and income of smallholder maize farmers across the country.
There is, therefore, the need for periodic sensitization and education of farmers through the mass media to encourage participation in the PFJ programme for improved yield and profitability. Such effort could be complimented through the timely supply of pesticides and fungicides and improve access to credit. Further, bad road networks leading to high transportation cost in the study area hinder maize production activities. Hence, the District Assembly should put in measures to ensure construction of the remaining untarred roads to ease transportation and cut down post-harvest losses in the study area. Moreover, the programme established under MoFA should continually invest in development of extension services since extension services could clearly define the objectives and deepened the provisions of the PFJ programme.
Acknowledgments
The authors appreciate the effort and patience of all the farmers and extension agents in the study area.
Disclosure statement
No potential conflict of interest was reported by the author(s).
Data availability statement
The data that support the findings of this study are openly available in Data_set at https://drive.google.com/file/d/1qaH10NXAuJNcO-EZTkO9Sh8zJO5qmNDt/view?usp=sharing.
Additional information
Funding
Notes on contributors
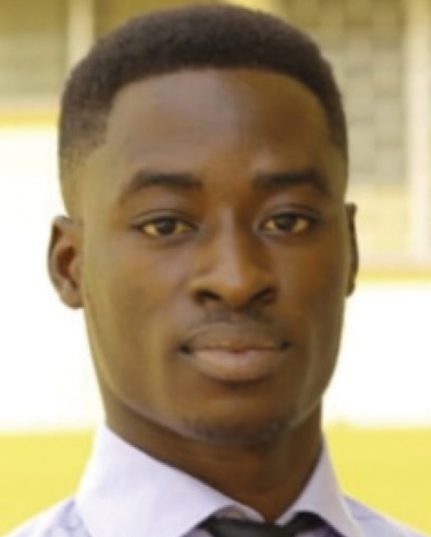
Stephen Prah
Stephen Prah is an MPhil student in Agricultural Economics at KNUST. Certified in Rainforest Alliance, Food Safety, Fairtrade, and Organic Farming. National Tutor at Greening Africa Together – Ghana, and Agronomist at Holland Greentech.
Bright O. Asante
Bright Owusu Asante, a Senior Lecturer, Department of Agricultural Economics, Agribusiness and Extension, KNUST, Kumasi. His research focusses on agricultural economics, agribusiness, food security and development economics.
Robert Aidoo
Robert Aidoo is an Associate Professor, Department of Agricultural Economics, Agribusiness and Extension, KNUST, Kumasi. He has conducted various studies in the agricultural/agribusiness sector over the past 20 years.
James O. Mensah
Osei Mensah James is an Associate Professor, Department of Agricultural Economics, Agribusiness and Extension of KNUST, Kumasi, specializes in agricultural value chains, food and consumer economics, entrepreneurship, TQM and agricultural project evaluation.
Fred Nimoh
Fred Nimoh is an Associate Professor, Department of Agricultural Economics, Agribusiness and Extension, KNUST, Kumasi. His research works include production economics, food and consumer economics, and development economics.
Notes
1. Treatment group consist of the maize farmers who are members of the Planting for Food and Jobs (PFJ) programme in the district.
2. Control group consist of maize farmers who are not members of the PFJ programme in the district.
References
- Abate, T., Fisher, M., Abdoulaye, T., Kassie, G. T., Lunduka, R., Marenya, P., & Asnake, W. (2017). Characteristics of maize cultivars in Africa: How modern are they and how many do smallholder farmers grow? Agriculture & Food Security, 6(1), 1–23. https://doi.org/10.1186/s40066-017-0108-6
- Abdallah, S., Alhassan, H., Donkoh, S. A., & Appiah-Adjei, C. (2021 2). Participation in “planting for food and jobs” programme and Commercialization among maize farm households in Savelugu Municipality, Ghana. Ghana Journal of Development Studies, 18(2), 1–24. https://doi.org/10.4314/gjds.v18i2.1
- Abdulai, A., & Huffman, W. (2014). The adoption and impact of soil and water conservation Technology: An endogenous switching regression application. Land Economics, 90(1), 26–43. https://doi.org/10.3368/le.90.1.26
- African Center for Transformation (ACET). (2017). Agriculture powering Africa’s economic Transformation. African Transformation Report.
- Anang, B. T., & Ayambila, S. N. (2020). Participation in agricultural extension and labor productivity: A case study of smallholder farmers in Ghana. International Journal on Food System Dynamics, 11(3), 297–306. https://doi.org/10.18461/ijfsd.v11i3.55
- Ansah, I. G. K., Lambongang, M., & Donkoh, S. A. (2018). Ghana’s planting for food and jobs programme: A look at the role of capability in farmers’ participation. Journal of Human Development & Capabilities, 21(2), 161–182. https://doi.org/10.1080/19452829.2020.1745162
- Asante B. O., Temoso O., Addai K. N., & Villano, R. A. (2019). Evaluating productivity gaps in maize production across different agroecological zones in Ghana. Agricultural Systems, 176, 102650. https://doi.org/10.1016/j.agsy.2019.102650
- Asante, B. O., Villano, R. A., & Battese, G. E. (2014). The effect of the adoption of yam minisett technology on the technical efficiency of yam farmers in the forest-savanna transition zone of Ghana. African Journal of Agricultural and Resource Economics, 9(2), 75–90. https://doi.org/10.22004/ag.econ.176478
- Awotide, B. A., Abdoulaye, T., Alene, A., & Manyong, V. M. (2015). Impact of access to credit on agricultural productivity: Evidence from smallholder cassava farmers in Nigeria. International Conference of Agricultural Economists, 1–34. Retrieved from http://purl.umn.edu/210969.
- Awuni, F. (2017). Fewer farmers registering for planting for food and jobs – minister. Availabile at: https://citifmonline.com/2017/06/fewer-farmers-registering-for-planting-for-food-jobs-minister/.
- Baffoe, G., Matsuda, H., Nagao, M., & Akiyama, T. (2014). The Dynamics of rural credit and its impacts on agricultural productivity: An empirical study in rural Ghana. OIDA International Journal of Sustainable Development, 7(5), 19–34. https://ssrn.com/abstract=2495848
- Bariw, S. A., Kudadze, S., Adzawla, W., & Yildiz, F. (2020). Prevalence, effects and management of fall army worm in the Nkoranza South Municipality, Bono East region of Ghana. Cogent Food & Agriculture, 6(1), 1800239. https://doi.org/10.1080/23311932.2020.1800239
- Brodie, E., Cowling, E., & Nissen, N. (2009). Understanding participation: A Literature Review. National Council for Voluntary Organisations.
- Cattaneo, M. D. (2010). Efficient semiparametric estimation of multi-valued treatment effects under ignorability. Journal of Econometrics, 155(2), 138–154. https://doi.org/10.1016/j.jeconom.2009.09.023
- Danso-Abbeam, G., & Baiyegunhi, L. J. (2018). Welfare impact of pesticides management practices among smallholder cocoa farmers in Ghana. Technology in society, 54, 10–19.
- DiFalco, S., Veronesi, M., & Yesuf, M. (2011). Does adaptation to climate change provide food security? A micro-perspective from Ethiopia. American Journal of Agricultural Economics, 93(3), 829–846. https://doi.org/10.1093/ajae/aar006
- Eneyew, A. (2012). Determinants of livelihood diversification in pastoral societies of Southern Ethiopia. Journal of Agriculture and Biodiversity Research. 1 (3), 43–52. http://www.onlineresearchjournals.org/JABR
- Ersado, L., Amacher, G., & Alwang, J. (2004). “Productivity and land enhancing technologies in Northern Ethiopia: Health, public investments, and sequential adoption. American Journal of Agricultural Economics, 86(2), 321–331. https://doi.org/10.1111/j.0092-5853.2004.00581.x
- Etwire, P. M., Dogbe, W., Wiredu, A. N., Martey, E., Etwire, E., & Robert, K. (2013). Factors influencing farmer ’ s participation in agricultural projects: The case of the agricultural value chain mentorship project in the northern region of factors influencing farmer ’ s participation in agricultural projects: The case of the agricultural value chain mentorship project in the northern region of Ghana. Journal of Economics & Sustainable Development, 4, No.10. https://www.iiste.org/Journals/index.php/JEDS/article/view/6509
- Food and Agriculture Organization (FAO). (2018). Ghana: Food and Agriculture Policy Development. (Retrieved March , 2021). www.fao.org
- Food and Agriculture Organization of the United Nations. (2022). The state of food security and nutrition in the World 2021. FAO.
- Ghana Statistical Service (GSS). (2021). Population and housing census: Summary report final results, ghana Statistical Service.
- IMANI International. (2017). The Highs and Lows of Government’s “Planting for Food and Jobs” campaign and Recommendations. IMANI Report, Ghana.
- Institute of Statistical, Social and Economic Research (ISSER). (2017). The state of the Ghanaian economy in 2016: A critical Review. University of.
- Ismaila, S., & Tanko, M. (2021). Exploring relative deprivation theory in the rice industry: Planting for food and jobs (PFJ) in northern Ghana. Technology in Society, 65, 101556. https://doi.org/10.1016/j.techsoc.2021.101556
- Jayne, T. S., Mason, N. M., Burke, W. J., & Ariga, J. (2018). Review: Taking stock of Africa’s second-generation agricultural input subsidy programs. Food Policy, 75(2018), 1–14. https://doi.org/10.1016/j.foodpol.2018.01.003
- Kassie, M., Shiferaw, B., & Muricho, G. (2011). Agricultural Technology, crop income and poverty alleviation in Uganda. World Development, 39(10), 1784–1795. https://doi.org/10.1016/j.worlddev.2011.04.023
- Khoza, T. M., Senyolo, G. M., Mmbengwa, V. M., Soundy, P., & Sinnett, D. (2019). Socio-economic factors influencing smallholder farmers ’ decision to participate in agro- processing industry in Gauteng province, South Africa. Cogent Social Sciences, 5(1), 1–14. https://doi.org/10.1080/23311886.2019.1664193
- Knoema. (2020). Maize production quantity. (Retrieved February , 2022 from). https://www.knoema.com
- Kuwornu, J. K. M., Bashiru, M., & Dumayiri, M. (2014). Farm households’ livelihood diversification into agroprocessing and non-agro-processing activities: Empirical evidence from Ghana. Information Management and Business Review, 6(4), 191–199. https://doi.org/10.22610/imbr.v6i4.1114
- Lambongang, M., Ansah, K., & Donkoh, S. A. (2019). Participation and yield effects of Ghana’s planting for food and jobs programme in Bunkpurugu-Yunyoo district. Ghana Journal of Agricultural Economics and Agribusiness, 2(1), 86–98. http://hdl.handle.net/123456789/2752
- Lokshin, M., & Sajaia, Z. (2004). Maximum likelihood estimation of endogenous switching regression models. Stata Journal, 4(3), 282–289. https://doi.org/10.1177/1536867X0400400306
- Lunceford, J. K., & Davidian, M. (2004). M. Stratification and weighting via the propensity score in estimation of causal treatment effects: A comparative study. Statistics in Medicine, 23(19), 2937–2960. https://doi.org/10.1002/sim.1903
- Ma, W., & Abdulai, A. (2019). IPM adoption, cooperative membership and farm economic performance: Insight from apple farmers in China. China Agricultural Economic Review, 11(2), 218–236.
- Mabe, F., Danso-Abbeam, G., & Ehiakpor, D. S. (2018). Assessment of implementation of planting for food and jobs (PFJ) programme: Lessons and ways forward. In Agriculture in Ghana today: Some critical topics for policy making, by UDS. University for Development Studies (UDS). http://hdl.handle.net/123456789/2282
- Maddala, G. S. (1986). Limited-dependent and qualitative variables in Econometrics. Cambridge University Press.
- Mensah, S. O., & Agbekpornu, H. (2015). Determinants of access to credit by Agribusiness Operators in the Kumasi Metropolis. American Journal of Experimental Agriculture, 6(5), 333–346. https://doi.org/10.9734/AJEA/2015/12477
- Ministry of Food and Agriculture (MoFA). (2017). Planting for Food and Jobs Concept-A Program to Stimulate Rapid Growth of the Ghanaian Agricultural Sector (Drafted: February 2017).
- Minten, B., & Barrett, M. (2008). Agricultural Technology, productivity, and poverty in Madagascar. World Development, 36(5), 797–822. https://doi.org/10.1016/j.worlddev.2007.05.004
- Motsoari, C., Cloete, P. C., & van Schalkwyk, H. D. (2015). An analysis of factors affecting access to credit in Lesotho’s smallholder agricultural sector. Development Southern Africa, 32(5), 592–602. https://doi.org/10.1080/0376835X.2015.1044077
- Mulungu, K., & Ng’ombe, J. (2019). Climate change impacts on Sustainable maize production in sub-Saharan Africa. A Review. https://doi.org/10.5772/intechopen.90033
- Ngwako, G., Mathenge, M., Gido, E., & Kgosikoma, K. (2021). Effect of market participation on household welfare among smallholder Goat farmers in Botswana. Journal of Agribusiness and Rural Development, 2(2), 151–160. https://doi.org/10.17306/J.JARD.2021.0t1362
- Nnadi, F. N., & Akwiwu, C. D. (2008). Determinants of Youths’ participation in rural Agriculture in Imo State, Nigeria. Journal of Applied Sciences, 8(2), 328–333. https://doi.org/10.3923/jas.2008.328.333
- Ntshangase, N. L., Muroyiwa, B., & Sibanda, M. (2018). Farmers’ perceptions and factors influencing the adoption of no-till conservation agriculture by small-scale farmers in Zashuke, KwaZulu-Natal Province. Sustainability, 10(2), 555. https://doi.org/10.3390/su10020555
- Nurideen, F. (2019). An assessment of the impact of the Planting for Food and Jobs Policy on smallholder farmers food security in the Wa West district. University of Ghana thesis.
- Nxumalo, K. K. S., & Oladele, O. I. (2013). Factors Affecting Farmers’ Participation in Agricultural Programme in Zululand District, Kwazulu Natal Province, South Africa. Journal of Social Science, 34(1), 83–88.
- Oladejo, J. A., Olawuyi, S. O., & Anjorin, T. D. (2011). Analysis of Women participation in agricultural production in Egbedore local government area of Osun State, Nigeria. International Journal of Agricultural Economics & Rural Development, 4(1), 1–11. http://www.lautechaee-edu.com/journal/ijaerd5/ijaerd5%20-%201.pdf
- Pauw, K. (2018). The state of agriculture in Ghana: Towards agricultural innovation in Ghana –an evidence-based approach. Retrieved at: https://www.poverty-action.org/sites/default/files/Pauw-agriculture-in-ghana.pdf.
- Pauw, K. (2022). A review of Ghana’s planting for food and jobs program: Implementation, impacts, benefits, and costs. Food Security, 14(5), 1321–1335. https://doi.org/10.1007/s12571-022-01287-8
- Quaye, W., Fuseini, M., Boadu, P., & Asafu-Adjaye, N. Y. (2019). Bridging the gender gap in agricultural development through gender responsive extension and rural advisory services delivery in Ghana. Journal of Gender Studies, 28(2), 185–203. https://doi.org/10.1080/09589236.2017.1419941
- Rahm, M. R., & Huffman, W. E. (1984). The adoption of reduced tillage: The role of human capital and other variables. American Journal of Agricultural Economics, 66(4), 405–413. https://doi.org/10.2307/1240918
- Richard, J. M. (2017). An Assessment of Determinants of farmers’ Choice of Dairy Goat Marketing Channels in Meru County, Kenya (No. 634-2018-5510). http://hdl.handle.net/11295/101239.
- Robins, J., Sued, M., Lei-Gomez, Q., & Rotnitzky, A. (2007). Comment: Performance of Double-robust estimators when “inverse probability” Weights are highly variable. Statistical Science, 22(4), 544e559. https://doi.org/10.1186/s40066-021-00351-4
- Sebatta, C., Mugisha, J., Katungi, E., Kashaaru, A., & Kyomugisha, H. (2014). Smallholder farmers’ decision and level of participation in the Potato market in Uganda. Modern Economy, 5(8), 895. https://doi.org/10.4236/me.2014.58082
- Sharma, N. (2016). Determining growers’ participation in contract farming in Punjab. Economic & Political Weekly, 51(2), 58–64.
- Tan, Z. (2010). Bounded, efficient and doubly robust estimation with inverse weighting. Biometrika, 97, 661–682.
- Tanko, M., Ismaila, S., Sadiq, S. A., & Aye, G. (2019). Planting for food and jobs (PFJ): A panacea for productivity and welfare of rice farmers in Northern Ghana. Cogent Economics & Finance, 7(1), 1693121. https://doi.org/10.1080/23322039.2019.1693121
- Tarawali, G., Iyangbe, C., Udensi, U. E., Ilona, P., Osun, T., Okater, C., & Asumugha, G. N. (2012). Commercial scale adoption of improved cassava varieties: A baseline study to highlight constraints of large-scale cassava-based agro-processing industries in Southern Nigeria. Journal of Food Agriculture & Environment, 10(3&4), 689–694. https://hdl.handle.net/10568/80867
- Tekuni, N., Kyere, G. H., Anang, S. A., & Mensah, M. O. (2021). Robustness of food security and policy trust on smallholder participation in Ghana’s planting for food and jobs programme. African Journal of Agricultural Research, 17(12), 1517–1523.
- Wooldridge, J. M. (2003). Econometric analysis of cross Section and Panel data. MIT Press.
- Wooldridge, J. M. (2007). Inverse probability weighted estimation for general missing data problems. Journal of Econometrics, 141(2), 1281–1301. https://doi.org/10.1920/wp.cem.2004.0504
- Yakubu, M., Gambo, A. J., Musah, W. M., Ayayi, O. J., & Usman, M. H. (2019). Factors influencing participation of cassava farmers in Survival farming Intervention programme in Kogi State, Nigeria. Journal of Agricultural Extension, 23(2), 22–33. https://doi.org/10.4314/jae.v23i2.3
- Yawson, D. O., Armah, F. A., Afrifa, E. K. A., & Dadzie, S. K. N. (2010). Ghana’s fertilize subsidy policy: Early Field Lesson from farmer in Central region. Journal Sustainable Development in Africa, 12(3), 191–203. https://jsd-africa.com/Jsda/V12No3_Summer2010_A/PDF/Ghana's%20Fertilizer%20Subsidy%20Policy,%20Early%20Field%20Lessons%20From%20Farmers%20in%20the%20Central%20Region%20(Yawson,%20Armah).pdf
- Zimmerman, R., Bruntrup, M., Kolavalli, S., & Flahertty, K. (2009). Agricultural policies in sub-Saharan Africa: Understanding CAAP and APRM policy process. Studies, No. 48, ISBN 978-3-88985-484-1, Deutsches Institut für Entwicklungspolitik (DIE), Bonn. German Development Institute.