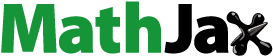
Abstract
With the increased opening of China’s dairy industry to the outside world and the cost advantages of imported dairy products, China’s dairy product import trade has grown rapidly in recent years. The rapidly changing global trading system highlights the importance of understanding the dynamics of China’s relationship with the world market as a major global importer of dairy products. In this study, on the basis of analyzing the mechanism of volatility spillover and the structure of China’s milk powder import trade, we selected Global Dairy Trade (GDT)’s milk powder as the representative of international milk powder and investigated the volatility spillover effect between GDT milk powder and China’s raw milk markets by using the Baba-Engle-Kraft-Kroner (BEKK)-generalized autoregressive conditional heteroscedasticity (GARCH) (1,1) model. The results showed that there was a bidirectional volatility spillover effect between GDT whole milk powder and China’s raw milk market; however, there was a unidirectional volatility spillover effect between the GDT skim milk powder and China’s raw milk market. China’s raw milk market had no volatility spillover effect on the GDT skim milk powder market.
1. Introduction
Agricultural commodity price volatility, a major market risk source, has become a concern for farmers (Huchet-Bourdon, Citation2011). As a perishable agricultural product, dairy farmers are more sensitive to the price changes of raw milk. In recent years, China’s raw milk prices appeared in a phenomenon of “boom and bust” cycles, which has become a prominent problem in the development of the milk industry. The factors that explain the price changes of raw milk include the cost of feed, market demand, structure of market players and government policies, etc. (Fotis & Polemis, Citation2021; Mat et al., Citation2021; Müller et al., Citation2018; Rezitis, Citation2019). One factor that cannot be ignored for China’s raw milk price is the price of international milk powder.
After the melamine incident in 2008, China’s milk powder imports increased rapidly. In 2019, China imported 1,014,843 metric tons of milk powder, up 10.05 times from 100,930 metric tons in 2008 (BAHVMARA & DAC, Citation2020; Gao, Citation2013). China’s milk powder is highly dependent on foreign trade, with net imports reaching 1,013,059 metric tons in 2019 and a trade deficit of US $ 3.12 billion (BAHVMARA & DAC, Citation2020). An important factor leading to the high imports of milk powder is low tariffs, especially since the signing of the “Free Trade Agreement between the Government of the People’s Republic of China and the Government of New Zealand” and the “Free Trade Agreement between the Government of Australia and the Government of the People’s Republic of China”, China’s tariffs on imported milk powder from New Zealand and Australia were reduced year by year until reaching zero.
Milk powder, the category with the highest proportion of imported dairy products in China, has a substitution effect on raw milk. Dairy farmers are the most vulnerable group in the industry chain and lack a stable and close interest linkage mechanism with dairy processing enterprises (Qian et al., Citation2011; Zhang, Citation2009). Thus, when the price of imported milk powder reconstituted into fluid milk is lower than that of domestic raw milk, imported milk powder has a huge competitive advantage, driven by profits, and Chinese dairy processing enterprises tend to use more imported milk powder as raw material, therefore giving up the use of domestic raw milk or lowering milk price, which has led to fluctuations in raw milk prices. At the same time, as pasteurized milk must use domestic raw milk as a source of production, the consumption structure of Chinese dairy products, which do not have pasteurized milk as a major consumption category, also facilitates the influence of international milk powder.
Due to the elevated level of openness in the Chinese dairy market, the raw milk industry in China has been exposed to the open international market before it has sufficiently matured. As a result, any change in the price of milk powder on the international market could significantly impact the volatility of raw milk prices in China. The volatility in raw milk prices will lead to instability in the incomes of dairy farmers, which will severely dampen the enthusiasm of dairy farmers in producing milk, and is not conducive to the healthy development of raw milk in China. In addition, as China is the largest importer of milk powder in the world (European Commission Milk Market Observatory, Citation2022), it is also worth paying attention to whether the volatility of the domestic raw milk price will affect the international milk powder price volatility.
Thus, this study uses the Baba-Engle-Kraft-Kroner (BEKK)-generalized autoregressive conditional heteroscedasticity (GARCH) model from an empirical perspective to explore the volatility linkage between the price returns of international milk powder and China’s raw milk. The structure of this paper is as follows: Section 2 is a literature review. Section 3 briefly explains the volatility spillover effect mechanism and China’s milk powder import trade structure. In Section 4, we introduce the theoretical model and explain the data. Section 5 presents the empirical results, and our conclusions are drawn in Section 6.
2. Literature review
World agricultural prices are becoming more volatile, which has caused great concern. In the European Union, the volatility of international agricultural commodity prices has intensified the debate regarding risk-related agricultural policies (Assefa et al., Citation2013). Agricultural commodity price volatility does not exist in isolation but is influenced by various factors. Unlike price transmission, price volatility transmission or spillover refers to the second moment relationship of variables, whereby the volatility of one market is affected by the volatility from other markets (Katusiime, Citation2019).
Research on the spillover of agricultural price volatility has focused on the food supply chains and energy-agricultural commodity markets (Assefa, et al., Citation2012). Certain studies conducted empirical research on the volatility spillover of agricultural commodities along the food supply chain. Rezitis (Citation2003) investigated the causal, price transmission, and volatility spillover effects between producer and consumer prices in the Greek lamb, beef, pork, and poultry markets by employing a GARCH approach. The empirical results showed the existence of significant price effects in the markets. Buguk et al. (Citation2003) analyzed price volatility spillover effects in the U.S. catfish supply chain. Results detected strong price volatility spillovers from feed materials to catfish feed and farm- and wholesale-level catfish prices. After that, some scholars began to build on the previous studies and to carry out deeper research. Assefa et al. (Citation2017) examined the relationship between price volatility transmission and market power in the German fresh pork supply chain. The results showed that retail market power limits the transmission of price and price volatility. Wan et al. (Citation2022) focused on the asymmetric transmission of price volatility in the agricultural supply chain and found the existence of asymmetric volatility transmission by applying it to the Chinese pork market.
Other studies examined the price volatility spillover of energy-agricultural commodities. For example, some scholars’ studies have shown the spillover effects of crude oil price fluctuations on the volatility of agricultural markets such as corn (Nazlioglu et al., Citation2012; Wu et al., Citation2011). Lu et al. (Citation2019) showed a bidirectional volatility spillover effect between crude oil and agricultural markets during the 2008–09 crisis, but after that, the integration between crude oil and agricultural markets has decreased. Moreover, in addition to the study of the spillover effect of crude oil on agricultural market volatility, many scholars began to focus on ethanol energy and added it to the study of the impact on agricultural price volatility (Gardebroek & Hernandez, Citation2013; Zhang et al., Citation2009).
Raw milk is a particularly perishable agricultural commodity, so its price volatility is more likely to attract great concern. Unlike the United States with a systematic and complete pricing system (Bolotova, Citation2017), China’s raw milk is basically market-oriented, and, in recent years, the price volatility has become more severe (Yu et al., Citation2014). Existing research on raw milk price volatility in China has mainly focused on exploring the characteristics of price volatility and verifying this through various empirical methods. Yu et al. (Citation2018) and Yu et al. (Citation2014) used Census X12 seasonal adjustment and the H-P filter method to analyze the characteristics of the volatility cycle of raw milk prices in China. Kang et al. (Citation2016) used GARCH type models to analyze the price volatility clustering, high-risk and high-returns, and information asymmetry for raw milk in China.
However, most of the research by scholars on the factors that influence the volatility of raw milk prices in China is theoretical analysis. Li et al. (Citation2010) proposed that the factors affecting the price volatility of raw milk and dairy products in China included the international dairy market price factor, the contradiction between the seasonal supply and demand factor, the feed price factor, the dairy processing enterprises market behavior factor, the industry risk factor, etc. They also proposed that the most crucial factors affecting the raw milk and dairy products market in China are the international dairy market price and the contradiction between the supply and demand of raw milk. Han and Liu (Citation2019) indicated that imported dairy products in international trade, especially milk powder, are used in the production of dairy products, such as liquid milk, replacing the supply of domestic raw milk. In addition, scholars have tested the impact of international milk powder on the domestic raw milk market through empirical analysis. Hu and Dong (Citation2016) used a general equilibrium model to simulate the impact of relative price changes of international milk powder on the domestic raw milk market, and found that a decline in the relative price of imported milk powder caused a decrease in raw milk production and a reduction in related employees. In the context of import growth, the consensus has been reached on the impact of international milk powder on China’s raw milk market. Few studies have focused on the volatility spillover effect of the international milk powder and domestic raw milk markets from an empirical perspective, especially the direction of the volatility spillover effect of different categories of international milk powder and China’s raw milk markets. Therefore, the research on this topic has extremely important theoretical and practical significance.
3. Volatility spillover effect mechanism and China’s milk powder import trade structure
3.1. Volatility spillover effect mechanism
The mechanism of the volatility spillover effect between international milk powder and China’s raw milk markets is as follows (Figure ):
Figure 1. The volatility spillover effect mechanism between international milk powder and China’s raw milk markets.
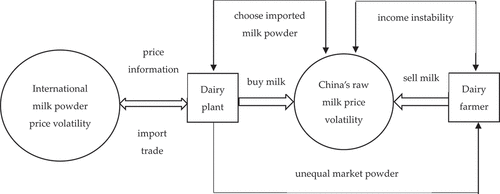
When prices at home and abroad hang upside down, price information will be directly transmitted to dairy plants, stimulating the enterprises to switch to milk powder imports; dairy plants have chosen to use imported milk powder for production due to the advantage of lower international milk powder costs, reducing the demand for domestic raw milk and directly contributing to its price volatility.
Due to the imperfect linking mechanism and unequal market power between dairy plants and dairy farmers, dairy farmers lack the negotiating power to price raw milk (Ma et al., Citation2018). If the steady income of dairy farmers is not guaranteed, culling or even withdrawal from the breeding industry will occur, which will have a certain impact on the volatility of raw milk prices in the country.
Conversely, due to cost reasons, Chinese dairy plants choose international milk powder to replace domestic raw milk, and a large number of imports may affect the price changes of international milk powder through supply and demand factors.
Therefore, the volatility spillover effect of international milk powder and China’s raw milk markets is influenced by dairy plants as the main body for price information and import trade channels.
3.2. China’s milk powder import trade structure
From the above analysis, we can see that price information and import trade are the main methods for volatility spillover at home and abroad, so it is necessary to briefly analyze the import structure of China’s milk powder, so as to determine the main sources of milk powder price information and the import countries that primarily affect China’s raw milk market. According to the China customs HS code and classification standard, imported milk powder includes three categories: HS04021000 (solid milk and cream, fat content ≤ 1.5%), HS04022100 (unsweetened solid milk and cream, fat content > 1.5%), and HS04022900 (sweetened solid milk and cream, fat content > 1.5%). Among them, HS04021000 is called skim milk powder (SMP), and HS04022100 and HS04022900 are called whole milk powder (WMP).
First, in Figure , the overall import of milk powder in China shows an increasing trend from 2011 to 2019. In particular, imports surged in 2014 as imported milk powder prices dropped rapidly. The high volume of imported milk powder led to excess stocks, triggering a drop in imports in 2015, then gradually increasing again. In terms of the category structure of imported milk powder, China’s milk powder imports are dominated by whole milk powder, accounting for more than 60%. As a result, whole milk powder is the type with the largest import demand in China’s milk powder import trade, which could have a huge impact on the country’s raw milk industry.
Figure 2. China’s imports of whole milk powder (WMP) and skim milk powder (SMP) and the proportion of WMP. Data source: (DRC, 2022).
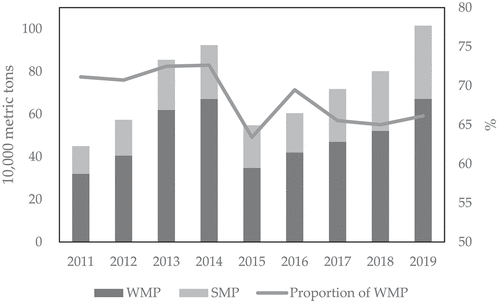
Secondly, from 2011 to 2019, New Zealand, Australia, and the United States were the top three countries of origin for milk powder imported into China (Figure ). New Zealand was the largest source country, accounting for more than 70% of the total milk powder imported over the years. From the perspective of a category (Figure ), China’s skim milk powder imports were mainly from New Zealand, but the United States, Australia, and other countries were also important source countries. However, among the importers of whole milk powder, New Zealand accounted for more than 90% in most years, and even reached 96.19% in 2012, which was an absolute monopoly. As a result, New Zealand milk powder, especially whole milk powder, has developed an inseparable relationship with the Chinese market.
Figure 3. Proportion of imports of imported milk powder from major source countries. Data source: (DRC, Citation2022).
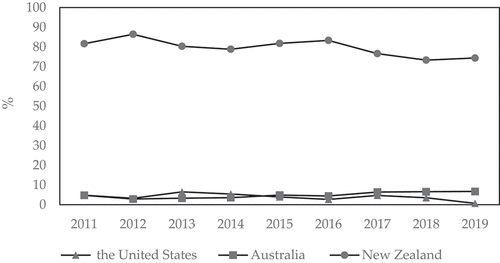
Figure 4. Proportion of imports of imported WMP (left) and SMP (right) from major source countries. Data source: (DRC, Citation2022).
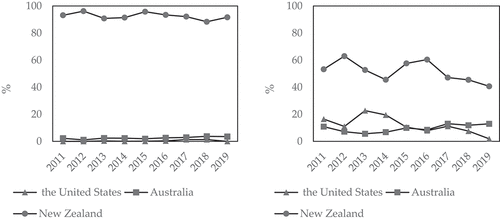
4. Methodology and hypothesis formulation
4.1. Model specification
The autoregressive conditional heteroscedasticity (ARCH) model (Engle, Citation1982) and the generalized autoregressive conditional heteroscedasticity (GARCH) model (Bollerslev, Citation1986) have become effective tools for investigating the volatility of the return on prices, especially financial assets. Researchers typically use multivariate GARCH models to investigate the volatility’s interdependence across multiple markets. Among them, the BEKK-GARCH model is one of the most popular models for measuring volatility spillovers. Hence, we employ the BEKK-GARCH model Engle and Kroner (Citation1995) presented in this study. In terms of the selection of the conditional variance lag order in the GARCH model, many scholars choose GARCH (1,1), which can be considered sufficient to describe the situation of most of the time series data in reality (Apergis & Rezitis, Citation2003; Hansen & Lunde, Citation2005). Therefore, this study utilizes the bivariate BEKK-GARCH (1,1) model to explore the volatility spillover effect of international milk powder and China’s raw milk markets.
The first step for this model is to determine the mean equations:
EquationEquation (1)(1)
(1) is known as the mean equation, where
denotes the explanatory variable vector;
denotes the coefficient vector, and
is the residual vector where the mean is zero and the variance is
. The purpose of the mean equation is to remove any linear dependence and, therefore, to produce uncorrelated residuals. If there is a dynamic relationship between the series data, researchers generally use the Vector Autoregression (VAR) model or Vector Error Correction (VEC) model for the mean equation. Otherwise, we can build the Autoregression (AR), Moving Average (MA), or Autoregressive and Moving Average (ARMA) model to remove linear dependence. In addition, for most asset return series, the serial correlations are weak, if any. Thus, building a mean equation amounts to removing the sample mean from the data if the sample mean is significantly different from zero (Tsay, Citation2010).
EquationEquation (2)(2)
(2) is the BEKK parameterization of the bivariate GARCH (1,1), which is also the focus of this study. C is a lower triangular constant matrix, A and B are 2 × 2 parameter matrix, which is related to the ARCH effect and GARCH effect, respectively. In order to clearly see the matrix elements of the variance equation, EquationEquation (2)
(2)
(2) can be written as:
In EquationEquation (3)(3)
(3) , we assumed that there are market 1 and market 2. h11,t and h22,t denote the conditional variances of market 1 and market 2; h12,t and h21,t denote the conditional covariances of market 1 and market 2; a12 and b12 reveal how the past squared error and the conditional variance of market 1 affect the conditional variance of market 2, also known as volatility spillover. Hence, examining the volatility spillover effect from market 1 to market 2 is equivalent to examining whether a12 and b12 are significantly zero. Similarly, the volatility spillover effect of market 2 to market 1 can also be tested (Li, Citation2015; Mademlis & Dritsakis, Citation2018).
In summary, the volatility spillover effect between market 1 and market 2 sets the following null hypothesis:
Null hypothesis 1:
H0: a12 = b12 = 0, which states that there is no volatility spillover effect from market 1 to market 2.
Null hypothesis 2:
H0: a21 = b21 = 0, which states that there is no volatility spillover effect from market 2 to market 1.
Null hypothesis 3:
H0: a12 = b12 = a21 = b21 = 0, which states that there is no volatility spillover effect between market 1 and market 2.
Taking the null hypothesis 1 as an example, if at least one of the coefficients and
rejects the null hypothesis at the significance level, this indicates the existence of a volatility spillover effect from market 1 to market 2. Similarly, for the null hypothesis 2 and the null hypothesis 3, the conclusions can be drawn in the same way.
The parameters of the BEKK-GARCH model are estimated by maximizing the Gaussian log-likelihood function:
where denotes the parameters to be estimated, N denotes the number of series, and T denotes the total number of samples.
4.2. Data
Our study examined the volatility spillover effects between international milk powder and China’s raw milk market; thus, we first determined which type of price can represent the international milk powder market. This study used the Global Dairy Trade (GDT) milk powder price to represent the international price of milk powder and analyzed its volatility spillover effect with China’s raw milk market.
Global Dairy Trade (GDT) is owned by Fonterra Cooperative Group and has focused on creating a global online market for dairy. Since 2008, GDT Events has provided trusted, credible market-based reference prices for globally traded core dairy ingredients. GDT Events is acknowledged and trusted as providing credible market-based reference prices for globally traded dairy ingredients, and GDT Events auction service is the world’s pre-eminent price discovery platform for globally traded dairy products, bringing together buyers and sellers of dairy ingredients from 70 countries to trade US$2–3 billion annually (Global Dairy Trade, Citation2022). Arguably, the GDT event price has become one of the most important indicators of the price of dairy products traded globally. Based on the global influence of GDT event prices and New Zealand’s status as the absolute leading source of milk powder imports for China, it is reasonable and feasible to choose the weighted average price of all contracts of GDT milk powder as a representative of the international milk powder price.
From the above analysis, it can be seen that whole milk powder, the main imported milk powder category in China, is likely to have the greatest impact on China’s raw milk market. In this study, the price of GDT whole milk powder was selected as the main research object to analyze its volatility spillover effect on China’s raw milk market. Although the volume of imports is not as large as that of whole milk powder, skim milk powder is an important part of imported milk powder. For the purpose of comparative analysis, the prices of GDT skim milk powder and China’s raw milk were also included in the analysis framework of this study.
This paper uses a time series approach to analyze the relationship between GDT milk powder prices and domestic raw milk prices using bi-weekly data from January 2011 to December 2019. The GDT milk powder prices were obtained from the Agriculture and Horticulture Development Board (AHDB) (Agriculture and Horticulture Development Board AHDB, Citation2022) and Global Dairy Trade (GDT) (Global Dairy Trade, Citation2022), the unit was converted into RMB through the exchange rate. The prices of raw milk in China were based on the weekly fixed-point monitoring data from the markets of 10 major raw milk-producing provinces by the Ministry of Agriculture. The data were from the Bric Agricultural Data (Bric Agricultural Data BAD, Citation2022) and the Ministry of Agriculture and Rural Affairs (MARA, Citation2022). It should be noted that the GDT data is a weighted average from all contracts. Since raw milk in China is weekly data, while the GDT milk powder is bi-weekly data, we screened and matched the price data of raw milk in China. The raw milk data after the screening was essentially the same or one-day different from the GDT milk powder in terms of the date, which can be used as the bi-weekly price data for raw milk in China corresponding to the GDT milk powder price.
In the study of price volatility, the price returns of a time series are usually considered due to the good statistical characteristics rather than the price series in levels (O’Connor et al., Citation2009). The price returns can be obtained by converting the series into logarithmic forms and then comparing the current and previous data.Footnote1 The formula is stated as follows:
where refers to the price return,
is the price at time t, and
is the price at time t-1. In this study,
,
and
are used to represent the price returns of GDT whole milk powder, GDT skim milk powder, and China’s raw milk, respectively.
4.3. Hypothesis formulation
Based on the above studies, we set the following hypotheses for the variance—covariance equation:
H1.
H0: a12=b12=0, no volatility spillover effect from GDT milk powder market to China’s raw milk market.
H2.
H0: a21=b21=0, no volatility spillover effect from China’s raw milk market to GDT milk powder market.
H3.
H0: a12=b12=a21=b21=0, no volatility spillover effect between the two markets.
Therefore, for the next study, we need to identify whether these coefficients are zero significantly.
5. Empirical analysis and results
5.1. Preliminary test
This section is devoted to demonstrating the mean equation and testing whether the price returns are suitable for the analysis of this model. First, we examined the stationarity of price returns by exploiting the Augmented Dickey and Fuller (ADF) unit root test (Dickey & Fuller, Citation1979, Citation1981). Table shows that the null hypothesis of the ADF test was rejected, indicating that all price returns have no unit root, that is, all the return series are stationary.
Table 1. The augmented Dickey and Fuller (ADF) unit root test
As the time-series variables are all stationary series, it is suitable to directly create a VAR model for the mean equation. Therefore, we separately constructed the VAR models of the GDT whole milk powder return and China’s raw milk return, and GDT skim milk powder return and China’s raw milk return. For and
, the VAR(3) model with a lag length of 3 was determined according to the Akaike Information Criterion (AIC). Similarly, for
and
, a VAR(2) model with a lag length of 2 was constructed. On the basis of this setting, the Granger causality test was performed on the variables, and the results showed that evidently the bidirectional Granger Causality did not exist between the GDT whole milk powder return and China’s raw milk return, nor between the GDT skim milk powder return and China’s raw milk return (Table ).
Table 2. Results of Granger causality test
By observing the Ljung—Box statistics Q of the return series (Table ), each variable had significant serial correlation. Therefore, it is necessary to construct AR, MA, or ARMA models for each variable to remove its linear dependence. After several trials for ,
, and
, we built ARMA(2,2), AR(1), and AR(2) models to eliminate the autocorrelation of residuals, respectively. Then, the residual graphs were extracted separately. As can be seen from Figure , the residual series of the three models all presented a “volatility clustering” phenomenon, that is, a large fluctuation tends to be followed by another large fluctuation, indicating the possible presence of conditional heteroscedasticity in the residual term.
Figure 5. Residuals of the mean equation for global dairy trade(GDT) WMP (left), GDT SMP (middle), and China’s raw milk (right).
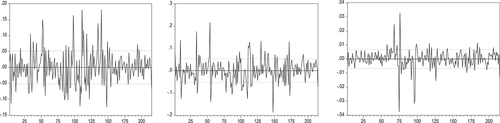
Table 3. The Q statistics of price returns
To further clarify whether there are ARCH effects in the residual series of the three mean equations, we applied the ARCH Lagrange Multiplier (LM) test to obtain the results of each equation using one and two lag (Table ). The results show that, for , the values of F-statistic and R-squared were significant at the 5% level, and for
and
, the values of F-statistic and R-squared were significant at the 1% level, so we are able to reject the null hypothesis, indicating that the ARCH effects exist in these series. Therefore, the volatility spillover effect between markets can be further analyzed.
Table 4. The autoregressive conditional heteroscedasticity (ARCH) Lagrange Multiplier (LM) test results
5.2. Empirical results
We used WinRATS 10.0 software to estimate the parameters of the BEKK-GARCH (1,1) model, and Table reports the estimation results. The results show that the coefficient of the conditional mean equation was very significant at the 5% level. On the one hand, this demonstrates that the price returns of GDT whole milk powder, GDT skim milk powder, and China’s raw milk were greatly affected by their own lag periods, on the other hand, this indicates that the setting of the mean equation forms was reasonable, and the correct conditional mean equation setting guarantees the validity of the conditional variance equation estimation.
Table 5. The estimation results of the BEKK-GARCH (1,1) model
Estimating the result of the variance-covariance equation is a key part of this study, as this can reflect the spillover effects of volatility between different markets. The detailed conclusions of the analysis are as follows:
For the GDT whole milk powder and China’s raw milk markets, the coefficients a11, a22, b11, and b22 were significantly different from zero, indicating that both the GDT whole milk powder market and China’s raw milk market were all related to the ARCH effect and GARCH effect, that is, both markets had characteristics of time-varying variance and the persistence of volatility. From the estimated results of GDT skim milk powder return and China’s raw milk return, the coefficients a11 and a22 were significantly different from zero, indicating that the GDT skim milk powder market was affected by its own past shocks and the volatility was persistent. b22 was statistically significant, which implies that the past volatility of China’s raw milk market affected the conditional variance.
For the GDT whole milk powder and China’s raw milk markets, the estimated coefficient a12 was significantly different from zero at the 1% level, indicating that the GDT whole milk powder market had an ARCH-type volatility spillover effect on China’s raw milk market, that is, the past shocks of the GDT whole milk powder market had a significant impact on the conditional variance of the raw milk market in China. The coefficient b21 was significantly different from zero at the 1% level, which means that China’s raw milk market had a GARCH-type volatility spillover effect on the GDT whole milk powder market, that is, the past conditional variance of China’s raw milk market had a significant impact on the current conditional variance of the GDT whole milk powder market. These analyses indicate that not only did the GDT whole milk powder market have a price volatility spillover effect on China’s raw milk market, but with the deepening of China’s participation in international dairy trade in recent years, China’s raw milk market also has a price volatility spillover effect on the GDT whole milk powder market. Therefore, we can conclude that there was a bidirectional volatility spillover effect between the GDT whole milk powder and China’s raw milk markets.
For the GDT skim milk powder and China’s raw milk markets, only the coefficient b12 was different from zero at the 10% level, indicating that the conditional variance of the GDT skim milk powder market affected the conditional variance of China’s raw milk market. However, the estimated coefficients a21 and b21 were not significant, showing that China’s raw milk market had no volatility spillover effect on the GDT skim milk powder market. Therefore, there was only a unidirectional volatility spillover effect between the GDT skim milk powder and China’s raw milk markets.
In addition, the Wald restriction test was used to further verify the three hypotheses of the volatility spillover effect. Specifically, for the GDT whole milk powder and China’s raw milk markets, three chi-squared statistics were all significant; therefore, all the null hypotheses can be rejected, which further confirms the existence of the bidirectional volatility spillover effect between the two markets. For the GDT skim milk powder and China’s raw milk markets, the chi-squared statistic value of the null hypothesis H2 was small and cannot be rejected, indicating that there was no volatility spillover effect from China’s raw milk market to the GDT skim milk powder market. However, the null hypotheses of both H1 and H3 were rejected, confirming the unidirectional volatility spillover effect of the two markets.
Finally, we conducted a multivariate ARCH effect test on the model and tested the standardized residuals of the model lagged at order 2 and order 5, respectively, and the results showed that the p-value could not reject the null hypothesis, indicating that the null hypothesis of “no ARCH effect” could not be rejected. Therefore, the BEKK-GARCH (1,1) model can effectively estimate the spillover effects of price volatility between markets.
6. Conclusions and discussion
The development of the raw milk industry in China is currently facing some challenges. Among them, the highly open foreign trade of Chinese dairy products and the rapid expansion of the import of international dairy products, especially milk powder, have put pressure on the domestic raw milk market to a certain extent. In addition, the Chinese government has set a target of maintaining milk self-sufficiency at 70%, meaning dairy consumption is mainly met by domestic raw milk supplies rather than imported powdered milk. As a result, the impact of international milk powder on the domestic raw milk market has caused great concern. Therefore, this study attempted to empirically answer the questions of whether there was a volatility spillover effect between international milk powder and China’s raw milk markets (that is, whether the volatility of international milk powder can be transmitted to the volatility of China’s raw milk; and whether the volatility of the China’s raw milk was transmitted to the volatility of international milk powder). On the basis of analyzing the mechanism of volatility spillover and the structure of China’s milk powder import trade, we selected GDT milk powder as the representative of international milk powder, and used the BEKK-GARCH (1,1) model to analyze the volatility spillover effect of the two markets.
The empirical results showed that a bidirectional volatility spillover effect between GDT whole milk powder and China’s raw milk market. Specifically, the past shocks of the GDT whole milk powder market had a significant impact on the conditional variance of China’s raw milk market; the past conditional variance of China’s raw milk market significantly impacted the current conditional variance of the GDT whole milk powder market. There was a unidirectional volatility spillover effect between the GDT skim milk powder and China’s raw milk markets. Specifically, the conditional variance of the GDT skim milk powder market affected the conditional variance of China’s raw milk market, but China’s raw milk market had no volatility spillover effect on the GDT skim milk powder market. In short, for both whole milk powder and skim milk powder, there was a volatility spillover effect from the GDT milk powder market to China’s raw milk market; however, the volatility spillover effect from China’s raw milk market was only to the GDT whole milk powder market.
The results of the study provide recommendations for Chinese policymakers. First, to better promote the sustainable development of the domestic raw milk industry, the government should monitor the uncertainties of the international milk powder market to identify the risks of increased volatility in the domestic raw milk market. The Chinese government could establish an early warning system for industrial security, by building a price data monitoring system, the government can publish timely and prompt price information on relevant domestic and international markets, provide price information services to farmers and enterprises, and help producers better capture market information for production. Second, the Chinese government should also make a contingency plan to deal with the sudden and drastic changes in international milk powder, so as to ensure the stable development of the domestic raw milk market, such as when a sharp drop in international milk powder prices will lead to volatility in domestic raw milk prices. In order to protect the interests of dairy farmers and stabilize the market, a dynamic milk powder reserve mechanism could be established in an appropriate time frame. In addition, the results of this study indicate that the Chinese market has a significant impact on the international milk powder market, indicating that China is occupying an increasingly important role in the international market for dairy products. Therefore, investors in the global dairy industry should pay close attention to the changes in the raw milk market in China.
In this study, since most of China’s milk powder is imported from New Zealand, we chose GDT milk powder as the representative of international milk powder. Future research should broaden the research objects, such as the price of milk powder (especially skim milk powder in the United States and Australia) as the research object, to further study the volatility spillover effect of each market and China’s raw milk market. In addition, future studies should also investigate the asymmetric volatility.
Author contributions
Conceptualization, Q.W., W.X.; Data Curation, Q. W., R.C.; Methodology, Q. W., W.X.; Formal Analysis, Q. W.; Writing, Q.W., R.C., W.X.
Acknowledgments
Zhejiang University and Zhejiang Sci-Tech University.
Disclosure statement
No potential conflict of interest was reported by the author(s).
Additional information
Funding
Notes on contributors
Qianqian Wang
Qianqian Wang is a lecturer at Zhejiang Sci-Tech University with research interests in agricultural market and agricultural policy.
Rongzhu Cheng
Rongzhu Cheng is a Distinguished Associate Professor at Sichuan Agricultural University with research interests in agricultural policy, agricultural trade and food value chain.
Wangfang Xu
Wangfang Xu is a PhD candidate in Zhejiang University. Her research interest includes agricultural trade and digital economy.
Notes
1. Unlike the infrequent trading phenomenon that may exist in the stock market, prices in the Chinese raw milk market are based on data from the Ministry of Agriculture’s spot monitoring of 500 county fair markets across the country, and trading volumes are generally stable. Similarly, GDT Events is the leading global auction for trading large volume dairy ingredients and reference price discovery. GDT operate on a worldwide scale with buyer/seller customers and stakeholders spread across more than 70 countries with different interests, languages, cultures, and time zones, trading USD 2–3 billion annually. It can be assumed that trading infrequency phenomenon is largely non-existent in both markets. For the Infrequent trading phenomenon see Fotis and Polemis (Citation2011, Citation2012).
References
- Agriculture and Horticulture Development Board (AHDB). Available online. Retrieved April 14, 2022) https://ahdb.org.uk/
- Apergis, N., & Rezitis, A. (2003). Agricultural price volatility spillover effects: The case of Greece. European Review of Agriculture Economics, 30(3), 389–15. https://doi.org/10.1093/erae/30.3.389
- Assefa, T. T., Meuwissen, M., Gardebroek, C., & Lansink, A. (2017). Price and volatility transmission and market power in the German fresh pork supply chain. Journal of Agricultural Economics, 68(3), 861–880. https://doi.org/10.1111/1477-9552.12220
- Assefa, T. T., Meuwissen, M. P. M., & Oude Lansink, A. G. J. M. (2013). Literature Review on price volatility transmission in food supply chains, the role of contextual factors, and the CAP’s market measures, Working Paper No. 4, ULYSSES project, EU 7th Framework Programme, Project 312182 KBBE.2012.1.4-05.
- Bollerslev, T. (1986). Generalized autoregressive conditional heteroskedasticity. Journal of Econometrics, 31(3), 307–327. https://doi.org/10.1016/0304-4076(86)90063-1
- Bolotova, Y. V. (2017). An analysis of milk pricing in the United States dairy industry. Agribusiness, 33(2), 194–208. https://doi.org/10.1002/agr.21456
- Bric Agricultural Data (BAD). Available online Retrieved May 14, 2022. http://www.agdata.cn/
- Buguk, C., Hudson, D., & Hanson, D. (2003). Price volatility spillover in agricultural markets: An examination of U.S. catfish markets. Journal of Agricultural and Resource Economics, 28(1), 86–99. https://doi.org/10.22004/ag.econ.30716
- Bureau of Animal Husbandry and Veterinary of Ministry of Agriculture and Rural Affairs (BAHVMARA); Dairy Association of China (DAC). (2020). China dairy Statistical Summary.
- Development Research Center of the State Council (DRC). International trade research and decision support System. Available online Retrieved April 20, 2022. http://trade.drcnet.com.cn/
- Dickey, D. A., & Fuller, W. A. (1979). Distribution of the estimators for autoregressive time series with a unit root. Journal of the American Statistical Association, 74(366a), 427–431. https://doi.org/10.1080/01621459.1979.10482531
- Dickey, D. A., & Fuller, W. A. (1981). Likelihood ratio statistics for autoregressive time series with a unit root. Econometrica, 49(4), 1057–1072. https://doi.org/10.2307/1912517
- Engle, R. F. (1982). Autoregressive conditional heteroscedasticity with estimates of the variance of United Kingdom inflation. Econometrica, 50(4), 987–1007. https://doi.org/10.2307/1912773
- Engle, R. F., & Kroner, K. (1995). Multivariate simultaneous generalized ARCH. Econometric Theory, 11(1), 122–150. https://doi.org/10.1017/S0266466600009063
- European Commission Milk Market Observatory. (2022). Available online. Retrieved Octocter 4, 2022. https://ec.europa.eu/info/sites/info/files/food-farming-fisheries/farming/documents/milk-trade-main-competitors_en.pdf
- Fotis, P., & Polemis, M. (2011). The use of economic tools in merger analysis: Lessons from US and EU experience. European Competition Journal, 7(2), 323–347. https://doi.org/10.5235/174410511797248333
- Fotis, P., & Polemis, M. (2012). The short-run competitive effects of merger enforcement. European Competition Journal, 8(1), 183–210. https://doi.org/10.5235/174410512800370025
- Fotis, P., & Polemis, M. (2021). The impact of regulation in shelf life on milk price. International Journal of the Economics of Business, 28(1), 19–47. https://doi.org/10.1080/13571516.2020.1819084
- Gao, H. B. (2013). China dairy yearbook 2012. China Agriculture Press.
- Gardebroek, C., & Hernandez, M. A. (2013). Do energy prices stimulate food price volatility? Examining volatility transmission between US oil, ethanol and corn markets. Energy Economics, 40, 119–129. https://doi.org/10.1016/j.eneco.2013.06.013
- Global Dairy Trade. Available online. Retrieved May 14, 2022. https://www.globaldairytrade.info/
- Han, L., & Liu, C. (2019). China’s dairy economic development trends, challenges and policy suggestions. Chinese Journal of Animal Science, 55(1), 151–156. https://doi.org/10.19556/j.0258-7033.2019-01-151
- Hansen, P. R., & Lunde, A. (2005). A forecast comparison of volatility models: Does anything beat a GARCH (1, 1)? Journal of Applied Econometrics, 20(7), 873–889. https://doi.org/10.1002/jae.800
- Huchet-Bourdon, M. (2011). Agricultural Commodity Price Volatility: An Overview. In OECD food, agriculture and fisheries papers, no. 52. OECD Publishing. https://doi.org/10.1787/5kg0t00nrthc-en
- Hu, B., & Dong, X. (2016). Study on the impacts of dairy import and the strategy choice for dairy safety: Trade condition of China agricultural safety net. Issues in Agricultural Economy, 1(37), 84–94+112. https://doi.org/10.13246/j.cnki.iae.2016.01.011
- Kang, H., Han, X., Liu, F., & He, Z. (2016). Analysis on price fluctuation of fresh milk in China: Based on GARCH-type model. Chinese Journal of Animal Science, 52(10), 9–13. https://doi.org/10.3969/j.issn.0258-7033.2016.10.002
- Katusiime, L. (2019). Investigating spillover effects between foreign exchange rate volatility and commodity price volatility in Uganda. Economies, 7(1), 1. https://doi.org/10.3390/economies7010001
- Li, S. (2015). Volatility spillovers in the CSI300 futures and spot markets in China: Empirical study based on discrete wavelet transform and VAR-BEKK-bivariate GARCH model. Procedia Computer Science, 55, 380–387. https://doi.org/10.1016/j.procs.2015.07.085
- Li, S., Zhou, X., & Cao, Z. (2010). Analysis on the price volatility of raw milk and dairy products in China. Chinese Journal of Animal Science, 46(4), 37–42.
- Lu, Y., Yang, L., & Liu, L. (2019). Volatility spillovers between crude oil and agricultural commodity markets since the financial crisis. Sustainability, 11(2), 396. https://doi.org/10.3390/su11020396
- Mademlis, D. K., & Dritsakis, N. (2018). Volatility between oil prices and stock returns of dow jones index: A bivariate GARCH (BEKK) approach. In N. Tsounis & A. Vlachvei (Eds.), ICOAE 2018, SPBE (pp. 209–221). Springer. https://doi.org/10.1007/978-3-030-02194-8_16
- Ma, Y., He, S., & Gao, Y. (2018). Dairy enterprises or dairy farmers, which should be centered on? Improving vertical integration policy of China’s dairy industry chain based on GHM mode. Journal of Nanjing Agricultural University(Social Sciences Edition), 18(6), 146–156+162. https://doi.org/10.19714/j.cnki.1671-7465.2018.0032
- Mat, B., Arikan, M. S., Çevrimli, M. B., Akin, A. C., & Tekindal, M. A. (2021). Factors affecting the price of raw milk in Turkey using panel data analysis. Ciência Rural, 51(11), 1–10. https://doi.org/10.1590/0103-8478cr20200937
- Ministry of Agriculture and Rural Affairs (MARA). Retrieved May 14, 2022. http://english.moa.gov.cn/
- Müller, M., Hanisch, M., Malvido, A., Rommel, J., & Sagebiel, J. (2018). The structural effect of cooperatives on price volatility in the European dairy sector. Applied Economics Letters, 25(8), 576–579. https://doi.org/10.1080/13504851.2017.1346358
- Nazlioglu, S., Erdem, C., & Soytas, U. (2012). Volatility spillover between oil and agricultural commodity markets. Energy Economics, 36, 658–665. https://doi.org/10.1016/j.eneco.2012.11.009
- O’Connor, D., Keane, M., & Barnes, E. (2009). Measuring volatility in dairy commodity prices. In Proceedings of the 113th EAAE Seminar, Chania, Crete, Greece.
- Qian, G., Guo, X., Guo, J., & Wu, J. (2011). China’s dairy crisis: Impacts, causes and policy implications for a sustainable dairy industry. International Journal of Sustainable Development & World Ecology, 18(5) , 434–441. https://doi.org/10.1080/13504509.2011.581710
- Rezitis, A. (2003). Mean and volatility spillover effects in Greek producer-consumer meat prices. Applied Economics Letters, 10(6), 381–384. https://doi.org/10.1080/1350485032000081299
- Rezitis, A. N. (2019). Investigating price transmission in the Finnish dairy sector: An asymmetric NARDL approach. Empirical Economics, 57(3), 861–900. https://doi.org/10.1007/s00181-018-1482-z
- Tsay, R. S. (2010). Analysis of financial time series (3rd ed.). John Wiley & Sons, Inc. https://doi.org/10.1002/9780470644560
- Wan, X., Li, C., & Yang, Z. (2022). Asymmetric price volatility transmission in agricultural supply chains: Evidence from the Chinese pork market. Mathematical Problems in Engineering, 2022, 1–11. https://doi.org/10.1155/2022/4801898
- Wu, F., Guan, Z., & Myers, R. J. (2011). Volatility spillover effects and cross hedging in corn and crude oil Futures. Journal of Futures Markets, 31(11), 1052–1075. https://doi.org/10.1002/fut.20499
- Yu, J., Han, X., Liu, F., & He, Z. (2014). Analysis on price volatility cycle of Chinese raw milk based on X12 seasonal adjustment method and H-P filter model. China Dairy Cattle, (18), 30–33. https://doi.org/10.3969/j.issn.1004-4264.2014.18.009
- Yu, H., Wu, J., & Zhang, R. (2018). Study on raw milk price fluctuation, dumping milk and regulatory policies. Journal of Huazhong Agricultural University (Social Sciences Edition), (1), 65–72+159. https://doi.org/10.13300/j.cnki.hnwkxb.2018.01.008
- Zhang, L. (2009). Interest linking mechanism: The crux and outlet of China’s dairy industry development. China Dairy Cattle, (11), 7–12. https://doi.org/10.3969/j.issn.1004-4264.2009.11.004
- Zhang, Z., Lohr, L., Escalante, C., & Wetzstein, M. (2009). Ethanol, corn, and soybean price relations in a volatile vehicle-fuels market. Energies, 2(2), 320–339. https://doi.org/10.3390/en20200320