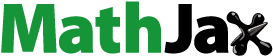
Abstract
The rapid spread of mobile phones holds the promise of transforming traditional extension into a digital extension service. To develop such a system, understanding the farmers’ service demand is a prerequisite issue. Identifying farmers’ willingness to pay (WTP) for mobile-based extension service has paramount importance to service system design and financial sustainability. The objective of this study was to evaluate farmers’ willingness to pay for agricultural extension services by using the Contingent Valuation method (CVM). A multistage sampling technique was used to select 280 households. The Tobit model was used to identify factors affecting farmers’ willingness to pay for agricultural extension service. Results reveal that 81.07 percent of the respondents are generally willing to pay for mobile phone-based extension services. On average, respondents were willing to pay for the service at 101.38 ETB annually. The results of the Tobit model indicate that the educational status of farmers' ownership of the mobile phone, information-seeking behavior of farmers, distance from home to the nearest town and access to electric power influence the willingness to pay for the service. The outcomes of the research would support policymakers to implement better design for the dissemination of mobile phone-based agricultural information to transform marginalized farmers in Ethiopia. To enhance willingness to pay, there should be a training package to farmers about mobile-based extension service through adult education and public media.
1. Introduction
The global population is estimated to become around 10 billion by 2050, and feeding it is a global challenge. Therefore, the current food production should be increase by at least 59-98.0 percent (Development, U. N., & ProgrammeUNDP, Citation2021). The requirement is very challenging, especially for sub-Saharan Africa (Abbott et al., Citation2021), particularly for the smallholder farmers producing 80.0% of its food for consumption (GIZ, Citation2021). Smallholder farmers are typically more challenged due to low access to information, technologies and low levels of skills and knowledge (Rajkhowa et al., Citation2021). These multifaceted challenges are primarily due to limited access to extension services for land and environmental management, production and marketing (Mapiye et al., Citation2021).
Agricultural extension is the basic mechanism for enhancing agricultural productivity, rural development, poverty reduction, and food security (Chayot, Citation2022; Davis et al., Citation2020), and it is also understood as a policy and legitimate instrument to bring about desired changes in socio-economic and environmental aspects (Gerba et al., Citation2017). The public-based agricultural extension system in most developing countries is primarily responsible for the dissemination of agricultural information and innovation. The system is operated by large staff of extension personnel with direct contact to geographically dispersed farmers. However, such a system is characterized by financial unsustainability due to high cost of extension personnel, supply driven, top-down approach, limited geographical coverage, limited-service outreach and not demand driven without the participation of the farmers (Atiso et al., Citation2021; Rajkhowa et al., Citation2021). Therefore, changing the traditional public-based agricultural extension system to modern digital-based agricultural extension enables us to transform the marginalized smallholder farmers (Mapiye et al., Citation2021). Hence, the extension system has to be transformed and updated in parallel with the digital age (Tinzaara et al., Citation2021).
The rapid spread of mobile phones holds the promise of transforming traditional extension to a digital extension service through delivering agricultural information related to weather forecast, recommended agronomic practices, marketing and early warning through mobile phone (Hidrobo et al., Citation2022). Mobile phone-based agricultural extension services get high consideration due to better effectiveness and efficiency (McCampbell et al., Citation2023), increase the performance of smallholder farmers’ agri-food chains (Nugroho, Citation2021) and enable us to promote sustainable agricultural production by connecting marginalized farmers to extension workers (Cole & Fernando, Citation2021; Molina-Maturano et al., Citation2021).
Currently, Ethiopia used a public-based agricultural extension approach. All extension services are delivered by and through a large staff of extension personnel. The country has more than 65,000 extension personnel with approximately 21 Development Agents (DAs) per 10,000 farmers, which is the third largest amount worldwide (ATA, Citation2017). The Ethiopian extension system uses Farmer Training Center (FTCs)-based agricultural extension, coupled with farmer groups such as one-in-five and development units. Despite such efforts to make the extension system effective and efficient, the system is not producing the desired results. It is failing due to its high administration cost, lack of professional knowledge of extension personnel and limited geographical coverage. Hence, introducing mobile phone-based agricultural extension service can solve the aforementioned challenges (Abebaw, Citation2020; Abebaw & Yared, Citation2019; Chayot, Citation2022). The current extension service in Ethiopia is almost entirely funded by the government. There is a need to reform and looking for an alternative way of agricultural extension service, which is pluralistic and cost-effective. Transferring the cost of extension services to farmers could enhance efficiency and promote financial sustainability of the agricultural extension service (Daniel & Teferi, Citation2015).
The fast-evolving use of mobile phones in the country can bring tremendous potential to revolve such a traditional extension system approach to a modern mobile phone-based extension approach (Berhanu et al., Citation2020). However, this ample opportunity could not be used due to the absence of involvement of government, non-government bodies, private sectors and other volunteer practitioners in formal service design and implementation. Farmers often use mobile phones to get information from family, friends, or traders informally. Unfortunately, such information is often inaccurate, unreliable and inadequate. To develop a formal mobile phone-based extension service, understanding the farmer’s service demand is a prerequisite matter. Hence, analyzing farmers’ willingness to pay (WTP) for mobile-based extension service has paramount importance to service system design and financial sustainability.
Mobile phone-based extension service is an operationalized mean that the use of mobile phone is an agricultural extension tool through Short Message Service (SMS), Interactive Voice Record (IVR), audio and video calling and obtaining internet services. Identifying farmers’ maximum WTP for the mobile-based extension service will help policymakers about agricultural extension to develop new system designs. WTP survey has already been recognized to be a crucial tool for decision-making (Makwinja et al., Citation2019) and ex-ante project design and to evaluate the sustainability of any program or service. Several studies were conducted in Ethiopia on the use and adoption of mobile phones for accessing agricultural information (Abebaw, Citation2020; Abebaw & Yared, Citation2019; Amir et al., Citation2016, Citation2016; Berhanu et al., Citation2020; Birke, Citation2020; Girma et al., Citation2021). However, there is no empirical evidence of the service demand of farmers. Hence, this study aimed to analyze farmers’ willingness to pay for mobile phone-based extension services in northwestern Ethiopia.
2. Material and methods
2.1. Description of the study area
This study was conducted in the northern part of Ethiopia, in particular, the district of Raya Azebo, which is located in the southern zone of the Tigray region. Mixed farming is practiced in the district, the major crops grown are sorghum, Eragrostis teff, barley, wheat and maize and vegetable crops such as onion, potato, green pepper, and leaf vegetable crops are grown. Cattle, chicken, sheep and goats are the main livestock reared and limited numbers of farmers rear camels, honeybees, donkeys and mules. Deforestation, soil erosion, shortage of rainfall and free grazing are the environmental constraints in the district. The socio-economic constraints in the district are poor linkage of farmers in marketing, information network and low adoption of agricultural innovation.
The agricultural extension system of the district is operated by development agents with direct contact to farmers or through FTCs on their respective disciplinary backgrounds crop, livestock and natural resource management at the kebele level. In the district, the average farmer to extension agent ratio is approximately one development agent to 500 farmers. DAs lack proper mentoring support from Subject Matter Specialists (SMS) based in the district office of agricultural development. The district office of agricultural development is characterized by weak institutional delegation and lack of capacity to plan and power to make decisions (See Figure ).
2.2. Method of data collection and data types
Qualitative information and quantitative data were collected from primary and secondary sources. Primary data were collected from respondents by using the interview schedule. Secondary data were collected from journals and district agricultural development office.
The Contingent Valuation Method (CVM) was used to estimate farmers’ willingness to pay for agricultural extension service. Use of CVM surveys is contended to be a feasible method of gathering information on preferences for providing public goods and services. Many studies used CVM to provide evidence that households are willing to pay a significant amount for the provision of a certain non-marketed service (Abebe & Geta, Citation2014; Adewale et al., Citation2022; Amare et al., Citation2016; Angella et al., Citation2014; Daniel & Teferi, Citation2015; Gosbert et al., Citation2019; Teferi et al., Citation2014).
To determine the initial bids, focus group discussion and a pretest survey from 12 non-sample farmers were conducted from Kukuftu kebele.Footnote1 In double bound CVM, first, households were asked their willingness to join for the service. Second, the second price was determined on the basis of the response to the first price. If the response of household was “yes”, the second price was some amount higher than the first price; if the initial response was “no,” the second price is some amount lower. The overall bidding method is presented in Figure .
2.3. Sampling techniques and sample size
To select the target sample respondents, a multistage sampling technique was used. First, Raya Azebo district was selected from northern Ethiopia due to its agricultural production potential area. Second, from the total 18 kebeles, four sampled potential kebeles, namely, Wargiba, Kara, Warabaye and Bagie, were identified. Finally, using the simple random method with probability proportional to size, 280 sampled respondents were selected by using Yamane’s formula sampling determination,
where n is the sample size, N is the number of target farmers from four sampled kebeles and e is the level of precision ( = 0.05).
2.4. Method of data analysis
2.4.1. Contingent valuation method
To identify the farmers’ maximum amount of pay in cash for agricultural extension service, the CVM technique was employed. It is one of the stated preference valuation methods. There are two types of CVM techniques: single-bound and double-bound. Single bound CVM has starting point bias, which means that answers are “anchored” on the initial figure stated by the questioner. Double-bound contingent valuation format is relatively better, and having higher statistical preference due to having more information is elicited about each respondent’s WTP (“follow-up” question in addition to the “yes-no” options of the single-bound dichotomous choice) than of the single-bound contingent method (Carson & Hanemann, Citation2005; Cooper et al., Citation2002). Hence, the double-bounded technique was employed.
2.5. Econometric analysis
The econometric model was used to identify factors affecting farmers willingness to pay for agricultural extension service. The dependent variable for this study was the maximum amount of pay in cash, and, therefore, it was continuous variable including zero. Relative to other econometric models, the Tobit model is specifically relevant for such a data set and has better statistical efficiency for the willingness to pay data set (Brockwell & Chan, Citation2006; Endalew et al., Citation2019; Stewart, Citation2009). According to Wilson and Tisdell (Citation2002), multiple linear regression model estimates become biased and inefficient depending on the number of zeros in relation to the number of observations in the data set. The Tobit model is a relatively better model for double-bound CVM (Birara et al., Citation2018, Citation2020). Hence, the Tobit model was employed for this study. The mathematical specification used for the Tobit model is as follows:
where Y is the maximum willingness to pay in terms of cash and βi are the coefficient of explanatory variables, Xi are explanatory variables (see Table ) and Ui is the error term.
Table 1. Measurement and coding of selected variables
3. Results and discussion
3.1. Socio-demographic characteristics of respondents
Respondent farmers were asked to mention their willingness to join before elicitation question. Among 280 samples, 81.07% of them were willing to join in mobile-based agricultural extension service, whereas the rest of them, 18.93%, were not willing to join and pay anything at all.
The descriptive statistics of the variables included in the model are presented in Table . The mean age of the respondents was 41.3 years. Willing farmers were relatively younger than not willing farmers (Table ).
Table 2. Descriptive statistics for continuous variables
The average farm size, income, frequency of extension contact, family size and livestock ownership were 0.91 hectare, 19400 ETB, 3.81 days, 4.33 man equivalent and 4.2TLU, respectively. As shown in Table , the home of the willing respondents was relatively far from town as compared to non-willing respondents. The average distance from home to nearest town for willing respondents is 13.7 km, whereas the average distance from home to nearest town for non-willing farmers is 11.7 km.
With respect to sex, the results indicate that 86.0% of the farmers were males. About 43.0% of them were formally educated and only 16.0% of the non-willing respondents were formally educated. Likewise, about 46.0% of the respondents possessed mobile phone and only 9.4% of non-willing respondents did not possess the same. Among 280 respondents, 47.0% of them had access to electric power. Out of 53 non-willing respondents, only 32.0% had access to electric power. With regard to information seeking behavior, about 51.0% of willing farmers were relatively high information seeker than non-willing farmers (24.0%) as indicated (Table ).
Table 3. Descriptive statistics for dummy variables
CVM results indicate that farmers were willing to pay with the ranges from 30 to 200 ETB annually for mobile-based agricultural extension service and average farmers were willing to pay 101 ETB annually as indicated (Table ).
Table 4. Willingness to pay status and stated bids payment response
It is evinced from Table that 36.07% of the respondent farmers were willing to pay the higher amount beyond the stated bids (yes-yes). The other 19.0% of them snubbed to pay both the lower and the next minimum bid (no-no) response. A total of 24.0% of sample farmers were willing to pay the first offered amount and refused to pay the next maximum amount (yes−no). On the other hand, 21.07% of the farmers hesitated the first bid but agreed to pay the next minimum bid (no−yes) (Table ).
3.2. Reasons for not willing to pay
Non-willing farmers were asked to mention the reasons for not willing to pay for mobile-based extension service. Farmers may be willing to join mobile-based extension schemes as they see the value in the product but may not be willing to pay as they unable to afford, lack of trust, lack of knowledge and externalize responsibility. Results shown in Table indicate that farmers were not willing to pay for the service not because of service rejection but because of the assumed perception of farmers that this is the responsibility of government or other donor’s agency (28.3%), inability to afford (26.4%), lack of knowledge (15.1%) and lack of trust (11.3%) and only 18.9% rejected the service (Table ).
Table 5. Distribution of the respondents based on their responses for not willing to pay for the mobile phone-based extension service
It is assumed that most of the non-willing farmers showed positive attitude regarding the service and it is a matter of externalizing responsibility, knowledge and trust. It is evinced from Table that the majority of respondents, 40.0%, were very eager toward mobile-based extension program if it was implemented, whereas, 25.7%, 18.9% and 11.9% were happy, eager and moderately happy toward mobile-based extension, respectively. From total respondents, only 3.57% totally rejected the service.
Table 6. Respondent farmers’ attitude toward mobile phone-based extension service
Willing farmers were asked to indicate their preference of ways of information exchange when the service scheme will be implemented. Accordingly, a majority of respondents, 48.9%, responded that information exchange forms have to be dynamic depending on the nature of information that is supposed to distribute. Likewise, the remaining 29.5%, 11.45% and 10.13% of willing farmers preferred both audio and video, video only and SMS based-extension service, respectively.
Willing farmers were further asked to mention the type of information for which farmers are willing to pay for services, and the result found that 31.7% of respondent farmers were willing to pay more for services on market-related information. More than quarter of willing farmers 26.9% were willing to pay priority for information related to recommended agronomic practices as shown in Table .
Table 7. Farmers’ responses on forms and types of agricultural information
3.3. Factors affecting maximum willingness to pay
The Tobit model estimated the farmers’ maximum willingness to pay for mobile phone-based agricultural extension service for 13 explanatory variables included in the model. Out of the 13 hypothesized variables, five variables were found to be statistically significant which influence farmers’ willingness to pay for mobile based-extension service (see Table ).
Table 8. Results of the Tobit model
3.4. Discussion of significant variables
3.4.1. Education status of farmers (EDUCATION)
As likeminded with hypothesis, education attended by farmers had significantly and positively affected willingness to pay. The output of the Tobit model reveals that the probability of the farmers’ willingness to pay would be increased by 8.3% if the farmer is formally educated. This is because using mobile-based service needs formal knowledge and skills. This result is consistent with the findings of previous studies (Ningsi et al., Citation2020; Rico et al., Citation2023; Spyridon et al., Citation2021). Additionally, Adewale et al. (Citation2022) confirmed that education level of farmers is a determinant factor that determines how much farmers will be willing to spend to access plant clinic services in Bangladesh, Rwanda and Zambia.
3.4.2. Ownership of mobile phone (MOBOWN)
As prior hypothesis, this variable had a statistically significant and positive influence on the farmers’ willingness to pay mobile-based extension service at the 1% significant level. The output of the Tobit model reveals that the likelihood of the farmers’ willingness to pay would be increased by 10% if the farmers’ head possesses mobile phone. This finding is in line with the results of the previous study (Tadesse & Shively, Citation2013).
3.4.3. Information-seeking behavior of farmers (INFOSEEK)
It was another significant variable that determined willingness to pay for mobile-based agricultural extension service positively at less than the 1% probability significant level. The Tobit model results showed that the possibility of the farmers’ willingness to pay for the service would be increased by 7.3% if farmers are information seeker. This result is similar to the study by Brighton et al. (Citation2022).
3.4.4. Access to electric or solar power (POWERACC)
The other significant variable that determined willingness to pay for mobile-based extension service was access to electric or solar power. The result of the model indicated that the probability of the farmers’ willingness to pay would be increased by 3% if farmers own their electric or solar power. This might be due to farmer’s who has own electric or power source can easily access and use to mobile battery. In support of the finding, Abebaw & Yared (Citation2019) and Lelethu & Lwandiso (Citation2022) inveterate that solar power use and access to electricity determine farmers’ use of mobile phone for agricultural market information.
3.4.5. Nearest distance from town (DISFTOWN)
Results revel that distance from home to nearest town significantly and positively determine willingness to pay mobile-based extension service.
The willingness to pay of the farmers increases by 0.26% for an increase in one kilometer from farmer resident to nearest town. This implies that the farther the distance of resident of respondents from nearest town, the maximum the amount they are willing to pay for the service. This also proves that farmers who live far from town are willing to pay for mobile-based extension service relatively more in comparison to farmers who live near to town. This is due to the fact that information can be easily accessed in towns and cities and marginalized farmers are relatively more willing to pay the service due to high information searching cost. This result is similar to the findings of Samson et al. (Citation2011) who stated that the elongated distance from home to market and town can increase the intensity of mobile phone use for agricultural transactions. However, this result is contradicted by Getaw & Godfrey (Citation2015) and Tadesse & Shively (Citation2013) who reported that the farmers who are close to the town center have a higher likelihood of using mobile phones for information searching than farmers who are far from such centers.
4. Conclusion and recommendation
This study scrutinized farmers’ willingness to pay for mobile phone-based extension service in northern Ethiopia. The results show that more than 8 out of every 10 farmers were willing to pay a certain fee to receive mobile phone-based extension service. A majority of farmers responded that information shall exchange in all forms including audio, video and SMS depending on the nature of information if the service is implemented in the future. The Tobit model was employed to answer the question what are the factors that influence farmers’ maximum willingness to pay for mobile-based extension service. The results of the model indicate that farmers’ level of education, mobile phone ownership, information-seeking behavior, distance from home to nearest town and access to electric or solar power had a significant influence on farmers WTP for mobile-based agricultural extension service.
Based on the findings of the study, the following recommendations are put forward;
Ministry agriculture may impart a training package to farmers on mobile-based extension service through adult education and public media.
Ministry of information and telecommunication should provide rural ICT infrastructures and has to promote digital literacy to farmers.
Rural electrification is necessary to increase mobile phone subscription of farmers in short run so that function of the service will be sustainable in the long run.
The government, private sectors or other interested agency may implement the service considering the education level of farmers, information-seeking behavior of farmers, mobile phone subscription status of farmers and information infrastructure prior to designing the service.
Acknowledgments
The author acknowledges Raya university for financial assistance toward completion of this study.
Disclosure statement
No potential conflict of interest was reported by the author(s).
Data availability statement
The data that support the findings of this study are available from the corresponding author upon reasonable request. You can get the author address from the abstract page.
Additional information
Funding
Notes on contributors
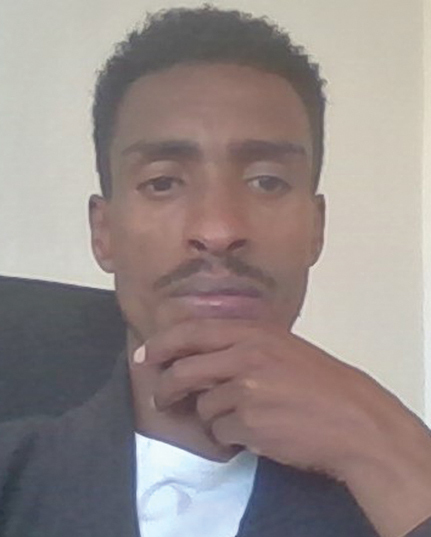
Abebaw Abebe
Abebaw Abebe obtained his BSc. degree in Agricultural economics from Jimma University and his MSc. degree in Agricultural information and communication management from Haramaya university. He is a lecturer and a researcher in the Department of Rural Development and Agricultural Extension at Mekdela Amba University. He is now engaged in teaching, research and community service. His special research interests are agricultural information analysis, socio-economic, agricultural market analysis, value chain analysis and food security.
Notes
1. Kebele is the lowest administration level in Ethiopia.
2. ETB= Ethiopia currency, and currently, 1$ is equivalent to 50 ETB and measured in thousands.
References
- Abbott, P., Checco, A., & Polese, D. (2021). Smart farming in sub-saharan Africa: Challenges and opportunities. Sensornets, 159–13. https://doi.org/10.5220/0010416701590164
- Abebaw, A. (2020). Challenges and opportunities of ICT use in Ethiopia. International Journal of Agricultural Extension, 08(1), 57–65. https://doi.org/10.33687/ijae.008.01.3069
- Abebaw, A., & Yared, M. C. (2019). Factors affecting the use of information and communication technologies for cereal marketing in Ethiopia. Journal of Agricultural & Food Information, 20(1), 59–70. https://doi.org/10.1080/10496505.2018.1438290
- Abebe, M. B., & Geta, E. (2014). Farmers’ willingness to pay for irrigation water use: the case of Agarfa District, Bale Zone, Oromiya National Regional State. ( M.Sc. thesis). Haramaya University,
- Adewale, M., Ogunmodede, J. A., Tambo, A. T., Adeleke, D. M., & Mary, O. O. (2022). Farmers’ willingness to pay towards the sustainability of plant clinics: Evidence from Bangladesh, Rwanda and Zambia. International Journal of Agricultural Sustainability, 20(7), 1360–1372. https://doi.org/10.1080/14735903.2022.2082018
- Amare, D., Mekuria, W., T/Wold, T., Belay, B., Teshome, A., Yitaferu, B., Tessema, T., & Tegegn, B. (2016). Perception of local community and the willingness to pay to restore church forests: The case of Dera district, northwestern Ethiopia. Forests, Trees and Livelihoods, 25(3), 173–186. https://doi.org/10.1080/14728028.2015.1133330
- Amir, M., Peter, N., & Muluken, W. (2016). The role of mobile phones in accessing agricultural information by smallholder farmers in Ethiopia. RUFORUM Working Document Series, 14(1), 395–402.
- Angella, N., Dick, S., & Fred, B. (2014). Willingness to pay for irrigation water and its determinants among rice farmers at Doho Rice Irrigation Scheme (DRIS) in Uganda. Journal of Development and Agricultural Economics, 6(8), 345–355. https://doi.org/10.5897/JDAE2014.0580
- ATA. (2017). Agricultural extension strategy of Ethiopia. Strategy report paper about Ethiopia agricultural extension system. Addis Ababa,
- Atiso, K., Folitse, B. Y., & Manteaw, S. A. (2021). Mobile telephony and agriculture information communication in Ghana: The Ho West district under review. Library Philosophy & Practice, 0_1–28. https://digitalcommons.unl.edu/libphilprac/4711
- Berhanu, D., Mequanent, M., & Biruk, F. (2020). Assessment of rural households ’ mobile phone usage status for rural innovation services in Gomma Woreda, Southwest Ethiopia. Cogent Food & Agriculture, 6(1), 1–13. https://doi.org/10.1080/23311932.2020.1728083
- Birara, E., Kassahun, T., & Zemen, A. (2018). Non-market measurement techniques of willingness to pay, the case of Environmental Resources: A review. Journal of Agro-Environment Science, 3(2).
- Birara, E., Wondimagegnhu, A., & Kassahun, T. (2020). Willingness to pay for church forest conservation: A case study in northwestern Ethiopia. Journal of Forest Science, 66(3), 105–116. https://doi.org/10.17221/154/2019-JFS
- Birke, F. M. (2020, January). Public agriculture extension and Information and Communication Technologies: A case study in South. A Doctoral dissertation in fulfillment of the requirement for the degree “Doktor Der Agrarwissenschaften” (Dr. Sc. Agr./Ph.D.) by the Faculty of Agricultural Sciences at the University of Hohenheim
- Brighton, N., Gideon, M., Tanyaradzwa, R., Peter, M., Charlene, M., & Basil, S. (2022). Rural small scale farmers’ smart mobile phone usage acceptance prognosticators for agricultural marketing information access. SN Social Science, 2(12), 356. https://doi.org/10.1007/s43545-022-00562-x
- Brockwell, A. E., & Chan, N. H. (2006). Long memory dynamic Tobit models. Journal of Forecasting, 25(5), 351–367. https://doi.org/10.1002/for.992
- Carson, R. T., & Hanemann, W. M. (2005). Contingent valuation. Handbook of Environmental Economics, 2, 821–936. https://doi.org/10.1016/S1574-0099(05)02017-6
- Chayot, G. (2022). The Ethiopian agricultural extension services: A mixed perspective. Cogent Food & Agriculture, 8(1), 1–11. https://doi.org/10.1080/23311932.2022.2132848
- Cole, S. A., & Fernando, A. N. (2021). ‘Mobile’izing agricultural advice technology adoption diffusion and sustainability. The Economic Journal, 131(633), 192–219. https://doi.org/10.1093/ej/ueaa084
- Cooper, J. C., Hanemann, M., & Signorello, G. (2002). One and-one-half-bound dichotomous choice contingent valuation. The Review of Economics and Statistics, 84(4), 742–750. https://doi.org/10.1162/003465302760556549
- Daniel, T., & Teferi, T. (2015). Determinates of small holder farmers willingness to pay for agricultural extension services: A case study from eastern Ethiopia. Africa Journal of Agricultural Research, 10(20), 2152–2158. https://doi.org/10.5897/AJAR2014.8698
- Davis, K. E., Babu, S. C., & Ragasa, C. (2020). Agricultural extension: Global status and performance in selected countries. Intl Food Policy Res Inst.
- Development, U. N., & Programme(UNDP). (2021, September). Precision agriculture for smallholder farmers
- Endalew, B., Wondimagegnhu, B. A., & Li, F. (2019). Determinants of households ’ willingness to pay for the conservation of church forests in northwestern Ethiopia: A contingent valuation study. Cogent Environmental Science, 5(1), 1–14. https://doi.org/10.1080/23311843.2019.1570659
- Gerba, L., Girma, K., Till, S., & Anna-Katharina, H. (2017). The agricultural extension system in Ethiopia: Operational setup, challenges and opportunities. ZEF Working Paper Series, ISSN 1864-6638 Center for Development Research, University of Bonn
- Getaw, T., & Godfrey, B. (2015). Mobile phones and farmers’ marketing decisions in Ethiopia. World Development, 68, 296–307. https://doi.org/10.1016/j.worlddev.2014.12.010
- Girma, Y., Kelil, A., & Yildiz, F. (2021). Mobile phone and beef cattle marketing: The case of Girar Jarso district of Oromia region, Ethiopia. Cogent Food & Agriculture, 7(1), 1911032. https://doi.org/10.1080/23311932.2021.1911032
- GIZ. (2021). Digital Agriculture platform blueprints. Digital agriculture platform blueprintswhite paper January 2021, Access Www. Mercycorpsagrifin.Org. www.mercycorpsagrifin.org
- Gosbert, L. S., Athman, K. A., & Jumanne, M. A. (2019). Factors determining crop farmers’ willingness to pay for agricultural extension services in Tanzania: A case of Mpwapwa and mvomero districts. Journal of Agricultural Extension & Rural Development, 11(12), 239–247. https://doi.org/10.5897/JAERD2019.1097
- Hidrobo, M., Palloni, G., Gilligan, D. O., Aker, J. C., & Ledlie, N. (2022). Paying for digital information: Assessing farmers’ willingness to pay for a digital agriculture and nutrition service in Ghana. Economic Development and Cultural Change, 70(4), 1367–1402. https://doi.org/10.1086/713974
- Lelethu, M., & Lwandiso, M. (2022). Factors affecting the using Information and Communication Technologies (ICTs) by livestock farmers in the Eastern Cape province. Cogent Social Sciences, 8(1). https://doi.org/10.1080/23311886.2022.2026017
- Makwinja, R., Kosamu, I. B. M., & Kaonga, C. C. (2019). Determinants and values of willingness to pay for water quality improvement: Insights from Chia Lagoon, Malawi. Sustainability, 11(17), 4690. https://doi.org/10.3390/su11174690
- Mapiye, O., Makombe, G., Molotsi, A., Dzama, K., & Mapiye, C. (2021). Towards a revolutionized agricultural extension system for the sustainability of smallholder livestock production in developing countries: The potential role of icts. Sustainability, 13(11), 5868. https://doi.org/10.3390/su13115868
- McCampbell, M., Adewopo, J., Klerkx, L., & Leeuwis, C. (2023). Are farmers ready to use phone-based digital tools for agronomic advice? ex-ante user readiness assessment using the case of Rwandan banana farmers. The Journal of Agricultural Education and Extension, 29(1), 29–51. https://doi.org/10.1080/1389224X.2021.1984955
- Molina-Maturano, J., Verhulst, N., Tur-Cardona, J., Güereña, D. T., Gardeazábal-Monsalve, A., Govaerts, B., & Speelman, S. (2021). Understanding smallholder farmers’ intention to adopt agricultural apps: The role of mastery approach and innovation hubs in Mexico. Agronomy, 11(2), 194. https://doi.org/10.3390/agronomy11020194
- Ningsi, R., Asnawi, & Abdullah, A. (2020). Effect of intrinsic factors on farmers’ willingness to pay on the success of artificial insemination of Bali cattle. The 2nd International conference of animal science and technology IOP Publishing, IOP Conf. Series: Earth and environmental science 492 012161. https://doi.org/10.1088/17551315/492/1/012161
- Nugroho, A. D. (2021). Agricultural market information in developing countries: A literature review. Agricultural Economics (Zemědělská ekonomika), 67(11), 468–477. doi:https://doi.org/10.17221/129/2021-AGRICECON
- Rajkhowa, P., Qaim, M., & Van Campenhout, B. (2021). Personalized digital extension services and agricultural performance: Evidence from smallholder farmers in India. PloS One, 16(10), e0259319. https://doi.org/10.1371/journal.pone.0259319
- Rico, A., Aminou, A., Miroslava, B., Vladimir, V., Wilfried, Y., & Jan, B. (2023). Analysis of the factors influencing the adoption of digital extension services: Evidence from the rice advice application in Nigeria. The Journal of Agricultural Education and Extension. doi:https://doi.org/10.1080/1389224X.2023.2222109
- Samson, P. K., Julius, J. O., & Noel, J. (2011). Use of mobile phone Technology in agricultural marketing: The case of smallholder farmers in Malawi. International Journal of ICT Research and Development in Africa, 2(2), 14–25. doi:https://doi.org/10.4018/jictrda.2011070102
- Spyridon, P., Uthpal, K., Amjath-Babu, T. S., & Fulco, L. (2021). Are farmers willing to pay for participatory climate information services? Insights from a case study in peri-urban Khulna, Bangladesh. Climate Services, 23(2021), 100241.
- Stewart, J. (2009). Tobit or not tobit? IZA discussion papers, No. 4588.
- Tadesse, G., & Shively, G. (2013). Repeated transaction in rural grain markets of Ethiopia. Journal of Development Studies, 49(9), 1172–1187. https://doi.org/10.1080/00220388.2012.740015
- Teferi, T., Daniel, T., & Sundara, D. (2014). Factors affecting farmers’ willingness to pay for agricultural extension services: The case of Haramaya district, Ethiopia. International Journal of Agricultural Science Research, 3(12), 268–277.
- Tinzaara, W., Oyesigye, E., Bocquet, C., Arkin, J., & Johnson, V. (2021). Mobile phone technology for increasing banana productivity among smallholder farmers in Uganda. Journal of Agricultural Extension & Rural Development. doi:https://doi.org/10.5897/JAERD2020.1191
- Wilson, C., & Tisdell, C. A. (2002). OLS and tobit estimates: When is substitution defensible operationally? University of Queensland, school of Economics. Economic Theory, Applications and Issues, Working Paper No. 15.