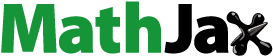
Abstract
New agricultural technologies and improved practices play a key role in increasing agricultural production and productivity, as well as improving national food security and overall social well-being. A large body of empirical literature shows how different technologies and improved practices can affect welfare indicators. However, rigorous evidence on the food security and nutritional implications of these technologies remains scarce in general and particularly in developing regions such as sub-Saharan Africa. This study uses panel data and adopts a fixed-effects approach as the main analysis method. Propensity score matching and endogenous treatment effects techniques are also used to test robustness and reduce selection bias in the estimation process. Thus, this study examines the relationship between adoption and nutrition, which has received little attention in most previous studies, and evaluates the impacts of three improved agricultural technologies (row planting, high-yielding seed varieties, and chemical fertilizers) on household food security and child nutrition. The results of the study show that the adoption of these technologies had a significant impact on both per capita consumption expenditure and child nutrition. In addition, the results of the differential impact analysis confirmed that impacts differed across the different segments of the households (quartiles based on area under improved technology and gender). We, thus, recommend the use of advanced agricultural technologies in combination (multiple types) rather than as separate technologies, and relevant institutions should promote the combination of advanced agricultural technologies, and the need for continued and large-scale public and private investment in the sector to address major development challenges. Finally, we note that the results from the current study have a broad and generic value that can be applied to a range of related cases in other developing countries.
PUBLIC INTEREST STATEMENT
Globally, millions of people are at risk of hunger and starvation. At the same time, several of them are fighting to survive in famine-like conditions. Increasing agricultural production and productivity is becoming the major agenda of governments and policy-makers. This action in turn highly depends on the types of agricultural technologies and farming techniques in use. Thus, this study explored the potential impact of adopting improved agricultural technologies on rural household food security and child nutrition. The results of the study showed that adopting of those agricultural technologies significantly improved both per capita consumption expenditure and child nutrition.
1. Introduction
A recent social welfare analysis showed that ensuring global food security is one of the major challenges (FAO, Citation2015a; Jaleta et al., Citation2018; Schipanski et al., Citation2016; World Food Programme, Citation2020), and malnutrition also remains a major development challenge (FAO, Citation2017; Gillespie & van den Bold, Citation2017; Schipanski et al., Citation2016). Globally, an estimated 345.2 million people will be food insecure in 2023 (The World Health Organization, Citation2023), a number that is more than double in 2020. Compared to the time before the COVID-19 pandemic, this is 200 million more people than it was before. More than 900,000 people in the world are struggling to survive in famine-like conditions. This is 10 times more than 5 years ago, an alarmingly rapid increase.Footnote1
One possible solution is to increase the productivity of agriculture. Increasing agricultural productivity in poor countries is critical to reducing hunger, which increases food availability and lowers food prices. Increasing the productivity of smallholders also increases their income and improves their ability to survive. Increasing agricultural productivity in developing countries is essential to reducing hunger and poverty, especially due to rapidly rising food prices.
Agricultural productivity growth, on the other hand, is highly dependent on the agricultural techniques and improved practices used. The diffusion and utilization of improved agricultural technologies and supervision of output enhancing improved practices can be traced back thousands of years to various regions of the world, including China, Mesopotamia, Egypt, and the Americas. The origins of national or government-sponsored extension and advisory services can be traced back to Ireland and Great Britain in the middle of the 19th century. During the Irish Potato Famine (1845–1851), agricultural advisors effectively supported Irish potato producers as they diversified into different agricultural products (Swanson & Rajalahti, Citation2010).
The adoption and implementation of innovations in a social system occur through the acceptance of individuals or groups. According to Feder et al. (Citation1985), adoption is the integration of an innovation in farmers’ normal farming actions over a long period of time. It should also be noted that the adoption of innovation is not a constant behavior but is characterized by the preferences and decisions of agents over time-based on many factors.
Several empirical studies (e.g. see Amare et al., Citation2012; Asfaw et al., Citation2012; Ayenew et al., Citation2020; Habtewold, Citation2018, Citation2021; Hundie & Admassie, Citation2016; Kassie et al., Citation2018; Mulugeta & Hundie, Citation2012; Wossen et al., Citation2019) showed that new agricultural technologies and improved practices play an important role, arguing that adoption of agricultural technologies can affect households’ welfare indicators like poverty, food security, productivity, employment, and income both directly and indirectly. This extensive literature shows that direct effects of technology adoption include increased productivity and lower unit costs of production, which can increase the incomes of adopters, while indirect benefits can be in the form of increased supply, which can lower food prices.
Increased productivity can also increase labor demand, which can lead to increased employment and incomes for the poor. In addition, some recent studies have found that technology adoption is a key activity to achieve food security (e.g., Addis & Sani, Citation2021; Asfaw et al., Citation2012; Aweke et al., Citation2021; Habtewold, Citation2020; Hailu et al., Citation2021; Sinyolo, Citation2020; Sissoko et al., Citation2022; Wekesa et al., Citation2018; Zegeye et al., Citation2022), reduces poverty levels (Biru et al., Citation2020; Habtewold, Citation2018, Citation2021; Verkaart et al., Citation2017; Wossen et al., Citation2019), improves households’ nutritional status (Issahaku & Abdulai, Citation2020; Tigabu & Gebeyehu, Citation2018; Zeng et al., Citation2017), increases dietary diversity and food security (Magrini & Vigani, Citation2016; Tesfaye & Tirivayi, Citation2018; Wordofa et al., Citation2020), positively contribute to women’s empowerment (Yilma et al., Citation2015), and reduces the risks of crop failures (Hagos et al., Citation2012).
As shown by some previous studies (e.g. Asfaw et al., Citation2012), in a large part of sub-Saharan Africa (SSA), the agricultural sector is the main source of economic growth. Improving the productivity, profitability, and sustainability of smallholder farming is therefore the most important way for these countries to escape poverty (Muzari et al., Citation2012; Odame et al., Citation2013; Ogada et al., Citation2014). Africa’s land resources could quickly produce an additional 100 million tons per year if intensively cultivated (Farsund et al., Citation2015), indicating that the continent has significant untapped potential. Some evidence also indicates that approximately 800 million hectares in SSA are ideal for rainfed agriculture (Utuk & Daniel, Citation2015), suggesting that Africa has a significant amount of arable land that can improve long-term nutrition in the region. Despite Africa’s enormous agricultural potential, Africa has struggled to fully adopt modern agricultural techniques. Unless Africa adopts modern or improved agricultural techniques that can increase climate change resilience and production, especially for subsistence farmers, the continent will waste the abundant natural resources it already has and will have to rely on expensive imports.
However, evidences indicate that much of African population is at risk of hunger and starvation. Currently, about 346 million people in Africa are malnourished. It is also indicated that in 2021 alone, about 7.2 million people in East Africa are at risk of hunger, and another 26.5 million face acute food insecurity (Verner et al., Citation2021; Wudil et al., Citation2022).
Another challenging issue is child nutrition, with acute malnutrition affecting at least 12.8 million children in the region during the same period. Similarly, in Ethiopia, almost 30% of households are in extreme poverty and hunger, which has persisted for the same number of years (The World Bank, Citation2015). More than 30% of the country’s population is malnourished and 41.3% of them face food inadequacy (FAO, Citation2015b); 36% of the country’s agricultural households are engaged in subsistence farming and live on less than $2 a day. Rapidly growing population and limited expansion of cultivated land can lead to a growing gap between food production and consumption needs. Therefore, improving the agricultural production and productivity of the country is not an option, but a necessity. Research in Africa shows that the adoption of improved agricultural techniques, although inconsistently and incompletely evaluated, generally improves social welfare as measured by income, food security, and poverty (e.g. Asfaw et al., Citation2012; Ayenew et al., Citation2020; Hundie & Admassie, Citation2016; Kangmennaang et al., Citation2017; Kassie et al., Citation2018; Pan et al., Citation2018; Shiferaw et al., Citation2014; Wossen et al., Citation2019).
For example, Asfaw et al. (Citation2012) investigated the adoption of modern agricultural technologies and their impact on the welfare of smallholder farmers in Tanzania and Ethiopia, and the results show that the adoption of technologies increased per capita consumption expenditure and led to a reduction in poverty levels. Similarly, Shiferaw et al. (Citation2014)investigated the adoption of improved wheat varieties and their impact on household food security in Ethiopia, and the results showed that results showed that the adoption increased the average per capita consumption expenditure of the sampled adopting households between 209 and 260 Ethiopian Birr (ETB).Footnote2
Similarly, Habtewold (Citation2021) studied the impact of climate-smart agricultural technology on multidimensional poverty in rural Ethiopia and the results showed a reduction in overall and living standard deprivations. Khonje et al. (Citation2015) evaluated the impact of improved cassava varieties in Zambia and the results showed that the introduction of improved cassava varieties significantly increased crop yield, household income, and food security. Asfaw et al. (Citation2012) and Amare et al. (Citation2012) studied the impact of improved agricultural technologies in Tanzania and found that the introduction of improved technologies such as pigeon pea and maize varieties increased household welfare through consumption expenditure.
On the other hand, some studies also show that food security depends not only on agricultural technologies but also on post-harvest technologies and new food processing technologies, including ozone and new non-thermal technologies such as cold plasma. For example, a study by Prabha et al. (Citation2015) showed that ozone technology (a triatomic gas that has recently emerged as an effective broad-spectrum disinfectant against pathogenic microbes) is widely used in post-harvest treatment, storage, and food processing technology in the field.
Again, the concept of cold plasma technology is based on the partial ionization of charged particles and gas molecules in the form of positive and negative ions, free radicals, electrons, and photons (Niveditha et al., Citation2021; Ucar et al., Citation2021; Umair et al., Citation2022), which is a recent technical measure whose main purpose is to maintain food quality and safety. In particular, it is a technology created for curing and bonding polymers, which is also used in several industries (Fernandez et al., Citation2013). Thus, apart from the widely known agricultural technologies like improved seeds, modern farming (row planting), fertilizer application, crop rotation, cluster farming, etc., oxidation technologies such as cold plasma and ozone are two emerging techniques that are widely utilized in food processing practice to maintain better food security.Footnote3
Regarding the impact of agricultural technologies and improved practices on nutrition, their beneficial effects are much less known, and relatively less research has been conducted on this aspect (but few exceptions include the studies of Issahaku & Abdulai, Citation2020; Kabunga et al., Citation2017; Teklewold et al., Citation2019; Tigabu & Gebeyehu, Citation2018; Wordofa et al., Citation2020; Zeng et al., Citation2017). Research conducted elsewhere outside Africa with the same objectives and under different conditions confirmed that agricultural technology and practices had a positive impact on well-being.
Taken together, the above review of the extensive existing literature helps us to identify the main channels through which the adoption of modern agricultural technologies and improved practices affect overall welfare. The extensive literature above and some recent studies point to evidence of increased efforts to adopt improved agricultural techniques and how this affects household welfare (see Asfaw et al., Citation2012, Citation2012; Biru et al., Citation2020; Habtewold, Citation2021; Kassie et al., Citation2015; Khonje et al., Citation2015; Tesfaye & Tirivayi, Citation2018; Verkaart et al., Citation2017; Wordofa et al., Citation2020). The scope, approaches, and methods employed by these studies, however, vary widely and they generate some common tendencies, yet reporting conflicting or/and inconclusive results.
Although much is known about the potential effects of different technologies on well-being based on the results of previous studies, many of these studies are mostly cross-sectional, based on similar data sets, mostly using a single technology and a simple assessment method, and focusing on very limited agricultural technology perspectives rather than packaging (but some notable exceptions are Bezu et al., Citation2014; Khonje et al., Citation2018; Smale & Mason, Citation2014). Therefore, there are doubts about the reliability of the results here. Most previous studies use a single agricultural technology and do not compare the multiple effects of different technologies or improved practices. This study examines the adoption of several combinations of improved agricultural techniques and practices, as the combination of improved agricultural techniques has a greater impact on social welfare than either alone. Using improved and updated data and methods, this study adds to existing knowledge in the field by comparing the multiple impacts of different technologies or improved practices. Based on two rounds of the Ethiopian Socioeconomic Survey (ESS) datasets this study makes four important contributions to the literature. First, we examine the welfare effects of three different technologies (row planting, high-yielding seed varieties (HYVs), and chemical fertilizers) using comprehensive and nationally representative household-level panel data employing different and plausible estimation methods that can account for selection biases. Only Khonje et al. (Citation2018) attempted to consider the impact of several agricultural technologies on welfare in Zambia but were limited to a combination of conservation agricultural practices and only to the case of improved maize varieties. Second, the study examines the impact over different time periods, as the adoption of technologies is a dynamic process. Rigorous impact assessment is important over time, and it is also important to update information based on currently available on-farm techniques. Third, the study also explores adoption-child nutrition links while most studies rarely explore.
Finally, the heterogeneous impact of the presented technologies between different household groups is also evaluated. This study is the first attempt to examine the differential impact of the adoption of three technologies on household food security and child nutrition. The single notable exception in the literature is the study of Olagunju et al. (Citation2020) who tried to examine the distributional impacts of adoption of drought-tolerant maize varieties on the productivity and welfare outcomes, however, the study used only a single technology, drought-tolerant maize varieties. In the current study, these technologies/practices are particularly chosen because they are widely practiced in almost all parts of the country and farm households are also familiar with them.
The rest of this study is organized as follows. Section 1 describes the methodology used for estimating the impact of the stated technology. The findings of the study are discussed in Section 2 while the remaining two sections give the concluding remarks and some policy implications of the study’s results.
2. Methodology
2.1. Conceptual framework of adoption decision and impact evaluation
In empirical impact evaluation, assessing and estimating the impact of technology adoption on households’ welfare outcome variables based on non-experimental data is one of the major challenges faced by researchers. Experimental data can provide information on the counterfactual situation to solve the problem of causal inference, which is a fundamental problem in non-experimental studies (Heckman et al., Citation1997). Becker (Citation2009) argues that the main challenge in impact assessment is causal inference and shows that it is uncommon to observe for the same unit i the treatment values Wi = 1 and Wi = 0 and similarly the outcome values Yi(1) and Yi(0). In addition, Amare et al. (Citation2012) and Asfaw et al. (Citation2012) explain that technology adoption is not randomly distributed between treatment and control groups, but households make their own adoption choices, which may differ systematically. Therefore, possible self-selection due to observed and unobserved household characteristics makes an assessment of the real welfare impact of technology adoption using observational data more difficult. If we fail to correctly account for this potential selection bias, it could lead to inconsistent estimates of the impact of technology adoption.
Households are assumed to maximize their utility function subject to budget constraints. Applying a random utility framework, households decide to adopt a technology if the adoption increases their utility levels. The difference between the utility of adoption () and non-adoption (
) is given as W* such that the utility maximizing farm household i will choose to adopt the technology if the utility gained from adopting is greater than the utility of not adopting the technology which is given by
(Feder et al., Citation1985; Jara-Rojas et al., Citation2012). However, the two utilities are unobservable as non-experimental data do not contain sample counterparts but can be expressed as a function of observable components in the latent variable model as follows:
where Wi is a binary variable for the use of new technology; Wi = 1 if the technology is adopted and Wi = 0 otherwise. β is a vector of the parameters to be estimated, is a vector that represents socio-economic and household characteristics, t is time (year dummies), and
is the random error term with mean zero and constant variance. EquationEquation 1
(1)
(1) is called the selection or treatment equation of new technology adoption.
The first empirical modeling approach the study adopts is the two-ways fixed-effects (FE) error components structure method which allows us to estimate treatment effects considering differing adoption times. We use the FE model to eliminate the effects of observable and unobserved household heterogeneity that are fixed over time, as a source of bias in estimates of the technologies’ impacts. However, commonly, the fixed-effects error structure only incorporates the potential influence of time-invariant unobservables (it does not control time-variant heterogeneity). Some recent studies also argue that FE approach could still produce biased estimates for the treatment variables’ coefficients (Arslan et al., Citation2017; Bezu et al., Citation2014; Manda et al., Citation2016). To address these limitations of the FE approach, we complemented the analysis by employing the non-parametric regression method, PSM, and endogenous treatment effects (ETE) model to assess the robustness of the results.
PSM is built on the intuitively attractive idea of contrasting the outcomes of the program’s participants with the outcomes of “comparable” non-participants. Differences in the outcomes between the two groups are attributed to the program’s effects (Heckman et al., Citation1998). PSM is a two-step procedure. First, a probability model for adopting an improved technology is estimated to calculate the probability (or propensity score) of adoption for each observation. In the second step, each adopter is matched to a non-adopter with similar propensity score values for estimating the average treatment effects on the treated (ATT). For the consistency of the estimated treatment effects, conditional independent assumptions must hold, namely the condition that selection in the program is independent of the outcome of participation. Some matching methods have been developed to match adopters with non-adopters with similar propensity scores. Asymptotically, all matching methods should yield the same results, even though there are trade-offs in terms of bias and efficiency in each method. However, PSM only controls for biases emanating from observed heterogeneity. Hence, we also added the ETE model to consider the endogeneity of adoption decisions (Heckman, Citation1976, Citation1978; Maddala, Citation1983; Wooldridge, Citation2010).
2.2. Model specification
The two-way fixed-effects error component structure is given by:
where is the outcome variable (food security in terms of consumption expenditures and child nutrition indicators) for household i in the adoption category at time t, Wi is the treatment indicator factor as defined in EquationEquation 1
(1)
(1) are household fixed-effects,
are the year effects or wave fixed-effects, and
is the random error term.
is a vector of household characteristics, and θ is the impact of interest in our case or a factor that captures the average treatment effects.
In the second estimation technique, following Cameron and Trivedi (Citation2005), Greene (Citation2012), Heckman (Citation1976, Citation1978), Maddala (Citation1983), and Wooldridge (Citation2010) we specify the ETE model as follows:
where are the covariates used for modeling the treatment assignment and the error terms
and
from EquationEquation 1
(1)
(1) and EquationEquation 3
(3)
(3) are bivariate normal with mean zero and the covariance matrix is given as:
It should be noted that the covariates and
are unrelated to the error terms (they are exogenous). Thus, this is termed as a constrained model because the variance and correlation parameters are identical across the two treatment and control groups.
is the variance of the disturbance term (
) in the main outcome regression EquationEquation 3
(3)
(3) , the variance of the error term (
) in the selection EquationEquation 1
(1)
(1) of technology adoption; and
is the covariance of
and
. The maximum likelihood estimates (MLE) provide us consistent and asymptotically efficient results. Using the MLE technique, we estimate the ETE model with different options.
EquationEquation 3(3)
(3) can be generalized to a potential-outcome model with separate variance and correlation parameters for the treatment and control groups as:
The likelihood function for this model is discussed in Maddala (Citation1983) and Greene (Citation2000) and it presents the standard method of reducing a bivariate normal to a function of a univariate normal and the correlation . The log likelihood function for EquationEquation 1
(1)
(1) and EquationEquation 3
(3)
(3) for farm household i (refer to Greene, Citation2000, p.180; Maddala, Citation1983, p.122) is expressed as:
where is the cumulative distribution function of the standard normal distribution. In the MLE method
and
are not directly estimated, instead
and
are directly estimated where they are expressed as
. The standard error of
is simply approximated through the delta method, which can be expressed by the functional form:
where D is the Jacobian of λ with respect to and
.
The third method that this study uses is PSM, which compares the outcomes of treated observations with the outcomes of comparable non-treated observations based on the computed propensity scores of the groups. The propensity score is defined as the conditional probability of receiving treatment given pre-treatment characteristics as:
where = {0, 1} is the indicator of the exposure to the treatment as defined in EquationEquation 1
(1)
(1) and X is the multidimensional vector of household characteristics.
One of the main advantages of matching methods is that it does not require any functional form assumptions for the outcome equation (i.e., it is non-parametric) and are therefore not susceptible to misspecification bias along that dimension (Rosenbaum & Rubin, Citation1983). A study by Rosenbaum and Rubin (Citation1983) shows that if exposure to a treatment (in our case, the adoption of technologies) is random in cells defined by X, it is also random in cells defined by values of a univariate variable . Thus, given a population of units denoted by i, if the propensity score P(Xi) is known, ATT can be estimated as:
2.3. Data sources and variables
The analysis is based on panel data obtained from the Ethiopia Socioeconomic Survey (ESS) Waves 2 and 3 datasets which is a collaborative project of the Central Statistical Agency (CSA) of Ethiopia and the World Bank’s Living Standards Measurement Survey (WB-LSMS). This study used only the two recent survey datasets, 2013 and 2015, which are used for the sake of accuracy and availability of relevant information. The first wave, collected in 2011, which has several missing information on factors like child food intake and row planting, was not included in the study.
The survey covered around 5,262 and 4,954 households drawn from the nine regional states and two city administrations in waves 2 and 3, respectively. The current study considered three types of agricultural technologies and improved practices – row planting, HYVs, and chemical fertilizers. After controlling and accounting for missing observations and non-applicable households (e.g., households with no children, households with no agricultural activities, etc.) the sample size for row planting technology included 3,875 households, while 5,295 households were taken for the HYVs category and 5,806 households were considered for chemical fertilizers for the two waves in total (see Figure for the details of sampling distribution). The reduction in the number of observations seems so large, however, this is due to the fact that the dataset contains several missing information on major indicators. Our final sample is an unbalanced panel type for all the three technologies. According to Hillier et al. (Citation2011) in any appropriate study unbalanced panel data helps to moderate the survivorship bias problem. In addition, Arellano (Citation2003) also argues that estimations constructed from unbalanced panel data are as good as studies based on balanced panel data and almost equally reliable.
Figure 1. Sample distribution across periods of the survey.
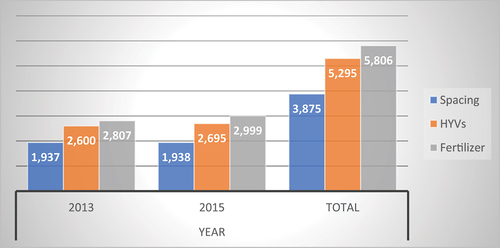
As the focus of this study is identifying the impact of improved agricultural technologies on food security and child nutrition, four outcome variables were used for evaluating the impact of these technologies: household per capita food consumption expenditure; average food intake per day per child; self-response to food shortages in households; and self-response to whether a household worried about the availability of food or not.
One of the challenges in relation to the data was the absence of information on the child nutrition indicator. We tried to use either anthropometric measurement indicators like height and weight and circumference and length of various body regions or the body mass index (BMI), but none of this information was included in the survey. A possible and available option was using the average food intake per day per child as a possible proxy for child nutrition outcome.
3. Empirical results and discussion
3.1. Results of the descriptive analyses
This study examined three different agricultural technologies and improved practices. In the first case, we considered the adoption of row planting, in which adoptersFootnote4 were farm households who planted either improved or local seeds (either wheat, maize, or both) in row planting and non-adopters were those who did not use the row planting method for improved or local varieties. Considering the second technology, we classified adopter households who planted any of the improved HYVs (two major crop varies planted in the country, wheat and maize are considered) and the final type of technology investigated is chemical fertilizers, in which adopters were households who used any type of chemical fertilizers while non-adopters were those who planted wheat or/and maize without applying chemical fertilizers.
Using these three different agricultural technologies enabled us to compare and identify which technology was highly associated with welfare enhancement and had a stronger impact on social well-being and related measures. Table reports the descriptive statistics for row planting and the result indicate that about 15% of the sample households adopted row planting (see Table in the Appendix). Summary statistics and tests of statistical significance on equality of means for continuous variables and equality of proportions for binary variables for adopters and non-adopters are presented in the table. A significant difference was revealed in consumption expenditure between the two subsamples. The summary statistics shows that adopters on average had more per capita food consumption expenditure (ETB 4,120 per year per adult equivalent), which is significantly higher than the equivalent of non-adopters (ETB 3,360). Although we found statistically insignificant results, non-adopters as compared to adopters faced higher level of food shortages and worried more about the availability of enough food throughout the year based on self-reported subjective responses.
Concerning child nutrition outcome, measured by the average food intake per day per child, on average adopter households had a higher food intake in which a child ate food about 3.9 times per day as compared to 3.7 times per day among non-adopters. Similarly, the logarithmic form (converted for the purpose of interpretation as a percentage values or elasticities) showed statistically significant differences between the two groups. Thus, without making any causal relationship, we prove that there is a difference in consumption per capita and child nutrition status between the two subsamples, though early to draw any conclusion. Though the unconditional descriptive statistics presented above suggest that row planting may have a role in improving household well-being, yet this simple comparison of consumption per capita and child nutrition has no causal interpretation as adoption itself is endogenous (Manda et al., Citation2018; Shiferaw et al., Citation2014; Zeng et al., Citation2017).
Looking at other characteristics and control variables, we found that adopters who had a higher level of education as measured by more than 6 years of schooling, were older by reported age, had better access to credit, received more information about seeding according to recommendations, and got more electricity for lighting. On the other hand, non-adopters had more livestock holdings and used more crop rotation methods for planting. The non-adopter group was also significantly distinguishable in terms of using more firewood as sources of light and cooking fuel, and most of them follow non-orthodox religions making them statistically different from the adopters. In this preliminary analysis, it is possible to observe that there were some significant differences between the adopters and non-adopters. However, the above differences may not be only due to the adoption of the technology, but instead it might be due to other factors (both observed and unobserved household characteristics). Therefore, we must conduct a thorough cause-and-effect evaluation using reliable evaluation methods. FE, ETE, and PSM methods were used in the empirical part of the study to identify and quantify these differences or find the impact of technologies.
3.2. Econometric results
3.2.1. Quality of matching and sensitivity analysis
Before assessing the causal effects of the technologies, we tested the quality of the matching process. Matching methods have been tested and passed various quality control tests. After estimating the propensity scores for the adopter and non-adopter groups, the common support space was checked. A visual inspection of the density distributions of the estimated propensity scores for the subsamples of the two groups (see Figures in the Appendix section) shows that the common support condition was met, meaning that there was substantial and considerable overlap in the distribution of the propensity scores of both adopters and non-adopters for all the three technologies. The bottom half of the figures show the propensity scores’ distribution for the non-adopters and the upper half stands for the adopters; the densities of the scores are on the y-axis.
The imbalance in the variables, such as the propensity score, between the treatment and control groups was the subject of the second test. Before matching for the three technologies, there was a about 98% propensity score disparity between the groups (not indicated here). After matching, this bias was greatly decreased (far below 3%). The test demonstrates that multiple variables have statistically significant differences prior to matching, but that after matching, the covariates were balanced, demonstrating the effectiveness of the PSM technique and providing guarantee that the two groups may be matched based on the common support restricted areas.
Covariate balance tests before and after matching, as shown in Table in the Appendix, were the third test run. Before matching, the standardized mean difference for the total variables used to calculate the propensity score for the three technologies was around 8.4%; after matching, it was about 3.2%. The joint significance of the covariates was always rejected after matching, although it was never rejected before matching, according to the p-values of the likelihood ratio tests reported. Additionally, with the three technologies, the pseudo R2 drastically decreased from 1.2–4.0% before matching to roughly 0.3–1.1% after matching. The likelihood ratio test was also statistically significant before matching for all the three technologies but it became insignificant after matching. The low pseudo R2, high bias reduction, and the insignificant p-values of the likelihood ratio test after matching suggest that the proposed specification of the propensity score was successful in terms of balancing the distribution of covariates between the two subsamples.
Finally, we examine the sensitivity of our estimated results to hidden bias using the Rosenbaum bound technique (Rosenbaum, Citation2002).Footnote5 Per capita consumption expenditure and child nutritionFootnote6 were the two main outcome variables that were subjected to the sensitivity analysis. This test is a type of proxy for examining the conditional independence assumption (CIA), which is the primary assumption of the PSM technique. To investigate the reliability of matching estimates to the CIA, Rosenbaum (Citation2002) devised the bounds test. The test enables us to ascertain the degree to which an unmeasured confounding variable must influence treatment selection in order to undermine the implications of a matching analysis. This provides reasonable confidence that there is a causal relationship between the treatment and the outcome variable even in the presence of potential unobserved variables. Thus, the test determines the point at which the estimated effects no longer hold (how robust the ATT is to unobserved heterogeneity).
Since both outcome variables are continuous, the sensitivity analysis findings were calculated in STATA version 15 using the rbounds command, and the results are presented in Table in the Appendix. The rbounds is used to calculate the maximum and minimum p-values from a Wilcoxon sign rank test between treatment and matched pairs, with different values of gamma, Γ (a range of possible values attributable to unobserved heterogeneity), and in our case, we use 1 to 2 and its increment is in the range of 0.1 as the majority of the literature arguesuggest (DiPrete & Gangl, Citation2004; Duvendack & Palmer-Jones, Citation2012). By observing how the p-value varies for increasing values of or how the size of the treatment effects changes with a higher of Γ, we can examine the robustness based on p-values. Rosenbaum (Citation2002) states that we can conclude a high sensitivity to hidden bias if the conclusions change for values of Γ just slightly higher than one and low sensitivity if the conclusions change at large values of Γ.
In the first row, the Γ is set to one, assuming total randomization through matching. The rbounds test for the first technology, row planting, demonstrates that the causal effects on per capita consumption spending would change at a relatively smaller bound statistic, Г = 1.2 (i.e., when the sensitivity parameter is equal to 1.2, the upper bound of the p-value becomes insignificant). This suggests that the two household groups chances of receiving treatment with the same observed variables can vary by up to 20% without changing the treatment’s impact. According to these findings, the ATT is not very sensitive to hidden bias (Willy et al., Citation2014); hence, the estimated effect is due to the adoption of row planting, aware of hidden bias. Concerning the impact of row planting on child nutrition, the sensitivity analysis shows that through the increase of Γ up to 1.7, the upper bound of the p-value does not exceed the 5% level of significance and until 1.8 does not exceed 10% level. This indicates that the result is not so vulnerable to unobserved bias. Thus, the result suggests that the positive and significant impact of row planting adoption on child nutrition is not sensitive to hidden-bias.
The results of the boundary tests for the final two technologies—HYVs and fertilizers—are essentially identical to those of row planting. According to the findings for the two outcome variables (see Table ), the effects of implementing all three technologies on per capita consumer expenditure are somewhat more sensitive to hidden bias than on child nutrition. Thus, the Rosenbaum bounds sensitivity analysis indicates that our results of the matching estimators, in general, are robust to hidden bias indicating that adoption of the three technologies have positive effects on both per capita consumption expenditure and child nutrition.
Following the aforementioned experiments, the results of the fixed effect model are presented in Table , which demonstrates that the adoption of row planting has a considerable impact on the nation’s food security. According to the results, adopters of row planting significantly improve their per capita consumption spending in all scenarios, both with and without control estimations, through Models 1 through 4. Under the four models, the overall average gains from implementing the technology on per capita consumption expenditure ranged from around ETB 605 to 756.
Table 1. FE results row planting: outcome variable is per capita consumption expenditure
In the first FE model’s specifications in Table , we included the treatment status of households and the observation period’s variables. The results showed that there was a strong impact of technology on food security. Based on Model 1, the per capita consumption expenditure of the adopters was higher by about ETB 756 as compared to non-adopters and the result was statistically significant. In Model 2 personal and household characteristics were added to the treatment and time variables. Again in the third model, we incorporated variables indicating access to social services along with credit and extension provisions. Finally, in the fourth FE regression model, we included other controls indicating households’ asset ownership.
The additional factors did not significantly alter the predicted treatment impact of the technology when we added more and more control variables at various levels. The average increase in per capita consumption expenditure in all the four FE models was statistically significant. These findings so demonstrate that the adoption of this technology has a beneficial and considerable influence on food security, even with increasingly more controls. The results for Model 4 in Table are not fully interpreted, however, a number of variables were statistically significant.
For ease of interpretation and for avoiding a possible rightward skewness, the consumption expenditure was transformed into logarithms; and the estimated impact of row planting on this transformed per capita consumption expenditure was found to be statistically significant. The estimated results reported in Column 1 of Table show that per capita consumption expenditure was about 10% higher for adopters. A similar result was also obtained for child nutrition in which the overall average gain in child nutrition was significantly higher for the adopters. A possible impact pathway for this is through increase in production then via consumption—where technology adoption improves productivity/profitability—that also increases incomes and enables households to feed their children more nutritious food.
Table 2. FE results row planting: outcome variables: ln per capita consumption expenditure, ln child nutrition, food shortage, and worry about food
Children in the technology adopter subsamples had increased food intake per day per child by about 6% due to the improved agricultural technologies (see Table , Column 2). However, it was observed that there was no association between row planting and the remaining two outcome variables, namely—self-response on food shortages and whether households worried about the availability of food or not. Hence, no causal interpretation will be inferred from the results in the last two columns of Table . Thus, adoption of row planting is not linked to a statistically significant change in solving problems related to food shortages and households’ fears about the availability of enough food throughout the year. The possible explanation here is that, though row planting is able to increase the short-term consumption level of adopters, there is a gap in terms of securing sustained availability of food in the household.
We also used PSM and ETE methods along with FE to check the consistency of the output of different estimation methods. Table reports the results of all the three estimation methods on the impact of row planting on food security measured by consumption expenditure. The PSM results are reported using four matching methods—nearest neighbourhood matching (NNM), kernel matching (KM), radius matching (RM), and stratification matching (SM)—while in the ETE approach we used estimation techniques including inverse-probability weighted (IPW), inverse-probability weighted regression adjustment (IPWRA), and linear regression with endogenous treatment effects (LRETE).
Table 3. FE, PSM, and ETE methods’ results on the impact of row planting on the welfare of rural households
As we see from Table , when FE is used, the average consumption per capita of the adopter households improved by about ETB 756. Similarly, using the log of consumption per capita as an outcome variable, the results show that technology adoption increased per capita consumption by about 13%. The increase in child nutrition was also statistically significant and higher for technology adopters by about 5 percentage points.
Using PSM and ETE approaches with various estimating settings, results that were almost identical were produced. When adopting row planting using the PSM method, the average increase in per capita consumption expenditure ranged from ETB 633 to 750 under the four algorithms, while when using the ETE method, the gain ranged from roughly ETB 644 to 649 under the three estimation options, and all of them were statistically significant. This measures the average difference in consumption expenditure of similar pairs of households that have different technological status. This demonstrates that farmers who adopted row planting had much higher per capita consumption expenditures than non-adopters.
Concerning the adoption of HYVs, Table reports the results of the FE method on per capita consumption expenditure. The results show that adoption of HYVs highly increased adopters’ per capita consumption expenditure. Looking at the impact conditioned on controlling for other factors, the FE results show an increase in per capita consumption expenditure by about ETB 707 for adopter households. This is equivalent to a 10 percentage points increase in per capita consumption expenditure which is statistically significant. This result supports the descriptive statistical analysis discussed earlier and shows that there was a significant difference in per capita food consumption between adopter and non-adopter households.
Table 4. FE results for HYVs: outcome variable is per capita consumption expenditure
Again, we estimated the FE model with different specifications for HYVs. In Table , we include the treatment status of households and the observation period’s variables in Column 1; in the second column, we add household characteristics with treatment and time variables; in the third column under Model 3 we incorporate the variables indicating access to social services like credit and extension provisions; and finally in the fourth FE regression model we incorporate control variables indicating households’ asset ownership. The inclusion of more control variables did not alter the estimated treatment effects of HYVs technology adoption in which HYVs significantly affected per capita consumption expenditure under all models.
The results of the PSM and ETE techniques also demonstrate that the per capita consumption expenditure of HYV adopters increased between ETB 532 and 617 when PSM is used and between ETB 553 and 749 when ETE is used. To put it another way, the results are statistically significant and are equivalent to an increase in the projected impact of HYVs on the transformed per capita consumption for adopters compared to non-adopters from 6% to 9% using PSM and 7% to 35% using ETE. The anticipated results for child nutrition likewise imply an increase in daily food intake for kids in HYV-adopted households. The estimated results show that the adoption of HYVs improved child nutrition in the range between 5% and 20% as we see from the last column of Table .
Table 5. FE, PSM, and ETE methods’ results on the impact of HYVs on per capita consumption and child nutrition
Table reports the FE estimation results of adoption of chemical fertilizers, the final technology, on per capita consumption expenditure. The result shows that chemical fertilizers improved per capita consumption expenditure of adopters by about ETB 437. We also estimated FE with the inclusion of control variables like household characteristics and access to assets and services, and the results remained robust and consistent throughout.
Table 6. FE method’s results for fertilizer use: outcome variable is per capita consumption expenditure
In general, households that adopted chemical fertilizers improved their food consumption expenditure through more yields leading to higher revenues and good access to social services that are linked to having more income. According to this result, fertilizer adoption raised adopters’ per capita consumption by about 1 percentage points on average as compared to the non-adopters, though the transformed per capita consumption had an insignificant effect (see Table ).
Table 7. FE, PSM, and ETE methods’ results on the impact of fertilizer use on the welfare of rural households
Table illustrates how the three estimating approaches all came to the same conclusion—namely, that the adoption of chemical fertilizers had a favorable and significant influence on the food security of adopter households—by using the transformed logarithms for per capita consumption expenditure. Based on these results, we can conclude that the use of chemical fertilizers led to an average rise in per capita consumption of 3% to 7% using PSM and 4% to 6.8% using ETE techniques. Additionally, as seen in the last column of Table , the adoption of chemical fertilizers has a significant positive impact on children’s nutrition. According to the PSM method, adoption of chemical fertilizers raised child nutrition in adopter households by about 55% on average as compared to non-adopters, while the impact using FE and ETE regressions showed an average of 56% increase in child nutrition.
A possible pathway is that children in adopting households may increase food intake per day by consuming more own-produced outputs as adoption potentially increases yield and adopting households may also have more access to other foods with increased in adoption of improved inputs like of chemical fertilizers. We can argue that these findings imply that better child nutrition outcomes can be achieved through chemical fertilizers adoption without gaining of additional land. The findings are consistent with previous literature (e.g., Zeng et al., Citation2017)
Concerning the remaining two of our outcome variables—food shortages and whether a household worried about the availability of food – (not reported here) similar to the previous technologies, no causal interpretation will be inferred from the chemical fertilizers adoption. This shows that chemical fertilizers adoption did not create differences between adopters and non-adopters concerning food shortages and availability.
The three improved agricultural technologies had a significant impact on household food security and child nutrition overall, according to the results of the FE, PSM, and ETE methods. Table provides a summary result for the two key outcome variables—consumption expenditure and child nutrition. Results are generally consistent across estimating techniques and show that the technologies under consideration have had a major positive influence. Adoption has almost nontrivial effects, despite the fact that the size of estimated effects significantly differs between estimating techniques. These are in line with earlier research findings (Amare et al., Citation2012; Asfaw et al., Citation2012; Habtewold, Citation2021; Jaleta et al., Citation2018; Kassie et al., Citation2018; Khonje et al., Citation2015; Manda et al., Citation2018; Shiferaw et al., Citation2014; Zeng et al., Citation2017).
Table 8. Summaries of FE, PSM, and ETE methods’ results for the three technologies
3.3. Heterogeneous impacts
3.3.1. Differential household-level impacts by area, gender and time
Beyond the conventional impact assessment methods, this study used a differential or distributional analysis to investigate the varied effects of the three technologies on food security and child nutrition in households and to take into account heterogeneity at various distributions of the outcomes. The results are shown in Table . This is because adoption has differential impacts on different segments of the households and across time. The households were divided into quartiles based on cultivated area under the three technologies, by household head’s gender and observation period. This time we employed the PSM stratification method that can identify the heterogeneity of the impacts among the different parts of the households.
Table 9. Heterogeneous/Differential impacts of the three technologies by area under improved technology, gender, and observation year
The analysis, in general, indicates that the three technologies positively influence households’ welfare under different categories. With respect to per capita consumption expenditure, the impacts of adoption of the three technologies are significant in almost all quartiles, except the first quantiles in case of row planting and fertilizer, and last quartile in HYVs adoption. The finding for the impact of row planting on per capita consumption expenditure shows that, in value terms, the gain ranged from ETB 470 (in the 3rd quartile) to ETB 1,345 (in the 4th quartile). This suggests that the influence of row planting on per capita consumption expenditure for adopter with greater areas of land under row planting is higher than households that use less of their land under row planting technology.
Similarly, both HYVs and fertilizer influenced per capita consumption expenditure positively. In the case of both technologies, the gain in per capita consumption increases up to the 2nd quartile and then declines from the 3rd through the 4th quartiles. In other words, the impacts of both technologies are found to be the highest in the lowest middle quartile (2nd quartile). Here it is important to note that, unlike row planting where farmers do not need significant cost to apply the technology, in both HYVs and fertilizer adoption farmers may be faced with a sudden increase in costs and a lack of access to improved inputs such HYVs and chemical fertilizers. This, therefore, suggests that farmers continuously use both HYVs and fertilizers up to certain levels and reduce after some levels due to the income or/and substitution effects of those inputs.
Concerning the impacts of row planting by gender, the technology makes male-headed households more food secure than those headed by females and the increment in per capita consumption expenditure is about ETB 821 per year. Female-headed households also benefit from the technology, but the impact is not statistically significant. Similar results were obtained when HYVs are adopted, however, the food security results reveal that when fertilizer is adopted, female-headed households benefited more from fertilizers as compared with their male counterparts in terms of raises in per capita consumption expenditure. Last, we explored the impacts on per capita consumption by year of observation and results for all technologies indicate that, though findings seem mixing, the influence of both row planting and fertilizers were higher in the year 2013 as compared to the following period, while HYVs influenced per capita consumption more in 2015.
Results from child nutrition indicator regressions further suggest that the nutrition impacts are largest among children with the lower quartiles for both row planting and fertilizers, while the highest impact was gained by the 4th quartiles for HYVs technology. The impacts of adoption of the three technologies are significant in all segments, except for female-headed households in case of row planting technology. In terms of percentage impact of row planting, child nutrition indicator increased in the range 3–6%. The results for other categories can be compared and interpreted in a similar fashion.
Generally speaking, this method of disaggregating impacts by various households segments gives us a vivid picture of the varied impacts of adoption of the three technologies on both food security and child nutrition. The empirical results showed that there is great variety in the differential affects of the technologies, and that the impacts of adoption of those technologies vary significantly along the different categories taken into consideration in the study.
4. Conclusion
This study investigated the possible impacts of row plantations, HYVs, chemical fertilizers, and other improved agricultural methods on rural households’ food security and child nutrition. The results of all estimations, methods demonstrated that the introduction of more effective agricultural technology had a strong, significant, and advantageous effect on per capita consumer expenditures and child nutrition. In order to evaluate the distributional effects of adoption impacts, we also evaluated the impacts of adoption of the three technologies across other household groups.
Regarding the results obtained from adopting the row planting technology, the average increase in per capita consumption expenditure in all estimation methods were statistically significant. The overall average gain of per capita consumption expenditure ranged from ETB 633 to 756 as compared to the non-adopters. Our study also showed that children in the technology adopter households had increased food intake per day per child. Adoption of HYVs also resulted in highly increased per capita consumption expenditures among the adopters. The estimated results of child nutrition also show that on average daily food intake for children in the adopter households (adoption of both row planting and HYVs) has significantly been increased.
Looking at the estimated technology adoption results for fertilizers, using all methods led to the same conclusions that the adoption of chemical fertilizers had a positive and significant impact on both per capita consumption and child nutrition of adopter households. We also noted that the pathway for the impact of technology adoption on child nutrition is not direct and instead it goes as technology adoption continues through time. Adoption improved productivity/profitability that increased incomes and enabled households to feed their children with more nutritious food. The impact of adoption also differed across the different categories considered as the differential household-level impact analysis shows. Concerning the remaining two of our four outcome variables—food shortages and whether a household worried about the availability of food or not—there was no strong support for the impact of these technologies.
5. Policy implications
Our study’s findings have two significant national policy implications. First, the findings indicate that all three technologies have significant positive impacts on per capita consumption expenditure and child nutrition, highlighting the need for ongoing, extensive public and private investments in agricultural research and the adoption of various technologies to address pressing developmental issues like widespread poverty and food insecurity.
Second, though the above technologies, in general, have positive impacts, the results of the differential impact analysis confirmed that impacts differed across the different segments of the households. Row planting revealed that as more of the technology is used its impact increase with no limit, but for the case of the remaining technologies, the impact increases up to certain levels. This calls a selective and careful intervention in identifying and using technologies based on conditions and farms behavior. Given that farmers’ preferences for variety attributes affect their likelihood of adopting improved varieties as well as their propensity to do so, technology supply should meet the needs of various farm household types classified according to their resource endowments, preferences, and constraints.
As with research in general, our study had some limitations and may suggest future research directions. First, the study is based only on two-round panel data. We recommend further research in the region using relatively longer panel data to cover the dynamics and long-term effects of the adoption of different technologies. Second, proxies for child nutrition could be improved using measures such as height and weight, as well as circumference and length of different body regions and body mass index (BMI).
Authors’ contributions
Both authors agree with this submission and have contributed equally to all parts of the manuscript.
Code availability
Code is available upon request.
Data availability
Data is available upon request.
Disclosure statement
No potential conflict of interest was reported by the author(s).
Additional information
Funding
Notes on contributors
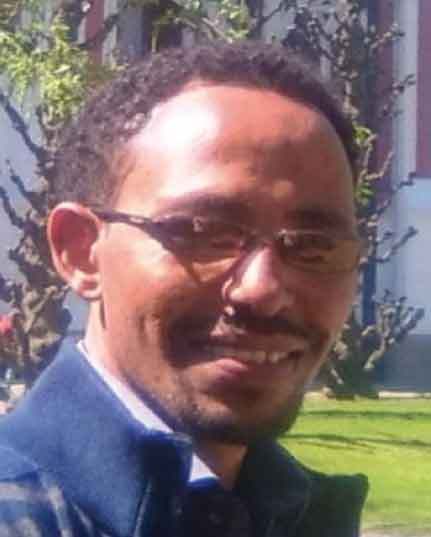
Tsegaye Mulugeta Habtewold
Tsegaye Mulugeta Habtewold (Ph.D) is an Assistant Professor of Economics at Department of Technology and Innovation Management, Adama Science and Technology University, Adama, Ethiopia. He holds PhD in Economics from Addis Ababa University in 2020 and MSc in Development Economics from Ethiopian Civil Service University in 2011. His research interest includes: adoption and impact assessment of improved agricultural technologies and their implications on poverty, food security, and gender in sub-Saharan Africa. Tsegaye has gained research expertise in several areas of agricultural economics and technology adoption and diffusion in Ethiopia. Currently, he is working on research related to improved agricultural technologies, women’s empowerment, and nutrition in east Africa.
Almas Heshmati
Almas Heshmati is a Professor of Economics at Jönköping University, Sweden. He held similar positions at the Sogang University, Korea University, Seoul National University, University of Kurdistan Hawler, and the MTT Agrifood Research (Finland). He was a Research Fellow at the World Institute for Development Economics Research (WIDER), The United Nations University during 2001-2004. From 1998 to 2001, he was an Associate Professor of Economics at the Stockholm School of Economics. He has a Ph.D. degree from the University of Gothenburg (1994), where he held a Senior Researcher position until 1998. His research interests include applied microeconomics, globalization, efficiency, productivity, and growth with application to manufacturing, agriculture and services, environmental economics, and well-being.
Notes
1. The earlier version of this paper was presented at the 4th Annual Eastern African Business and Economics Watch (4th EABEW, 2019), an international conference organized by the University of Rwanda in collaboration with Jönköping International Business School (JIBS), Kigali, Rwanda, June 12–14, 2019.
2. Birr is the official currency of Ethiopia. On January 26, 2022, $1.00 = 49.53 ETB.
3. Interested readers can also refer to the application of non-destructive techniques in maintaining food security.
4. Note: for all the three technologies a farm household is considered to be an adopter if it uses the stated technology for five and above years consecutively.
5. The sensitivity analysis is carried out applying rbound and mhbounds stata command provided by DiPrete and Gangl (Citation2004) and Becker and Caliendo (Citation2007), respectively. The rbound is performed on continuous outcomes variables and is based on the Wilcoxon sign rank test and Hodges-Lehmann point estimate for sign rank test, while mhbounds focuses on the case of binary outcomes variables and is based on Mantel and Haenszel (Citation1959) test statistic.
6. Rosenbaum bound test results for the remaining outcome variables are not reported here to save space, however, results are available upon request from the authors.
References
- Addis, Y., & Sani, S. (2021). Impact of adoption of improved agricultural production technologies on cereal crops productivity and farmers’ welfare in Central Ethiopia. Indian Journal of Science and Technology, 14(44), 3280–27. https://doi.org/10.17485/IJST/v14i44.1306
- Amare, M., Asfaw, S., & Shiferaw, B. (2012). Welfare impacts of maize–pigeon pea intensification in Tanzania. Agricultural Economics, 43(1), 27–43. https://doi.org/10.1111/j.1574-0862.2011.00563.x
- Arellano, M. (2003). Panel data econometrics. Oxford university press.
- Arslan, A., Belotti, F., & Lipper, L. (2017). Smallholder productivity and weather shocks: Adoption and impact of widely promoted agricultural practices in Tanzania. Food Policy, 69, 68–81. https://doi.org/10.1016/j.foodpol.2017.03.005
- Asfaw, S., Kassie, M., Simtowe, F., & Leslie, L. (2012). Poverty reduction effects of agricultural technology: A micro-evidence from Tanzania. Journal of Development Studies, 48(9), 1288–1305. https://doi.org/10.1080/00220388.2012.671475
- Asfaw, S., Shiferaw, B., Simtowe, F., & Lipper, L. (2012). Impact of modern agricultural technologies on smallholder welfare: Evidence from Tanzania and Ethiopia. Food Policy, 37(3), 283–295. https://doi.org/10.1016/j.foodpol.2012.02.013
- Aweke, C. S., Hassen, J. Y., Wordofa, M. G., Moges, D. K., Endris, G. S., & Rorisa, D. T. (2021). Impact assessment of agricultural technologies on household food consumption and dietary diversity in eastern Ethiopia. Journal of Agriculture and Food Research, 4, 100141. https://doi.org/10.1016/j.jafr.2021.100141
- Ayenew, W., Lakew, T., & Kristos, E. H. (2020). Agricultural technology adoption and its impact on smallholder farmers welfare in Ethiopia. African Journal of Agricultural Research, 15(3), 431–445. https://doi.org/10.5897/AJAR2019.14302
- Becker, S. O. (2009). Methods to estimate causal effects theory and applications. U Stirling, Ifo, CESifo and IZA last update: 21 August 2009. Stirling Management School.
- Becker, S. O., & Caliendo, M. (2007). Sensitivity analysis for average treatment effects. The Stata Journal, 7(1), 71–83. https://doi.org/10.1177/1536867X0700700104
- Bezu, S., Kassie, G. T., Shiferaw, B., & Ricker-Gilbert, J. (2014). Impact of improved maize adoption on welfare of farm households in Malawi: A panel data analysis. World Development, 59, 120–131. https://doi.org/10.1016/j.worlddev.2014.01.023
- Biru, W. D., Zeller, M., & Loos, T. K. (2020). The impact of agricultural technologies on poverty and vulnerability of smallholders in Ethiopia: A panel data analysis. Social Indicators Research, 147(2), 517–544. https://doi.org/10.1007/s11205-019-02166-0
- Cameron, A. C., & Trivedi, P. K. (2005). Microeconometrics: Methods and applications. Cambridge University Press.
- DiPrete, T. A., & Gangl, M. (2004). 7. Assessing bias in the estimation of causal effects: Rosenbaum bounds on matching estimators and instrumental variables estimation with imperfect instruments. Sociological Methodology, 34(1), 271–310. https://doi.org/10.1111/j.0081-1750.2004.00154.x
- Duvendack, M., & Palmer-Jones, R. (2012). High noon for microfinance impact evaluations: Re-investigating the evidence from Bangladesh. The Journal of Development Studies, 48(12), 1864–1880. https://doi.org/10.1080/00220388.2011.646989
- FAO. (2015a). Climate change and global food systems: Global assessments and implications for food security and trade. FAO.
- FAO. (2015b). The State of Food Security in the World. http://www.fao.org/3/a-i4646e.pdf.
- FAO. (2017) . Nutrition-sensitive agriculture and food systems in practice: Options for intervention. Rome.
- Farsund, A. A., Daugbjerg, C., & Langhelle, O. (2015). Food security and trade: Reconciling discourses in the food and Agriculture Organization and the World trade Organization. Food Security, 7(2), 383–391. https://doi.org/10.1007/s12571-015-0428-y
- Feder, G., Just, R. E., & Zilberman, D. (1985). Adoption of agricultural innovations in developing countries: A survey. Economic Development and Cultural Change, 33(2), 255–298. https://doi.org/10.1086/451461
- Fernandez, A., Noriega, E., & Thompson, A. (2013). Inactivation of Salmonella enterica serovar typhimurium on fresh produce by cold atmospheric gas plasma technology. Food Microbiology, 33(1), 24–29. https://doi.org/10.1016/j.fm.2012.08.007
- Gillespie, S., & van den Bold, M. (2017). Agriculture, food systems, and nutrition: Meeting the challenge. Global Challenges, 1(3). https://doi.org/10.1002/gch2.201600002
- Greene, W. H. (2000). Econometric analysis (4th ed.). NJ: Prentice Hall.
- Greene, W. H. (2012). Econometric analysis (7th ed.). Stern School of Business.
- Habtewold, T. M. (2018). Adoption and impacts of improved agricultural technologies on rural poverty. In A. Heshmati & H. Yoon (Eds.), Economic growth and development in Ethiopia. Perspectives on development in the Middle East and North Africa (MENA) region (pp. 13–35). Springer.
- Habtewold, T. M. (2020). Impacts of Improved Agricultural Technologies Adoption on Multidimensional Welfare Indicators in Rural Ethiopia [ Doctoral dissertation]. Addis Ababa University.
- Habtewold, T. M. (2021). Impact of climate-smart agricultural technology on multidimensional poverty in rural Ethiopia. Journal of Integrative Agriculture, 20(4), 1021–1041. https://doi.org/10.1016/S2095-3119(21)63637-7
- Hagos, F., Jayasinghe, G., Awulachew, S. B., Loulseged, M., & Yilma, A. D. (2012). Agricultural water management and poverty in Ethiopia. Agricultural Economics, 43(s1), 99–111. https://doi.org/10.1111/j.1574-0862.2012.00623.x
- Hailu, M., Tolossa, D., Girma, A., & Kassa, B. (2021). The impact of improved agricultural technologies on household food security of smallholders in Central Ethiopia: An endogenous switching estimation. World Food Policy, 7(2), 111–127. https://doi.org/10.1002/wfp2.12029
- Heckman, J. (1976). The common structure of statistical models of truncation, sample selection and limited dependent variables and a simple estimator for such models. Annals of Economic and Social Measurement, 5, 475–492.
- Heckman, J. (1978). Dummy endogenous variables in a simultaneous equation system. Econometrica, 46(4), 931–959. https://doi.org/10.2307/1909757
- Heckman, J., Ichimura, H., Smith, J. A., & Todd, P. E. (1998). Characterizing selection bias using experimental data. Econometrica, 66(5), 1017–1098. https://doi.org/10.2307/2999630
- Heckman, J., Ichimura, H., & Todd, P. E. (1997). Matching as an econometric evaluation estimator: Evidence from evaluating a job training programme. The Review of Economic Studies, 64(4), 605–654. https://doi.org/10.2307/2971733
- Hillier, D., Pindado, J., De Queiroz, V., & De La Torre, C. (2011). The impact of country-level corporate governance on research and development. Journal of International Business Studies, 42(1), 76–98. https://doi.org/10.1057/jibs.2010.46
- Hundie, B., & Admassie, A. (2016). Potential impacts of yield-Increasing Crop technologies on productivity and poverty in two districts of Ethiopia. In F. Gatzweiler & J. von Braun (Eds.), Technological and institutional innovations for marginalized smallholders in agricultural development (pp. 397–421). Springer International Publishing.
- Issahaku, G., & Abdulai, A. (2020). Can farm households improve food and nutrition security through adoption of climate‐smart practices? Empirical evidence from northern Ghana. Applied Economic Perspectives and Policy, 42(3), 559–579. https://doi.org/10.1093/aepp/ppz002
- Jaleta, M., Kassie, M., Marenya, P., Yirga, C., & Erenstein, O. (2018). Impact of improved maize adoption on household food security of maize producing smallholder farmers in Ethiopia. Food Security, 10(1), 81–93. https://doi.org/10.1007/s12571-017-0759-y
- Jara-Rojas, R., Bravo-Ureta, B. E., & Díaz, J. (2012). Adoption of water conservation practices: A socioeconomic analysis of small-scale farmers in Central Chile. Agricultural Systems, 110, 54–62. https://doi.org/10.1016/j.agsy.2012.03.008
- Kabunga, N. S., Ghosh, S., Webb, P., & Loor, J. J. (2017). Does ownership of improved dairy cow breeds improve child nutrition? A pathway analysis for Uganda. PloS One, 12(11), e0187816. https://doi.org/10.1371/journal.pone.0187816
- Kangmennaang, J., Kerr, R. B., Lupafya, E., Dakishoni, L., Katundu, M., & Luginaah, I. (2017). Impact of a participatory agroecological development project on household wealth and food security in Malawi. Food Security, 9(3), 561–576. https://doi.org/10.1007/s12571-017-0669-z
- Kassie, M., Marenya, P., Tessema, Y., Jaleta, M., Zeng, D., Erenstein, O., & Rahut, D. (2018). Measuring farm and market level economic impacts of improved maize production technologies in Ethiopia: Evidence from panel data. Journal of Agricultural Economics, 69(1), 76–95. https://doi.org/10.1111/1477-9552.12221
- Kassie, M., Teklewold, H., Marenya, P., Jaleta, M., & Erenstein, O. (2015). Production risks and food security under alternative technology choices in Malawi: Application of a multinomial endogenous switching regression. Journal of Agricultural Economics, 66(3), 640–659. https://doi.org/10.1111/1477-9552.12099
- Khonje, M., Manda, J., Alene, A. D., & Kassie, M. (2015). Analysis of adoption and impacts of improved maize varieties in eastern Zambia. World Development, 66, 695–706. https://doi.org/10.1016/j.worlddev.2014.09.008
- Khonje, M. G., Manda, J., Mkandawire, P., Tufa, A. H., & Alene, A. D. (2018). Adoption and welfare impacts of multiple agricultural technologies: Evidence from eastern Zambia. Agricultural Economics, 49(5), 599–609. https://doi.org/10.1111/agec.12445
- Maddala, G. S. (1983). Limited-dependent and qualitative variables in econometrics. Cambridge University Press.
- Magrini, E., & Vigani, M. (2016). Technology adoption and the multiple dimensions of food security: The case of maize in Tanzania. Food Security, 8(4), 707–726. https://doi.org/10.1007/s12571-016-0593-7
- Manda, J., Alene, A. D., Gardebroek, C., Kassie, M., & Tembo, G. (2016). Adoption and impacts of sustainable agricultural practices on maize yields and incomes: Evidence from rural Zambia. Journal of Agricultural Economics, 67(1), 130–153. https://doi.org/10.1111/1477-9552.12127
- Manda, J., Gardebroek, C., Kuntashula, E., & Alene, A. D. (2018). Impact of improved maize varieties on food security in Eastern Zambia: A doubly robust analysis. Review of Development Economics, 22(4), 1709–1728. https://doi.org/10.1111/rode.12516
- Mantel, N., & Haenszel, W. (1959). Statistical aspects of the analysis of data from retrospective studies of disease. Journal of the National Cancer Institute, 22(4), 719–748.
- Mulugeta, T., & Hundie, B. (2012). Impacts of adoption of improved wheat technologies on households’ food consumption in Southeastern Ethiopia. Selected Poster prepared for presentation at the International Association of Agricultural Economists (IAAE) Triennial Conference, Foz do Iguaçu, Brazil, August 18-24, 2012.
- Muzari, W., Gatsi, W., & Muvhunzi, S. (2012). The impacts of technology adoption on smallholder agricultural productivity in sub-Saharan Africa. Journal of Sustainable Development, 5(8), 69–77. https://doi.org/10.5539/jsd.v5n8p69
- Niveditha, A., Pandiselvam, R., Prasath, V. A., Singh, S. K., Gul, K., & Kothakota, A. (2021). Application of cold plasma and ozone technology for decontamination of Escherichia coli in foods-a review. Food Control, 130, 108338. https://doi.org/10.1016/j.foodcont.2021.108338
- Odame, H., Alemu, D., Kimenye, L., Kabutha, C., & Oduori, L. H. (2013). Why the low adoption of agricultural technologies in Eastern and Central Africa? Association for Strengthening Agricultural Research in Eastern and Central Africa. https://www.asareca.org/sites/default/files/publications/LOW%20ADOPTION%20(pdf%20for%20web).pdf.
- Ogada, M. J., Mwabu, G., & Muchai, D. (2014). Farm technology adoption in Kenya: a simultaneous estimation of inorganic fertilizer and improved maize variety adoption decisions. Agricultural and Food Economics, 2(1), 1–18. https://doi.org/10.1186/s40100-014-0012-3
- Olagunju, K. O., Ogunniyi, A. I., Awotide, B. A., Adenuga, A. H., & Ashagidigbi, W. M. (2020). Evaluating the distributional impacts of drought-tolerant maize varieties on productivity and welfare outcomes: An instrumental variable quantile treatment effects approach. Climate and Development, 12(10), 865–875. https://doi.org/10.1080/17565529.2019.1701401
- Pan, Y., Smith, S. C., & Sulaiman, M. (2018). Agricultural extension and technology adoption for food security: Evidence from Uganda. American Journal of Agricultural Economics, 100(4), 1012–1031. https://doi.org/10.1093/ajae/aay012
- Prabha, V., Barma, R. D., Singh, R., & Madan, A. (2015). Ozone technology in food processing: A review. Trends in Biosciences, 8(16), 4031–4047.
- Rosenbaum, P. R. (2002). Observational studies (2nd ed.). Springer.
- Rosenbaum, P. R., & Rubin, D. B. (1983). The central role of the propensity score in observational studies for causal effects. Biometrica, 70(1), 41–55. https://doi.org/10.1093/biomet/70.1.41
- Schipanski, M. E., MacDonald, G. K., Rosenzweig, S., Chappell, M. J., Bennett, E. M., Kerr, R. B., Blesh, J., Crews, T., Drinkwater, L., Lundgren, J. G., & Schnarr, C. (2016). Realizing resilient food systems. BioScience, 66(7), 600–610. https://doi.org/10.1093/biosci/biw052
- Shiferaw, B., Kassie, M., Jaleta, M., & Yirga, C. (2014). Adoption of improved wheat varieties and impacts on household food security in Ethiopia. Food Policy, 44, 272–284. https://doi.org/10.1016/j.foodpol.2013.09.012
- Sinyolo, S. (2020). Technology adoption and household food security among rural households in South Africa: The role of improved maize varieties. Technology in Society, 60, 101214. https://doi.org/10.1016/j.techsoc.2019.101214
- Sissoko, P., Synnevag, G., & Aune, J. B. (2022). Effects of low-cost agricultural technology package on income, cereal surplus production, household expenditure, and food security in the drylands of Mali. AIMS Agriculture and Food, 7(1), 22–36. https://doi.org/10.3934/agrfood.2022002
- Smale, M., & Mason, N. (2014). Hybrid seed and the economic well-being of smallholder maize farmers in Zambia. The Journal of Development Studies, 50(5), 680–695. https://doi.org/10.1080/00220388.2014.887690
- Swanson, B. E., & Rajalahti, R. (2010). Strengthening agricultural extension and advisory systems: Procedures for assessing, transforming, and evaluating extension systems, the World Bank Agriculture and rural Development discussion paper 45,
- Teklewold, H., Gebrehiwot, T., & Bezabih, M. (2019). Climate smart agricultural practices and gender differentiated nutrition outcome: An empirical evidence from Ethiopia. World Development, 122, 38–53. https://doi.org/10.1016/j.worlddev.2019.05.010
- Tesfaye, W., & Tirivayi, N. (2018). The impacts of postharvest storage innovations on food security and welfare in Ethiopia. Food Policy, 75, 52–67. https://doi.org/10.1016/j.foodpol.2018.01.004
- Tigabu, D. G., & Gebeyehu, M. F. (2018). Agricultural extension service and technology adoption for food and nutrition security: Evidence from Ethiopia. Forum for Agricultural Research in Africa (FARA) Research Report, 3(4), 30.
- Ucar, Y., Ceylan, Z., Durmus, M., Tomar, O., & Cetinkaya, T. (2021). Application of cold plasma technology in the food industry and its combination with other emerging technologies. Trends in Food Science & Technology, 114, 355–371. https://doi.org/10.1016/j.tifs.2021.06.004
- Umair, M., Jabbar, S., Ayub, Z., Muhammad Aadil, R., Abid, M., Zhang, J., & Liqing, Z. (2022). Recent advances in plasma technology: Influence of atmospheric cold plasma on spore inactivation. Food Reviews International, 38(sup1), 789–811. https://doi.org/10.1080/87559129.2021.1888972
- Utuk, I. O., & Daniel, E. E. (2015). Land degradation: A threat to food security: A global assessment. Journal of Environment & Earth Science, 5(8), 13–21.
- Verkaart, S., Munyua, B. G., Mausch, K., & Michler, J. D. (2017). Welfare impacts of improved chickpea adoption: A pathway for rural development in Ethiopia? Food Policy, 66, 50–61. https://doi.org/10.1016/j.foodpol.2016.11.007
- Verner, D., Roos, N., Halloran, A., Surabian, G., Ashwill, M., Vellani, S., & Konishi, Y. (2021). Insect and hydroponic farming in Africa: The new circular food economy. World Bank Publications.
- Wekesa, B. M., Ayuya, O. I., & Lagat, J. K. (2018). Effect of climate-smart agricultural practices on household food security in smallholder production systems: Micro-level evidence from Kenya. Agriculture & Food Security, 7(1), 1–14. https://doi.org/10.1186/s40066-018-0230-0
- Willy, D. K., Zhunusova, E., & Holm-Müller, K. (2014). Estimating the joint effect of multiple soil conservation practices: A case study of smallholder farmers in the Lake Naivasha basin, Kenya. Land Use Policy, 39, 177–187. https://doi.org/10.1016/j.landusepol.2014.02.017
- Wooldridge, J. M. (2010). Econometric analysis of cross Section and panel data (2nd ed.). MIT Press.
- Wordofa, M. G., Sassi, M., & Yildiz, F. (2020). Impact of agricultural interventions on food and nutrition security in Ethiopia: Uncovering pathways linking agriculture to improved nutrition. Cogent Food & Agriculture, 6(1), 1724386. https://doi.org/10.1080/23311932.2020.1724386
- The World Bank. (2015). World Development indicators.
- The World Food Programme. (2023). A global food crisis. 2023: Another year of extreme jeopardy for those struggling to feed their families. https://www.wfp.org/global-hunger-crisis
- World Health Organization. (2020). The state of food security and nutrition in the world 2020: Transforming food systems for affordable healthy diets (Vol. 2020). Food & Agriculture Organization.
- Wossen, T., Alene, A., Abdoulaye, T., Feleke, S., Rabbi, I. Y., & Manyong, V. (2019). Poverty reduction effects of agricultural technology adoption: The case of improved cassava varieties in Nigeria. Journal of Agricultural Economics, 70(2), 392–407. https://doi.org/10.1111/1477-9552.12296
- Wudil, A. H., Usman, M., Rosak-Szyrocka, J., Pilař, L., & Boye, M. (2022). Reversing years for global food security: A review of the food security situation in sub-Saharan Africa (SSA). International Journal of Environmental Research and Public Health, 19(22), 14836. https://doi.org/10.3390/ijerph192214836
- Yilma, T., Berg, E., & Berger, T. (2015). Empowering African women through agricultural technologies: The case of irrigation technology in northern Ghana. Food System Dynamics, 6(2), 64–80.
- Zegeye, M. B., Fikire, A. H., & Assefa, A. B. (2022). Impact of agricultural technology adoption on food consumption expenditure: Evidence from rural Amhara Region, Ethiopia. Cogent Economics & Finance, 10(1), 2012988. https://doi.org/10.1080/23322039.2021.2012988
- Zeng, D., Alwang, J., Norton, G. W., Shiferaw, B., Jaleta, M., & Yirga, C. (2017). Agricultural technology adoption and child nutrition enhancement: Improved maize varieties in rural Ethiopia. Agricultural Economics, 48(5), 573–586. https://doi.org/10.1111/agec.12358
Appendix A:
Supporting Tables
Table A1. A Description of the variables by type for row planting technology
Table A2. Covariate balance indicators before and after matching: quality test
Table A3. Test of sensitivity analysis to hidden biases (Rosenbaum bounds test results)
Appendix B:
Figures
Figure B1. Propensity score distribution and common support for propensity score estimation of technology one: spacing.
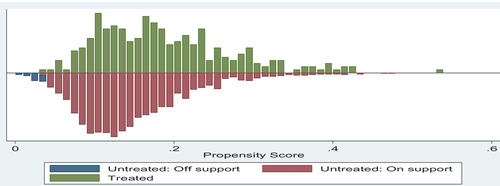
Figure B2. Propensity score distribution and common support for propensity score estimation of technology two: HYVs.
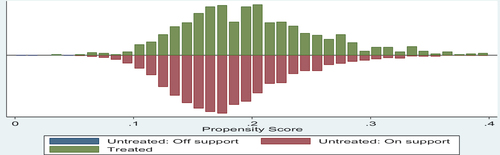
Figure B3. Propensity score distribution and common support for propensity score estimation of technology three: Fertilizers.
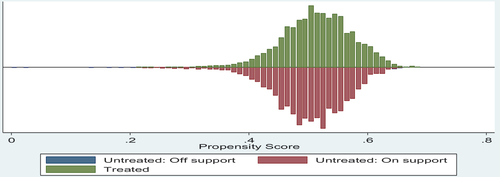