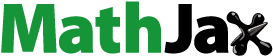
Abstract
In the face of increasing global unsustainable risks such as poverty, hunger, and pollution. Building sustainable agriculture (SA) in the digital age is a fundamental task for human survival. Based on the coupled coordination perspective, this paper constructs an SA system that takes into account more stakeholders by considering poverty alleviation and income increase (PI), food security (FS), and green agriculture (GA) as subsystems. The impact of digital technology on SA is systematically analyzed through data from 276 prefecture-level and above cities in China from 2005 to 2020. The study shows that digital technology has a significant upgrading effect on SA and its subsystems. And digital technology is more likely to promote SA in the developed eastern region and peripheral cities. Moreover, agricultural productivity and labor productivity play a mediating mechanism in the process of digital technology for SA. Digital financial inclusion fuels the high input process of digital technology for incentivizing SA, PI, and GA, but it cannot affect the highly technical process of digital farming. Further research found that the incentives of digital technology for SA and GA are characterized by the nonlinear characteristic of increasing marginal effects. Due to the second digital divide, there is a U-shaped incentive process of digital technology for PI to fall and then rise. Finally, the spillover nature of digital technology leads to spatial spillovers in its contribution to SA development.
1. Introduction
Failure of climate action, high incidence of extreme weather events, and collapse of ecosystems are considered the most severe set of risks among the top 10 global risks for the next decade. Meanwhile, a joint report by the Food and Agriculture Organization of the United Nations (FAO) and the World Food Programme (WFP), “Hunger Hotspots,” released on 6 June 2022, says the sustainability of the globally interconnected network of food and agriculture farmers is under threat. Climate change, COVID-19, and geopolitical conflicts have resulted in 53 countries and territories facing severe food crisis, resulting in a population needing emergency assistance that may climb to 222 million. Specifically, 180 million people still face food crises in countries such as Africa, where the natural environment is harsh, and Japan, South Korea, India, and China, where there are “too many people and too little land” (Li et al., Citation2014; Smil, Citation1999). In addition, the extensive use of agrochemical inputs such as fertilizers and pesticides to increase food production has become a common solution to address the population growth that may be faced in the long term (Roussaki et al., Citation2022). However, the current agricultural crisis and short-sighted coping strategies are not conducive to sustainable human development (Chandio et al., Citation2023). The FAO has summarized the major solutions to the current contradictions in agricultural production into three areas, namely, poverty eradication and income generation to eliminate all poverty, food security to eliminate all hunger, and low-carbon and environmentally friendly green agriculture (Caputo et al., Citation2020). This is the main topic in agriculture that promotes the goal of human sustainability, called Sustainable Agriculture (SA), and has become a common political topic of discussion around the globe.
The evaluation indicators of SA should have a practical guiding role, and some international organizations have already established SA evaluation indicator systems and successfully applied them in practice. On the one hand, FAO proposed the “Pressure-State-Response” (PSR) framework and developed the SA evaluation index system. On the other hand, since a significant portion of the United Nations membership consists of underdeveloped and developing countries, the FAO has thus increased its focus on equity and efficiency, especially about extreme poverty. In more developed agricultural systems, such as the European Union (EU) and the Organization for Economic Cooperation and Development (OECD) member countries, policy objectives and research orientations towards SA focus on areas such as energy efficiency, pollution reduction, and carbon reduction (Stevenson & Lee, Citation2001). In conclusion, based on the above authoritative definitions, this paper summarizes SA as a theoretical and practical paradigm covering the three pillars of poverty alleviation and income increase (PI), food security (FS), and green agriculture (GA).
In today’s digital era, with the widespread application of digital technologies in areas of agriculture represented by the Internet, the Internet of Things, big data, cloud computing, etc., new opportunities have been brought to SA. First, digital technology is characterized by scalability. This not only reduces the cost of communicating information but also supports farmers in realizing the rapid collection and linking of agricultural factors of production. It is important to note that digital technology not only facilitates the advancement of modern information processing technologies but also strengthens the competitive potential of farmers to access markets and increase off-farm employment opportunities (Nie et al., Citation2021). This revolution at the technological end of the spectrum has narrowed the information divide between farmers and citizens and reduced the urban-rural income gap, which provides the underlying impetus for the goal of poverty reduction (Park & Mercado, Citation2016; Zhang et al., Citation2023).
Secondly, in the face of the global food and nutrition crises, how to improve the security of the food supply is a topic that concerns the survival of humankind. Several countries are making mission-critical innovations to their food systems to achieve food and nutrition security. For example, the Netherlands has adopted a “circular agriculture” paradigm, Nicaragua has a strong focus on agroecology, and New Zealand has recently moved towards a “digital bioeconomy” (Klerkx & Rose, Citation2020). On 24 September 2014, the U.S. led the formation of the Global Alliance for Climate-Smart Agriculture (GACSA), which proposes to improve agricultural productivity and income with digital technology, which can help farmers in regions such as Vietnam increase their food production. FAO is launching its “Hand in Hand” action plan in 2019, one of which is to help 54 countries and territories with large proportions of people living in poverty to transform their agri-food systems securely through digital technology. The extensive utilization of new-generation digital technologies for refined cultivation, green grain storage, and intelligent production and processing provides technological security for the goals of food supply security and improved nutritional base (Mushi et al., Citation2022). On the one hand, IoT can be applied to monitor soil parameters and weather conditions; drones and smart glasses can be used for soil monitoring and sampling. These fine-tuned digital farming techniques help farmers identify and manage a wider cultivation area and increase yields per unit area (Trivelli et al., Citation2019). On the other hand, with the improvement in living standards, people pay more attention to food quality and safety (Wang et al., Citation2020). The digital intelligence program can be used for spoilage monitoring in food supply chain logistics to guarantee the quality and safety of food distribution (Tsolakis et al., Citation2019).
Thirdly, long-term crude agricultural development has brought about a severe situation of tightening resource constraints, ecological degradation, and soil degradation. Although ecosystems act as a direct bridge between agriculture and human society, expanding arable land areas has a crowding-out effect on other species due to population growth and increased industrialization (Balmford et al., Citation2005). Pesticides and fertilizers with long-term polluting and destructive effects are widely used to alleviate the conflict between humans and nature, increase agricultural yields per unit area, and enhance soil fertility (FAO, Citation2009; García et al., Citation2020; Lal, Citation2010). However, considering the existence of an environmental crisis and an agricultural crisis, the objectives of modern agriculture cannot be identified simply in terms of economic dimensions, such as income and output, but also by focusing on the environmental externalities arising from agricultural activities (Dunlap et al., Citation1993; Knickel et al., Citation2017). This is because green agriculture is more in line with the global sustainable development goals and the concept of carbon neutrality (Qin et al., Citation2022). The information-cascading ability of digital technology eases the natural contradiction between agricultural development, ecological resources development and utilization, and human survival, providing new ideas for green agriculture (Guo et al., Citation2022).
At a time when the global crisis is gradually intensifying, poverty alleviation and income increase for the majority of farmers, food and nutritional safety and security, and the protection of the ecological environment have become bottom-line issues that cannot be ignored. Therefore, a systematic and organically coordinated evaluation system for SA that includes the above elements needs to be constructed urgently. At the same time, digital technologies are driving profound changes in human society and agricultural systems in several areas. However, the question of whether digital technologies can create a stable and balanced dynamic for SA and what challenges exist needs to be identified and analyzed in depth during the research process. This is because the long-term strategic value of whether digital technology can drive SA and thus support the flourishing of human civilization must be answered.
This study has the following three marginal contributions. First, we explore and develop a system of SA evaluation indicators that accommodates a broader range of stakeholders. Based on data from 276 cities above the prefecture level in China from 2005 to 2020, we explore indicators that can support SA from three subsystems: poverty alleviation and income increase, food security, and green agriculture. Second, we draw on the idea of harmony to respond to the level of development of SA by measuring the degree of coupled coordination among the three subsystems. Unlike the simple cumulative approach to measuring SA integrated performance alone (Gómez-Limón & Sanchez-Fernandez, Citation2010; Mili & Martínez-Vega, Citation2019; Van Cauwenbergh et al., Citation2007), our methodology is significant in realizing the goal of an organic SA in which systems are coordinated and progress in a balanced manner. Thirdly, we not only find that digital technology has a driving effect on the positive cycle of PI-FS-GA but also further isolate the positive incentive process of increasing the “marginal effect” of digital technology on SA and GA through the threshold effect, as well as the U-shape incentive process on PI. The above nonlinear conclusion expands the application boundary of digital divide theory in the research field of SA.
The rest of the paper is organized as follows. The second part is the Literature background, which focuses on the literature review and theory combined related to the impact of digital technology on PI, FS, GA, and SA, based on which the corresponding research hypotheses are proposed; the third part describes the model design, indicator selection, and data sources, including the construction of mediator models, panel thresholds, and spatial panel econometric models. The fourth part presents the results and analysis of empirical tests, including benchmark regression, robustness test, nonlinear effect test, regional heterogeneity analysis, mechanism effect test, and spatial spillover effect analysis. The fifth part summarizes and discusses the study results and provides policy recommendations based on the findings.
2. Literature background
2.1. Digital technology and PI
The level of economic development varies widely between countries, and yet every political group has made it their political vision to work towards the elimination of internal poverty and income disparity (Wang et al., Citation2022). In developing countries with large populations and agricultural pressures, digital technology is building a new social form in which everything is interconnected and giving digital opportunities to lift the disadvantaged groups, represented by farmers, out of poverty and de-marginalize them, and driving the sustainable development of agricultural activities (Simone & Christopher, Citation2003). From the perspective of technological innovation, digital technology has the innate advantages of inter-temporal information dissemination, data creation, reduction of transaction costs, sharing, etc., which dramatically breaks down the spatial limitations of agricultural development, eases the contradiction between supply and demand during the activities of agriculture, and achieves the purpose of balancing fairness and efficiency (Chantarat & Barrett, Citation2012). A more accessible environment for agricultural development further motivates farmers and raises the upper limit of their income (Mushi et al.,). First, digital technology reduces information asymmetry between farmers and markets, helping farmers obtain more comprehensive information about market transactions and providing pricing power and income potential (Driouchi et al., Citation2006). Second, in the age of information of everything interconnected, new ideas, knowledge, and technology open sharing rights to farmers, promote the enthusiasm of farmers for Internet entrepreneurship, provide non-farm jobs, and increase the income of rural laborers (Chantarat & Skuterud, Citation2004; Li et al., Citation2023). Thirdly, as ICTs have made financial services more accessible to farm households, support from finance has had a significant impact on economic development, thus contributing to poverty alleviation (Zhang & Liu, Citation2015). In conclusion, digital technology can effectively reduce the cost of information between farmers and other social actors, enriching agricultural social networks and contributing to farmers’ poverty alleviation and income increase through three main channels: sharing resources, improving efficiency, and creating opportunities (Leng et al., Citation2020).
It is worth cautioning that the findings of Forman et al. (Citation2012) suggest that for regions with relatively weak educational bases and low population densities, the construction of digital infrastructures has little or no impact on incomes. Even if the government provides appropriate policy subsidies, such as subsidizing digital infrastructure in terms of human and physical capital, the impact on residents’ income may be limited. In sum, digital technology has a limited impact on incomes in rural areas with low population densities and a relatively weak educational base.
2.2. Digital technology and FS
Since eradicating hunger and ensuring food security is one of the United Nations 2030 targets for sustainable development (UN, 2015), a secure food supply is of great significance to the sustainable development of human beings (Lin et al., Citation2022). Among them, integrating digital technology with the whole food industry chain and thus ensuring food security has become a new task in the intelligent era.
The specific ways in which digital technology empowers food security are as follows: First, the spread of digital technology and the layout of the Internet of Things (IoT) in agriculture have allowed farmers to increase their technical knowledge of agricultural production through ICTs, enabling them to use production inputs such as fertilizers and pesticides more efficiently (Hong et al., Citation2019; Lawin & Tamini, Citation2019). That is, digital technology fosters a pool of scientific and technological talent for modern food production, and they are the new breakthrough point for improving the efficiency of food production (Klerkx & Rose, Citation2020). Secondly, the application of digital technologies such as smartphones, robots, blockchain, 5 G, AI, etc., has facilitated the construction of intelligent and refined agriculture, thus enhancing the comprehensive production capacity of agriculture and improving the overall efficiency of the food industry (Leng et al., Citation2020). Ma and Wang (Citation2020) study also concluded that the use of the Internet allows farmers to learn about the use of seeds and fertilizers more quickly, which has a positive impact on increased food production. Third, digital inclusive finance alleviates the problems of difficult, expensive, and cumbersome financing faced by farmers (Munyegera & Matsumoto, Citation2018) and advances the development of agricultural insurance (Hu et al., Citation2022), increasing farmers’ incentives to grow food and bringing about an increase in food production (Wang et al., Citation2018). In conclusion, there are complex mechanisms of influence between digital technologies and food production, and a study by Chandio et al. (Citation2023) suggests that there is even a bidirectional causal relationship between the two.
2.3. Digital technology and GA
Economic development and environmental protection have always been hotly debated topics. In particular, in the Kuznets environmental curve, there is a U-shaped relationship between environmental protection and economic growth, i.e., the goal of a sustainable environment requires a sustainable economy as a foundation. Embedding this theory in agricultural systems, green agriculture becomes a derivative consequence of increased food production and income from poverty. This judgment is supported by the study of Jiang et al. (Citation2022), who first argued that digital technology has facilitated the development of the rural digital economy. Secondly, it is believed that digital technology, with its inherent advantages of higher permeability, more powerful marginal effect, and more prominent network externalities, can realize the green agricultural development model of “traceable quality and measurable environment” through efficient interconnection and intercommunication with agriculture production, operation, and consumption.
The greening effect of digital technologies affecting agriculture is threefold. First, greening in the food production process, i.e., emphasizing the reduction of pesticide, fertilizer, and film use, source management, and the development of environmentally friendly farming models (Sanders, Citation2006). At this time, digital technology can optimize the early warning system of pollution sources and improve the efficiency of carbon reduction and emission reduction through the accurate monitoring of environmental data resources, such as air quality, river water quality, pollution discharge, and carrying capacity of the environment (Zhong et al., Citation2022). Specifically in the planting process, digital technology can accurately analyze the ecological conditions, environmental capacity, and resource status of each area, thus determining the optimal amount of fertilizer and agricultural chemicals and realizing a dynamic balance between agricultural production and the protection of the ecological environment (Robinson et al., Citation2022). Secondly, green agriculture focuses on green and pollution-free food production, processing, and transportation to achieve high efficiency and sustainability (Struik & Kuyper, Citation2017). Digital technology can effectively integrate various information resources into production decision-making to guarantee the supply of green agricultural products, reduce the waste of resources and the emission of pollution, and realize the efficiency and greenness of the production process (Hampton et al., Citation2013). Thirdly, the application of digital technology has integrated with the financial sector and given birth to digital inclusive finance, which has encouraged the green development of agriculture from the port of environmental cost expenditure (Guo et al., Citation2022).
In industrialized agrarian societies, small farmers are the fundamental form of organization of agricultural production, and their environmental actions require a degree of economic grounding. That is, through redundant resources for the development and application of green technologies, thus breaking the Kuznets trap of agricultural organization. However, digital technology can be a double-edged sword, potentially increasing food production and farm incomes on one side and exacerbating environmental damage on the other (Hall & Vredenburg, Citation2003). Despite the catalytic role of digital technologies in enhancing agricultural productivity, the use of digital technologies may increase energy consumption and new emissions, and the increase in production capacity may also bring indirect environmental pressure (Belkhir & Elmeligi, Citation2018; Lange et al., Citation2020; Malmodin & Lundén, Citation2018). In addition, Khattak et al. (Citation2022) argue that governments relax environmental regulations when economic indicators such as employment and income are poor, so low-cost, high-polluting technologies are more likely to be adopted, thus ignoring the green dynamics in digital technologies, which ultimately leads to an increase in pollutant emissions. In this paper, we will further verify, at the level of emissions and pollution treatment, the environmental impact of digital technologies in agriculture.
2.4. Digital technology to enhance SA systems
When placing the production safety of food, farmers’ poverty alleviation and income increase, and the greening of agriculture in the systematic framework of sustainable agriculture, first of all, we should make it clear that SA is a Complex Adaptive System (CAS). Farmers, as Adaptive Agents, can learn or accumulate experience through repeated exchanges with the environment as well as other subjects, and this interaction drives the development of the agricultural system (Wang et al., Citation2020). Thus, the process of farmers learning and applying digital technologies and improving their incomes cannot be achieved without the nutrients and raw materials that food provides us with, and even more so without the ecosystems that safeguard us throughout the process (Chandio et al., Citation2022; Liu & Zhou, Citation2023). On the one hand, it needs to be made clear that food-for-green agricultural production methods, such as intermittent farming and returning farmland to forests, will run counter to the demand for land and resources by a growing population (Roussaki et al., Citation2022). Therefore, in the context of the imbalance between food supply and demand, green agriculture should replace polluting farming practices through more environmentally friendly technologies and products while guaranteeing increased food income for farmers (Shin & Choi, Citation2015). Digital technology can increase the yield per acre area, improve food supply, and reduce dependence on land (Phalan et al., Citation2011). Intelligent and precise farming techniques, which reduce the misuse of chemical fertilizers and pesticides and lower the environmental burden while improving yields and incomes, are all pathways to green agriculture (Balmford et al., Citation2018). From another perspective, poverty is seen by the UN as the greatest unsustainability, exacerbating the destruction of and competition for nature. In turn, environmental crises can trigger crises in food cultivation, leading to a return to poverty brought about by hunger, creating a vicious cycle of unsustainability (Chandio et al., Citation2023).
In short, in the SA system, FS and PI are the prerequisites for the practice of GA, while GA is the ecological substrate that nourishes the first two. Currently, digital technologies that bring about improvements in agricultural productivity (Hrustek, Citation2020) and increase the coverage area of financial inclusion (Munyegera & Matsumoto, Citation2018) will inevitably have an impact on SA and its subsystems (Nfu, Citation2019). When digital technology advances one of these subsystems, it is equivalent to promoting the synergistic rise of all three subsystems, ultimately realizing the advancement of the entire SA system (Hickey et al., Citation2019; Klerkx et al., Citation2019). The system logic of Digital Technology for SA is shown in Figure .
Although scholars have found evidence from different fields that digital technology plays an essential incentive role in areas such as farmers’ entrepreneurship to increase incomes (Li et al., Citation2023), cereal yields (Chandio et al., Citation2022, Citation2023), and improving the productivity of agriculture (Trivelli et al., Citation2019). These research orientations focusing on agriculture to meet the short-term needs of the present generation ignore the long-term strategies for the survival of future generations in the face of the problem of agriculture in support of sustainable human development. According to the sustainability theory, we need to start from the principle of intergenerational equity and focus on the equitable, efficient, coordinated, and multidimensional sustainable development of agriculture (Jiang et al., Citation2022; Kamble et al., Citation2020; Luan et al., Citation2023). Therefore, this paper incorporates the three PI-FS-GA into an efficient and fair system to establish an SA system that is beneficial to the elimination of all poverty, the eradication of all hunger, the realization of harmonious coexistence between human beings and nature, and that has the value of intergenerational equity. At the same time, based on the idea of multi-dimensional perception, we explore whether the popularization and application of digital technology can promote the progress of a coordinated and comprehensive agricultural system.
In summary, the coupled coordination of the three subsystems is considered the comprehensive development level of SA, and the following research hypotheses are formulated by considering efficiency and financial inclusion as the influencing mechanisms:
H1:
Digital technology enhances SA.
H2:
Labor productivity, labor productivity, and financial inclusion can influence the effect of digital technology on SA enhancement.
3. Research design
3.1. Modeling
3.1.1. Base regression model
To test the above research hypotheses, the following basic model is first constructed for the
In Equationequation (1)(1)
(1) , the
is the level of agricultural sustainability of city i in period t, and
is the digital technology level indicator of city i in period t, and the vector
represents a series of control variables;
represents the individual fixed effects of city i that do not vary over time, and
then controls for time fixed effects;
represents a randomized disturbance term. Furthermore, in Equationequation (2)
(2)
(2) , the
is the poverty alleviation and income increase situation of city i in period t; EquationEquation (3)
(3)
(3)
is the food security situation of city i in period t; and in Equationequation (4)
(4)
(4)
is the green agriculture development level of city i in period t.
3.1.2. Mechanism testing models
In addition to the direct effects captured in equations (1)-(4), to discuss the possible mechanisms of action of digital technology for SA and its subsystems, labor productivity (Labor_rate) and agricultural productivity (Tecniq) are tested to see if they are mechanisms of action between the two. The specific test steps are as follows: based on the significance of the coefficients of digital technology on the explanatory variables SA, PI, FS, and GA passing the test, construct the linear regression equation of digital technology on the mediator variable M, as well as the regression equation of digital technology and the mediator variable M on the explanatory variables. Subsequently, the significance of the relevant regression coefficients was used to determine whether mediating effects existed. Among them, the mediating variable M contains two variables Labor_rate and Tecniq, and to simplify the expression, the four explanatory variables SA, FS, and GA are grouped into Y, and regression tests are conducted separately. The specific form of the above regression model is set as follows:
3.1.3. Threshold effect models
Considering that there may be nonlinear effects of digital technology on SA and subsystems as the level of digital technology development increases, this paper constructs a panel threshold model to test this:
The threshold variable in EquationEquation (7)(7)
(7) is
,
(·) is an indicator function that takes the value of 1 or 0, which is 1 if the condition in parentheses is satisfied, and 0 otherwise. EquationEquation (7)
(7)
(7) considers a single-threshold scenario, which can be expanded to a multi-threshold scenario based on steps such as econometric testing of the sample data.
3.1.4. Spatial measurement models
To further discuss the spatial spillover effects of digital technology on SA, a spatial interaction term between the two and the control variables is introduced in equation (1), which is further extended into a spatial panel econometric model:
EquationEquation (8)(8)
(8) includes the spatial interaction terms of the explained and explanatory variables and is known as the Spatial Durbin Model (SDM). Where ρ represents the spatial autoregressive coefficient, and W is the spatial weight matrix, to improve the robustness of the empirical results, this paper adopts three regression methods: geographic distance matrix, economic distance nested matrix, and adjacency matrix.
and
are the elasticity coefficients of the core explanatory variables as well as the spatial interaction terms of the control variables.
3.2. Measurement and description of variables
3.2.1. Measurement of sustainable agriculture
We are based on the FAO’s definition of the connotation of SA, as well as scholars’ related research and measurement methods (Gómez-Limón & Sanchez-Fernandez, Citation2010; Mili & Martínez-Vega, Citation2019; Van Cauwenbergh et al., Citation2007). Combining the gaps in current research, this paper measures SA through three secondary indicators of poverty alleviation and income increase, food security, and green agriculture, and the multidimensional indicator system is in Table . First, poverty alleviation and income increase, food security, and green agriculture were measured separately for 276 cities at the prefecture level and above in China from 2005 to 2020 by the entropy weight-TOPSIS method. Secondly, the coupling coordination degree of the three subsystems is obtained through the coupling coordination model① as a proxy variable for SA. The specific indexes are set as follows:
Poverty alleviation and income increase. Poverty is one of the significant factors in the unsustainable crises facing the globe. Placing poverty alleviation and income increase under the SA framework not only follows the principle of setting the UN Sustainable Goals but also highlights the importance of eradicating poverty on a global scale. This paper measures poverty alleviation and income increase in three dimensions: living conditions, production efficiency, and economic performance.
Food security. A secure food supply is the basis for the stable development of agricultural systems and human societies. Referring to the studies of scholars (Cai et al., Citation2020; Zhang et al., Citation2021; Zhou et al., Citation2020), this paper measures food security in terms of resource base, production resilience, and structural rationality.
Green agriculture. Chen et al. (Citation2022) argued that green agriculture implies green development connotations such as ecological protection, pollution reduction, and carbon reduction. Accordingly, this paper uses environmental protection, pollution treatment, and agricultural carbon emissions to constitute a green agriculture indicator, reflecting the inputs and outcomes of environmental management in agriculture in each region.
Table 1. SA evaluation indicator system
3.2.2. Measuring the level of digital technology development
As far as the existing research is concerned, the evaluation methods for digital technology mainly include the keyword word frequency count method, the single index evaluation method, and the multi-dimensional index system evaluation method. Since digital technology is an integrated technology application system, evaluation from a multidimensional perspective will be more comprehensive and accurate. This paper draws on the OECD definition of digital technology, considering that the Internet, information infrastructure, and technological innovation are indispensable for digital technology (OECD, Citation2019). Therefore, digital interconnection, digital informatization, and digital technological innovation are used as secondary indicators to measure the level of development of digital technology and are measured by the entropy weight-TOPSIS method. Specific indicators are shown in Table .
Table 2. Indicators for measuring the level of digital technology development
3.2.3. Control variables
To more fully analyze digital technology spillovers in SA, it is also necessary to set control variables that may affect SA.
The city-level control variables are specified below: teachers’ qualifications (Tech): expressed as the proportion of full-time teachers in rural compulsory education schools with a bachelor’s degree or higher; Human capital (Edu): expressed in terms of the average number of years of schooling of rural residents; physical capital (House): expressed in terms of the per capita housing area of rural residents; water: expressed in terms of the penetration rate of safe drinking water; infrastructure (Road): expressed in terms of the rate of hardening of village roads.
The control variables at the provincial level are specified below: provincial agricultural development (Lnargri): expressed as the logarithm of the province’s total agricultural output value for the year; income disparity (Gini): indicated by the Gini coefficient for the year; degree of policy support (RD): expressed by the intensity of the province’s R&D investment for the year; level of science and technology (Tecniq): indicated by the province’s marketization of technological achievements for the year.
3.3. Data sources and descriptive statistics
In this paper, a study is conducted for 276 cities at prefecture level and above in China, and 2005–2020 is selected as the study period, resulting in a final balanced panel data for 4416 city-years. The data for the study were obtained from the China Urban Statistical Yearbook, China Regional Economic Statistical Yearbook, statistical annual reports of some prefecture-level cities, and the Wind Information Database.
Table shows the descriptive statistics of the main variables in this paper. The results showed that the mean value of SA was 0.7195, the maximum value was 0.8439, the minimum value was 0.6291, and the standard deviation was 0.0250, indicating some differences in SA between regions. Digital, Labor_rate, and Technique present small mean values and a large gap between the minimum and maximum values. Among the control variables, there are significant differences across regions in Tech, Edu, House, Water, Road, Lnargri, and RD.
Table 3. Results of descriptive statistics of variables
4. Empirical tests of the impact of digital technologies on sustainable agriculture
4.1. Baseline regression results
To test whether hypotheses H1, we reflect the results of the underlying regression models (1)-(4) through Table . In columns (1) and (2), the estimated coefficients of the core explanatory variable Digital are significantly positive at the 1% level, indicating that digital technology promotes SA and hypothesis H1a is tested.
Table 4. Benchmark regression results of digital technology affecting sustainable agricultural development and subsystems
From columns (3)-(8), it is found that the estimated coefficients of Digital are all significantly positive, i.e., digital technology can positively affect poverty alleviation and income increase, food security, and green agriculture, supporting hypothesis H1b.
By comparing the coefficients of Digital, we find that digital technology has significant differences in the excitation results of different subsystems of SA, which are GA, PI, and FS in descending order. First, digital technology is more likely to produce a green effect in the production of agriculture and give play to the pollution reduction and carbon reduction function of digital technology. Unlike Lange et al. (Citation2020), who argue that digital technology is not conducive to energy efficiency, we find evidence of energy savings from digital technology in the agricultural sector. Secondly, the application of digital technology enhances farmers’ access to income-generating channels and the sharing of public resources, as well as the quality of life of farmers, to lift them out of poverty and increase their incomes. Thus, it seems that our study finds the income-enhancing effects of digital technology out of poverty from a more systematic perspective, which is a further enrichment of Li et al. (Citation2023) who found the income-motivating effects of digital technology only at the level of farmers’ entrepreneurship. Thirdly, on the one hand, digital technologies have certain professional thresholds and input cycles, thus leading to a slower process of transforming them into modern agricultural technologies and advancing food production. On the other hand, because the second digital divide is more likely to occur in rural areas, the ability of farmers to access, process, and create digital resources lags behind that of citizens, resulting in a digitalized food production system that is very slow to increase yields. Our findings, while supporting the conclusion that digital technology increase food production (Chandio et al., Citation2022), go further to identify the invisible resistance that the digital divide creates in the food production process (Luan et al., Citation2023; Millan et al., Citation2021).
4.2. Robustness tests
4.2.1. Controlling for fixed effects
Cities with higher-quality agricultural development will also prioritize the development of the Internet and thus have a “first-mover advantage” in the application of digital technology, making the causality judgment in the empirical part of this paper face endogenous problems. This is done by setting provincial fixed effects to mitigate changes in the macro-systemic environment that may result from the widespread development of digital technology. The results in columns (1)-(4) of Table suggest that the previous findings of the article remain robust after accounting for systematic changes in macro factors.
Table 5. Robustness test of digital technology affecting sustainable agricultural development
4.2.2. Substitution of explanatory variables
Considering that this paper uses a system coupling coordination degree for the SA composite measure, which is different from previous studies (Gómez-Limón & Sanchez-Fernandez, Citation2010; Mili & Martínez-Vega, Citation2019; Van Cauwenbergh et al., Citation2007), there may be some bias. Therefore, in this paper, all the indicators of SA are re-measured by the entropy weight-TOPSIS method to obtain a brand new result, which is denoted as SA1. The results in columns (5)-(6) of Table show that after replacing the explanatory variables, the regression results remain consistent with the previous findings.
4.2.3. Instrumental variable (IV) approach
Selecting appropriate instrumental variables for the core explanatory variables is the primary method for solving the endogeneity problem. The instrumental variable we have chosen is a distance-type variable calculated using a geographic information system (GIS), which is the spherical distance between the host city and Hangzhou. This instrumental variable correlates with the degree of digital technology in the region. First of all, it is well known that the development of digital finance represented by Alipay originated in Hangzhou, so Hangzhou is in a leading position in terms of Internet development, and it can be expected that the closer it is to Hangzhou geographically, the better the degree of Internet development should be, and the higher the level of digital technology. Second, we must also account for the homogeneity of this instrumental variable, i.e., it does not satisfy the Exclusion Restriction (ERC) through some omitted variables, particularly the level of economic development, which affects the SA. Because Hangzhou is only one of the important cities in China’s economic development②, a smaller distance from Hangzhou does not imply a higher level of economic growth and a better level of SA development, and homogeneity is approximately satisfied. It should be noted that the raw data for the instrumental variables chosen are in cross-sectional form and cannot be used directly in the econometric analysis of panel data. Referring to Nunn and Qian’s (Citation2014) treatment of this problem, a time-varying variable is introduced to construct the panel instrumental variable. Specifically, the number of Internet broadband accesses in the previous year is cross-multiplied with the spherical distance from each city to Hangzhou, respectively, as an instrumental variable for the city’s digital technology development index in that year.
The results in columns (7) and (8) of Table show that the effect of digital technology on enhancing SA still holds after accounting for endogeneity, with the results significant at least at the 5% level. In addition, for the test of the original hypothesis “insufficient identification of instrumental variables”, the p-value of Kleibergen-Paap rk’s LM statistic is 0.000, which is a significant rejection of the original hypothesis; In the test of weak identification of instrumental variables, the Wald F-statistic of Kleibergen-Paap rk is greater than the p-value of the Stock-Yogo weak identification test by 10%. Overall, the above tests justify the selection of the cross term between the number of Internet broadband accesses in the previous year and the spherical distance from each city to Hangzhou as the instrumental variable for digital technology.
4.3. Mechanism analysis
4.3.1. Test of mediating effects of labor productivity, agricultural productivity
Digital technology not only enhances the efficiency of resource allocation in agricultural society but also contributes to the improvement of agricultural productivity and productivity of agricultural labor (Chantarat & Barrett, Citation2012), which plays a significant role in achieving the goal of sustainable agricultural development (Zhou et al., Citation2022). Therefore, this paper explores the mediating mechanism of digital technology affecting SA in terms of agricultural productivity and productivity of agricultural labor. Among them, agricultural productivity (Technique) is measured by the total power of agricultural machinery; agricultural labor productivity (Labor_rate) is measured by the ratio of the value added of the primary industry to the total sown area of crops.
In summary, it is significant to analyze the transmission mechanism of the impact of digital technology on SA from the perspective of labor production rate and agricultural productivity. In this paper, we choose the mediation effect model (5) and (6) to test, and the results are shown in Table .
Table 6. Mechanism test of digital technology affecting SA development
First, in column (1) of Panel A, the regression coefficient of Digital is significantly positive below the 1% level, indicating that digital technology enhances labor productivity. Secondly, from column (2) of Panel A, it is found that the coefficients of Labor_rate are all significantly positive, while the Sobal test is significant. It indicates that labor productivity increase is the mediator of the role of digital technology in promoting the level of SA. Finally, further analysis of columns (3)-(5) in Panel A shows that digital technology can increase labor productivity and drive PI, FS, and GA through this path. Taken together, digital technology can create positive incentives for the subsystems of SA through the path of labor productivity enhancement, which ultimately supports the comprehensive progress of SA. In Panel B, we find similar conclusions to those in Panel A. Digital technology can positively incentivize the subsystems of SA through the pathway of enhancing agricultural productivity (Technique), which ultimately supports the comprehensive progress of SA.
Digital technology reduces the use of pesticides and fertilizers and increases economic benefits by increasing the capacity and efficiency of agricultural production (Überbacher et al., Citation2019). On the one hand, the information-based precision farming model reduces the damage to soil and the environment caused by agricultural activities and promotes the green development of agriculture (Chen et al., Citation2020; Meena et al., Citation2020). On the other hand, high-performance digital technologies drive up food production and increase farm incomes. Moreover, this impact is not independent; digital technology will simultaneously enhance PI, FS, and GA and have a coordinated upward sustainable effect on agriculture (Ali & Dahlhaus, Citation2022).
4.3.2. Tests of the moderating effect of digital financial inclusion
Through the previous analysis, we find that finance will always function as a booster in the field of SA. To this end, we further verify whether finance functions as a mechanism as digital technology affects SA. To further focus on the sustainable effects of digital technology, we refer to the Peking University Digital Inclusive Finance Index (2011–2021) and use digital inclusive finance as a moderating variable, denoted as Finance. Regression results for moderated effects are in Panel C of Table .
From columns (2), (3), and (4) of Panel C, the coefficients on the interaction term Finance*Digital are all significantly positive. This suggests that digital financial inclusion has encouraging effects, such as improving the capital constraints of farmers and providing the cost of applying green technologies, which have contributed to the level of digital technology for PI, GA, and SA as a whole. However, since FS is a process that is highly dependent on technological innovation (Chandio et al., Citation2023), it is clear that financial capital cannot play a role in the specific production processes of digital agriculture.
4.4. Regional heterogeneity tests
This is even though we find nonlinear incentives for digital technology to have different marginal effects on SA and subsystems at various stages of development in the threshold effect test. However, the fact is that there is an imbalance in the level of economic development between regions, as well as differences in the level of digital infrastructure. Therefore, the digital divide may lead to regional heterogeneity in the results of the impact of digital technology on SA. Therefore, we make further heterogeneity tests based on geographic location and city class as the basis of grouping. On the one hand, two groups of samples are divided into eastern, central, and western cities. On the other hand, we include municipalities, sub-provincial cities, and provincial capitals as center cities and the rest as peripheral cities according to the city class. Separately, the results of the heterogeneity test are in Table .
Table 7. Test of regional heterogeneity in the impact of digital technology on SA development and subsystems
Based on the geographic location heterogeneity test in Panel A. The results in columns (1), (2), (7), and (8) indicate that digital technology contributes significantly more to SA and GA in the East than in the Midwest. This is similar to our findings in the nonlinearity test, mainly that the eastern region has a more developed digital infrastructure and is more likely to drive SA and GA through digital technology. The results in columns (3), (4), (5), and (6) show that digital technology has a significant role in contributing to PI and FS in the main agricultural production areas of the central and western regions where land resources are more abundant. However, in the eastern part of the country, where there is more land and less land, digital technology has no significant role in securing PI and FS.
In Panel B, the tests are grouped by city class. The results show that digital technology is more likely to positively incentivize SA, PI, and GA in peripheral cities. However, this incentive is not significant for central cities. This result may be explained by the fact that central cities are mainly the hubs of the State in organizing economic activities and allocating resources, the hubs of the State’s comprehensive transportation and information network, the State’s scientific, educational, cultural and innovation centers, and the “spire cities” with the most comprehensive strengths in the State’s urban system, and that their agricultural development is not the most important in comparison with that of the peripheral cities. Therefore, compared with the center city, the integration of digital technology and agricultural development in peripheral cities is closer, making the dividends of digital technology-enabled SA released more fully.
5. Further analysis
5.1. Non-linear impact analysis
Metcalfe’s law that the value of a network is the square of its number of users is validated globally (Zhang& Xu, Citation2023). It is worth exploring whether there is such a nonlinear trend in the impact of digital technology in SA systems as farmers access the network. To this end, we use a panel threshold model to test this. Based on Hansen’s (Citation1999) method, the existence of the threshold effect was tested after 300 iterations of sampling via bootstrap, and the specific results are shown in Table .
Table 8. Regression results of the model of digital technology affecting SA and subsystem thresholds
In column (1), with SA as the explanatory variable, Digital passes the single-threshold test but fails the double-threshold test. The results of the single-threshold regressions show that the contribution of digital technology to SA is characterized by a significant “marginal effect” of increasing nonlinearity. That is, the higher the level of digital technological development, the stronger the incentive effect on SA. Meanwhile, we find similar findings in the threshold effect test in column (3), where digital technology has a marginal incremental boost to GA. Stated differently, the cost of digital technology inputs that drive SA or GA goals has a law of diminishing marginal effect as digital technology becomes more deeply integrated with agriculture. Our conclusion is the same as that of Jiang et al. (Citation2022) at the GA level, which we further enrich at the SA level by validating the application of Metcalfe’s law in agriculture in multiple dimensions.
In column (2), Digital significantly passes the double threshold test. Yet Digital has a U-shaped incentive process for PI that decreases and then increases. This result suggests that digital technology has a nonlinear trajectory of change that first hinders and then facilitates income increase out of poverty. First, at the early stage of the popularization of digital technology development, due to the emergence of the second digital divide, it is difficult for digitally illiterate farmers to utilize digital technology to process information resources, which reduces their relative competitive advantage in the digital era. First, at the early stage of the popularization of digital technology development, due to the emergence of the second digital divide, it is difficult for digitally illiterate farmers to utilize digital technology to process information resources, which reduces their relative competitive advantage in the digital era. On the one hand, this leads to their lack of timely access to digitized public services, and on the other hand, it leads to their inability to earn a new type of professional income in society. At the initial stage, as farmers have low literacy and digital adoption capacity (Hidalgo et al., Citation2020), the overall digital literacy of farmers is enhanced as digital information technology is gradually accepted and mastered by farmers. As a result, farmers can not only access public services such as digital healthcare and education but also realize job search and entrepreneurship through digital platforms (Li et al., Citation2023). This has safeguarded the right of farmers to enjoy social equalization services on the one hand and expanded their income-generating channels on the other. Therefore, digital technology is not a panacea for farmers’ poverty alleviation and income increase, which is probably a source of moral hazard.
5.2. Analysis of spatial spillover effects
First, an essential feature of digital technology is that it supports the enhancement of the breadth and depth of interregional linkages in economic activities through efficient information transfer, thereby compressing spatial and temporal distances. Yilmaz et al. (Citation2002) focused on spatial spillovers from informatization by empirically examining panel data from 48 U.S. states. Keller (Citation2002), on the other hand, adds a discussion of the spillover distance from the perspective of knowledge and technology diffusion. In addition, the production methods of food (Zhang et al., Citation2021), the practices of green agriculture (Shen et al., Citation2020; Wu et al., Citation2013; Xu et al., Citation2020; Zhou et al., Citation2019), are strongly spatially correlated. Therefore, the impact of digital technologies on SA systems will likely have further spatial spillovers, which is an essential inspiration for exploring the issue of regional development divides in the era of the Internet of Everything.
In particular, the methodology requires a spatial autocorrelation test for the digital technology development index and the SA index. Therefore, we calculated the spatial effects for each year under the geographic distance matrix using the Moran’I index method, as shown in Table . The results show that the Moran’I index of the level of development of digital technology and the level of development of SA under the weight of geographic distance from 2005 to 2020 have reached the significance level of 1%, which indicates that the digital technology and the sustainable development of agriculture in each region have significant spatial autocorrelation, that is, there is a phenomenon of clustering of the two in the spatial distribution.
Table 9. Characterizing the “spread” of digital technology and SA, 2005–2020
Second, the spatial spillover effects of digital technologies on SA are examined. Table reports the results of the regressions under three different spatial weight matrices. Based on the idea of Elhorst (Citation2014), the LM test, SDM model fixed effects, Hausman test, and SDM model simplified test were used sequentially. The SDM model with spatio-temporal double fixed effects was finally determined to be the optimal choice. To increase the robustness of the estimation, we adopt a spatial lag model (SLM) with spatio-temporal double fixed effects for the test. The results in Table show that the spatial autoregressive coefficient of SA in the SDM model is significantly positive, and the coefficient of the spatial interaction term of Digital is also positive, which indicates the spatial existence of not only exogenous interaction effects of digital technology but also endogenous interaction effects of SA in the sample cities. However, the values of the regression coefficients of the spatial interaction terms cannot be used directly to discuss the marginal impacts of digital technology on SA, because analyzing spatial spillovers between regions through simple point regression results would produce erroneous estimates. Therefore, it is necessary to explain the impact of digital technology on SA in the region as well as in neighboring areas using a partial differential explanation of the change in variables, i.e., using both direct and indirect effects, as can be seen in Table , which shows that there is a significant presence of indirect impacts of digital technology on SA.
Table 10. Regression results of the spatial model of the impact of digital technology on sustainable agricultural development
From the above, it can be seen that the process of digital technology to promote the rise of SA containing job-seeking and entrepreneurship, supply of agricultural products, pollution reduction, and carbon reduction, etc., due to the spillover effect of digital technology, makes farmers between different regions share equalized social services in the digital dividend. At the same time, different regions can learn from each other and mutually benefit from the digital infrastructure to promote SA.
6. Discussion and conclusions
At a time when the wave of digitization is driving the global agriculture 4.0 revolution, it is significant to study the role of digital technology in creating a sustainable human home from the agricultural sector. This paper is based on the technique of coupled coordination to establish a new SA evaluation system that includes poverty alleviation and income increase, food security, and green agriculture. By collecting a panel observation sample of 276 prefecture-level cities in China from 2005 to 2020, the systematic impact of digital technology on SA and its intrinsic mechanism are examined in a multi-dimensional manner using fixed-effects model, threshold model, mediated-effects model, and spatial model.
The main conclusion is as follows: digital technology has become an essential force driving SA, and this conclusion still holds through robustness tests such as the introduction of instrumental variables. However, the effect of digital technology on SA and GA presents a nonlinear change with increasing marginal effects. But for PI, it shows a U-shaped incentive process of decreasing and then increasing. Given location factors, digital technology is more likely to boost SA and GA in the eastern region and PI and FS in the central and western areas. In addition, peripheral cities are more likely to get SA, PI, and GA boosted by digital technology. Mechanism analysis shows that enhancing agricultural labor productivity and stimulating productivity in agriculture is the mechanism of action of digital technology for SA, and digital financial inclusion plays the role of a positively regulated facilitator. Finally, digital technology has spatial spillover effects on SA incentives, suggesting that digital technology contributes to a balanced pattern of agricultural production across regions.
Our innovative work is integrated into the process and conclusions of the study. We adopt a sustainable framework to shape a more systematic paradigm of agricultural development and a coupled coordination technique to measure SA systems, enriching scholars’ studies that have examined the impact of digital technology on the agriculture problem from a single indicator only (Chandio et al., Citation2023; Li et al., Citation2023). At the same time, our research incorporates moral and ethical issues on environmental pollution, poverty, and social equity, enriching the framework of research on agricultural issues, which is an orientation with long-term value (Jiang et al., Citation2022; Klerkx & Rose, Citation2020). Unlike Lange et al. (Citation2020), who argue that digital technology increases environmental burdens, we find a positive effect of digital technology on pollution and carbon reduction from the agricultural sector. We find the incentive effect of increasing the marginal impact of digital technology in SA and GA, a study that enriches the applicability of Metcalfe’s Law from the field of agriculture.
We summarize the challenges in advancing SA goals with digital technology. Luan et al. (Citation2023) argued that the process of acceptance of new technologies is slower among farmers in backward areas because of the digital divide. Our study comes to a similar conclusion that the digital divide leads to a U-shaped incentive process where digital technology first decreases and then increases PI. The difference is that in the second half of the rise of the U-shape, our conclusions yield evidence of practices that bridge the digital divide, a dynamic that complements the linear paradigm of the consequences of the digital divide (Li et al., Citation2023). In addition, we find that the imbalance in regional economic development and the lagging digital literacy and environmental awareness of farmers lead to the inhibition of digital technology to function as a food production enhancer (Xu, Citation2023). Further, it also leads to difficulties in the effectiveness of digital technologies in realizing the green potential of the Midwest.
Based on the spatial spillover effect of digital technology, the open and shared digital era will become an essential opportunity for humanity to work together toward sustainability. At a time when Industry 4.0 is driving the development of Agriculture 4.0, how to rebuild a sustainable agricultural system in the digital age is a fundamental topic for the survival of all humanity. Especially in developing countries and backward regions, strengthening information-sharing communication and learning will be a historic moment in solving the economic, demographic, and environmental crises.
To further strengthen the role of digital technology as an engine for sustainable agriculture, the findings of this paper also have the following policy implications:
Increase the development of digital infrastructure in backward regions to narrow the first digital divide between the areas. Based on realizing full coverage of communication networks in rural areas, improve the construction of digital infrastructure in rural areas to ensure that urban and rural residents receive equal digital dividends.
Poverty is the greatest unsustainability, and the Government should increase the area of coverage and the scale of protection of inclusive finance. Only by giving farmers access to more financial support can poverty be reduced and prevented from returning to poverty, and the application of green technologies is accelerated.
On the one hand, it is necessary to promote the relevant knowledge of farmers in the field of e-commerce and to increase their entrepreneurial skills and innovative capacity, which will help to broaden their income channels. On the other hand, the Government should guide the job market to set reasonable entry thresholds, reduce unnecessary digital skill requirements, and give farmers more room to choose their careers. This will allow farmers with low educational attainment and advanced age to obtain fair employment opportunities and avoid vulnerable groups in the digital age falling into the trap of returning to poverty.
The government can make it easier for farmers who maintain traditional farming patterns to reap the dividends of smart agriculture by increasing investment in research and development of digitized, low-threshold, high-efficiency, and green planting technologies. Of course, it is necessary to develop online information regulation strategies to reduce the disturbance of immature digital technologies to the productive life of farmers.
SA is a systematic production + life practice paradigm, which requires the government to increase the popularization of the SA concept. Gradually increase the environmental awareness of farmers while ensuring that the science is sound, and develop a fine-tuned monitoring program that will result in a gradual and sustainable process.
By leading inter-country and inter-regional communication and bridging digital technology barriers, more people can participate in digitalization and work together to promote sustainable human civilization.
In many countries in Asia, Africa, and Latin America, the development of digital technologies is still in its infancy, and traditional agricultural production methods are used to cope with multiple crises. Although the sample data of our study is based on China, which has some limitations, China’s experience in digital technology development, as the world’s second-largest digital economy, still has value to share. Due to the difficulty of data collection, we used relevant data at the prefecture level, which is relatively in-depth for the way the digital divide is stripped away. Future research could take a field research approach to study the impact of the digital divide on SA.
① The degree of coupling and coordination is, where
is the degree of coupling, T is the comprehensive coordination index between the three major subsystems,
indicates the respective comprehensive level index of poverty alleviation and income increase, food security and green agriculture, which is measured by the entropy weight-TOPSIS method, and
denotes the coefficient of determination to be determined, and this paper considers that all the systems in the sustainable agriculture are in the same important position, i.e.,
is all 1/3.
② According to the CEIC database, Hangzhou’s total GDP ranked 8th in China in 2018.
appendix file.docx
Download MS Word (35.5 KB)manuscript.docx
Download MS Word (179.1 KB)Disclosure statement
No potential conflict of interest was reported by the author(s).
Supplementary material
Supplemental data for this article can be accessed online at https://doi.org/10.1080/23311932.2023.2282234
References
- Ali, B., & Dahlhaus, P. (2022). The role of FAIR data towards sustainable agricultural performance: A systematic Literature Review. Agriculture, 12(2), 309. https://doi.org/10.3390/agriculture12020309
- Balmford, B., Green, R. E., Onial, M., Phalan, B., & Balmford, A. (2018). How imperfect can land sparing be before land sharing is more favourable for wild species? The Journal of Applied Ecology, 56(1), 73–26. https://doi.org/10.1111/1365-2664.13282
- Balmford, A., Green, R. E., & Scharlemann, J. P. (2005). Sparing land for nature: Exploring the potential impact of changes in agricultural yield on the area needed for crop production. Global Change Biology, 11(10), 1594–1605. https://doi.org/10.1111/j.1365-2486.2005.001035.x
- Belkhir, L., & Elmeligi, A. (2018). Assessing ICT global emissions footprint: Trends to 2040 & recommendations. Journal of Cleaner Production, 177, 448–463. https://doi.org/10.1016/j.jclepro.2017.12.239
- Cai, J., Ma, E., Lin, J., Liao, L., & Han, Y. (2020). Exploring global food security pattern from the perspective of spatio-temporal evolution. Journal of Geographical Sciences, 30(2), 179–196. https://doi.org/10.1007/s11442-020-1722-y
- Caputo, P., Zagarella, F., Cusenza, M. A., Mistretta, M., & Cellura, M. (2020). Energy-environmental assessment of the UIA-OpenAgri case study as urban regeneration project through agriculture. The Science of the Total Environment, 729, 138819. https://doi.org/10.1016/j.scitotenv.2020.138819
- Chandio, A. A., Akram, W., Sargani, G. R., Twumasi, M. A., & Ahmad, F. (2022). Assessing the impacts of meteorological factors on soybean production in China: What role can agricultural subsidy play? Ecological Informatics, 71, 101778. https://doi.org/10.1016/j.ecoinf.2022.101778
- Chandio, A. A., Bashir, U., Akram, W., Usman, M., Ahmad, M., & Jiang, Y. (2023). What role do international remittance inflows play in boosting agricultural productivity? Empirical analysis of emerging Asian economies. International Journal of Emerging Markets. https://doi.org/10.1108/IJOEM-06-2022-1019
- Chandio, A. A., Gokmenoglu, K. K., Khan, I., Ahmad, F., & Jiang, Y. (2023). Does internet technology usage improve food production? Recent evidence from major rice-producing provinces of China. Computers and Electronics in Agriculture, 211, 108053. https://doi.org/10.1016/j.compag.2023.108053
- Chandio, A. A., Gokmenoglu, K. K., Sethi, N., Ozdemir, D., & Jiang, Y. (2023). Examining the impacts of technological advancement on cereal production in ASEAN countries: Does information and communication technology matter? The European Journal of Agronomy, 144, 126747. https://doi.org/10.1016/j.eja.2023.126747
- Chandio, A. A., Sethi, N., Dash, D. P., & Usman, M. (2022). Towards sustainable food production: What role ICT and technological development can play for cereal production in Asian–7 countries? Computers and Electronics in Agriculture, 202, 107368. https://doi.org/10.1016/j.compag.2022.107368
- Chantarat, S., & Barrett, C. B. (2012). Social network capital, economic mobility and poverty traps. Journal of Economic Inequality, 10(3), 299–342. https://doi.org/10.1007/s10888-011-9164-5
- Chantarat, P., & Skuterud, M. (2004). Internet job search and unemployment durations. The American Economic Review, 94(1), 218–232. https://doi.org/10.1257/000282804322970779
- Chen, Y., Li, S., & Cheng, L. (2020). Evaluation of cultivated land use efficiency with environmental constraints in the Dongting Lake Eco-economic Zone of Hunan province, China. Land, 9(11), 440. https://doi.org/10.3390/land9110440
- Chen, Z., Sarkar, A., Rahman, A., Li, X., & Xia, X. (2022). Exploring the drivers of green agricultural development (GAD) in China: A spatial association network structure approaches. Land Use Policy, 112, 105827. https://doi.org/10.1016/j.landusepol.2021.105827
- Driouchi, A., Azelmad, E. M., & Anders, G. C. (2006). An econometric analysis of the role of knowledge in economic performance. The Journal of Technology Transfer, 31(2), 241–255. https://doi.org/10.1007/s10961-005-6109-9
- Dunlap, R. E., Beus, C. E., Howell, R. E., & Waud, J. (1993). What is sustainable agriculture? An empirical examination of faculty and farmer definitions. Journal of Sustainable Agriculture, 3(1), 5–41. https://doi.org/10.1300/J064v03n01_03
- Elhorst, J. P. (2014). Matlab software for spatial panels. International Regional Science Review, 68(2), 401–420.
- FAO. (2009). FAO's Director-General on How to feed the World 2050. Population and Development Review, 35(4), 837–839. https://doi.org/10.1111/j.17284457.2009.00312.x
- Forman, C., Goldfarb, A., & Greenstein, S. (2012). The internet and local wages: A puzzle. American Economic Review, 102(1), 556–575. https://doi.org/10.1257/aer.102.1.556
- García, V. R., Gaspart, F., Kastner, T., & Meyfroidt, P. (2020). Agricultural intensification and land use change: Assessing country-level induced intensification, land sparing and rebound effect. Environmental Research Letters, 15(8), 085007. https://doi.org/10.1088/1748-9326/ab8b14
- Gómez-Limón, J. A., & Sanchez-Fernandez, G. (2010). Empirical evaluation of agricultural sustainability using composite indicators. Ecological Economics: The Journal of the International Society for Ecological Economics, 69(5), 1062–1075. https://doi.org/10.1016/j.ecolecon.2009.11.027
- Guo, H., Gu, F., Peng, Y., Deng, X., & Guo, L. (2022). Does digital inclusive finance effectively promote agricultural green development?—A case study of China. International Journal of Environmental Research and Public Health, 19(12), 6982. https://doi.org/10.3390/ijerph19126982
- Hall, J., & Vredenburg, H. (2003). The challenges of innovating for sustainable development. MIT Sloan Management Review, 45(1), 61–68.
- Hampton, S. E., Strasser, C. A., Tewksbury, J. J., Gram, W. K., Budden, A. E., Batcheller, A. L., Duke, C. S., & Porter, J. H. (2013). Big data and the future of ecology. Frontiers in Ecology and the Environment, 11(3), 156–162. https://doi.org/10.1890/120103
- Hansen, B. E. (1999). Threshold effects in non-dynamic panels: Estimation, testing, and inference. Journal of Econometrics, 93(2), 345–368. https://doi.org/10.1016/S0304-4076(99)00025-1
- Hickey, L. T., Hafeez, A. N., Robinson, H., Jackson, S. A., Leal-Bertioli, S. C. M., Tester, M., Gao, C., Godwin, I. D., Hayes, B. J., & Wulff, B. B. H. (2019). Breeding crops to feed 10 billion. Nature Biotechnology, 37(7), 744–754. https://doi.org/10.1038/s41587-019-0152-9
- Hidalgo, A., Gabaly, S., Morales-Alonso, G., & Urueña, A. (2020). The digital divide in light of sustainable development: An approach through advanced machine learning techniques. Technological Forecasting and Social Change, 150, 119754. https://doi.org/10.1016/j.techfore.2019.119754
- Hong, Y., Heerink, N., Zhao, M., & van der Werf, W. (2019). Intercropping contributes to a higher technical efficiency in smallholder farming: Evidence from a case study in Gaotai County, China. Agricultural Systems, 173, 317–324. https://doi.org/10.1016/j.agsy.2019.03.007
- Hrustek, L. (2020). Sustainability driven by agriculture through digital transformation. Sustainability, 12(20), 8596. https://doi.org/10.3390/su12208596
- Hu, X., Wang, Z., & Liu, J. (2022). The impact of digital finance on household insurance purchases: Evidence from micro data in China. The Geneva Papers on Risk and Insurance - Issues and Practice, 47(3), 538–568. https://doi.org/10.1057/s41288-022-00267-5
- Jiang, Q., Li, J., Si, H., & Su, Y. (2022). The impact of the digital economy on agricultural green development: Evidence from China. Agriculture, 12(8), 1107. https://doi.org/10.3390/agriculture12081107
- Kamble, S. S., Gunasekaran, A., & Gawankar, S. A. (2020). Achieving sustainable performance in a data-driven agriculture supply chain: A review for research and applications. International Journal of Production Economics, 219, 179–194. https://doi.org/10.1016/j.ijpe.2019.05.022
- Keller, W. (2002). Trade and the transmission of technology. Journal of Economic Growth, 7(1), 5–24. https://doi.org/10.1023/A:1013461025733
- Khattak, S. I., Ahmad, M., Haq, Z., Shaofu, G., & Hang, J. (2022). On the goals of sustainable production and the conditions of environmental sustainability: Does cyclical innovation in green and sustainable technologies determine carbon dioxide emissions in G-7 economies. Sustainable Production and Consumption, 29, 406–420. https://doi.org/10.1016/j.spc.2021.10.022
- Klerkx, L., Jakku, E., & Labarthe, P. (2019). A review of social science on digital agriculture, smart farming and agriculture 4.0: New contributions and a future research agenda. NJAS: Wageningen Journal of Life Sciences, 90–91(1), 1–16. https://doi.org/10.1016/j.njas.2019.100315
- Klerkx, L., & Rose, D. (2020). Dealing with the game-changing technologies of agriculture 4.0: How do we manage diversity and responsibility in food system transition pathways? Global Food Security, 24, 100347. https://doi.org/10.1016/j.gfs.2019.100347
- Knickel, K., Ashkenazy, A., Chebach, T. C., & Parrot, N. (2017). Agricultural modernization and sustainable agriculture: Contradictions and complementarities. International Journal of Agricultural Sustainability, 15(5), 575–592. https://doi.org/10.1080/14735903.2017.1373464
- Lal, R. (2010). Managing soils and ecosystems for mitigating anthropogenic carbon emissions and advancing global food security. BioScience, 60(9), 708–721. https://doi.org/10.1525/bio.2010.60.9.8
- Lange, S., Pohl, J., & Santarius, T. (2020). Digitalization and energy consumption. Does ICT reduce energy demand? Ecological Economics: The Journal of the International Society for Ecological Economics, 176, 106760. https://doi.org/10.1016/j.ecolecon.2020.106760
- Lawin, K. G., & Tamini, L. D. (2019). Tenure security and farm efficiency analysis correcting for biases from observed and unobserved variables: Evidence from benin. Journal of Agricultural Economics, 70(1), 116–134. https://doi.org/10.1111/1477-9552.12275
- Leng, C., Ma, W., Tang, J., & Zhu, Z. (2020). ICT adoption and income diversification among rural households in China. Applied Economics, 52(33), 3614–3628. https://doi.org/10.1080/00036846.2020.1715338
- Lin, Q., Dai, X., Cheng, Q., & Lin, W. (2022). Can digital inclusive finance promote food security? Evidence from China. Sustainability, 14(20), 13160. https://doi.org/10.3390/su142013160
- Liu, Y., & Zhou, M. (2023). Can rural e-commerce narrow the urban–rural income gap? Evidence from coverage of Taobao villages in China. China Agricultural Economic Review, 15(3), 580–603. https://doi.org/10.1108/CAER-09-2022-0221
- Li, F., Zang, D., Chandio, A. A., Yang, D., & Jiang, Y. (2023). Farmers’ adoption of digital technology and agricultural entrepreneurial willingness: Evidence from China. Technology in Society, 73, 102253. https://doi.org/10.1016/j.techsoc.2023.102253
- Li, Y., Zhang, W., Ma, L., Wu, L., Shen, J., Davies, W. J., Oenema, O., Zhang, F., & Dou, Z. (2014). An analysis of China’s grain production: Looking back and looking forward. Food and Energy Security, 3(1), 19–32. https://doi.org/10.1002/fes3.41
- Luan, B., Zou, H., & Huang, J. (2023). Digital divide and household energy poverty in China. Energy Economics, 119, 106543. https://doi.org/10.1016/j.eneco.2023.106543
- Malmodin, J., & Lundén, D. (2018). The energy and carbon footprint of the global ICT and E&M sectors 2010–2015. Sustainability, 10(9), 3027. https://doi.org/10.3390/su10093027
- Ma, W., & Wang, X. (2020). Internet use, sustainable agricultural practices and rural incomes: Evidence from China. The Australian Journal of Agricultural and Resource Economics, 64(4), 1087–1112. https://doi.org/10.1111/1467-8489.12390
- Meena, R. S., Kumar, S., Datta, R., Lal, R., Vijayakumar, V., Brtnicky, M., Sharma, M. P., Yadav, G. S., Jhariya, M. K., Jangir, C. K., Pathan, S., Dokulilova, T., Pecina, V., & Marfo, T. (2020). Impact of agrochemicals on soil microbiota and Management: A Review. Land, 9(2), 34. https://doi.org/10.3390/land9020034
- Mili, S., & Martínez-Vega, J. (2019). Accounting for regional heterogeneity of agricultural sustainability in Spain. Sustainability, 11(2), 299. https://doi.org/10.3390/su11020299
- Millan, J. M., Lyalkov, S., Burke, A., Millan, A., & van Stel, A. (2021). Digital divide’among European entrepreneurs: Which types benefit most from ICT implementation? Journal of Business Research, 125, 533–547. https://doi.org/10.1016/j.jbusres.2019.10.034
- Munyegera, G. K., & Matsumoto, T. (2018). ICT for financial access: Mobile money and the financial behavior of rural households in Uganda. Review of Development Economics, 22(1), 45–66. https://doi.org/10.1111/rode.12327
- Mushi, G. E., DiMarzo Serugendo, G., & Burgi, P. Y. (2022). Digital technology and services for sustainable agriculture in Tanzania: A Literature Review. Sustainability, 14(4), 2415. https://doi.org/10.3390/su14042415
- NFU. (2019). The future of food 2040. Retrieved May 7, 2023, from https://www.nfuonline.com/nfu-online/news/the-future-of-food-2040/.
- Nie, P., Ma, W., & Sousa-Poza, A. (2021). The relationship between smartphone use and subjective well-being in rural China. Electronic Commerce Research, 21(4), 983–1009. https://doi.org/10.1007/s10660-020-09397-1
- Nunn, N., & Qian, N. (2014). US food aid and civil conflict. The American Economic Review, 104(6), 1630–1666. https://doi.org/10.1257/aer.104.6.1630
- OECD. (2019). Agri-environmental indicators. http://www.oecd.org/greengrowth/sustainable-agriculture/agri-environmentalindicators.Html
- Park, C. Y., & Mercado, R. V. (2016). Does financial inclusion reduce poverty and income inequality in developing. Palgrave Macmillan UK. Mercado.
- Phalan, B., Onial, M., Balmford, A., & Green, R. E. (2011). Reconciling food production and biodiversity conservation: Land sharing and land sparing compared. Science, 333(6047), 1289–1291. https://doi.org/10.1126/science.1208742
- Qin, T., Wang, L., Zhou, Y., Guo, L., Jiang, G., & Zhang, L. (2022). Digital technology-and-services-driven sustainable transformation of agriculture: Cases of China and the EU. Agriculture, 12(2), 297. https://doi.org/10.3390/agriculture12020297
- Robinson, S. V., Nguyen, L. H., & Galpern, P. (2022). Livin’on the edge: Precision yield data shows evidence of ecosystem services from field boundaries. Agriculture, Ecosystems & Environment, 333, 107956. https://doi.org/10.1016/j.agee.2022.107956
- Roussaki, I., Doolin, K., Skarmeta, A., Routis, G., Lopez-Morales, J. A., Claffey, E., Mora, M., & Martinez, J. A. (2022). Building an interoperable space for smart agriculture. Digital Communications and Networks, 9(1), 183–193. https://doi.org/10.1016/j.dcan.2022.02.004
- Sanders, R. (2006). A market road to sustainable agriculture? Ecological agriculture, green food and organic agriculture in China. Development & Change, 37(1), 201–226. https://doi.org/10.1111/j.0012-155X.2006.00475.x
- Shen, J., Zhu, Q., Jiao, X., Ying, H., Wang, H., Wen, X., Xu, W., Li, T., Cong, W., & Liu, X. (2020). Agriculture green development: A model for China and the world. Frontiers of Agricultural Science and Engineering, 7(1), 5–13. https://doi.org/10.15302/J-FASE-2019300
- Shin, D. H., & Choi, M. J. (2015). Ecological views of big data: Perspectives and issues. Telematics and Informatics, 32(2), 311–320. https://doi.org/10.1016/j.tele.2014.09.006
- Simone, C., & Christopher, S. (2003). Can information and communications technology applications contribute to poverty reduction? Lessons from rural India. Information Technology for Development, 10(2), 73–84. https://doi.org/10.1002/itdj.1590100203
- Smil, V. (1999). China’s agricultural land. China Quarterly, 158, 414–429. https://doi.org/10.1017/S030574100000583X
- Stevenson, M., & Lee, H. (2001). Indicators of sustainability as a tool in agricultural development: Partitioning scientific and participatory processes. International Journal of Sustainable Development & World Ecology, 8(1), 57–65. https://doi.org/10.1080/13504500109470063
- Struik, P. C., & Kuyper, T. W. (2017). Sustainable intensification in agriculture: The richer shade of green. A review. Agronomy for Sustainable Development, 37(5), 39. https://doi.org/10.1007/s13593-017-0445-7
- Trivelli, L., Apicella, A., Chiarello, F., Rana, R., Fantoni, G., & Tarabella, A. (2019). From precision agriculture to industry 4.0.: Unveiling technological connections in the agrifood sector. British Food Journal, 121(8), 1730–1743. https://doi.org/10.1108/BFJ-11-2018-0747
- Tsolakis, N., Aivazidou, E., & Srai, J. S. (2019). Sensor applications in agrifood systems: Current trends and opportunities for water stewardship. Climate, 7(3), 44. https://doi.org/10.3390/cli7030044
- Überbacher, R., Ceipek, R., Hautz, J., Matzler, K., & De Massis, A. (2019). Digital IoT technology, firm performance and family involvement: A resource utilization perspective. Academy of Management Proceedings, 2019(1), 14697. https://doi.org/10.5465/AMBPP.2019.14697abstract
- Van Cauwenbergh, N., Biala, K., Bielders, C., Brouckaert, V., Franchois, L., Cidad, V. G., Hermy, M., Mathijs, E., Muys, B., Reijnders, J., Sauvenier, X., Valckx, J., Vanclooster, M., Van der Veken, B., Wauters, E., & Peeters, A. (2007). SAFE—A hierarchical framework for assessing the sustainability of agricultural systems. Agriculture, Ecosystems & Environment, 120(2–4), 229–242. https://doi.org/10.1016/j.agee.2006.09.006
- Wang, M., Bai, L., Gong, S., & Huang, L. (2020). Determinants of consumer food safety self-protection behavior—an analysis using grounded theory. Food Control, 113, 107198. https://doi.org/10.1016/j.foodcont.2020.107198
- Wang, J., Bi, S., Li, Y., & Lyu, K. (2018). The effect of formal credit constraint on grain production. Journal of Agrotechnical Economics, 5, 28–39. https://doi.org/10.13246/j.cnki.jae.2018.05.003
- Wang, H., Grant, W. E., & Teague, R. (2020). Modeling rangelands as spatially-explicit complex adaptive systems. Journal of Environmental Management, 269, 110762. https://doi.org/10.1016/j.jenvman.2020.110762
- Wang, Y., Huo, Z., Li, D., & Zhang, M. (2022). Evaluation of common prosperity level and regional difference analysis along the Yangtze river economic Belt. International Journal of Environmental Research and Public Health, 19(19), 11851. https://doi.org/10.3390/ijerph191911851
- Wu, L., Zhong, Y., Shan, L., & Qin, W. (2013). Public risk perception of food additives and food scares. The case in Suzhou, China. Appetite, 70, 90–98. https://doi.org/10.1016/j.appet.2013.06.091
- Xu, C. (2023). Towards balanced low-carbon development: Driver and complex network of urban-rural energy-carbon performance gap in China. Applied Energy, 333, 120663. https://doi.org/10.1016/j.apenergy.2023.120663
- Xu, G., Sarkar, A., & Qian, L. (2020). Does organizational participation affect farmers’ behavior in adopting the joint mechanism of pest and disease control? A study of Meixian County, Shaanxi province. Pest Management, 77(3), 1428–1443. https://doi.org/10.1002/ps.6161
- Yilmaz, S., Haynes, K. E., & Dinc, M. (2002). Geographic and network neighbors: Spillover effects of telecommunications infrastructure. Journal of Regional Science, 42(2), 339–360. https://doi.org/10.1111/1467-9787.00262
- Zhang, A., Chandio, A. A., Yang, T., Ding, Z., & Liu, Y. (2023). Examining how internet use and non-farm employment affect rural households’ income gap? Evidence from China. Frontiers in Sustainable Food Systems, 7, 1173158. https://doi.org/10.3389/fsufs.2023.1173158
- Zhang, C., & Liu, C. (2015). The impact of ICT industry on CO2 emissions: A regional analysis in China. Renew. Renewable and Sustainable Energy Reviews, 44, 12–19. https://doi.org/10.1016/j.rser.2014.12.011
- Zhang, X. Z., & Xu, Z. W. (2023). Facebook and Tencent data fit a cube law better than Metcalfe’s law. Journal of Computational Science & Technology, 38(2), 219–227. https://doi.org/10.1007/s11390-022-2845-7
- Zhang, Q., Zhang, F. F., Wu, G. Y., & Mai, Q. (2021). Spatial spillover effects of grain production efficiency in China: Measurement and scope. Journal of Cleaner Production, 278(1), 121062. https://doi.org/10.1016/j.jclepro.2020.121062
- Zhong, R., He, Q., & Qi, Y. (2022). Digital Economy, Agricultural Technological Progress, and Agricultural Carbon Intensity: Evidence from China. International Journal of Environmental Research and Public Health, 19(11), 6488. https://doi.org/10.3390/ijerph19116488
- Zhou, Z., Liu, W., Wang, H., & Yang, J. (2022). The impact of environmental regulation on agricultural productivity: From the perspective of digital transformation. International Journal of Environmental Research and Public Health, 19(17), 10794. https://doi.org/10.3390/ijerph191710794
- Zhou, C., Zhang, R., Ning, X., & Zheng, Z. (2020). Spatial-temporal characteristics in grain production and its influencing factors in the Huang-Huai-Hai plain from 1995 to 2018. International Journal of Environmental Research and Public Health, 17(24), 1–19. https://doi.org/10.3390/ijerph17249193
- Zhou, L., Zhen, F., Wang, Y., & Xiong, L. (2019). Modeling the spatial formation mechanism of poverty-stricken counties in China by using geographical detector. Sustainability, 11(17), 4752. https://doi.org/10.3390/su11174752