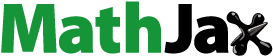
Abstract
The current study investigates how genotype by environment interaction (GEI) complicates superior cultivar selection in onion breeding. Employing a randomized block design with three replications, we assessed GEI effects on onion yield and identified adaptable, stable genotypes. The sensitivity of onion genotypes to environmental changes significantly influences yield and quality, necessitating adaptability across diverse climatic conditions. We conducted multilocation trials evaluating 28 onion genotypes across four distinct locations, each representing varying environmental factors. Through phylogenetic analysis, genotypes were categorized into four clusters: cluster I (3), cluster II (4), cluster III (15), and cluster IV (6). Rigorous assessment of yield performance, employing additive main effects and multiplicative interaction models, particularly the AMMI model, revealed five genotypes demonstrating remarkable stability and potential across diverse environmental conditions: RO-1626, RO-1623, RO-1639, RO-1625, and RO-1627. Notably, genotypes from cluster II exhibited the highest marketable yield (277.06 q/ha) and total yield (295.66 q/ha), indicating adaptability to varied environmental conditions. These findings hold promise for breeding high-yielding onion varieties resilient to diverse environments, ensuring stability, adaptability, and quality in cultivation.
REVIEWING EDITOR:
1. Background
Onion (Allium cepa L.) is a significant bulbous crop belonging to the Alliaceae family (Ghodke et al., Citation2018). India has the most onion cultivation area globally and ranks second only to tomatoes in terms of global onion production, following China, contributing approximately 20% of global production (Fayos et al., Citation2022; Khade et al., Citation2022; Yusupov et al., Citation2021). During the fiscal year 2022-23, the country exported 2,525,258.35 metric tons of fresh onions worldwide, amounting to a total value of USD 561.38 million, as reported by APEDA 2022-23. However, the productivity per unit area is notably lower than that of leading producers such as China, the USA, and the Netherlands. Onions are considered highly export-oriented crops that contribute significantly to foreign exchange earnings. Indian onions are categorized as short-day onions and are grown in three distinct seasons: 50%–60% in rabi followed by 20%–25% in kharif and late kharif (Gopal, Citation2015; Tripathy et al., Citation2014). The kharif season denotes the agricultural period in India driven by the monsoon, encompassing the cultivation and harvesting of crops amidst the rainy season. It usually entails crop transplantation in July and August, while the late kharif season involves transplanting in October and November. Conversely, the rabi season pertains to winter crop cultivation, notably significant for onion production in India. Onions provide a plethora of health benefits owing to their rich content of flavonoids, anthocyanins, fructooligosaccharides, and organosulfur compounds (Bal et al., Citation2019; Bisen & Emerald, Citation2016; Ko et al., Citation2015; Putnik et al., Citation2019).
Despite its widespread recognition, onion plants face considerable challenges in genetic enhancement because of its semi-perishable nature, short lifespan, and vulnerability to several diseases such as Stemphylium blight, purple blotch and anthracnose that cause significant damage to onions (Khar et al., Citation2022). Onion marketable yield can be greatly impacted by climate and environmental conditions. Varieties that thrive in one environment may not necessarily flourish in other environments or in a range of climates. The genotype-by-environment interaction (GEI) is an important phenomenon in plant breeding which defines the differential response of genotypes to various environments as a multiple environment trials (METs) (Rodrigues et al., Citation2014). Analyzing the interactions between genotypes and environmental conditions at various sites is an effective method for determining the adaptability and stability of genotypes (Bustos-Korts et al., Citation2019). Modeling the GEI and measuring genotypic performance and stability are essential for enhancing selection efficiency and determining the adaptability of a genotype within METs (Rodrigues et al., Citation2014). The yield potential is defined by genotype, and the extent to which that potential is realized is determined by external factors. The main effects of the genotype, the environment, and the genotype × environment interaction (GxE interaction) were combined to determine the yield of a given genotype in a given environment, making genotype stability essential for consistent performance under varying environmental conditions, whether the yields are low or high (Kang, Citation2002).
Crop yields, both current and potential, can vary for various reasons, including low genetic stability, limited diversity, inadequate adaptation, and the absence of high-yielding crop varieties (Bal et al., Citation2021). The estimation of genetic diversity among potato onion genotypes was conducted to aid in the identification of various groups of parents for the purpose of generating diversity (Gupta et al., Citation2023). A study assessing the genetic diversity of seventeen garlic genotypes utilized both morphological characteristics and molecular markers. Gupta et al. (Citation2022) utilized seventeen pairs of SSR markers and twenty-four morphological traits in the DUS test for the genetic diversity analysis of ten onion varieties. The results unveiled notable variation, facilitating the discrimination of the onion varieties. Furthermore, a significant correlation was noted between the diversity measures of SSR markers and morphological traits. A total of twenty-three morphological traits associated with the foliage and bulb characteristics of garlic plants were examined and classified into quantitative and qualitative parameters (Gupta et al., Citation2021). The cornerstone of plant breeding lies in genetic diversity, which is fundamental to all improvement efforts. Since yield is a quantitative trait influenced by both genetic and environmental factors, breeding high-yielding onion genotypes presents a complex challenge. Selecting stable and high-yielding genotypes in METs, especially when strong GEIs are present, becomes even more challenging. Quantifying and reducing the GEI remain top priorities for any breeding program.
Therefore, evaluating available germplasms is essential for enhancing genetic diversity, identifying stable and adaptable genotypes, and developing high-yielding onion varieties. To evaluate genotype stability in a variety of environments and determine the best places to cultivate them, METs are frequently carried out. Genetic advancement in plant breeding programs is impeded by GEI, which weakens the correlation between genotypic and phenotypic values (Fox et al., Citation2012). Planning breeding programs and determining how many tests are needed to assess how adaptable the breeding material is to various environments depend heavily on the degree of GEI.
Given that some genotypes exhibit notable differences in performance across various environments, the GEI is an important consideration when choosing genotypes for particular target environments. Numerous statistical models, including hybrid analysis with additive main effects and multiplicative interaction (AMMI) as a biplot, are used to illustrate interaction patterns, pinpoint genotypes that exhibit stability across various environments, and graphically depict the interplay between genotypes (G), environments (E), and GEIs (Chatterjee et al., Citation2019; Oladosu et al., Citation2017). Breeders can select stable and adaptable genotypes across diverse environments by using genotype (G) main effect plus genotype × environment interaction (GGE) models to quantify and understand patterns of GEI and evaluate genotype performance under various environmental conditions (Ebem et al., Citation2021; Krishnamurthy et al., Citation2021).
The ultimate goal of any plant breeding and selection programme is to produce genotypes that can consistently perform well in a variety of circumstances. Understanding and accounting for the GEI is essential for designing cultivars that are more adaptable to varying environmental conditions, as they provide difficulties in finding such stable genotypes. Given this context, our main goal was to highlight how Indian onion genotypes differ in their performance under various environmental circumstances. Furthermore, we simultaneously selected for genotypes that were highly stable and performed well. For breeding programmes that are successful, these chosen genotypes can be useful milestones.
2. Materials and methods
The present study aimed to evaluate the stability of yield performance of various onion genotypes at four distinct locations across India, each representing distinct agroclimatic zones. These locations included the ICAR-Directorate of Onion and Garlic Research in Pune located at (latitude: 27.19’00°N, longitude: 82.25’00° E), Chandra Shekhar Azad University of Agriculture and Technology in Kanpur situated at the (latitude: 26.29’28°N, longitude: 80.18’25°E), the Regional Research Station at the National Horticulture Research and Development Foundation in Karnal located at (latitude: 29.74’86°N and longitude: 76.99’69°E) and Tamil Nadu Agriculture University in Coimbatore situated at the (latitude: 11.01’22° N, longitude: 76.93’54° E). The experiment was conducted using a randomized block design with three replications, and data were collected for eleven crucial parameters, including marketable yield (q/ha), total yield (q/ha), double bulbs (%), bolter bulbs (%), TSS (%), average bulb weight (g), days to harvest, thrips incidence (%), purple blotch incidence (%), Stemphylium blight incidence (%), and storage loss (%). The geographical locations and weather data are provided in Supplementary Table 1. A total of 28 red onion genotypes were selected for the current experiment from the onion germplasm collection maintained by ICAR-DOGR, Pune. These genotypes were sourced from various locations across the country, including Maharashtra, Telangana, Andhra Pradesh, Chhattisgarh, and Karnataka. Detailed information on all the genotypes is outlined in . The seedlings of 28 red onion genotypes, approximately 45 days old, were transplanted into beds measuring 1x1 m in width and 15 cm in height, with a spacing of 15 × 10 cm. The cultivation practices used were in accordance with the recommendations provided by ICAR-DOGR in Pune. The crop was harvested after more than 50% of the neck fell, and the yield data were meticulously recorded.
Table 1. Detailed information on all the genotypes used in the current study.
The onion yield data collected from all four experimental sites were subjected to analysis of variance (ANOVA) employing a randomized block design with three replications. This research was conducted using SAS software version 9.3 and PROC GLM, treating genotypes and environments as separate factors. The effect of the GEI was explored through the AMMI model, employing JMP Genomics version 10 software. The AMMI stability was computed using STABILITYSOFT software (https://manzik.com/stabilitysoft/), as detailed in Pour-Aboughadareh et al. (Citation2019). Clustering analysis was conducted using MVSP software to group the ecotypes, and this was achieved through unweighted pair group method with arithmetic mean (UPGMA) analysis. Genotypic selection was further validated by employing principal component analysis (PCA)-based biplots and the AMMI model. For the stability analysis of the AMMI, Wricke’s ecovalence (Wi) was calculated using the following formula:
The concept of ecovalence (Wi2), introduced by Wricke (Citation1962), quantifies genotypic contributions to the sum of squares of the GEI. The Wi value for the ith genotype is determined by squaring and summing its interactions across different environments. Genotypes characterized by lower Wi values exhibit minor deviations from the mean across environments, indicating heightened stability.
In the AMMI model, stability constraints such as the regression coefficient (β_i1), pooled deviation of the environment and G × E interactions (MSD_i), mean square deviation from regression (S2d_i), linear response of traits to environmental effects (α_i2), and deviation from the linear response (λ_i) were computed. These constraints serve to assess the stability of each genotype in response to various environmental factors. As proposed by Eberhart and Russell (Citation1966), a genotype is deemed stable when it demonstrates a high mean yield, a regression coefficient of unity (bi = 1), and a minimal deviation from the regression coefficient (S2di) approaching 0, or a value close to it, implying nonsignificant deviation. This analytical approach facilitates the identification of stable genotypes for a specific trait across diverse environments and pinpoints genotypes that exhibit heightened responsiveness to both favorable and unfavorable environmental conditions.
3. Results
The combined ANOVA for marketable yield across all four locations revealed highly significant effects of both environment and genotype at the 1% level of significance (). The multilocation evaluation indicated significant differences in marketable yield among all four locations. The GEI had the highest sum of squares (430063.68), signifying its strong influence on the selection of genotypes for specific environmental conditions. The ANOVA showed that marketable yield was significantly influenced by genotype, environment, and GEI, with sums of squares of 125621.98, 4247310.49, and 430063.68, respectively (). Genotypes demonstrating stable yield performance across different environments are generally considered to have broad adaptability to various conditions. However, genotypes that exhibit high yield potential under specific environmental conditions while performing poorly in nontargeted environments are regarded as cultivars with specific adaptability.
Table 2. Analysis of variance for the marketable yield (q/ha) of twenty-eight genotypes in a multi-environmental evaluation.
Table 3. IPCA scores and environmental means of the marketable yield of twenty-eight genotypes across four locations.
The yield performance of 28 genotypes at four different locations was recorded and compared with that of popular onion cultivars, namely, Bhima Light Red, Bhima Kiran, Bhima Shakti, and L-355, which previously demonstrated the best performance at their respective locations (). Notably, at the Pune location, all the studied genotypes performed exceptionally well in terms of marketable yield, with RO-1630 and RO-1664 genotypes achieving the highest marketable yields, surpassing the popular cultivar Bhima Shakti used as a reference. Conversely, RO-1757 had the lowest marketable yield. In Karnal and Coimbatore, most genotypes exhibited average yield performance, while in Kanpur, all genotypes exhibited the lowest yield performance, with genotypes RO-1670, RO-1751, RO-1669, RO-1665, and RO-1747 achieving the highest marketable yields, outperforming the best check, Bhima Kiran. In contrast, genotype RO-1623 had the lowest marketable yield at Kanpur among all genotypes and across all four locations. At Coimbatore locations, genotypes RO-1755, RO-1756, and RO-1757 achieved the highest yields, while genotype RO-1630 had the lowest yield compared to the best check, Bhima Light Red.
Overall, the results indicate that RO-1755, RO-1756, and RO-1757 consistently achieved the highest yields in both Karnal and Coimbatore, with RO-1630 exhibiting the lowest yield. However, its performance varied substantially, with RO-1630 recording the highest marketable yield at the Pune location. Conversely, genotype RO-1757, which recorded the lowest marketable yield at Pune, performed exceptionally well at Karnal and Coimbatore and achieved an average performance at Kanpur. This significant variation in yield performance could be attributed to the diverse climatic conditions across these locations.
3.1. AMMI analysis
The AMMI ANOVA, which examined the yield outcomes of the twenty-eight genotypes across the four environments, exhibited significant results at the 1% significance level for the GEI, as depicted in . This finding underscores the notable variation among the evaluated onion genotypes.
3.2. AMMI I biplot analysis
In the present study, the varying environmental conditions, as shown at different research locations, were the primary source of variation. The biplot analysis demonstrated that a main effect with a PCA score near zero suggested limited interaction effects. Furthermore, if a genotype and an environment exhibit the same sign on the PCA axis, their interaction is deemed positive, whereas opposite signs indicate a negative interaction. The positions of the environments on the PCA plot were more widely dispersed than those of the genotypes (). This indicates that the variability due to environmental differences is more pronounced than the differences between genotypes. Genotypes with PCA scores near zero are generally regarded as adaptable across environments. In the biplot, the RO-1623, RO-1626, RO-1625, RO-1624, RO-1639, RO-1756, and RO-1619 genotypes are positioned near the axes shared by all four environments, implying that they are considered more stable genotypes suitable for an extensive array of environmental conditions studied.
3.3. AMMI-II biplot analysis
The GEI was additionally subdivided into three interaction principal component axes (IPCAs) namely, IPCA1, IPCA2, and IPCA3, which contributed 41.0%, 24.7%, and 34.3%, respectively, to the interaction. However, when combined, IPCA1 and IPCA2 accounted for 65.7%, IPCA1 and IPCA3 contributed 75.3%, and IPCA2 and IPCA3 contributed 59.0% of the interaction sum of squares (). These findings indicate that all three combinations of IPCA axes explain more than 50% of the interaction sum of squares of the genotypes. The IPCA1 versus IPCA2 relationship () illustrates the extent of interaction between each genotype and environment. The environments and genotypes positioned most distant from the origin are highly accommodating and fit poorly. According to the biplot of IPCA1 against IPCA2, genotypes closer to the center of the biplot are more stable, while those scattered further from the center are more sensitive to environmental interactions. For instance, RO-1670 and RO-1675 exhibited stable activity at Karnal, while RO-1630 was stable and highly productive at Pune but scattered far from its origin at Coimbatore and Kanpur. On the other hand, the RO-1667, RO-1675, RO-1629, L-355, RO-1751, and RO-1670 genotypes are scattered away from the biplot origin, indicating their greater sensitivity to environmental factors.
Figure 3. AMMI-II biplots of PCA1, PCA2 and PCA3 (Note: 1-Karnal, 2-Kanpur, 3-Pune and 4-Coimbatore).

In this study, the twenty-eight onion genotypes were ranked based on their Wricke’s ecovalence values, ranging from the lowest to the highest (). Genotypes RO-1626, RO-1623, RO-1639, RO-1625, and RO-1627 demonstrated notable stability, with Wricke’s ecovalence values of 2.31, 3.02, 3.68, 4.29, and 6.52, respectively. Conversely, Genotype RO-1630 displayed the least stability, characterized by a high Wricke’s ecovalence value of 416.70. The next most common were RO-1664 (154.93), RO-1757 (120.84), RO-1751 (88.18), and RO-1670 (78.54) (). Examining the mean yields, regression coefficients (bi = 1), and S2di values (), the genotypes RO-1623, RO-1625, RO-1626, RO-1627, and RO-1639 emerged as stable and high yields across various environments. Conversely, the genotypes RO-1630, RO-1664, RO-1751, and RO-1757 were identified as high yielding but unstable, suggesting potential challenges in adapting to diverse environments.
Table 4. IPCA scores, genotype means and Wricke ecovalence of the marketable yield of twenty-eight genotypes across four locations.
Table 5. AMMI stability measures for the 28 onion genotypes.
3.4. Cluster analysis
The cluster analysis revealed four distinct clusters, Cluster I-IV, comprising 3, 4, 15, and 6 genotypes, respectively (). The largest number of genotypes, accounting for 53.57% of all the genotypes, was accommodated by major cluster III. Cluster I included genotypes such as RO-1756, RO-1665, and RO-1619. Cluster II included Bhima Shakti, RO-1751, RO-1664, and RO-1630. Cluster III included Bhima Kiran, RO-1670, RO-1669, RO-1629, RO-1628, L-355, Bhima Light Red, RO-1640, RO-1626, RO-1757, RO-1755, RO-1747, RO-1671, RO-1625, and RO-1621, while Cluster IV included RO-1642, RO-1627, RO-1657, RO-1639, RO-1624, and RO-1623 (). Selecting genetically diverse parents for hybridization is a crucial aspect of any crop improvement program aimed at obtaining desirable segregants. When examining the mean performance of genotypes within each cluster concerning marketable yield and associated traits, it becomes evident that genotypes in Cluster II, specifically Bhima Shakti, RO-1751, RO-1664, and RO-1630, demonstrated the highest values across various parameters, including marketable yield (277.06 q/ha), total yield (295.66 q/ha), double bulb percentage (0.43%), bolter bulb percentage (0.31%), average bulb weight (60.78 g), storage loss (24.27%), and disease incidence of purple blotch (11.08%) (as indicated in ). Taken together, these findings suggest that the genotypes included in Cluster II are well suited for use in crop improvement programs, particularly when aiming to increase marketable yield, total yield, average bulb weight, and other relevant factors.
Figure 5. Dendrogram of 28 onion genotypes based on yield and important yield components using the Euclidean distance coefficient and UPGMA clustering.

Table 6. Mean performance of genotypes in individual clusters for marketable yield and its component traits.
4. Discussion
A primary objective of plant breeding initiatives is to enhance stability and ensure consistent crop yields across varied environments. This entails identifying cultivars with desirable traits, particularly high productivity, and evaluating their adaptability through multi-environment trials. The development of new genotypes with both high yield potential and stability is paramount. In our study, we conducted a field experiment to assess 28 onion genotypes under diverse environmental conditions. This comprehensive approach aims to select genotypes that demonstrate high productivity, resilience, and adaptability, thereby contributing to the development of more robust onion varieties. However, significant genotype by environment interaction (GEI) can impede genotype selection by complicating the identification of outstanding genotypes and diminishing the benefits of assortment. It is crucial to assess and comprehend these GEIs, especially when selecting genotypes suitable for diverse and substantially varying target environments (Yan, Citation2011). According to Mederos-Ramirez et al. (Citation2021), GEI in improvement programs profoundly influences the attainment of new cultivars and more stable and adapted genotypes. Evaluating genotypes under diverse environmental conditions is a critical step in identifying stable genotypes with elevated yields tailored to specific conditions and in pinpointing sites that most accurately reflect the preferred environment (Nowosad et al., Citation2016).
Several previous studies have delved into the adaptability and stability of plant genotypes (Abdipour et al., Citation2017; Goksoy et al., Citation2019; Subaşı & Başalma, Citation2021). An ideal genotype should not only demonstrate superior performance but also maintain stability within and across diverse environments. Various statistical methods have been proposed to assess the consistency of individual genotypes in different environments (Annicchiarico, Citation2002). Therefore, it is recommended that assessments of yield and robustness be conducted concurrently in trials carried out at multiple sites. Multi-environment trials (METs) play a pivotal role in plant breeding endeavors by evaluating the productivity and adaptability of crops across various environmental conditions (Malosetti et al., Citation2013). This approach helps alleviate the impact of genotype by environment interaction (GEI) and improves the precision of selection (Jiwuba et al., Citation2020). Analysis of variance revealed that marketable yield was significantly influenced by genotype, environment, and GEI, with sums of squares of 125621.98, 4247310.49, and 430063.68, respectively (). The significance of GEIs implies that genotypes with greater stability should be prioritized in breeding programs for crop improvement, as indicated by De Vita et al. (Citation2010) and Khar et al. (Citation2008).
Genotypes showing consistent yield performance across diverse environments are generally deemed to possess broad adaptability to various conditions. However, genotypes with high yield potential in specific environmental settings but poor performance in others are considered to have specific adaptability, as highlighted by De Vita et al. (Citation2010) and Pavlovic et al. (Citation2017). Moreover, genotype by environment interaction (GEI) has emerged as a significant finding in multi-environment trials (METs) across various crops, including maize, wheat, garlic, rice, chickpea, barley, and lentil, as reported by Dehghani et al. (Citation2006), Koutis et al. (Citation2012), Karimizadeh et al. (Citation2013), Munawar et al. (Citation2013), Singh et al. (Citation2013), Akter et al. (Citation2015), Erdemci (Citation2018), and Bocianowski et al. (Citation2019). Studies by Singh et al. (Citation2011) and Tripathy et al. (Citation2014) have also documented variations in yield among different onion varieties.
Genotype stability is highly responsive to changing environmental conditions and influenced by various unpredictable sources of variation, as Kang (Citation2002) suggests. The AMMI model has been widely used to understand the dynamics of genotype by environment interaction (GEI) patterns and identify stable cultivars suited to specific target environments, as highlighted by Gauch (Citation2013). ANOVA analysis revealed the statistical significance of GEI, indicating significant variation among the 28 onion genotypes studied. Similar findings were reported by Brdar-Jokanovic et al. (Citation2016) and Pavlovic et al. (Citation2017) for onions, and by Mohammed (Citation2020) and Admassu et al. (Citation2008) for maize. However, it’s notable that the variance attributed to environmental factors was forty times greater than the variation attributed to genotypes within the overall variability. This larger proportion of environmental variation can be attributed to significant disparities in soil types, annual precipitation, average temperatures, and other weather conditions, as explained by Mohammed (Citation2020). AMMI analysis, as outlined by Hagos and Abay (Citation2013), offers a valuable method for assessing genotype stability across various locations, utilizing Principal Component Analysis (PCA) scores and AMMI Stability Value (ASV) values derived from the model’s Interaction Principal Components Axes (IPCA1 and IPCA2). Lower ASV values of genotypes indicate greater stability across different environments, as elucidated by Purchase et al. (Citation2000), aiding in the identification of genotypes with stable performance, crucial for breeding programs aimed at developing resilient and adaptable varieties. Gauch (Citation2006) proposed determining the optimal model for AMMI by predicting it using the first two IPCAs, aligning with the calculation of ASV, incorporating these two IPCA scores, as proposed by Purchase et al. (Citation2000). Therefore, any combination of these IPC axis combinations is sufficient for elucidating the GEI of onion genotypes. The model was considered adequate for explaining the total GEI component, as suggested by Yan and Rajcan (Citation2002). When the GEI belongs to the same sector, the correlation is positive, while it is negative when the GEI belongs to an opposing sector, following the observation of Osiru et al. (Citation2009).
Comparable findings have been documented in various crops, including onions (Pavlovic et al., Citation2017), sweet potatoes (Adebola et al., Citation2013), and maize (Brankovic-Radojcic et al., Citation2018). The adaptability and stability of genotypes are essential prerequisites for introducing a cultivar for widespread cultivation in a specific region. However, the presence of GEI poses a challenge in selecting varieties that maintain stability across changing environments, as highlighted by Khar et al. (Citation2008). The METs play a pivotal role in breeding programs, providing valuable data for evaluating GEI. Given the potential of GEI to influence the performance of genotypes across diverse environments, investigating its impact on yield becomes imperative, as indicated by Kaya et al. (Citation2006). It’s worth noting that the linear regression model lacks a comprehensive analysis of genotype interactions in specific environments and does not facilitate the identification of potential genotypes suitable for particular conditions, as observed by Erdemci (Citation2018).
In our study, Shula’s Stability Variance (σ2ᵢ) and GE Variance component (θi) exhibited lower values, with regression coefficients (bi) close to unity (). The mean rank values presented in confirm that RO-1626 secured a lower position, indicating both higher yield and stability. Our selection of superior and stable genotypes aligns with the methodology proposed by Pour-Aboughadareh et al. (Citation2019), where genotypes with lower average ranking values are preferred. Notably, RO-1629 emerged as the highest-ranked genotype based on five parametric stability methods. Additionally, the mean rank values in further underscore the superior and stable attributes of RO-1626, as it achieved a lower score. This consistent criterion for selecting genotypes with reduced average ranking values aids in identifying superior and stable genotypes across diverse environments.
The cluster analysis effectively categorizes onion genotypes into four distinct clusters (), with Cluster II demonstrating notable stability and adaptability characteristics. This insight holds significant value for crop improvement programs, providing valuable guidance on potential genotypes for enhancing marketable yield and other relevant traits. Moreover, previous research has indicated that genotypes within a cluster displaying elevated mean values for specific traits could yield more favorable results in their progeny when used as parents. This suggests the potential utility of this approach in hybridization programs aimed at generating improved segregants, as noted by Arya et al. (Citation2017), Singh et al. (Citation2017), and Singh et al. (Citation2020).
Furthermore, as highlighted by Ghaderi et al. (Citation1984), increasing the genetic divergence between parent plants leads to a greater prevalence of divergent alleles at targeted loci. Consequently, in the F2 and F3 generations following a cross between distantly related parents, the recombination of these loci significantly enhances the prospects for efficiently selecting factors related to yield. Genotypes originating from distant clusters can serve as valuable reservoirs of genes associated with diverse desirable traits in onion species. These findings are in line with the research of Chattopadhyay et al. (Citation2013), Singh et al. (Citation2017), Dangi et al. (Citation2018), and Singh et al. (Citation2020), who also emphasized that utilizing genotypes from clusters with greater genetic distance in hybridization programs leads to a broad spectrum of variation in offspring.
Similar outcomes emphasizing the significance of genetic diversity have been documented by researchers elsewhere, including Khar et al. (Citation2008), Singh et al. (Citation2013), and Singh et al. (Citation2020), in their respective onion studies. This collective body of research underscores the crucial role of genetic diversity and the potential benefits of leveraging distant clusters in onion breeding programs to enhance variability and improve desirable traits in progeny. Overall, these findings underscore the challenges posed by GEIs in crop improvement programs. Taken together, these findings emphasize the need for comprehensive assessments that consider both yield and stability across diverse environments and suggest that selecting genotypes with broad adaptability and utilizing genetic diversity through hybridization can contribute to successful crop improvement.
5. Conclusion
This study underscores the significant influence of genotype, environment, and their interaction on yield stability in onion cultivation. Our findings underscore the substantial impact of geographical variations on onion genotype performance, with RO-1630 and RO-1664 demonstrating the highest marketable yields at Pune locations. Stability analysis pinpointed five genotypes (RO-1626, RO-1623, RO-1639, RO-1625, and RO-1627) with consistent performance across all four locations. These stable genotypes present promising prospects for breeding programs aimed at enhancing yield, adaptability, and bulb quality. Investing in long-term, multi-location genetic testing can be pivotal in the development of high-yielding, adaptable onion varieties. Further research is imperative to elucidate the molecular mechanisms underpinning genetic diversity and stability in these genotypes, paving the way for more informed breeding strategies.
Authors’ contributions
Conceptualization of research (AJG, VM, MS); Designing of the experiments (AJG, VM); Contribution of experimental materials (AJG); Execution of field/lab experiments and data collection (AJG); Analysis of data and interpretation (AJG, APB, PM); Preparation of the manuscript (AJG, PM, APB, YPK, PAG). Edit and finalization of manuscript (AJG, VM, PM).
Consent for publication
The work is presented in the manuscript with the consent of all authors.
Ethics approval and consent to participate
The manuscript was not submitted anywhere else, and results were presented without fabrication, falsification, or inappropriate data manipulation. Research does not pose any threat to public health or national security.
Supplemental Material
Download MS Word (15.2 KB)Acknowledgements
We sincerely acknowledge All India Network Research Project on Onion and Garlic (AINRPOG) under ICAR-Directorate of Onion and Garlic Research, Rajgurunagar, Pune for support, technical inputs and resources. This work was financially supported by Indian Council of Agriculture Research, New Delhi.
Disclosure statement
The authors declare that they have no competing interests.
Data availability statement
The authors confirm that the data supporting the findings of this study are available within the article [and/or] its supplementary materials.
Additional information
Funding
Notes on contributors
Amar Jeet Gupta
Amar Jeet Gupta, PhD, is affiliated with the esteemed ICAR-Directorate of Onion and Garlic Research (ICAR-DOGR) located in Pune, Maharashtra, India. His research primarily focuses on the development of improved varieties, development of male sterile lines, hybrid seed production, molecular breeding, and F1 hybrids in onion.
Yogesh P. Khade
Yogesh P. Khade, Ph.D., is a dedicated researcher affiliated with the esteemed ICAR-Directorate of Onion and Garlic Research (ICAR-DOGR) located in Pune, Maharashtra, India. His research interests lie in crop production, post-harvest technology, and crop improvement.
Ashwini P. Benke
Ashwini P. Benke, PhD, is a distinguished researcher affiliated with the renowned ICAR-Directorate of Onion and Garlic Research (ICAR-DOGR) situated in Pune, Maharashtra, India. Her research interests encompass various facets of plant science, including plant genetic resources, cytogenetics, molecular breeding, plant tissue culture.
Pawan Mainkar
Pawan Mainkar, PhD, is a distinguished researcher affiliated with the prestigious ICAR-Directorate of Onion and Garlic Research (ICAR-DOGR) situated in Pune, Maharashtra, India. His primary research interests lie in biotic and abiotic stress management in plants, particularly focusing on chickpea, pigeonpea, soybean, and onion.
Pranjali A. Gedam
Pranjali A. Gedam, PhD, is a dedicated researcher affiliated with the ICAR-Directorate of Onion and Garlic Research (ICAR-DOGR) situated in Pune, Maharashtra, India. Her research interests primarily revolve around the management of abiotic stress in onion crops.
Vijay Mahajan
Vijay Mahajan, PhD, is a distinguished researcher affiliated with the ICAR-Directorate of Onion and Garlic Research (ICAR-DOGR) based in Pune, Maharashtra, India. His expertise lies in the field of vegetable breeding and improvement, with a particular focus on enhancing the genetic potential and traits of onion and garlic varieties.
Major Singh
Major Singh, PhD, is a respected researcher affiliated with the ICAR-Directorate of Onion and Garlic Research (ICAR-DOGR) in Pune, Maharashtra, India. His expertise lies in the field of vegetable breeding and biotechnology, focusing particularly on onion and garlic crops.
References
- Abdipour, M., Vaezi, B., Younessi-Hamzekhanlu, M., & Ramazani, S. H. R. (2017). Nonparametric phenotypic stability analysis in advanced barley (Hordeum vulgare L.) genotypes. Journal of Crop Science and Biotechnology, 20(4), 1–15. https://doi.org/10.1007/s12892-017-0050-0
- Adebola, P. O., Shegro, A., Laurie, S. M., Zulu, L. N., & Pillay, M. (2013). Genotype x environment interaction and yield stability estimate of some sweet potato (Ipomoea batatas (L.) Lam) breeding lines in South Africa. Journal of Plant Breeding and Crop Science, 5(9), 182–186. https://doi.org/10.5897/jpbcs2013.0387
- Admassu, S., Nigussie, M., & Zelleke, H. (2008). Genotype-environment interaction and stability analysis for grain yield of maize (Zea mays L.) in Ethiopia. Asian Journal of Plant Sciences, 7(2), 163–169. https://doi.org/10.3923/ajps.2008.163.169
- Akter, A., Hasan, M. J., Kulsum, U., Rahman, M., Khatun, M., & Islam, M. (2015). GGE biplot analysis for yield stability in multi-environment trials of promising hybrid rice (Oryza sativa L.). Bangladesh Rice Journal, 19(1), 1–8. https://doi.org/10.3329/brj.v19i1.25213
- Annicchiarico, P. (2002). Genotype x environment interactions - challenges and opportunities for plant breeding and cultivar recommendations. Rome, Italy. http://www.fao.org/docrep/005/y4391e/y4391e00.HTM
- APEDA. 2022-2023. Agricultural and Processed Food Products Export Development Authority: Fresh onions export. https://agriexchange.apeda.gov.in/indexp/Product_description_32headChart.aspx?gcode=0201
- Arya, V. K., Singh, J., Kumar, L., Kumar, R., Kumar, P., & Chand, P. (2017). Genetic variability and diversity analysis for yield and its components in wheat (Triticum aestivum L.). Indian Journal of Agricultural Research, 51(OF), 128–134. https://doi.org/10.18805/ijare.v0iOF.7634
- Bal, S., Maity, T. K., & Maji, A, Dept. of Vegetable Science, Bidhan Chandra Krishi Viswavidyalaya, Mohanpur, West Bengal (741 235), India. (2022). Assessment of genetic variability, heritability and genetic gain for yield and quality traits in onion (Allium cepa L.). International Journal of Bio-Resource and Stress Management, 13(7), 674–682. https://doi.org/10.23910/1.2022.2919
- Bal, S., Maity, T. K., & Sharangi, A. B. (2021). Morphological and biochemical characterization of onion (Allium cepa L.) germplasm by principal component analysis. J Pharmacogn Phytochem, 10(1), 121–124. https://www.researchgate.net/profile/Solanki-Bal/publication/348137834
- Bal, S., Maity, T. K., Sharangi, A. B., & Maji, A. (2019). Screening of onion (Allium cepa L.) germplasm against purple blotch disease. Journal of Pharmacognosy and Phytochemistry, 8(6), 546–548. https://www.researchgate.net/publication/337185420
- Bisen, S. P., & Emerald, M. (2016). Nutritional and therapeutic potential of garlic and onion (Allium sp.). Current Nutrition & Food Science, 12(3), 190–199. https://doi.org/10.2174/1573401312666160608121954
- Bocianowski, J., Niemann, J., & Nowosad, K. (2019). Genotype-by-environment interaction for seed quality traits in interspecific cross-derived Brassica lines using additive main effects and multiplicative interaction model. Euphytica, 215(1), 7. https://doi.org/10.1007/s10681-018-2328-7
- Brankovic-Radojcic, D., Babic, V., Girek, Z., Zivanovic, T., Radojĉic, A., Filipovic, M., & Srdic, J. (2018). Evaluation of maize grain yield and yield stability by AMMI analysis. Genetika, 50(3), 1067–1080. https://doi.org/10.2298/GENSR1803067B
- Brdar-Jokanovic, M., Girek, Z., Ugrinovic, M., Sikora, V., Djalovic, I., & Zdravkovic, J. (2016). AMMI model in the analysis of genotype by environment interaction of conventionally and organically grown onion. Genetika, 48(3), 1027–1038. https://doi.org/10.2298/GENSR1603027B
- Bustos-Korts, D., Romagosa, I., Borràs-Gelonch, G., Casas, A. M., Slafer, G. A., & van Eeuwijk, F. (2019). Genotype by environment interaction and adaptation. In. Crop Science. 4070-4094, https://doi.org/10.1007/978-1-4939-8621-7_199
- Chatterjee, C., Debnath, M., Karmakar, N., & Sadhukhan, R. (2019). Stability of grass pea (Lathyrus sativus L.) genotypes in different agroclimatic zone in eastern part of India with special reference to West Bengal. Genetic Resources and Crop Evolution, 66(7), 1515–1531. https://doi.org/10.1007/s10722-019-00809-2
- Chattopadhyay, A., Baran Sharangi, A., Dutta, S., Das, S., & Denre, M. (2013). Genetic relatedness between quantitative and qualitative parameters in onion (Allium cepa L.). Vegetos, 26(1), 151–157. https://doi.org/10.5958/j.2229-4473.26.1.021
- Dangi, R., Kumar, A., & Khar, A. (2018). Genetic variability, heritability, and diversity analysis in short day tropical onion (Allium cepa). Indian J Agric Sci, 88(6), 140–149. https://doi.org/10.56093/ijas.v88i6.80653
- De Vita, P., Mastrangelo, A. M., Matteu, L., Mazzucotelli, E., Virzì, N., Palumbo, M., Storto, M. L., Rizza, F., & Cattivelli, L. (2010). Genetic improvement effects on yield stability in durum wheat genotypes grown in Italy. Field Crops Research, 119(1), 68–77. https://doi.org/10.1016/j.fcr.2010.06.016
- Dehghani, H., Ebadi, A., & Yousefi, A. (2006). Biplot analysis of genotype by environment interaction for barley yield in Iran. Agronomy Journal, 98(2), 388–393. https://doi.org/10.2134/agronj2004.0310
- Ebem, E. C., Afuape, S. O., Chukwu, S. C., & Ubi, B. E. (2021). Genotype × environment interaction and stability analysis for root yield in sweet potato [Ipomoea batatas (L.) Lam. Frontiers in Agronomy, 3, 665564. https://doi.org/10.3389/fagro.2021.665564
- Eberhart, S. A., & Russell, W. A. (1966). Stability parameters for comparing varieties 1. Crop Science, 6(1), 36–40. https://doi.org/10.2135/cropsci1966.0011183X000600010011x
- Erdemci, I. (2018). Investigation of genotype × environment interaction in chickpea genotypes using AMMI and GGE biplot analysis. Turkish Journal of Field Crops, 23(1), 20–26. https://doi.org/10.17557/tjfc.414846
- Fayos, O., Echávarri, B., Vallés, M. P., Mallor, C., Garcés-Claver, A., & Castillo, A. M. (2022). A simple and efficient method for onion pollen preservation: Germination, dehydration, storage conditions, and seed production. Scientia Horticulturae, 305, 111358. https://api.semanticscholar.org/CorpusID:251421693 https://doi.org/10.1016/j.scienta.2022.111358
- Fox, P. N., Crossa, J., Romagosa, I., & Fox, P. N. (2012). Multi-environment testing and genotype × environment interaction. In R. A Kempton & M. Cerezo (Eds.), Statistical Methods for Plant Variety Evaluation (pp. 117–138.). Springer: Dordrecht. https://doi.org/10.1007/978-94-009-1503-9_8
- Gauch, H. G. (2006). Statistical analysis of yield trials by AMMI and GGE. Crop Science, 46(4), 1488–1500. https://doi.org/10.2135/cropsci2005.07-0193
- Gauch, H. G. (2013). A simple protocol for AMMI analysis of yield trials. Crop Science, 53(5), 1860–1869. https://doi.org/10.2135/cropsci2013.04.0241
- Ghaderi, A., Adams, M. W., & Nassib, A. M. (1984). Relationship between genetic distance and heterosis for yield and morphological traits in dry edible bean and faba bean. Crop Science, 24(1), 37–42. https://doi.org/10.2135/cropsci1984.0011183x002400010009x
- Ghodke, P. H., Andhale, P. S., Gijare, U. M., Thangasamy, A., Khade, Y. P., Mahajan, V., & Singh, M. (2018). Physiological and biochemical responses in onion crop to drought stress. International Journal of Current Microbiology and Applied Sciences, 7(1), 2054–2062. https://doi.org/10.20546/ijcmas.2018.701.247
- Goksoy, A. T., Sincik, M., Erdogmus, M., Ergin, M., Aytac, S., Gumuscu, G., Gunduz, O., Keles, R., Bayram, G., & Senyigit, E. (2019). The parametric and nonparametric stability analyses for interpreting genotype by environment interaction of some soybean genotypes. Turkish Journal of Field Crops, 24, 28–38. https://doi.org/10.17557/tjfc.562637
- Gopal, J. (2015). Onion research in India: Status and challenges. Progressive Horticulture, 47(1), 1. https://doi.org/10.5958/2249-5258.201
- Gupta, A. J., Anandhan, S., Manjunathagowda, D. C., Benke, A. P., Mahajan, V., Kad, S. K., & Singh, M. (2022). Complement test for distinctiveness, uniformity and stability testing of kharif onion (Allium cepa L.) varieties. Genetic Resources and Crop Evolution, 69(6), 2217–2229. https://doi.org/10.1007/s10722-022-01372-z
- Gupta, A. J., Jayaswall, K., Khar, A., Mahajan, V., Kad, S. K., & Singh, M. (2021). Analysis of genetic diversity among Indian garlic (Allium sativum L.) genotypes using SSR markers and morphological traits. Veg Sci, 48(02), 219–227. https://doi.org/10.61180/vegsci.2021.v48.i2.15
- Gupta, A. J., Khade, Y. P., Mahajan, V., Hange, S. R., Shalaka, R. S., & Singh, M. (2023). Morphological and Molecular Characterization of Multiplier Onion (Allium cepa var. aggregatum) Genotypes. Plant Molecular Biology Reporter, https://doi.org/10.1007/s11105-023-01415-4
- Hagos, H. G., & Abay, F. (2013). AMMI and GGE biplot analysis of bread wheat genotypes in the northern part of Ethiopia. Journal of Plant Breeding and Genetics, 1, 12–18. https://esciencepress.net/journals/index.php/JPBG/article/view/50
- Jiwuba, L., Danquah, A., Asante, I., Blay, E., Onyeka, J., Danquah, E., & Egesi, C. (2020). Genotype by environment interaction on resistance to cassava green mite associated traits and effects on yield performance of cassava genotypes in Nigeria. Frontiers in Plant Science, 11, 572200. https://doi.org/10.3389/fpls.2020.572200
- Kang, M. S. (2002). Genotype-environment interaction: progress and prospects. Quantitative Genetics, Genomics and Plant Breeding, 221–243. https://doi.org/10.1079/9780851996011.0221
- Karimizadeh, R., Mohammadi, M., Sabaghni, N., Mahmoodi, A. A., Roustami, B., Seyyedi, F., & Akbari, F. (2013). GGE biplot analysis of yield stability in multi-environment trials of lentil genotypes under rainfed condition. Notulae Scientia Biologicae, 5(2), 256–262. https://doi.org/10.15835/nsb529067
- Kaya, Y., Akçura, M., & Taner, S. (2006). GGE-Biplot analysis of multi-environment yield trials in bread wheat. Turkish Journal of Agriculture and Forestry, 30(5), 325–337. https://doi.org/10.3906/tar-0604-6
- Khade, Y. P., Salunkhe, S. R., Manjunathagowda, D. C., Sinhasane, S. R., Mahidar, G. T. Y., Mahajan, V., & Singh, M. (2022). Molecular characterization of short-day onion genotypes by intron length polymorphic (ILP) markers. Genet. Resour. Crop Evol, 69, 2077–2086.
- Khar, A., Devi, A. A., & Lawande, K. E. (2008). Analysis of genetic diversity among Indian garlic (Allium sativum L.) cultivars and breeding lines using RAPD markers. Indian J Genet Plant Breed, 68(1), 52–57.
- Khar, A., Galván, G. A., & Singh, H. (2022). Allium breeding against biotic stresses. In K. Chittaranjan (Ed.), Genomic Designing for Biotic Stress Resistant Vegetable Crops (pp. 233–259). Springer International Publishing.
- Ko, E. Y., Nile, S. H., Sharma, K., Li, G. H., & Park, S. W. (2015). Effect of different exposed lights on quercetin and quercetin glucoside content in onion (Allium cepa L.). Saudi Journal of Biological Sciences, 22(4), 398–403. https://doi.org/10.1016/j.sjbs.2014.11.012
- Koutis, K., Mavromatis, A. G., Baxevanos, D., & Koutsika-Sotiriou, M. (2012). Multienvironmental evaluation of wheat landraces by GGE Biplot Analysis for organic breeding. Agricultural Sciences, 03(01), 66–74. https://doi.org/10.4236/as.2012.31009
- Krishnamurthy, S. L., Sharma, P. C., Sharma, D. K., Singh, Y. P., Mishra, V. K., Burman, D., Maji, B., Mandal, S., Sarangi, S. K., Gautam, R. K., Singh, P. K., Manohara, K. K., Marandi, B. C., Chattopadhyay, K., Padmavathi, G., Vanve, P. B., Patil, K. D., Thirumeni, S., Verma, O. P., … Singh, R. K. (2021). Additive main effects and multiplicative interaction analyses of yield performance in rice genotypes for general and specific adaptation to salt stress in locations in India. Euphytica, 217(2), 1–15. https://doi.org/10.1007/s10681-020-02730-7
- Malosetti, M., Ribaut, J. M., & van Eeuwijk, F. A. (2013). The statistical analysis of multi-environment data: Modeling genotype-by-environment interaction and its genetic basis. Frontiers in Physiology, 4, 44. https://doi.org/10.3389/fphys.2013.00044
- Mederos-Ramirez, A., Ortiz-Perez, R., & Postal, G. (2021). Analysis of the genotype-environment interaction in soybean crop (Glycine max (L) Merrill). Cultivos Tropicales, 42(1), 1–10. https://doi.org/10.1016/j.fcr.2020.107922
- Mohammed, A. (2020). Genotype by environment interaction and yield stability analysis of open pollinated maize varieties using AMMI model in Afar Regional State, Ethiopia. Journal of Plant Breeding and Crop Science, 12(1), 8–15. https://doi.org/10.5897/JPBCS2019.0839
- Munawar, M., Hammad, G., & Shahbaz, M. (2013). Evaluation of maize (Zea mays L.) hybrids under different environments by GGE biplot analysis. Journal of Agriculture and Environmental Sciences, 13(9), 1252–1257. https://doi.org/10.5829/idosi.aejaes.2013.13.09.11036
- Nowosad, K., Liersch, A., Popławska, W., & Bocianowski, J. (2016). Genotype by environment interaction for seed yield in rapeseed (Brassica napus L.) using additive main effects and multiplicative interaction model. Euphytica, 208(1), 187–194. https://doi.org/10.1007/s10681-015-1620-z
- Oladosu, Y., Rafii, M. Y., Abdullah, N., Magaji, U., Miah, G., Hussin, G., & Ramli, A. (2017). Genotype × Environment interaction and stability analyses of yield and yield components of established and mutant rice genotypes tested in multiple locations in Malaysia*. Acta Agriculturae Scandinavica. Section B, Soil and Plant Science, 67(7), 590–606. https://doi.org/10.1080/09064710.2017.1321138
- Osiru, O. M., Olanya, O. M., Adipala, E., Kapinga, R., & Lemaga, B. (2009). Yield stability analysis of Ipomoea batatus L. cultivars in diverse environments. Australian Journal of Crop Science, 3(4), 213–220. https://search.informit.org/doi/abs/10.3316/INFORMIT.037732748411875
- Pavlovic, N., Girek, Z., Zdravkovic, M., Gvozdanovic-Varga, J., Moravcevic, D., & Zdravkovic, J. (2017). Mode of inheritance and AMMI analysis of onion (Allium cepa L.) bulb traits. Genetika, 49(2), 729–742. https://doi.org/10.2298/GENSR1702729P
- Pour-Aboughadareh, A., Yousefian, M., Moradkhani, H., Poczai, P., & Siddique, K. H. M. (2019). STABILITYSOFT: A new online program to calculate parametric and non-parametric stability statistics for crop traits. Applications in Plant Sciences, 7(1), e01211. https://doi.org/10.1002/aps3.1211
- Purchase, J. L., Hatting, H., & van Deventer, C. S. (2000). Genotype × environment interaction of winter wheat (Triticum aestivum L.) in South Africa: II. Stability analysis of yield performance. South African Journal of Plant and Soil, 17(3), 101–107. https://doi.org/10.1080/02571862.2000.10634878
- Putnik, P., Gabrić, D., Roohinejad, S., Barba, F. J., Granato, D., Mallikarjunan, K., Lorenzo, J. M., & Bursać Kovačević, D. (2019). An overview of organosulfur compounds from Allium spp.: From processing and preservation to evaluation of their bioavailability, antimicrobial, and anti-inflammatory properties. Food Chemistry, 276, 680–691. https://doi.org/10.1016/J.FOODCHEM.2018.10.068
- Rodrigues, P. C., Malosetti, M., Gauch, H. G., & van Eeuwijk, F. A. (2014). A weighted AMMI algorithm to study genotype-by-environment interaction and QTL-by-environment interaction. Crop Science, 54(4), 1555–1570. https://doi.org/10.2135/cropsci2013.07.0462
- Singh, S. R., Ahamed, N., Srivastava, K. K., Kumar, D., & Yousuf, S. (2020). Assessment of genetic divergence in long day onion (Allium cepa L.) through principal component and single linkage cluster analysis. Journal of Horticultural Sciences, 15(1), 17–26. https://doi.org/10.24154/jhs.v15i1.778
- Singh, S. R., Ahmed, N., Srivastva, K. K., Singh, D. B., Singh, A., & Yousuf, S. (2017). Genetic diversity assessment in European carrot genotypes. Indian Journal of Horticulture, 74(1), 56–61. https://doi.org/10.5958/0974-0112.2017.00014.7
- Singh, R. K., Bhonde, S. R., & Gupta, R. P. (2011). Genetic variability in late kharif (Rangada) onion (Allium cepa L.). Journal of Applied Horticulture, 13(01), 74–78. https://doi.org/10.37855/jah.2011.v13i01.18
- Singh, S., Jha, A., Acharya, S., Shukla, S., & Acharya, N. (2020). Determination and Estimation of Allicin in Allium sativum. Journal of Evolution of Medical and Dental Sciences, 9(49), 3711–3715. https://doi.org/10.14260/jemds/2020/815
- Singh, B. G., Kumar, H., Gupta, V., & Brar, S. P. (2013). GGE biplot analysis for characterization of garlic (Allium sativum L.) germplasm based on agro-morphological traits. International Journal of Plant Breeding and Genetics, 7(2), 106–110. https://api.semanticscholar.org/CorpusID:208028596
- Subaşı, İ., & Başalma, D. (2021). Assessment of genotype × environment interaction of safflower (Carthamus tinctorius L.) genotypes by parametric and non-parametric methods. European Journal of Agriculture and Food Sciences, 3(1), 112–118. https://doi.org/10.24018/ejfood.2021.3.1.233
- Tripathy, P., Sahoo, B. B., Priyadarshini, A., Das, S. K., & Dash, D. K. (2014). Standardization of Kharif onion cultivars. International Journal of Bio-Resource and Stress Management, 5(2), 269–274. https://doi.org/10.5958/0976-4038.2014.00566
- Wricke, G. (1962). On a method of understanding the biological diversity in field research. Z Pfl-Zücht, 47(1), 92–96. https://www.scirp.org/reference/referencespapers?referenceid=2062232
- Yan, W. (2011). GGE Biplot vs. AMMI graphs for genotype-by-environment data analysis. Journal of the Indian Society of Agricultural Statistics, 65(2), 181–193. https://www.cabidigitallibrary.org/doi/full/10.5555/20113313412
- Yan, W., & Rajcan, I. (2002). Biplot analysis of test sites and trait relations of soybean in Ontario. Crop Science, 42(1), 11–20. https://doi.org/10.2135/cropsci2002.1100
- Yusupov, Z., Deng, T., Volis, S., Khassanov, F., Makhmudjanov, D., Tojibaev, K., & Sun, H. (2021). Phylogenomics of Allium cepa (Amaryllidaceae) provides new insights on domestication of onion. Plant Diversity, 43(2), 102–110. https://doi.org/10.1016/j.pld.2020.07.008