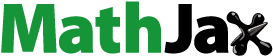
Abstract
This research intends to aid product managers at early stages (before detailed design stage) of product development to identify and thus benchmark the minimal risk prone design concept for further development and commercialization. As requirements pertaining to relevant functional divisions within the enterprise are needed to be included at the project initiation stage of design process, it can be easily ascertained that pertinent techno-commercial risks be included for development of the predictive framework. Bayesian network methodology has been deployed to draw the relationships amongst various risk parameters corresponding to different functional divisions. The devised framework has been validated and tested using a real-life case from construction and mining equipment industry. The key benefit arising out of my evolved methodology is that product development agencies within manufacturers would be able to iteratively converge upon the design concepts representing moderate to lower risk as far as effective execution of downstream organizational processes such as production, sourcing, distribution etc. is concerned.
PUBLIC INTEREST STATEMENT
Choosing right product design concept during early stages of new product development is a critical decision that has direct bearing on the downstream tactical and operational decisions such as supply chain planning, aggregate planning, aftersales stocking, vendor selection and so forth. Therefore, it is imperative that at early stages of product development itself, original equipment manufacturer must be able to identify the design concepts that would yield least risk to the enterprise. Therefore, to this end in this research we develop an analytical model that would enables us to benchmark one design concept over the other thus providing enterprises with a pragmatic tool intended to enhance their competitive advantage
1. Introduction
New product development (NPD) endeavors to realize an end product starting from a concept such that customer’s needs are satisfied or exceeded while meeting the manufacturability aspects (Clark & Wheelwright, Citation1993). NPD (being a concurrent project) seeks to achieve the desired product configurations within cost and quality targets before developmental and commercialization related activities can be executed (Kalluri & Kodali, Citation2014; Kayis et al., Citation2006; Schmitt & Linder, Citation2013). These definitions aptly imply that product development (specifically in an original equipment manufacturer) is a collaborative concern that takes into account considerations related to other functional divisions such as product development, sourcing, production, service and so forth.
Owing to the fact that multifunctional inputs drive the NPD processes, it is often associated with numerous uncertainties that can undermine desired organizational targets. Therefore, managing and mitigating the risks emanating from considerations pertaining to other functional divisions on NPD becomes imperative. These risks are defined as uncertain events that can derail NPD’s technical and commercial objectives (Chin et al., Citation2009; Leithold, Woschke, Heiko, & Kratzer, Citation2016). Furthermore, need for a structured and coherent approach that enables product managers at early stage of product development to make decisions concerning alternative design concepts from technical risk standpoint has been gaining momentum owing to the complexities associated with product development projects. Several studies have concluded that risk identification and mitigation in most organizations is often carried out employing informal and unsystematic ways and is primarily driven by top management’s perceptions. (Cooper, Citation2006, Goswami & Tiwari, Citation2014; Kayis, Arndt, & Zhou, Citation2007).
In this research, we devise an analytical risk assessment methodology for a product characterized by modularity. The methodology seeks to converge at a design solution associated with least risk profile as far as the enterprise is concerned. We have adopted the approach devised by Agard and Basseto (Citation2013) for modeling a modular product. Contrary to the approach devised by Chin et al. (Citation2009), we propose a risk evaluation methodology for a complex product containing disparate modules in contrast to considering the product to have a unified and integrated architecture. We use Bayesian network to bridge the causal relationships between pertinent technical risk factors due to its ability to map correlation(s) among respective risk factors. Our approach (to establish the relationships amongst various risk factors) is in-line with the approach devised by Wang and Tseng (Citation2011), wherein customer preference modeling was done for product design process using Naive Bayes principle. This research complements the work done by Ringen, Holtskog, and Martinsen (Citation2012), Wang and Tseng (Citation2011) and Goswami & Tiwari, (Citation2014) thus proposing a systematic framework for gauging product and process novelty at nascent stages of development process. Unlike these studies however (except that of Goswami & Tiwari, Citation2014; Goswami, Citation2018), our work focuses on developing an analytical model for technical risks emanating from interplays between technical objectives of pertinent functional divisions (organizational stakeholders). The primary novelty of our work pertains to (1) development of analytical risk evaluation methodology for a product characterized by modularity in contrary to simpler products driven by integrated architecture; (2) the developed methodology considers technical risks emanating from considerations of various functional divisions that are part of internal value chain of any organization, thus viewing the risk mitigation for design related decision-making within the NPD from a systems (enterprise) standpoint. We illustrate our devised analytical model employing an industry case example of canopy in heavy equipment machinery.
The rest of of the paper is organized as follows. Section 2 presents a state-of-the-art literature review of recent relevant research. Section 3 describes problem environment in detail, followed by model framework and solution methodology in Section 4. Section 5 illustrates our methodology with a real-life case example, while the model validation has been done in Section 6. Finally, discussions and concluding remarks are enumerated in Section 7.
2. Literature survey
A number of researchers have addressed the issue of risk identification, assessment, evaluation and mitigation in context of enterprise processes and internal projects. In our critical literature review, we classify these researches into two distinct categories: (a) theoretical/empirical studies and (b) analytical frameworks.
2.1. Theoretical/empirical frameworks
Thamhain et al., (Citation2013) inferred that effective handling of risks and uncertain events require management insights that go beyond traditional quantitative tools and techniques, employing the field study of 35 major product development projects spanning across 17 high-technology firms. This study emphasizes the role of collaboration across all facets of the cross functional project team. Marmier, Gourc, and Laarz (Citation2013) evolved a decision-making tool on the basis of principles of a coherent process between risk and project management teams, thus aiding project managers to choose ways that can improve project success rate. This decision-making framework was demonstrated employing a case study from aerospace industry. Mu, Peng, and Maclachlan (Citation2009) employing survey data from Chinese manufacturers illustrated that risk mitigation strategies aimed at specific risk factors such as organizational and technological contribute both individually and interactively to influence the performance of NPD projects. Toh and Miller, (Citation2016) investigated impact of individual risk aversion and ambiguity aversion during design concept selection process based on the exploratory study of 38 undergraduate students. The study found that individual risk aversion is significantly correlated with creative concept selection and idea generation. Taha et al. (Citation2015) devised a methodology for selecting the best product design from a sustainability perspective employing the case study of screw design and utilizing Analytic hierarchy process (AHP). Wu, Lin, and Wu (Citation2015)’s contribution pertained to scenario planning and decision tree analysis for competitiveness of NPD programs. Bertoni, Bertoni, and Isaksson (Citation2018) evolved an EVOKE framework grounded in allowing benchmarking of design options with respect to sub-system level requirements. The paper contributed essentially in terms of methodology contributing towards gathering and dispatching relevant information about “design intent’ of complex systems.
2.2. Analytical studies
Patil, Grantham, and Steele (Citation2012) proposed a Business Risk in Early Design (B-RED) method for preliminary risk assessment based on the historical data from business failures. Tian, Wang, Wang, and Zhang (Citation2017) employing a life cycle assessment technique developed a novel MCDM (multi criteria decision-making) method, thus solving green product design selection problems using linguistic information. Wang, Lin, and Huang (Citation2010) transformed the organizational performance measures into project performance metric by exploiting the properties of quality function deployment (QFD). A key contribution of this research was in terms of development of a systematic procedure for risk identification, assessment, response planning, and control. Shah et al. (Citation2016) devised a value-risk based decision-making tool for evaluating the performance of various manufacturing scenarios. This study derived the values and concerns from qualitative objective statements and risk statements. These statements were consolidated, and subsequent analysis was done to obtain global indicators of value and risk. Aikhuele and Turan (Citation2016) evolved a user friendly and reliable methodology for evaluation and selection of product design concepts employing a score function and weighted normalized hamming distance method. The approach was illustrated in context of selection and evaluation of various printed circuit board concepts for an electronics manufacturing company in Malaysia.
Although the presented studies have addressed the aspects related to risk identification, assessment, evaluation and mitigation from multiple perspectives, a crucial research gap persists in bridging the theoretical risk dimensions emanating from involvement of various functional divisions within the enterprise in the product development projects with quantitative decision-making such as design concept selection in the product development process. Our research seeks to abridge this crucial gap.
3. Problem environment
3.1. Product description
Suppose the enterprise seeks to converge upon a design concept (DC); wherein Pa needs to be selected for subsequent commercialization. Pa needs J disparate modules for the product to be fully functional. j depicts a particular module; where j = 1, 2, 3…..J. Let represent kth module instance for module “j” such that k = 1, 2, 3…Kj, where Kj is the count of module instances for module “j”.
Different product design concepts may contain one or more than one number of same module instances.
3.2. Enterprise risk modeling
A product at an early design stage typically requires inputs from a number of functional divisions. These inputs represent the objective of respective functional divisions. For example, corresponding to functional division product development, primary objective is associated with designing a product that meets/exceeds all functional/customer/statutory related requirements. Each functional division has a pertinent risk factor depicted as parent node. Additionally, an individual parent node is associated with various related root nodes against which all possible DCs are to be assessed. All nodes are characterized by risk states: high risk (H), medium risk (M), and low risk (L). These risk factors are defined in Appendix section.
In this research, each root node, dq, has a single parent node, Cr. The probabilistic risk of a root node being in individual state, i.e., either in H, M, and L influences the probabilistic risk of a parent node being in a given state, thus impacting the overall enterprise risk for a DC. Table demonstrates the designations for various nodes/combination of node for respective q, r, and t values.
Table 1. Designations for nodes
The overall network constituted of the root nodes, parent nodes, and overall enterprise node termed drives the Enterprise Risk Index (ERI) for feasible DCs are shown in Figure .
Suppose, n different states, S1, S2, S3… ….Sn have no parent node and probability of a particular state Sb:P(Sb) need to be specified. Prior and conditional probabilities of individual states of node can be derived by first populating pair-wise comparison matrix and then using the systematic probability generation framework for determination of prior as well as conditional probability devised by Chin et al. (Citation2009) and employed by Goswami and Tiwari (Citation2014) and Goswami (Citation2018).
4. Model framework and solution methodology
In this paper, the objective is to find such a DC that minimizes the overall ERI (enterprise risk index). ERI is proposed as an indirect measure implying that a particular DC has the strongest likelihood of being most favorable for an enterprise in terms of being cost efficient for development with desired quality attributes and characterized by delivery efficiency to the end consumer. Let represent the probability of parent node “r” being in state “S2”such that
.If the parent node “r” is in state “H”, then
=
.
represents the probability of tth paired combination of node being in risk state “S3”. Similarly,
represents the probability of combination “t” node of module “j” of module instance “k” in state “S3”.
The expected value of resultant enterprise risk node of module instance “k” corresponding to module “j” for parent node “r” being in high risk state given that combination of root nodes “t” is in high risk state, medium risk and low risk state can be mathematically expressed as ,
Our purpose is to converge at the least risky DC as far as the enterprise is concerned. Hence, the objective function is formulated as minimization of summation of all the enterprise risk nodes for respective modules such that select module instance is in risk state “H”.
The flow map of the proposed solution methodology is illustrated in Figure
5. An illustrative case example
In the illustrative example, for sake of brevity, we consider only two functional agencies, i.e., design and supply chain. The methodology has been demonstrated using a case example of canopy of heavy machinery equipment whose basic configuration is illustrated in Figure .
Figure 3. Isometric views of ROPS canopy (adopted from Goswami & Tiwari, Citation2014).
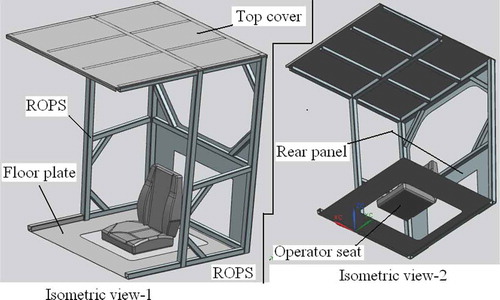
A canopy primarily has four major modules namely floor plate, rollover protection system (ROPS), fall over protection system (FOPS), and top cover.
5.1. Enlist module instances for each module
The module instances under consideration is enlisted in Table . These are known in advanced and assumed to be derived from initial CAD (computer added design) modeling.
Table 2. Details of various module instances
5.2. Determine the conditional probabilities for overall network
Pair wise comparisons are performed utilizing inputs from the experts, i.e., functional leaders from individual functional divisions (in our case two functional areas: design, supply chain) who are qualified to provide such inputs and have a wide exposure with strategic, technical, and operational areas.
Considering node “PR” (product risk) as an example. As illustrated in the network given in Figure, the root nodes “CPD” and “RDC” has a parent node “PR”. Deploying the method devised by Chin et al. (2006), we first obtain
Employing the convention adopted in Table , following equations can be populated:
When the CPD is in risk state H, the expert completes the matrix by responding to the question Without taking into account the effect of other roots nodes on ‘PR’, when ‘CPD’ is in the risk state H, which state of ‘PR’ is more likely to occur and how much more likely?. Similar to Saaty’s AHP method and as illustrated by Chin et al. (Citation2009), the probabilities for each state are calculated and CR/CI scores are populated. These resulting probabilities are presented in Table .
Table 3. Resultant probabilities of PRRR conditional of CPD (H)
When “CPD” is in risk state (H), the probability of “PR” being at risk state (M) is half the probability of “PR” at risk state (H) and probability of “PR” being at risk state (L) is one third the probability of “PR” at risk state (H). This assertion is quite reasonable as intricacies associated with complex products often results in increased chances of reliability and functional issues during the product’s lifecycle. Using the method suggested by Chin et al. (Citation2009), the probabilities of PR at different risk states contingent on the three risk states of CPD and RDC; and the probabilities of SCR on different risk states of CSC and SES are determined and presented in Table .
Table 4. Resulting probabilities
Afterwards, the probability of high risk state of node “PR” conditional on each state of its root nodes, i.e., “CPD” and “RDC” can be determined as follows:
Using the above approach, conditional probability values are calculated and listed below:
5.3. Generate the prior probabilities for different module instances
For a given module and corresponding to the respective root nodes, all module instances are compared amongst each other and by answering the question Considering the similarity of existing modules at the company which state is more likely to and how much more likely. This procedure is repeated for all the 4 root nodes. The resulting prior probabilities are populated in Table .
Table 5. Prior probabilities
5.4. Calculate ERI for all the selected MPDC (most preferred design concept)
For calculating the ERI (say for parent node r, corresponding to tth combination of root nodes, module instance k of jth module) represented as, following equations have been used:
Extending formula given by Equation 6 for risk states “M” and “L” and corresponding to r = 1, t = 1, and q = 1 and 2, we obtain
This value represents the enterprise risk index corresponding to parent node “PR”, paired combination of nodes “CPD” and “RDC”, module 1 and its module instance, i.e., M1,1.
Similarly, we can determine ERI (2,2,1,1) that represents the enterprise risk index corresponding to parent node “SCR”, paired combination of nodes “CSC” and “SES” for module instance M1,1.
Using the above-mentioned approach, we can find the optimal combination of MPDC that would give lowest value of ERI, i.e., 4.72. The corresponding combination of module instance would be M1,1, M2,2, M3,2, M4,1.
6. Model validation
The orthogonal sectional views of two instances of ROPS modules, i.e., M2,1 and M2,2 are depicted in Figure .
M2, is made up of two different plates welded together at two different location each of which has uniform thickness, while M2,2 is constituted of a sheet bent at six different locations and joined at one location using welding process.
Contrasting the ERI values obtained for M2,1 and M2,2 (part details given in Figure ), we find out that M2,2 has a lower value of ERI (1.333) as against that of M2,1 that has a value of 1.554.This gap in ERI values is attributable to the fact that even though M2,1 is associated with significantly higher number of joints compared to M2,2; M2,1 will be relatively easier to produce due to a simpler material section thus eliminating the need for intricate fixturing for maintaining more precise tolerances as would be required for M2,2.
For the validation of our devised framework, we have compared ERI and calculated utility values using framework devised by Chin et al. (Citation2009) where design alternative with higher calculated utility values is selected) for different module instances enlisted in Table . Figure shows this comparison.
Referring to Figure , we can conclude that ERI and utility values have an inverse relationship implying that a module instance with lesser ERI value has a higher utility value, hence enterprise would benefit from selecting it for further development and commercialization.
7. Discussions and concluding remarks
The need to consider product design considerations including its architecture has been well established in the extant research literature. One such work recently has been that of Nepal, Monplaisir, and Famuyiwa (Citation2012), wherein aspects such as BOM (bill of material) were integrated with Supply chain considerations with an aim to improve the Supply chain efficiencies over a long horizon for modular products. This study considered three important stages of the product supply chain viz. procurement, assembly and end stage. Our work complements this research in the sense that three crucial parent node level risks considered in our research, i.e., sourcing, operational, and market risk roughly corresponds to procurement stage, assembly stage, and end stage in the said research. Furthermore, like the work of Nepal et al. (Citation2012), wherein total supply chain compatibility index has been conceptualized as a proxy for supply chain efficiency; our work positions SCRI as a surrogate index to measure supply chain risks for the given product. However, in contrast to the research of Nepal et al. (Citation2012), our work considers the risk propensities (in terms of independent and interdependent risk states) when considering the different product module instance. This is a key distinction of our work in that by including relevant supply chain risk states, we are further able to model the intricacies (e.g., depending upon the capabilities of vendors and relationship with an OEM, varying levels of sourcing risks may pertain corresponding to different vendors for the same OEM) of a modern-day supply chain.
Modularity with the given product under consideration is a key enabler that aids us in systematically evaluating the risk profile for a product constituted of a number of modules. Since our focus is on selection of the MPDC that yields the lowest ERI, hence we have considered high risk states for parent nodes. The current research ignores the interdependence of various root nodes amongst each other in order to bypass “curse of dimension” issue. The analytical methodology has been demonstrated using a real-life case for just two functional agencies namely design and supply chain, but in other real-life cases, depending on the industry domains numerous other divisions can be involved, in which case, the corresponding risk factors would create a more complex network. An obvious extension of this research would be to map the interdependence among risks belonging to different functional areas and assess the behavior of the developed model. However, in such a case the number of probabilities would increase exponentially and enormous effort would be needed to determine those probabilities. Furthermore, we have assumed that the expert’s judgments are fair and without biases. However, this might not be true always as few experts might have biased opinions that our devised methodology cannot model.
Notations and variables
Notations
= state for high risk; M = state for medium risk; L= state for low risk
= notation for parent node.
q = notation for root node.
= notation for modules.
= notation for module instances.
Index for combination of root nodes.
rth parent node.
qth root node.
tth paired combination of root node.
Mj,k = kth module instance for jth module.
S1 = state of nature for root node;
S2 = state of nature for parent node;
S3 = state of nature for combination of node;
Variables
= likelihood of parent node “r” being in risk state “S2” contingent upon on root node “q” being in risk state “S1”.
= likelihood of parent node “r” being in risk state “S2” contingent upon paired combination node “t” being in risk state “S3”.
= likelihood of combination node “t” of module “j” of module instance “k” being in risk state “S3”.
= likelihood of root node “q” of module “j” of module instance “k” in risk state “S2”.
= 1, if the module instance “k” is chosen for module “j”.
0, otherwise.
Additional information
Funding
Notes on contributors
Mohit Goswami
Mohit Goswami, PhD from Indian Institute of Technology-Kharagpur in the department of Industrial and Systems Engineering and Master of Science from Missouri University of Science and Technology, Rolla, USA in Engineering Management has more than a decade of rich industry experiences in global organizations like Tata Motors Ltd. (Pune), Caterpillar Inc. (Illinois, USA), and Tata Hitachi Construction Machinery Ltd. (Jamshedpur). During his stint in industry, he was associated with functions such as product design and development and manufacturing. He has published research papers in reputed journals such as International Journal of Production Research, Journal of Engineering Design, and International Journal of Advanced Manufacturing Technology.
References
- Agard, B., & Basseto, S. (2013). Modular design of product family for quality and cost. International Journal of Production Research, 51(6), 1648–1667. doi:10.1080/00207543.2012.693963
- Aikhuele, D. O., & Turan, F. M. (2016). An interval fuzzy-valued M-TOPSIS model for design concept selection. In Z. B. Ibrahim (Ed.), The national conferences for postgraduate research 2016. Pahang: University Malaysia.
- Bertoni, M., Bertoni, A., & Isaksson, O. (2018). EVOKE: A value-driven concept selection method for early system design. Journal of Systems Science and Systems Engineering, 27(1), 46–77. doi:10.1007/s11518-016-5324-2
- Chin, K., Tang, D., Yang, J., Wong, S., & Wang, H. (2009). Assessing new product development project risk by Bayesian network with a systematic probability generation methodology. Expert Systems with Applications, 36(6), 9879–9890. doi:10.1016/j.eswa.2009.02.019
- Clark, K. B., & Wheelwright, S. C. (1993). Managing new product and process development. New York, NY: Free Press.
- Cooper, R. G. (2006). Managing technology development project. Research Technology Management, 49(6), 23–31. doi:10.1080/08956308.2006.11657405
- Goswami, M. (2018). Supply chain centric product line selection: A functional risk focused approach. International Journal of Production Research. doi:10.1080/00207543.2018.1436788
- Goswami, M., & Tiwari, M. K. (2014). A predictive risk evaluation framework for modular product concept selection in new product design environment. Journal of Engineering Design, 25(1–3), 150–171. doi:10.1080/09544828.2014.921806
- Kalluri, V., & Kodali, R. (2014). Analysis of new product development research: 1998–2009. Benchmarking: An International Journal, 21(4), 527–618. doi:10.1108/BIJ-06-2012-0040
- Kayis, B., Arndt, G., & Zhou, M. (2007). A risk mitigation methodology for new product and process design in concurrent engineering projects. CIRP Annals Manufacturing Technology, 56(1), 167–170. doi:10.1016/j.cirp.2007.05.040
- Kayis, B., Arndt, G., Zhou, M., Savci, S., Khoo, Y. B., & Rispler, A. (2006). Risk quantification for new product design and development in a concurrent engineering environment. CIRP Annals Manufacturing Technology, 55(1), 147–150. doi:10.1016/S0007-8506(07)60386-2
- Leithold, N., Woschke, T., Heiko, H., & Kratzer, J. (2016). “Optimising NPD in SMEs: A best practice approach. Benchmarking: An International Journal, 23(1), 262–284. doi:10.1108/BIJ-05-2015-0054
- Marmier, F., Gourc, D., & Laarz, F. (2013). A risk oriented model to assess strategic decisions in new product development projects. Decision Support Systems, 56(2013), 74–82. doi:10.1016/j.dss.2013.05.002
- Mu, J., Peng, G., & Maclachlan, D. L. (2009). Effect of risk management strategy on NPD performance. Technovation, 29(2009), 170–180. doi:10.1016/j.technovation.2008.07.006
- Nepal, B., Monplaisir, L., & Famuyiwa, O. (2012). Matching product architecture with supply chain design. European Journal of Operational Research, 216(2), 312–325. doi:10.1016/j.ejor.2011.07.041
- Patil, R., Grantham, K., & Steele, D. (2012). Business risk in early design: A business risk assessment approach. Engineering Management Journal, 24(1), 35–46. doi:10.1080/10429247.2012.11431927
- Ringen, G., Holtskog, H., & Martinsen, K. (2012). User friendly framework for measuring product and process novelty in the early stages of product development. Procedia, CIRP, 3(2012), 513–518. doi:10.1016/j.procir.2012.07.088
- Schmitt, R., & Linder, A. (2013). Technical complaint management as a lever for product and process improvement. CIRP Annals Manufacturing Technology, 62(1), 435–438. doi:10.1016/j.cirp.2013.03.040
- Shah, L., Etienne, A., Siadat, A., & Vernadat, A. F. (2016). Decision-making in the manufacturing environment using a value-risk graph. Journal of Intelligent Manufacturing, 27(3), 617–630. doi:10.1007/s10845-014-0895-6
- Taha, Z., Salaam, H., Phoon, A., Phoon, S. Y., Tuan Ya, T. M., & Mohamad, M. R. (2015). Application of integrated sustainability assessment: Case study of a screw design. International Journal of Industrial Engineering, 22(1), 1–10.
- Thamhain, H. (2013). Managing risks in complex projects. Project Management Journal, 44(2), 20–35. doi:10.1002/pmj.21325
- Tian, Z.-P., Wang, J., Wang, J.-Q., & Zhang, H.-Y. (2017). Simplified neutrosophic linguistic multi-criteria group decision-making approach to green product development. Group Decision and Negotiation, 26(3), 597–627. doi:10.1007/s10726-016-9479-5
- Toh, C. A., & Miller, S. R. (2016). Choosing creativity: The role of individual risk and ambiguity aversion on creative concept selection in engineering design. Research in Engineering Design, 27(3), 195–219. doi:10.1007/s00163-015-0212-1
- Wang, J., Lin, W., & Huang, Y. H. (2010). A performance-oriented risk management framework for innovative R&D projects. Technovation, 30(11–12), 601–611. doi:10.1016/j.technovation.2010.07.003
- Wang, Y., & Tseng, M. M. (2011). Integrating comprehensive customer requirements into product design. CIRP Annals Manufacturing Technology, 60(2011), 175–178. doi:10.1016/j.cirp.2011.03.091
- Wu, J. Z., Lin, K. S., & Wu, C. Y. (2015). Integration of scenario planning and decision tree analysis for new product development: A case study of a smartphone project in Taiwan. International Journal of Industrial Engineering, 22(1), 171–182.
Appendix
Design
Parent node
PR (Product risk): Measure of extent that production processes within the enterprise coupled with associated form, fitness, and function in its life cycle cannot be met. This is expressed in probability.
Root nodes
CPD (Complexity of product): Degree to which intricacies of design influences the functional capabilities of the product. More complex the design, higher would be the likelihood of performance related issues in the product’s lifecycle.
RDC (Research and development capability): Degree to evaluate firm’s competency to realize functional products. This can be gauged by the competency of product design team and their prior record in resolving the design issues.
Supply chain
Parent node
SCR (Supply chain risk): Chances that vendor would not be in a position to deliver components within desired cost, timeline, and performance criterions expressed in probability.
Root nodes
CSC (Complexity of supply chain): Degree to which supply chain performance indicators can be evaluated from a technical standpoint from the intricacies of the product.
SES (Similarity to existing supply): Degree to which, similarity and commonality in terms technical attributes of new products can be compared with that of existing products within the firm.