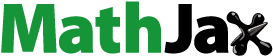
Abstract
The emergence of the Internet has influenced business methods in the world, which made online shopping has become popular due to its practical strengths. Students are one of the potential markets of online shopping in Indonesia. This research investigates the factors influencing university students’ online shopping behaviour in Surabaya as one of the fastest-growing cities in Indonesia, an important issue that has never been explored. The survey dataset is analyzed by using Structural Equation Modeling-Partial Least Squares (SEM-PLS) as well as PLS Predictive-Oriented Segmentation (PLS-OLS) to group the students based on their online behaviour. Both methods are applied due to the fact that the sample size is relatively small. The analysis shows that the students’ online shopping behaviour is significantly influenced by enjoyment, perceived risk, and social influence. Clustering with PLS-POS leads to three segments of students based on behaviour: those mostly influenced by social influence and perceived risk, those influenced by enjoyment and website quality, and those influenced by website quality and trust and security. These results can be a meaningful knowledge and input for the online business owners in Indonesia in designing their marketing strategy.
PUBLIC INTEREST STATEMENT
Online shopping has become a lifestyle nowadays, especially in Surabaya City, Indonesia. Students are the most potential market of the online shopping. There have been numerous online shopping providers and the competition among them is very tight. Therefore, effective and market-based business strategies to attract the potential buyers are essential. One of the strategies is to study the students’ online shopping behavior. This study revealed the important factors influencing the students’ online shopping behavior, i.e. enjoyment, perceived risk and social influence. Therefore, online shopping providers should focus more on those aspects. The website of the online shopping needs to be designed as simple as possible to make the buyers enjoy searching for the products. Guarantee that the online transaction using the platform has to be pointed out too. Moreover, business strategies that make it possible to reach the peer group of buyers need to be formulated such as through social media, which is very popular for university students.
1. Introduction
The Internet has played an important role in daily lives, such as sending messages, other communication, acquiring information, playing games The Internet has introduced the simplicity of doing real-time online shopping with complete features such as looking at the details of the products, price evaluation and comparison, quality assessment, choosing the service type, and processing the payment (Katawetawaraks & Dan Wang, Citation2011). Online shopping provides more information and alternatives to customers about products and price comparisons, as well as providing convenience and simplicity in finding something online. It has been proven also to give more satisfaction to modern consumers with regard to convenience and saving time (Butler & Dan Peppard, Citation1998; Li & Dan Zhang, Citation2002). On the other side, some consumers feel inconvenience with online shopping due to distrust, which thus leads to a negative influence on consumer online shopping behaviour (Katawetawaraks & Dan Wang, Citation2011). Online shopping behaviour can be seen from the process of purchasing products and services through the Internet. Moreover, it also relates to consumer psychological conditions (Li & Dan Zhang, Citation2002). Online shopping behaviour has a significant role in achieving the main goal of e-commerce, which is influenced by internal and external factors. Consumer behaviour has become a focus in many research studies, in particular in the marketing field, because it can significantly support the company’s strategy (Veronika, Citation2013).
Indonesia is one of the potential markets for online shopping. Statistical data have shown that the number of online shoppers has increased over time. In 2016, the number of online shoppers reached 84.2 million in Indonesia, increasing four times compared to the data in 2014, i.e. 21.1 million people (APJII, Citation2016). Among this number, about 18.8 million people did at least once transaction a month. This behaviour is supported by the development of smartphone technology, which offers inexpensive prices and an improvement in the quality of the online network in Indonesia. The Association of Internet Service Providers (APJII) revealed survey results showing that 89.7% of students practice online shopping, ranking them as the top users by percentage. Considering the fact that students comprise the first market for online shopping in Indonesia, it is important to study their shopping behaviour. The information will be important for online shopping providers to improve their business in order to grab this potential market segment.
Many studies have been conducted dealing with online shopping behaviour; however, none of them has been specifically investigating the behaviour of university students in Indonesia, as the biggest and most potential market. The studies have tried to analyse important aspects of online shopping behaviour and have attracted researchers from various countries. Aghdaie, Piraman, and Fathi (Citation2011) analysed the factors affecting the consumer’s attitude of trust and their impact on Internet purchasing behaviour, while Lai and Wang (Citation2012) focused their study on online shopping behaviour. Enrique, Carla, Joaquin, and Silvia (Citation2008) discussed the influence of online shopping information dependency and innovativeness on Internet shopping adoption. Heijden, Verhagen, and Creemers (Citation2003) and Jiang, Chen, and Wang (Citation2008) specifically investigated the influence of the trust perspective on online purchasing intention, while Hernandez, Jimenez, and Martın (Citation2011) focused on consumer characteristics such as age, gender, and income. More country-specific studies have been conducted such as that by Diallo, Chandon, Cliquet, and Philippe (Citation2013), who discussed store brand-customer behaviour in France. The factors influencing consumers’ online shopping in China and Malaysia have been investigated by Gong, Stump, and Maddox (Citation2013) and Harn, Khatibi, and Ismail (Citation2006), respectively. Orapin (Citation2009) carried out a study on the factors influencing Internet shopping behaviour in Thailand. Two other studies carried out by Hu et al. (Citation2009) and Peng, Wang, and Cai (Citation2008) focused on students’ online shopping behaviour, of which the latter study was conducted in China.
To study student behaviour towards online shopping, this research carries out statistical analysis using Structural Equation Modelling (SEM), i.e. a method to represent, estimate, and test the relationship network among variables (latent variables) (Suhr, Citation2006). SEM is a comprehensive statistical approach to test a hypothesis about the relationship between observed and latent variables. SEM has been widely applied in many research studies involving perception and behaviour. Covariance SEM analysis requires a sufficient number of samples, as it is a parametric statistical approach that depends on some assumptions, in particular about the normality of the distribution. The number of samples usually used in SEM ranges from 200 to 800 (Ghozali, Citation2008). In fact, the collected data are not always normally distributed, and the number of samples could be very limited due to some restrictions. Therefore, it is necessary to consider another approach which is flexible and free of assumptions. As an alternative, variance-based SEM, called Partial Least Squares (PLS), can be applied, which focuses on exploration studies (Vinzi, Chin, Henseler, & Wang, Citation2010). SEM-PLS has been applied in many studies such as those of Jamil and Nik Kamariah (Citation2011) and Jamil and Nik Kamariah (Citation2011).
Understanding the online shopping behaviour is still of high interest due to its practical implications, proven by some more recent studies on this issue. Previous studies have identified three to six factors influencing online shopping customer behaviour. Another study conducted by Napitupulu dan Kartavianus (Citation2014) concluded that ease in payment, trustworthiness, and information quality significantly influence the customer decision. Lim, Osman, Salahuddin, Romle, and Abdullah (Citation2016) investigated the relationship between subjective norm, perceived usefulness, and online shopping behavior while mediated by purchase intention among university students. Akalamkam and Mitra (Citation2017) specifically looked at the factors that influence the extent of usage of different information sources in pre-purchase information search by online shoppers. Other research investigating the customer behaviour towards online shopping in Bangladesh and India have been conducted by Rahman et al. (Citation2018) and Jain (Citation2018), respectively. Based on these reasons, this research carries out analysis to find out the factors influencing online shopping behaviour for university students in Indonesia, using a limited sample size. The factors to be investigated are perceived risk, trust, quality of website, enjoyment, social influence, and online advertising. These factors are unobserved and cannot be directly measured, and hence SEM analysis can be applied. The use of a limited sample size led to the choice of using SEM-PLS. Furthermore, the PLS-POS will be applied to observe the heterogeneity among groups toward the online shopping behaviour.
2. Research methodology
2.1. Data source and sampling methodology
The data used in this study are primary data collected from a survey to university students in Surabaya, Indonesia. The questionnaire comprised questions about respondent characteristics and other questions which support the development of relevant indicators to measure the online shopping behaviour. In an initial screening, respondents had to be students who had undertaken online shopping within the last 3 months. The questions were built on a 5-point Likert scale: (1) strongly disagree, (2) not agree, (3) less agree, (4) agree, (5) strongly agree.
Following Scheaffer, Mendenhall, Ott, and Dan Gerow (Citation2012), the following steps were carried out to determine the sample size adopted in this research:
Purpose. The purpose of the survey was to obtain data about students’ online shopping behaviour in Indonesia.
Targeted population. The target of the survey was students in Indonesia (university students in Surabaya city, East Java).
Sampling design. It is assumed that the students' characteristics are homogeneous, and hence, the simple random sampling can be applied to collect the data. The minimum number of samples was calculated with the following formula:
where n is sample size, N is the number of the population, and e is the error tolerance. It is known that the total number of students was 20,448. Using the formula, with 5% error tolerance we obtained n = 393 as the number of samples. The respondents were randomly sampled from the total student population.
2.2. Research variables
The variables collected in the survey were respondent characteristics and the indicators for the latent variables. The respondent characteristics included gender, age, semester, allowance, daily Internet usage, most visited online shopping website (platform), transaction frequency, type of product purchased most often, price interval, type of payment method, and type of used device. The indicators for the latent variables are summarized in Table .
Table 1. Summary of the indicators
Figure depicts the concept design and framework used in this research.
3. Results and discussion
3.1. Respondent characteristics
The survey results reveal that 59% of the respondents were female students, while 41% were male. About 21.7% of respondents used the Internet less than 8 h a day, 57.8% used it for 8–16 h a day, and the rest used it more than 16 h a day. Regarding the frequency of online shopping, 57.7% of respondents made one online shopping transaction per month, 30.1% did online shopping twice a month, and the rest were more than two times. Interestingly, 98.8% of the respondents said that they purchased new products with online shopping. Fashion was the most purchased product type (47%), followed by electronics (19.3%); the rest were for skincare, accessories, internet and mobile credit and household needs. Most of the respondents conducted online shopping from a mobile phone (75.9%), followed by a laptop (21.7%) and other devices (2.4%). A debit card was the dominant payment method (78.3%), compared to e-banking or other types of payment.
3.2. Analysis of factors influencing students’ online shopping behaviour
The first step of the analysis was to evaluate the measurement model as well as the structural model, applied to both the outer and inner model. The result of the analysis is given below.
Validity test (outer model)
The following hypothesis testing was used to show the significance of parameters and indicator variables on the measurement model (outer model) as well as on the structural model (inner model). The hypothesis in PLS included testing the parameters λ and γ, tested by the t-statistic. The significance of the parameters can be evaluated by a resampling bootstrap procedure with a replication of B = 500. The hypothesis is defined as follows:
H0: λi = 0 (the i-th indicator is not significant)
H1: λi ≠ 0 (the i-th indicator is significant)
Using a 5% significance level, the t-statistic was 1.96. Table lists the results of the t-statistic outer model.
Table 2. Testing for measurement model (outer model)
We can see that the loading factors of all indicators are greater than rtabel = 0.213 and the t-statistics are greater than 1.96. This indicates that all indicators are valid and significant, and hence they are valid indicators for the corresponding latent variable.
Reliability test (outer model)
The reliability test is intended to see whether the indicators are reliable enough to measure the latent variable. The reliability can be measured by the composite reliability value; it is reliable if the composite reliability value is greater than 0.7.
Table shows that the composite reliability values are all above 0.7, which means that all indicators are reliable enough to measure the latent variable.
Table 3. Reliability test
Evaluation of structural model (inner model)
The main goal of this part is to investigate the predefined factors influencing the students’ online shopping behaviour. After having a valid and reliable measurement model, we need to evaluate the structural model. The evaluation of the structural model (inner model) is used to assess the relationship among variables. This part is basically to investigate variables influencing the students’ online shopping behavior. The evaluation is conducted by looking at the R-square (R2) and Q-square Predictive Relevance (Q2). The R2 shows the ability of the exogenous latent variable to explain the variability of the endogenous variable. The Q-Square Predictive Relevance (Q2) is used to validate the ability of the model to predict. If the Q2 closes to 1, it can be said that the structural model has a relevance prediction. The hypotheses to test the structural model are as follows:
H0: 0 vs H0:
0
by which the following variable relationships are tested:
Enjoyment towards online shopping behaviour
Online advertising towards online shopping behaviour
Perceived risk towards online shopping behaviour
Quality of website towards online shopping behaviour
Social influence towards online shopping behaviour
Trust and security towards online shopping behaviour
The results of the tests can be seen in Table .
Table 4. Structural test (inner model)
Table shows that the t-statistics of three variables (enjoyment, perceived risk, and social influence) are greater than 1.96, which means that those variables significantly influence the students’ online shopping behaviour. The t-statistics for three other variables (online advertising, quality of website, and trust and security) are lower than 1.96, indicating that those three variables do not significantly influence the students’ online shopping behaviour.
The loading factor values show the strength of the relationship between each latent variable and online shopping behaviour. The highest loading factor is the perceived risk (in absolute value). However, the value is negative, indicating that the higher the risk of shopping based on the student’s perception, the lower the incentive for doing online shopping. This suggests that producers really have to take the security issue into account. The website has to be designed so that students are convinced that there will be no or low risk by proceeding with an online transaction. The second highest loading factor is social influence, with a positive value. This indicates that the student's intention for doing online shopping was highly influenced by that person’s community. It is reasonable to have this result, considering the fact that students are at an age when they can be easily influenced by external factors (people in their environment). There are many cases in Indonesia showing that students took bad actions simply because they wanted to have something similar to what their peers had. From the perspective of the producer, market segmentation will be an important step to be carried out. The third highest loading factor is for the enjoyment variable. It is worth noting that students may spend a lot of time online (as revealed in this survey), simply scrolling and browsing through interesting products. Online shopping offers a high level of enjoyment compared to traditional shopping. It can be done anytime, everywhere, and provides many choices, which thus saves a lot of time. The other three variables do not significantly influence students’ online shopping behaviour.
Based on the values in Table , the structural model involving only significant variables can be written as
The enjoyment offered by online shopping induced the students to prefer online over conventional shopping. For a potential customer with limited time because of a study load or workload, online shopping can be an interesting choice due to its practicality. The availability of various brands and types of goods makes online shopping even more enjoyable. The perceived risk toward online shopping is mostly due to the missing interaction between customer and seller, as well as the intangibility of the product, i.e. the quality of the product cannot be directly seen by the customer. The customer often thinks about the risk of getting a poor quality or defective product, or worries that the product will not be sent by the seller. Social influence, through conversation or interaction as well as recommendations about new products, tends to increase the interest in online shopping.
Clustering with PLS-POS
The PLS-POS is one of the segmentation methods oriented to predicting the relationships among constructs. The purpose of this analysis is to cluster the students based on their behavior characteristics, e.g. the factors influencing their intention to online shopping behaviors. The first step in conducting a PLS-POS analysis is to form the initial segmentation. Finding the optimal number of segments (between k = 2 and k = 3) can be done by comparing the average-weighted R-square. The average weighted R-square is the R-square weighted based on the number of groups. The values of the average weighted R-square are given in Table .
Table 5. Average weighted R2
From the table, we see that the average weighted R-square for k = 3 is greater than k = 2, and hence, forming three clusters is the best choice. The percentage of each segment corresponding to the number of respondents in the cluster can be seen in Table .
Table 6. Percentage of respondents in each segment
Table presents more detail about the characteristics of respondents in each segment.
Table 7. Respondent clusters based on segment
Based on the gender and intensity of online shopping, there is little difference between segments 1, 2, and 3. The favourite website is Shopee, which refers to the fact that segments 1 and 2 mostly buy products from Shopee and Tokopedia, while segment 3 prefers to shop from Shopee and Lazada. This seems to be related to some features provided by the platform, as well as the discount and free shipping cost. In fact, Shopee was elected as the most attractive online shopping platform in Indonesia in 2018. Segment 1 consists of students who spend more money on online shopping compared to segments 2 and 3. All segments mostly purchase fashion and electronics. This analysis is able to reveal information about the latent variable which tends to influence online shopping behaviour for each segment. The rate of influence between exogenous latent variable and endogenous latent variable in each class segment is shown in Table .
Table 8. Path coefficient
Table presents a comparison of coefficients on the structural model analysed globally as well as on each segment. Respondents in segment 1 have the perception that enjoyment does not really relate to the decision to carry out online shopping. They argue that perceived risk has a strong negative influence on online shopping, while social influence is the most influential variable for online shopping. Other variables such as online advertising, trust and security, and the quality of the website have a positive impact on online shopping. In contrast to segment 1, students in segment 2 argue that enjoyment strongly influences their decision to undertake online shopping, while social influence has only a weak influence. Segment 3 consists of students who argue that the quality of the website strongly influences their online shopping behaviour. Enjoyment is also one of the important factors.
4. Conclusions
Based on this analysis, we found that most of the online shoppers were female students who spent about 100,000–200,000 IDR for purchasing fashion products at Shopee, which was their favourite platform. Male students tended to buy electronics from Tokopedia. Due to the small sample size, it was not feasible to apply the standard SEM approach, and hence SEM-PLS was applied. The analysis using SEM-PLS showed that enjoyment, perceived risk, and social influence are three factors that significantly influence the university students in conducting online shopping. In order to determine the specific target of students' segment as the potential market, clustering the students' characteristics has been carried out. Clustering the students using PLS-POS revealed that there were three different segments related to online shopping behaviour. For segment one (59.036% of respondents), online shopping tends to be influenced by social influence and the perception of risk. For segment two (19.277%), the decision to shop online is influenced by enjoyment and the quality of the website. The last segment is concerned with the quality of the website and trust and security. These results are based on a survey conducted in Indonesia. Considering the fact that students’ characteristics and lifestyles might be similar among university students in Indonesia, the results can, therefore, be generalized for a general case of university students in Indonesia. The analytical method can be applied to other cases with limited sample size.
Additional information
Funding
Notes on contributors
Heri Kuswanto
Heri Kuswanto is an Associate Professor at the Department of Statistics, Institut Teknologi Sepuluh Nopember (ITS) Indonesia. He has received his Ph.D. from the School of Economics and Management, Leibniz Hannover University Germany. His research interest includes econometrics, data analysis and computational statistics.
Wildan Bima Hadi Pratama
Wildan Bima Hadi Pratama is a student at the Department of Statistics-ITS. He graduated from the Department of Statistics, ITS. His interest includes marketing research and multivariate analysis.
Imam Safawi Ahmad
Imam Safawi Ahmad is an Assistant Professor at the Department of Actuarial Science-ITS. He has received his Master from the Department of Statistics ITS. His research Interest includes marketing research, business statistics and actuarial science.
Mutiah Salamah
Mutiah Salamah is a senior lecturer at the Department of Business Statistics-ITS. She graduated from Airlangga University, Indonesia. Her research interest includes Business statistics and regression analysis.
References
- Aghdaie, S. F., Piraman, A., & Fathi, S. (2011). An analysis of factors affecting the consumer’s attitude of trust and their impact on internet purchasing behaviour. International Journal of Business and Social Science, 2(23), 147–13.
- Akalamkam, K., & Mitra, J. K. (2017). Consumer pre-purchase search in online shopping: Role of offline and online information sources. Business Perspectives and Research, 6(1), 42–60. doi:10.1177/2278533717730448
- APJII. (2016). Infografis penetrasi dan perilaku pengguna internet Indonesia 2016. Jakarta: Author.
- Butler, P., & Dan Peppard, J. (1998). Consumer purchasing on the internet: Processes and prospects. European Management Journal, 16(5), 600–610. doi:10.1016/S0263-2373(98)00036-X
- Diallo, M. F., Chandon, J.-L., Cliquet, G., & Philippe, J. (2013). Factors influencing consumer behaviour towards store brands: Evidence from the French market. International Journal of Retail & Distribution Management, 41(6), 422–441. doi:10.1108/09590551311330816
- Enrique, B. A., Carla, R. M., Joaquin, A. M., & Silvia, S. B. (2008). Influence of online shopping information dependency and innovativeness on internet shopping adoption. Online Information Review, 32(5), 648–667. doi:10.1108/14684520810914025
- Ghozali, I. (2008). Generalized Structured Component Analysis (GSCA). Semarang: Universitas Diponegoro.
- Gong, W., Stump, R. L., & Maddox, L. M. (2013). Factors influencing consumers’ online shopping in China. Journal of Asia Business Studies, 7(3), 214–230. doi:10.1108/JABS-02-2013-0006
- Harn, A. C., Khatibi, A., & Ismail, H. (2006). E-commerce: A study on online shopping in Malaysia. Journal of Social Science, 13(3), 231–242.
- Heijden, H. V., Verhagen, T., & Creemers, M. (2003). Understanding online purchase intentions: Contribution from technology and trust perspectives. European Journal of Information Systems, 2(1), 41-48.
- Hernandez, B., Jimenez, J., & Martın, M. J. (2011). Age, gender and income: Do they really moderate online shopping behaviour? Online Information Review, 36(1), 113–133. doi:10.1108/14684521111113614
- Hu, Y., Sun, X., Jing, Z., Zhang, X., Luo, F., & Huang, L. (2009). A university student behavioral intention model of online shopping based on TAM. International conference on information management, innovation management and industrial engineering, Xian-China. IEEE Computer Society (pp. 625–628).
- Jain, D. (2018). Analysis of consumer buying behavior towards online shopping: A case study of Kota city- Rajasthan. Retrieved from https://www.researchgate.net/publication/323998478_Analysis_Of_Consumer_Buying_Behavior_Towards_Online_Shopping_A_Case_Study_Of_Kota_City-_Rajasthan
- Jamil, N. A., & Nik Kamariah, M. N. (2011). To investigate the drivers of online purchasing behavioral in Malaysia based on the Theory of Planned Behavior (TPB): A Structural Equation Modeling (SEM) approach. International Conference On Management, Penang-Malaysia (453–460).
- Jiang, J. C., Chen, C. A., & Wang, C. C. (2008). Knowledge and trust in e-consumers’ online shopping behavior. International symposium on electronic commerce and security, Guangzhou-China. IEEE Computer Society (pp. 652–656).
- Katawetawaraks, C., & Dan Wang, C. L. (2011). Online shopper behavior: Influences of online shopping decision. Asian Journal of Business Research, 1(2), 1–9. doi:10.14707/ajbr.112
- Lai, E., & Wang, Z. (2012). An empirical research on factors affecting customer purchasing behavior tendency during online shopping. Proceeding of the 2012 IEEE International Conference on Computer Science and Automation Engineering, Beijing-China.l- 583–586.
- Li, N., & Dan Zhang, P. (2002). Consumer online shopping attitude and behavior: An assessment of research. Information System Proceedings of English Americas Conference, Dallas-Texas, (pp. 508–517).
- Lim, Y. J., Osman, A., Salahuddin, S. M., Romle, A. R., & Abdullah, S. (2016). Factors influencing online shopping behavior: The mediating role of purchase intention. Procedia Economics and Finance, 35, 401–410. doi:10.1016/S2212-5671(16)00050-2
- Napitupulu dan Kartavianus. (2014). A structural equations modeling of purchasing decision through E-commerce. Journal of Theoretical and Applied Information Technology, 60(2), 358–364.
- Orapin, L. (2009). Factors influencing internet shopping behavior: A survey of consumers in Thailand. Journal of Fashion Marketing and Management, 13(4), 501–513. doi:10.1108/13612020910991367
- Peng, H., Wang, C., & Cai, J. (2008). An empirical investigation on the adoption of online hopping of university students in China- international seminar on business and information management (pp. 498–501). Wuhan: Wuhan University.
- Rahman, M. A., Islam, M. A., Esha, B. H., Sultana, N., Chakravorty, S., & Molnar, A. (2018). Consumer buying behavior towards online shopping: An empirical study on Dhaka city, Bangladesh. Cogent Business & Management, 5, 1514940. doi:10.1080/23311975.2018.1514940
- Scheaffer, R. L., Mendenhall, W., III, Ott, R. L., & Dan Gerow, K. (2012). Elementary survey sampling seventh edition. Boston: Brooks/Cole Cengage Learning.
- Suhr, D. (2006). The basics of structural equation modeling. Colorado: University of Northern Colorado.
- Veronika, S. (2013). Journal of competitiveness: Motivation of online buyer behavior. Pardubice: University of Pardubice, Faculty of Economics and Administration.
- Vinzi, V., Chin, W., Henseler, J., & Wang, H. (2010). Handbook of partial least square: Concept. Method, and application. Heidelberg: Springler-Verlag Berlin.
- Yu, T., & Dan Wu, G. (2007). Determinants of internet shopping behavior: An application of reasoned behavior theory. International Journal of Management, 24(4), 744–762.