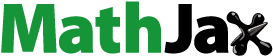
Abstract
In recent years, the relationship between doctors and patients is becoming more and more tense. Many hospitals are paying more attention to the satisfaction of patients, because this is an important indicator to measure the quality of hospital services. In China, it is often a case that large-scale comprehensive hospitals such as the tertiary hospitals are always overcrowded and the waiting time for treatment is much higher than the time of treatment. The long waiting time will undoubtedly lead to lower patient satisfaction. Thus, how to improve patient satisfaction is focused on how to reduce patient waiting time. This study is based on the discrete event simulation (DES) method to construct an optimization model for the dental service process of a tertiary hospital in Changzhou. The data is collected through field research, and then we set the model parameters according to the existing service model. We suggest that adding pre-examination into the nursing triage process can improve the hospital’s service quality and enhance the resource utilization under limited resource conditions with reducing patient waiting time. In addition, we can inspire the hospital and other medical institutions to do some further improvements. After applying the proposed model, it can be seen that the effect is very significant that the average waiting time for treatment of the patient is reduced from 173 minutes to 45 minutes, and the length of stay (LOS) of the patient in the hospital is reduced from 209 minutes to 130 minutes.
PUBLIC INTEREST STATEMENT
As we all know, overcrowding in hospitals is a serious problem in China, leading to tense relationship between doctors/nurses and patients. Since patients’ satisfaction is closely related to their waiting time for treatment, hence, reducing patients’ waiting time can improve the efficiency of hospitals and both doctors’ and patients’ satisfaction. In order to achieve that goal, this study constructs an optimization model for the dental service process based on the discrete event simulation (DES) method. We suggest that adding pre-examination into the nursing triage process can improve the hospital’s service quality and enhance the resource utilization under limited resource conditions with reducing patient’s waiting time. In addition, the new process and strategy could be implemented in other departments and medical institutions.
1. Introduction
An economic boom over the past three decades has transformed China from a poor farming nation to the world’s second-largest economy. With an increasing development of science and technology, the living standards of public has been improved a lot, contributing to higher demand and requirements of medical service quality. Meanwhile, people’s life expectancy has been improved and the maternal mortality rates are lowered. However, the system cannot adequately support China’s population of more than one billion people. The characteristics, large population base and limited per capital resources, have caused serious social pressure on various medical institutions, such as doctor–patient relationship issues and hospital congestion problems, which make hospital service quality difficult to be improved with a low patient satisfaction. It is also easy for medical staff to generate negative emotions, leading to more serious problems. The major gaps and inequalities threaten to undermine China’s progress, social stability and financial health.
Recently, the health care industry in China has experienced challenges such as overcapacity, unhealthy relationship between doctors and patients, which have made government and some related administrations identified how to resolve such a serious issue. The last few decades have witnessed that Chinese government has been sparing no effort in alleviating pressure on public by taking some measurements and enacting some policies. Even though there are some effective approaches implemented to improve services, such as hierarchical medical system, multi-level medical security system, we still cannot ignore the fact: overcrowded in the hospital, low satisfaction with patients, long waiting time for services. Therefore, in order to change this situation, improving the quality of hospital services and patients’ satisfaction have become a top priority consideration for hospital management departments and government departments. He, Cai and Wang evaluated resource allocation for optimal use of medical workers for adjusted registration procedure in West China Hospital of Sichuan University. They used data to construct a simulation model of registration procedure for this hospital (He et al., Citation2016). At the same time, according to the literature research and surveys, we can find that the waiting time in the queue and the length of staying in the hospital are important indicators that affect patients’ satisfaction. Needless to say, how to reduce the waiting time of patients is very crucial.
Based on the field research of the department of stomatology in the tertiary hospital in Changzhou, this paper builds a DES simulation model via AnyLogic software based on the existing service process and layout. Several interviews will be conducted with the doctors, nurses and patients from the territory-tier hospitals. The data collected through the survey is used to set parameters, such as patient arrival rate, distribution type, average registration time, average waiting time. Then, we found that the patient arrival report sequence has a significant impact on hospital performance through process analysis and literature analysis. According to the information entropy theory, we have done the entropy calculation for the original service system based on the patient type and found that the information entropy value is very high, which means that the system is extremely chaotic. Thus, in part simulation model design, we consider reducing the patient type as much as possible to increase the order of the system, scientifically proposing the pre-examination during the nurse triage process optimization model. With the same resources and parameters, the average waiting time of the patient and the average length of stay in the hospital were reduced by 117 minutes and 79 minutes, respectively, and the effect was significant and could be extended to other hospitals. Finally, the corresponding constructive suggestions would be given in our conclusion.
2. Literature reviews
2.1. The application of DES in healthcare
Recently, health expenditures have increased rapidly while the proportion of the population with access to health care has declined. With the widespread of the computer technology, DES has gradually been applied to healthcare, economy, and logistics and transportation fields to solve that problem. Over the last 30 years, there is an increasing trend of publishing paper on healthcare field with DES model with no sign that the flow will cease. Some authors chose DES model to estimate the relative effects and cost-effectiveness and DES is one of the most common modelling techniques in Health Technology Assessment (McMeekin et al., Citation2019). Kotiadis and Tako have been dedicated into this field for many years and published a paper about a facilitated post-model coding in DES. They explain in more detail the facilitation process and the tools used to support of stakeholders with a case study in healthcare (Kotiadis & Tako, Citation2018). Tako and Kotiadis propose a framework that is designed to support the modelers’ interaction with a group of stakeholders for healthcare, called PartiSim, which stands for DES (Tako & Kotiadis, Citation2015). Robinson shows empirical evidence of carrying out facilitated model with a group of healthcare professionals at an outpatient’s eye clinic, called SimLean (Robinson et al., Citation2014). Through a plethora of reviews, MM Gunal and M Pidd concluded that publications are divided into five categories: hospital scheduling and organization, infection and communicable disease, costs of illness and economic evaluation, screening, and finally miscellaneous (Gunal & Pidd, Citation2010). Jun and Fone classifies models into three parts according to the objectives of the studies of which they are part: scheduling and patient flow, sizing and planning of beds, rooms and staff, a discussion on future-research areas (Fone et al., Citation2003; Jun et al., Citation1999).
With comprehensive analysis of previous scholars’ research, most of literature research is concentrated on specific units such as emergency departments, outpatient departments, etc., to provide specific solutions for specific hospitals. Few modeling experiments for the entire hospital, or to propose a general model approach is due to the complexity of the overall modeling and the difficulty of data collection. In addition, with regard to the application of the DES simulation model, few scholars have given specific implementation procedures. In most of the literature, they are used as only the auxiliary testing tools. There are relatively many methods of using operational planning and empirical research.
On the other hand, on the basis of DES modeling, there are many researches on patient classification based on scheduling. Some scholars have proposed to divide patients into new patients which means they have not received treatments and return patients which means they return to secondary treatments (Cox et al. Citation1985), and others have proposed to classify according to the variability of treatment service time by doctors (Klassen & Rohleder, Citation1996). It has also been suggested to make a category according to the type of process (Vanden et al., Citation2000). Tugba Cayirli, Emre Veral and Harry Rosen proposed patient arrival sequence such as NRNR …, has a significant impact on the efficiency of the outpatient clinic, even exceeding the importance of the appointment rules based on empirical research of the classification scheme of “new/return”, which means new arrival for the initial diagnosis and return to the secondary treatment (Cayirli et al., Citation2006). Most of problems related to the triage work are mainly concentrated on the emergency department, while the literature in the outpatient clinic is relatively small, with the focus mainly on the serious disease triage based. There is very little information about the nurses’ pre-screening work assisting to the doctors in a certain department. In addition, the accuracy of the triage is considered to be the level of triage of nurses and experts with the same assigned patients (Farrohknia et al., Citation2011). However, the concept of triage accuracy is slightly different from the existing reliability research concept. The reliability of the triage system includes the consistency of various types of evaluators between nurses and nurses, as well as between nurses and specialists, and the accuracy of triages only calculates the consistency between nurses and experts. Based on the domestic and international literature, we can see that DES is a universal and effective simulation modeling method in medical process analysis.
2.2. The application of entropy information in system analysis
The concept of entropy was first proposed by the German physicists R. Clausius and L. Boltgman (Durowoju et al., Citation2012), originally used in thermodynamics to measure the degree of molecular state chaos, and then N. Wiener and C.E. Shannon, the American mathematician and the founder of cybernetics and information theory, proposed a broader information entropy to describe the uncertainty of the source and solve the problem of quantitative measurement of information (Nakagawa & Yasui, Citation2003).
Information entropy is defined as the macroscopic description and overall order measuring the system structure (Liu, Citation2012). Since there is a certain relationship between entropy and chaos, that is, the larger the entropy value, the more chaotic the system, the greater uncertainty, the lower the order and vice versa. The application of information entropy is very extensive and has been infiltrated in various disciplines. Beginning in the late 1980 s, with the concept of “complexity science”, information entropy has been gradually applied to economy and management fields, especially in the research of the utility of organizational structure.
In recent years, many scholars have introduced information entropy into management science, some based on information entropy theory to do enterprise’s competitive advantage evaluation research, using gray correlation and information entropy combined with relevant theoretical methods to study the quantitative analysis of enterprise development. So that we can assess the competitive advantage of enterprises effectively and scientifically (Zhao et al., Citation2004). And some analyze the evolutionary nature of management systems from order to disorder according to the principle of entropy increase (Wu & Hu., Citation2008). There are also some establishing the vector spatial approach of enterprise management system complexity evaluation, extending the scope of research on the complexity of enterprise management organizations (Liu & Jiang, Citation2003). Some use entropy theory to analyze the role of entropy in enterprise organizational innovation and new strategic models, from three-dimensional perspectives of management information, functions and structures, discussing about the quantification of complexity of enterprise system management with a new dimension proposed to measure the complexity of enterprise system management: management entropy (Song et al., Citation2003). At the same time, information entropy can be introduced into various evaluation mechanisms as one of the important tools of quantitative research. For example, the information entropy theory is used to quantitatively study the evolution and development of regional transportation structure, and the information entropy of transportation structure is proposed by building index of transportation structure and using the principal component analysis method and the gray correlation analysis combined to demonstrate (Yu et al., Citation2011). In addition, the research on the evaluation of community information resource allocation is based on the conditional information entropy through rough set approach (Xie et al., Citation2013). In some fields, entropy plays a critical role not only in measuring for tweet & apos in the new media like Twitter but also in comprehending uncertainty reduction theory and emoticons as a way to convey information (Son et al., Citation2019). Plus, there are some applications of information entropy in healthcare, such as using information entropy to evaluate the classification efficiency of a threshold point of the expression level boundary for lung cancer (Zhao et al., Citation2019). With information entropy and three-parameter interval, information security risk assessment is proposed so that to increase the accuracy of information processing (Huang et al., Citation2018). We draw on the research of predecessors and introduce information entropy to help us clarify the direction of optimizing the improvement process and improve the orderliness of the whole system.
3. Simulation model design
3.1. Setting parameters
This paper uses AnyLogic 8.3.3 software for DES modeling and simulation as shown in Figure . The simulation time is 500 minutes. It is more realistic to simulate the operation of the entire stomatology department through the field investigation of a large hospital in Changzhou. According to interview records with doctors and nurses, the stomatology department has the highest number of visits on Monday. The average number of visits from Tuesday to Friday in the morning is about 120, and the average in the afternoon is 90. The average number of people on the workday is about 210. On average, the number of doctors working in the stomatology department is 10, and the number of nurses is 4. The number of technicians is 4, and there are 10 working windows with available facility and equipment (we will call it service windows in the next part). However, according to the survey, the types of patient visits are mainly concentrated on three service windows, and the remaining service windows have few patients. And even if there are some patients, the consultations will last for up to 2 hours, so considering the generality and effectiveness of the model, we set up 3 service windows as working simultaneously in the model, one X-Ray radiology equipment, and thus the number of resources for the model has been set up as shown in Table .
Table 1. Setting of parameters of resources
According to the statistics of the arrival rate of patients, it is found that there is one patient arriving at the registration area every 30 seconds. In order to simulate the situation in stomatology department more realistically, we use the actual arrival rate of 0.5 person/minute as the value of the parameter patient arrival rate λ. Most of the parameters are set with the triangular (min, max, mode) distribution in minutes. We set the value of v, walking speed of the patient at different nodes, obey the triangular (min, max, mode) distribution based on the distance and walking time between each node, such as the actual distance from the registration area to the stomatology department. Since we assume to apply our collected data as much as possible and triangular distribution is appropriate for computer simulation and decision making, we decide to use triangular distribution instead of Poisson distribution or others that researchers are usually used to. Similarly, we have set up the parameter WT_R, waiting time in the queue for registration, obeying the triangle distribution triangular (2, 20, 14) according to our observations. There are 8 manual registration service windows with the average waiting time 16 minutes, and the average time of automatic-service machine is 2 minutes based on our sample statistics during the period between 8.45am and 9.45am. Besides, the queuing service rules are all set to FIFO, which is a first-come, first-served service, regardless of the priority service factor. In the same way, we have collected some data of the patients in the stomatology department, including waiting time for the doctor’s service between 9:45am and 16:00pm, and calculated the maximum, minimum and average values. Considering the different types of patients, if some are newly diagnosed, and some are finished but seek for treatment again after the examination. Therefore, we set two different waiting parameters, containing WT_NPNEW (waiting time for service of new patients) and WT_NPRETURN (waiting time for service of return patients), obeying the triangular distribution (3, 100, 70) and triangular distribution (3, 60, 50) respectively. The same doctor’s treatment time can also be divided into ST_NEW (service time for new patients) and ST_RETURN (service time for return patients), but because the statistical test shows that there is no significant difference between the two, and in order to reduce the impact of other parameters of the model on the general optimization, we unified the two sets of doctor service time parameters ST obeying the distribution of triangular (3, 15, 5), radiology X-Ray test time XT obeying the distribution of triangular (5, 7, 6), and the specific relevant parameters are set as shown in Table .
Table 2. Setting of parameters in the service process
3.2. The process design and analysis of information entropy
Based on the settings of each parameter and the real operation of the hospital, we designed a dental service flow chart as shown in Figure . After 500 minutes of simulation running, the statistics shows that a total of 204 patients arrived, but only 72 patients left. Plus, the average waiting time for registration of patients was 12 minutes and the average waiting time for doctors of patients was 173 minutes. The average LOS was 209 minutes, and the utilization of each resource is shown in Table . We calculated utilization by setting the speed of doctors and nurses as uniform distribution (meters per second) and setting X-ray and stomatology department service as static agent types. Through simulation and test, we want to achieve more efficient healthcare procedure for both doctors and patients by designing a new process instead of adding up more resources such as doctors and nurses. With increasing the number of service windows, the overall efficiency can be effectively improved, which is consistent with the reality. At the same time, we analyzed the process and found that the bottleneck of the process is waiting for the nurse to call for medical treatment, so further analysis and testing is carried out for waiting for nurse this process.
Table 3. The resources utilization of the original process model
Information entropy is used as an indicator to illustrate the process order of the stomatology department. To be specific, it is one of the theories to support our new design model which could not only reduce patients’ waiting time but also improve the efficiency of the hospital with lower disorderliness. According to the information entropy theory and data collected from the simulation, we calculated the information entropy of the whole system with the value up to 0.989 based on the Formula (1), which means that the system is highly uncertain. Furthermore, because of different types of patients, including new patients and return for further treatment patients, the sequence of treatment orders is irregular, leading to high chaos in the system. Therefore, so as to optimize the entire service process and reduce the chaos in the system, we have to find some ways to reduce the information entropy.
Since we divide patients as two categories, new patients and return patients, we use the binary information entropy function. In this function, we can easily get that there should be a maximum number only if p1 = p2 = 0.5. If we can enlarge the gap between p1 and p2 as much as possible, we can reduce the information entropy significantly. Therefore, we will follow this principle to try to eliminate the type of return patients leading to more proportions of new patients and less proportions of return patients in the stomatology department so that we could get smaller number of information entropy in the system.
Combining with the literature and our tests in the simulation model, we proposed to add pre-examination into the nursing triage process which means reducing the number of return patients as many as possible. It is often a case that new patients spent several hours in waiting for doctors’ treatment, but only with 2–3 min consultant and then would be arranged to do some examinations, such as X-ray. They waste so much time on waiting instead of doing examinations in advance. However, it is very easy to avoid that problem. Firstly, nurses have a good command of professional knowledge and they have accessed to patients for so many years so that they are qualified to give the initial suggestion whether the patient needs to do pre-examination like simple X-Ray or not. Secondly, they have abilities to do the triage process with rich experience, even more than those doctors who just graduated from the university. Thirdly, we can find that nurses have more free time compared with doctors so that it is accessible for them to do that job. Finally, adding pre-examination into the nursing triage process could not only enable the hospital to take a good advantage of human resources, but also optimize the entire process and improve the efficiency with lower disorderliness. Therefore, considering the theoretical and practical factors, we redesign the process model as shown in Figure , and make optimization based on the original model under the same parameters. Then, we make a comparison between the original process model and new model to observe whether the parameter WT_NP and LOS have decreased significantly.
4. Results and discussion
As mentioned above with the analysis of the hospital based on information entropy theory and our process model design, we use the AnyLogic 8.3.3 tool to run the model and get significant results compared with the original process simulation. We make a comparison from several dimensions: the number of patients arriving and leaving, the average waiting time, the average length of stay (including maximum, minimum values and standard deviation of length of stay of patients in the hospital) and the average treatment time. Finally, we compare the utilization of various resources of the hospital. Multiple dimensions validate the effectiveness of the optimized process we designed.
After running the simulation test and the data obtained, as shown in Table , we can clearly see that the waiting time for the doctor’s treatment of the patient is significantly reduced from the original 173 minutes to 45 minutes, with an average reduction of 2 h of waiting time. The overall length of stay (LOS) in the hospital has been significantly reduced from 209 minutes to 130 minutes, which means that the patient may only be necessary to stay in the hospital for 2 hours, rather than going to the hospital early in the morning to waiting in the queen and spending the whole morning time in the hospital. And the standard deviation of length of stay goes down from 72.52 to 52.23, with lower variance. It means that the length of stay of patients is more stable than the original situation. It is obvious that new process model has greatly helped patients save time and improved the efficiency of patients looking for medical treatment, thereby greatly improving patient satisfaction. What’s more, according to the ratio of the number of patients leaving divided by the number of patients arriving, from 35.12% to 57.38%, it is obvious that the efficiency of the patient flow has been improved a lot in the hospital. At the same time, we can find that, without changing the amount of resources, we have made full use of the existing resources, and the utilization rate of each resource has been significantly improved, especially for the utilization of X-Ray equipment which has increased from 38% to 94%, as shown in Table .
Table 4. The comparison of statistic parameters between the original process model and new model
Table 5. The comparison of resources utilization between the original process model and new model
Furthermore, we take the information entropy into a consideration to verify our results. As we mentioned about how to calculate the information entropy before, we have done a demonstrable analysis about the formula. When the possibilities of different types of patients showing in the hospital are same, there is a maximum value of the information entropy. In other words, if the number of new patients and return patients are extremely closed, even equal, the information entropy is closer to the peak value, which means more disorderliness in the system. Although the principle of increase of entropy is widespread in nature and everything has an inclination to develop toward a state of freedom with the more chaotic system, however, we can make some changes artificially to increase the order of the system, such as reducing the disorderliness of the system from the perspective of reducing patient types through process design. The optimized process significantly reduces the number of return patients who return to the clinic due to no pre-examination before visiting the doctor. In other words, the number of first-time coming patients increases, contributing to widening the gap between the two. Therefore, the system’s information entropy is declined and the degree of system chaos will also be reduced. Further explanation, the service of the stomatology clinic in the hospital is more orderly than the original.
Overall, according to our data from the simulation and analysis of information entropy, there is no doubt that hospitals can effectively prompt their process and operation efficiency by adding the pre-examination into nursing triage process.
5. Conclusions
The professional in hospital has pointed out that nursing triage is mainly to assign patients to different doctors in terms of the priority of patients’ sequence and the specific work progress in the stomatology department, with acquiring the patient’s demand first. Consequently, it can be seen that there is a great room for optimization and improvement in the nurse triage processing. According to our comprehensive simulation test and data analysis, we draw the following important conclusions:
The efficiency could be improved a lot when adding the pre-examination into nursing triage process and make a preliminary diagnosis for the patient in advance. For example, if a patient needs to remove the wisdom tooth but does not have the X-Ray image of his or her teeth, then it is necessary to take an early shot to help the doctor to successfully treat the patient and make the doctor service process smoother and more efficient.
Adding the pre-examination into nursing triage process effectively reduces the overall length of stay of patients in the hospital and the waiting time for doctors to treat them. The effect is significant, thus improving the satisfaction of patients.
Adding the pre-examination into nursing triage process can fully increase the resource utilization rate under the premise of the existing resources, reducing the additional cost for the hospital, and improve the operational efficiency of medical institutions.
Adding the pre-examination into nursing triage process significantly reduces the information entropy of the whole system and improves the stability and orderliness of the entire service system.
The strategy, pre-examination for patients in the nursing triage process, is easy to extend to other departments in the hospital so that boost the entire service efficiency of the hospital.
The DES simulation model can be well applied to medical modeling. With AnyLogic software, it is convenient and simple to visualize the model’s process optimization design, and other simulation methods can be combined to achieve a model using multiple simulation methods, which is a good tool for simulation.
Although the simulation model was designed based on the stomatology department of a hospital, the conclusion is not limited to a specific hospital or a specific department. Nurses exist in all departments of the hospital and have an irreplaceable role. Adding the pre-examination into nursing triage process, patients could be checked by nurses in advance and this can be extended to other outpatient departments. Only with some training for nurses, they can effectively help doctors complete the initial diagnosis work, which relieves the doctor’s work to some extent. For patients, the new process model could save a lot of waiting time for treatment. For hospitals, pre-examination by nurses can improve the utilization of existing resources, and further boost the efficiency of the entire service process. The service performance of the hospital has been significantly improved, and the patient’s satisfaction has also increased, thus alleviating the tension between doctors and patients, which is beneficial to the government and the society, achieving a win-win effect.
However, although the nurse has certain professional knowledge and ability, after all, they have to replace the doctor to make a decision whether patients need to do X-Ray, thus there is still a certain risk, such as the patient’s distrust for nurses’ capability. According to some experts from hospitals, it is said that the strategy could be realized to transfer some certain rights to nurses, which should be written into official regulations. Therefore, adding the pre-examination into nursing triage process requires training and guidance for the nurses in the early stage, so as to ensure that the accuracy of the examinations performed on the patients, and make sure that patients are psychologically assured that the early diagnosis by nurses is not significant to doctors. Eliminating differences of pre-examination decided by nurses or by doctors in patients’ thoughts can ensure the smooth progress of the treatment process. In addition, this measurement only involves preliminary examinations, and does not cause any substantial harm to the patient’s body, condition, etc., so the risk itself is very low, let alone coupled with the previous training and guidance for nurses.
In conclusion, the optimization strategy, adding the pre-examination into nursing triage job, is a good choice both in terms of theoretical effects and operational feasibility.
Additional information
Funding
Notes on contributors
Qiaodan He
We all collaborated together to dedicate into our innovative research. Everyone has made their own contributions to this study. Ms. Qiaodan He focused on designing the new process and doing DES simulation by using AnyLogic. Besides, she conducted the survey and talked with some doctors and nurses working in the stomatology department. Mr. Huo is the supervisor of our research project and provides resources for our research. Meanwhile, he has given some sensible suggestions for our study. Ms. Pan is a professional doctor in the hospital and she is responsible for providing data and practical advice for our research project. Because of the perfect cooperation, we have completed this study successfully.
References
- Cayirli, T., Veral, E., & Rosen, H. (2006). Designing appointment scheduling systems for ambulatory care services. Health Care Manage Science, 9(1), 47–14. https://doi.org/10.1007/s10729-006-6279-5
- Cox, T. F., Birchall, J. F., & Wong, H. (1985). Optimizing the queuing system for an ear, nose and throat outpatient clinic. Journal of Applied Statistics, 12(2), 113–126. https://doi.org/10.1080/02664768500000017
- Durowoju, O. A., Chan, H. K., Wang, X., & Dekkers, R. (2012). Entropy assessment of supply chain disruption. Journal of Manufacturing Technology Management, 23(8), 998–1014. https://doi.org/10.1108/17410381211276844
- Farrohknia, N., Castrén, M., Ehrenberg, A., Lind, L., Oredsson, S., Jonsson, H., Asplund, K., and Göransson, K. E. (2011). Emergency department triage scales and their components: A systematic review of scientific evidence. Scandinavian Journal of Trauma, Resuscitation and Emergency Medicine, 19(1), 42. https://doi.org/10.1186/1757-7241-19-42
- Fone, D., Hollinghurst, S., Temple, M., Round, A., Lester, N., Weightman, A., Roberts, K., Coyle, E., Bevan, G., and Palmer, S. (2003). Systematic review of the use and value of computer simulation modelling in population health and health care delivery. Journal Public Health Medicine, 25(4), 325–335. https://doi.org/10.1093/pubmed/fdg075
- Gunal, M. M., & Pidd, M. (2010). Discrete event simulation for performance modelling in health care: A review of the literature. Journal of Simulation, 4(1), 42–51. https://doi.org/10.1057/jos.2009.25
- He, Y., Cai, B., & Wang, M. (2016). Research on optimization of registration procedure in emergency department based on system simulation. Procedia Computer Science, 91, 37–46. https://doi.org/10.1016/j.procs.2016.07.039
- Huang, Y., Tang, Z., & Liang, J. (2018). Information security risk assessment based on information entropy and three-parameter interval. Computer Engineering, 44(12), 178–183.
- Jun, J. B., Jacobson, S. H., & Swisher, J. R. (1999). Applications of discrete event simulation in health care clinics: A survey. Journal Operational Research Society, 50(2), 109–123. https://doi.org/10.1057/palgrave.jors.2600669
- Klassen, K. J., & Rohleder, T. R. (1996). Scheduling outpatient appointments in a dynamic environment. Journal of Operations Management, 14(2), 83–101. https://doi.org/10.1016/0272-6963(95)00044-5
- Kotiadis, K., & Tako, A. A. (2018). Facilitate post-model coding in Discrete Event Simulation (DES): A case study in healthcare. European Journal of Operational Research, 266(3), 1120–1133. https://doi.org/10.1016/j.ejor.2017.10.047
- Liu, G. (2012). The influence of demand factors in the change of industrial structure: Based on the theory and application of information entropy method. Systems Engineering-Theory Methodology Application, 21 (1), 70–75. (In Chinese). doi: 10.3969/j.issn.1005-2542.2012.01.011
- Liu, Y., & Jiang, Z. (2003). Theory of entropy and dissipative structure in enterprise management. Journal of Xi’an Jiaotong University (Social Science), 1 (23), 88–91. (In Chinese). CNKI:SUN:XAJD.0.2003-01-015
- McMeekin, P., Flynn, D., Allen, M., Coughlan, D., Ford, G. A., Lumley, H., Balami, J. S., James, M. A., Stein, K., Burgess, D., & White, P. (2019). Estimating the Effectiveness and Cost-effectiveness of Establishing Additional Endovascular Thrombectomy Stroke Centres in England: A Discrete Event Simulation. BMC Health Services Research, 19(1), 821. https://doi.org/10.1186/s12913-019-4678-9
- Nakagawa, T., & Yasui, K. (2003). Note on reliability of a system complexity considering entropy. Journal of Quality in Maintenance Engineering, 9(1), 83–91. https://doi.org/10.1108/13552510310466990
- Robinson, S., Worthington, C., Burgess, N., & Radnor, Z. J. (2014). Facilitated modelling with discrete-event simulation: Reality or Myth. European Journal of Operational Research, 234(1), 188–197. https://doi.org/10.1016/j.ejor.2012.12.024
- Son, J., Lee, J., Larsen, K. R., & Woo, J. (2019). Understanding the uncertainty of disaster tweets and its effect on retweeting: The perspectives of uncertainty reduction theory and information entropy. Journal of the Association for Information Science and Technology, 1–17. https://doi.org/10.1002/asi.24329
- Song, H. L., Liu, Q. S., & Liu, L. J. (2003). Management entropy theory: A new scale for the evaluation of system complexity of organization management in enterprise. Journal of Management Sciences in China, 6 (3), 19–27. (In Chinese). CNKI:SUN:MTJN.0.2004-09-039
- Tako, A. A., & Kotiadis, K. (2015). PartiSim: A Framework for Participative Simulation Modelling. European Journal of Operational Research, 244(2), 555–564. https://doi.org/10.1016/j.ejor.2015.01.046
- Vanden, B. P. M., & Dietz, C. D. (2000). Minimizing expected waiting in a medical appointment system. IIE Transactions, 32(9), 841–848. https://doi.org/10.1080/07408170008967443
- Wu, R. M., & Hu., D. (2008). Information entropy method for evaluating system structure. Journal of Intelligence, 27(11), 3–6.(In Chinese). CNKI:SUN:QBZZ.0.2008-11-000
- Xie, Y. L., Zou, K., Jiang, Z. Y., & Xiao, K. (2013). Research on the evaluation of community information resource allocation based on rough set condition information entropy. Chinese Journal of Management Science, 21 (11), 52–56. (In Chinese).
- Yu, S., Liu, Y., & Liang, X. Information entropy evolution and dynamic analysis of regional transportation structure. (2011). Journal of Transportation Systems Engineering and Information Technology, 11(4), 91–96. (In Chinese). https://doi.org/10.1016/S1570-6672(10)60134-1
- Zhao, H., Chen, X. H., & Deyu, L. (2004). Evaluation of enterprise competitive advantage based on grey relevance theory and information entropy theory. Journal of Modernization of Management, 4, 13–16. (In Chinese). CNKI:SUN:GLXX.0.2004-04-002
- Zhao, Z., Peng, H., Zhang, X., Zheng, Y., Chen, F., Fang, L., & Jinyan, L. (2019). Identification of lung cancer gene markers through kernel maximum mean discrepancy and information entropy. BMC Medical Genomics, 12(8), 183. https://doi.org/10.1186/s12920-019-0630-4