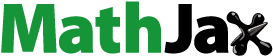
Abstract
This paper examines the empirical validity of Nicolosi’s optimal strategy for a hedge fund manager under a specific payment contract. The contract specifies that the manager’s payment consists of a fixed payment and a variable payment, which is a performance-based payment. The model assumes that the manager is an Expected Utility agent who maximises his/her expected utility by buying and selling the asset at appropriate moments. Nicolosi derives the optimal strategy for the manager by assuming a Black-Scholes setting where the manager can invest either in an asset or in a money account. The asset price follows geometric Brownian motion and the money account has a constant interest rate. I experimentally test Nicolosi’s optimal strategy to investigate whether the agents invest according to the optimal strategy. To meet the aim of this paper, I compare the empirical support of the optimal strategy with other possible strategies. The results show that Nicolosi’s optimal strategy receives strong empirical support for explaining the subjects’ behaviour, though not all of the subjects follow the optimal strategy. Having said this, it seems that the subjects somehow follow the intuitive prediction of Nicolosi’s optimal strategy in which the decision-maker responds to the difference between the managed portfolio and the benchmark to determine the portfolio allocation.
PUBLIC INTEREST STATEMENT
This paper tries to examine the empirical validity of Nicolosi (Citation2018) through a laboratory experiment. Nicolosi (Citation2018) provides the optimal strategy for the hedge fund manager where he/she has to manage the investor’s funds under a specific contract. The fund manager can invest either in an asset or in a money account where the asset price is a subject to random and money account has a constant return. The results show that the optimal strategy of Nicolosi (Citation2018) receives strong empirical support for explaining the subjects’ behaviour by comparing it with other possible strategies—though not all of the subjects follow the optimal strategy. They somehow follow the intuitive prediction of Nicolosi’s optimal strategy in determining the portfolio allocation. This sheds the lights that portfolio strategy, at some point, can be well-predicted by a theoretical strategy.
1. Introduction
The hedge fund industry has grown enormously in the last few decades. It may be best defined as the private investment vehicle deploying a wide range of investment strategies in order to achieve a high rate of return, though there are alternative definitions for it (Hildebrand, Citation2005). It has a wide variety of investments such as stock, bonds, real estate and other commodities. The hedge fund manager is then responsible to manage the investor’s funds under a specific contract. The contract initially specifies the investor’s target (usually referred to as the benchmark), the investment period and the payment scheme. The payment typically is based on the manager’s performance with respect to a pre-specified benchmark; though the benchmark may be arbitrarily set by the investor. The better is the manager’s performance with respect to the benchmark, the higher is the manager’s payment.
Clearly, the payment scheme determines the manager’s behaviour, given his/her risk attitude, in managing the investor’s funds (Palomino & Prat, Citation2003). The investor employs this payment scheme to meet his/her benchmark, and the manager maximises his/her expected utility by buying and selling the asset at appropriate moments given the payment scheme. So once the contract is agreed, the manager chooses his/her portfolio strategy, given the risk attitude, to ensure beating the benchmark at maturity, in order to maximise the manager’s utility.
Much literature has explored the optimal portfolio strategy for the hedge fund manager in order to maximise his/her expected utility under a specific contract. Notable amongst these recently are Browne (Citation1999), Carpenter (Citation2000), Gabih et al. (Citation2006), Hodder and Jackwerth (Citation2007), Panageas and Westerfield (Citation2009), and Guasoni and Obloj (Citation2016) which investigate the optimal portfolio choice for the manager in continuous-time with respective to a selected benchmark by the investor; this literature being motivated by the work of Merton (Citation1969, Citation1971)).Footnote1 One clear conclusion from this literature is that the benchmark level determines the manager’s behaviour, given his/her risk attitude (measured by the level of risk aversion). In particular, this literature investigates how the manager’s risk attitude affects his/her allocation decision: that is, how much to allocate in the risky asset. Generally, the literature shows that the manager is highly likely to hold more of the asset (that is, take on more risk) when the portfolio value is below the benchmark, in order to increase his/her chance of ending up with a higher payment. Contrariwise, the manager should reduce his/her portfolio volatility (by holding less of the asset) when the performance is relatively above the benchmark.
This paper examines the empirical validity, with a laboratory experiment, of Nicolosi (Citation2018) which investigates the dynamic optimal strategy for the hedge fund manager under a performance-based payment. Nicolosi derives the optimal portfolio strategy for the manager to maximise his/her expected utility subject to the given investment funds. The optimal strategy is dynamic portfolio choice decisions that maximises the manager’s expected utility at maturity. The intuition behind this solution is similar to the existing literature in which the optimal strategy manages the manager’s risk-taking behaviour, given his/her level of risk aversion, in order maximise his/her expected utility at maturity. One crucial implication of Nicolosi’s optimal strategy is that, during the trading period, the manager should not hold a high allocation in the asset when his/her portfolio is above the benchmark. Mutatis mutandis, the manager should allocate his/her portfolio to the asset when his/her portfolio value is lower than that of the benchmark. Following the optimal strategy, thus, helps the manager to end up earning both fixed and variable payments as his/her portfolio value is higher than that of the benchmark at maturity—hence receiving the maximum utility.
The aim of this paper is to investigate how close the actual behaviour is to the optimal strategy of Nicolosi given the estimated risk aversion. Actual behaviour is then compared with other strategies to check the empirical validity of Nicolosi’s optimal strategy. I estimate the individual risk aversion—elicited from the actual choice—which best explains behaviour and use it to compute the optimal strategy and the portfolio value at maturity. In the next section, I thoroughly describe Nicolosi’s optimal strategy. Section 3 describes the experimental design, Section 4 describes the econometric specification, Section 5 presents the results and analysis, and Section 6 discusses and concludes.
2. Nicolosi’s optimal strategy for the fund manager
Nicolosi explores the optimal strategy for the hedge fund manager who wants to maximise his/her expected utility subject to the investment funds. The optimal strategy is specified through two types of payment: a fixed payment and a variable payment, where the variable payment is based on the over-performance at maturity with respect to the benchmark. So, the manager surely earns the fixed payment and will earn the variable payment depending on what (s)he achieves. The benchmark is a linear combination of the investment invested in the risky and riskless assets, and the over-performance is achieved if the manager makes a higher portfolio value than that of the benchmark at maturity. Nicolosi imposes two important rules of the game: 1) the manager allocates the fund between an asset (risky) and a money account (riskless), and 2) the manager’s performance is assessed by the value of the portfolio at maturity. He also assumes that the asset price follows geometric Brownian motion while the money account provides a constant interest rate.
The hedge fund manager starts the game by receiving the investment funds (W0) from the investor and takes responsibility to invest in the financial market. There are two types of the financial market where the manager can invest, the risky asset market and the money market. The risky asset market trades an asset whose price (S) fluctuates over time t. The money market is riskless and gives a constant return (r). What the manager does then is to set portfolio allocation to be invested in the asset (θ) and in the money market (1—θ). The investor asks if the manager can achieve, at least, the benchmark (Y) from the investment funds over an investment period T.Footnote2 This benchmark is the basis of manager’s performance measure and it is used to determine his/her payment; this payment will be explained later. The investor arbitrarily sets his/her benchmark as the value at maturity of a portfolio with a constant proportion (β) invested in the asset and a constant proportion (1—β) in the money market. The investor then sees what would happen to his/her benchmark value at maturity (YT) following this scheme.
The manager agrees on a contract, determined by the investor, which sets the investment period and the payment scheme for the manager. The investor pays the manager depending on what the manager achieves at maturity (WT). The payment (Π) consists of two terms, a fixed and a variable payment. The fixed payment is a percentage (K) of the initial investment funds and the variable payment is a share (α) on the over-performance (WT—YT)+ relative to the benchmark. It is assumed that there is no penalty for the manager if (s)he under-performs relative to the benchmark.Footnote3 It follows that the manager will always earn non-negative payment irrespective of his/her performance. However, the better is the performance compared to the benchmark, the higher is the payment for the manager.
Nicolosi assumes a Black-Scholes settingFootnote4 with the asset price following standard geometric Brownian motion. We can write this as: dSt = St(μdt + σdZt); where St is the asset price at time t ∈ [0,T), μ and σ are trend and volatility of the asset price, respectively, and Z is a standard Brownian motion which follows N(0,1). The asset price follows the geometric Brownian motion, hence it is defined as:
where Vt = Ztdt0.5 is the increment of a Wiener processFootnote5 and S0 is the initial asset price. Both the manager and the investor are aware of this process and its parameters.
Given the contract, the investor will pay the manager with a linear combination of the fixed and the variable payment which can be written as: Π = K + α(WT—YT)+. The first term is the fixed payment (K) and the second term is the variable payment where α is a positive underlying managed portfolio minus benchmark at maturity (WT—YT)+. So the higher is the (WT—YT)+, the higher is the manager’s payment.
The manager is assumed to be an Expected Utility (EU) agent who maximises his/her expected utility from the payment. The model assumes that the utility function of the manager is that of constant relative risk aversion (CRRA) with a parameter risk aversion γ.Footnote6 In addition, it assumes that the manager is strictly risk-averse, so that γ > 0. Therefore, the manager’s problem is written as follows:
where ξ is the state price densityFootnote7 which is defined as ξT = exp[-(r + 0.5X2)T—XZT] and ξ0 = 1—where X = (μ—r)/σ and X > 0. Although EquationEquation (2)(2)
(2) is a static problem, it is maximised through optimising the dynamic problem throughout the investment period by setting the optimal allocation θ* subject to Wt.Footnote8 Crucial to this approach is to define the optimal portfolio at maturity (WT*). Carpenter (Citation2000) proposes the solution of this problem in which WT* depends on YT, since the manager would never want WT ∈ (0,YT], and the realisation of ξT. It is given by:
where I(x) = (u’)−1(x) is the inverse function of the marginal utility and I{.} is the indicator function over {.} and ξˆ is the threshold state price density. This is the final optimal portfolio strategy of Nicolosi which depends on the benchmark (Y) and the state price density (ξ). As a part of the solution, there exists a unique Lagrange multiplier λ* > 0 to ensure that is satisfied for any WT* > YT.
Proposition 1 of Nicolosi (Citation2018) proposes the optimal portfolio strategy throughout the investment period (Wt*) that leads to the optimal portfolio value at maturity (WT*). Given the manager’s risk aversion γ, the optimal portfolio strategy Wt* for any β ≤ βm—where βm = X/σ—is:
where Et[.] is the expectation of the optimality conditional to the information at time t which is ξt. Since ξ follows Markovian process, for which the future probability is determined by its most recent value, we can rewrite ξT as:
The corresponding optimal strategy to achieve Wt* as in EquationEquation (4)(4)
(4) given the manager’s risk aversion γ is:
where θM = βm/γ the Merton’s strategy (Merton, Citation1971) in the dynamic optimisation problem without compensation scheme and ɸ(.) is the cumulative distribution function (cdf) of the normal distribution. What C1, C2, C3, d1, d2 and d3 mean are defined in Appendix B. All parameters in EquationEquation (6)(6)
(6) are pre-determined except the risk aversion γ; both λ* and ξ are solved from the solution to the final optimal portfolio (Wt*) as in EquationEquation (3)
(3)
(3) . Therefore, this paper reports on an experiment to see how close the subjects’ choices are, of the θt, to those optimal choices as in the theory and elicit the risk aversion γ from the subjects’ choice.
3. Experimental design
The actual experiment design differs in two aspects from the theoretical design: a non-consequential and a consequential difference. The non-consequential difference is that the experimental design implements a discrete approximation to the continuous time problem, due to computer system limitation. Each discrete time step has a length dt = 0.1 second—hence the asset price changes every 0.1 second. The consequential difference is that the subjects were allowed to allocate their portfolio in the asset market (θ) only between 0% and 100%. By this, the subjects were not allowed to short-sell asset and borrowing and lending money in order to avoid a large negative payment for the subjects. However, the theory allows −∞ < θ < ∞.
There were 10 problems in the real experiment, all of the same type; the number of problems was chosen arbitrarily considering the experiment duration.Footnote9 The problems vary in the μ and σ of the Brownian motion parameters and, more importantly, vary in βm that has to satisfy the condition of β ≤ βm. By this, it differs the chance of knowing the final benchmark since the higher is the βm, the (relatively) easier to predict the final benchmark; as more allocation in the money market. However the problems were randomly ordered for all subjects.
The experiment was preceded by two practice problems. The subjects were given paper and on-screen instructions, and a simulation practice to generate the actual asset price with adjustable parameters (µ and σ) before going on to practice session. They were informed (in non-technical terms) that the asset price followed geometric Brownian motion, and were presented with as many simulations as they liked of such motion. Each simulation lasted for one minute; subjects could see how as many simulated asset price paths as they wanted. After they were clear of what being asked to do and of how the asset price is generated, they started the practice session; after that, they started the real experiment. At the beginning of every problem, subjects were told all parameters for that problem (S0, K, α, T, t, β, µ, σ, W0, r); the initial price S0, initial wealth W0, and interest rate r are always 25 ECU, 100 ECU and 0 ECU, respectively, in every problem. They were also given six examples of the asset price chart for given parameters in every problem. Given all this information, subjects were asked to set their θ0 before the trading period.
Subjects were shown all update information during trading (the managed portfolio value in the asset, in the money account and in total, the benchmark value, the asset price, trading time and portfolio allocation in both asset and money market). They adjusted their portfolio allocation in the stock market using a slider. In addition, short instructions and the parameters used were displayed on the trading screen. They could start trading anytime they wished by clicking the “START” button. Each problem lasted for one minute in the practice session and three minutes in the real experiment. Subjects were also shown their performance (the managed portfolio value, the benchmark value and the payoff) by the end of every problem.
Monetary incentives were provided in accordance with the theory. One problem from the real experiment was randomly drawn to determine the subjects’ payment. Subjects were asked to draw a disk themselves from a closed bag containing the numbered disks from 1 to 10—this identifies the problem number. The conversion rate is £1:3 ECU rounded up to the nearest 5 pence. The payment then will be added to a show-up fee of £3.
The experiment was conducted in the EXEC Lab, University of York. Invitation messages were sent through hroot (Hamburg registration and organization online tool) to all registered subjects in the system. 73 university members participated in this experiment: 46 males and 27 females. Composition of their educational degree was: 49 subjects were bachelor, 15 subjects were master, 7 subjects were PhD, 1 subject was diploma and 1 subject did not report his/her educational degree (See Figure below).
I targeted the subjects who were or had been enrolled in the specific study that teaches finance and/or Brownian motion (e.g., Economics, Finance, Physics, Mathematics and Statistics). Most of them (48 subjects) had participated in at least one economic experiment prior to this experiment. The average payment to the subjects was £8.1 and the average duration of the experiment (including reading the instructions and the payment session) was around one and quarter hours. Communication was prohibited during the experiment. The experimental software was written (mainly by Alfa Ryano) in Python 2.7.
4. Econometric specification
I use maximum likelihood to estimate the parameter of the model—risk aversion (γ), estimating subject by subject. Maximum likelihood requires a specification of the stochastic nature of the data to capture the noise or error in the subjects’ choice (θt). I assume this error is independent in every period during trading (t). Since the optimal choice (θt*) takes any values, I consider a normal distribution to specify the stochastic story to account for this case. I assume that the choice of θt was normally distributed with mean θt* (so that there is no bias) and standard deviation ς; I will report s = 1/ς which indicates the precision. My estimation takes into account the difference between the model and the actual as the latter is bounded between 0 and 1—as I have explained above. This strategy is thought as inferring risk aversion from the subjects’ decisions depending on the context of this experiment itself; in which it remains valid to follow the assumption of risk-averse agent as in Nicolosi (Citation2018).Footnote10
Before I turn to the specification of the log-likelihood function, I introduce further notations which will be used in the estimation to create an interval around θt since the log-likelihood function is continuous, while the actual choices were discrete, with steps of 0.1 second:
Given these notations, the log-likelihood function finds the probability that θt lies within θt+ and θt− for any given γ (risk aversion). Under this specification, the contribution to the likelihood of an observation θt is:
where ɸ is the cdf of a normal distribution with parameters θt* (mean) and 1/s1 (standard deviation) given an observation θt. For this specification, I estimate γ1 (risk aversion) and s1 (precision).
I also estimate using the average dataset. This is addressed to minimise the noise since the discrete time step (t) is quite fast (0.1 second). By this, I take an average of the dataset on every second, excluding the initial decision which remains as a single data—that is every 10 discrete time step (t). I denote subjects’ choice as θ¯i in this specification where i is the average discrete time step. Given this specification, the contribution to the likelihood of an observation θ¯i is:
where θ¯i+ = θ¯i + 0.005 and θ¯i− = θ¯i—0.005. Let me call these two specifications as Nicolosi 1, following the specification in EquationEquation (8)(8)
(8) , and Nicolosi 2, following the specification as in EquationEquation (9)
(9)
(9) . As with previous specification, I estimate γ2 (risk aversion) and s2 (precision) in this specification.
One may think of other stochastic assumptions to underlie estimation. For example, I could use a beta distribution to specify the stochastic of the subjects’ choices since they are bounded between 0 and 1. However, I start simple in this paper with a normal distribution specification.
To give a proper assessment to Nicolosi’s optimal strategy, I fit the data additionally assuming both random and risk-neutral choices. The former (random choice) assumes that the choice of θt is random following a uniform distribution; that every allocation decision is equally likely to occur. The latter (risk-neutral choice) assumes that the choice of θt follows risk-neutral behaviour. Theoretically, the risk neutrality returns either −Inf or Inf, depending on the asset price change. Here I assume that θt is 1 if the asset price goes up, otherwise 0 if the asset price goes down. Note crucially, neither of these alternatives involves parameter risk aversion γ.
Again I consider a normal distribution to specify the stochastic story for both random and risk-neutral choices. I also estimate using both all observations and the average dataset. The contribution to the log-likelihood for these specifications adopts EquationEquations (8)(8)
(8) and (Equation9
(9)
(9) ) as appropriate. For these specifications, let me call random choice specification as Random 1 (for the all-observation estimation) and Random 2 (for the average-dataset estimation), and Risk Neutral 1 (for the all-observation estimation) and Risk Neutral 2 (for the average-dataset estimation) for risk-neutral choice.Footnote11
5. Results and analyses
One main purpose of this paper is how well the optimal strategy of Nicolosi (Citation2018) explains the subjects’ choice compared to other strategies (random and risk-neutral choices). The analyses for this purpose use all observations and average dataset from the real experiment. The former sees 1,801 decisions while the latter sees 181 decisions in each problem for each subject. However, the risk-neutral choice will see 1,800 and 180 decisions respectively, excluding the initial decision, since it is drawn following the realisation of the price change.
Additionally, I develop a simple strategy from a regression model using variables in Nicolosi (Citation2018). This is a simplification of the theory as in EquationEquation (6)(6)
(6) ; hereinafter referred to as Simple 1 (for the all-observation estimation) and Simple 2 (for the average-dataset estimation). As previously, I compare Nicolosi’s optimal strategy and this simple strategy given the estimated risk aversion γ from both the all-observation and average-dataset estimations.
Before going on the main analyses, I estimate the individual risk aversion and precision in Nicolosi 1 and Nicolosi 2, which can be found in Figures and .
The results between both estimates show that estimate in Nicolosi 2 returns less risk-averse and higher precision on average than that of estimates in Nicolosi 1.Footnote12 This can be a further point of interest, but I take this merely as a consequence of using different approach since the main purpose of this study is to test the empirical validity of Nicolosi’s optimal strategy.
The estimated risk aversion then is used to compute the individual final portfolio values (WT* as in EquationEquation (3)(3)
(3) ) across all problems. It should be the case that following the optimal strategy (Wt*) in each t < T will return a better final portfolio value than that of the final benchmark value given the estimated risk aversion. There are 730 portfolios at maturity in total from 10 problems across 73 subjects. Figure shows comparisons of the optimal portfolio (WT*) and the benchmark values (YT) at maturity across all problems in both Nicolosi 1 and 2.
Figure 4. The optimal portfolio (WT*) vs the benchmark (YT) at maturity given the estimated risk aversion in Nicolosi 1 and Nicolosi 2.
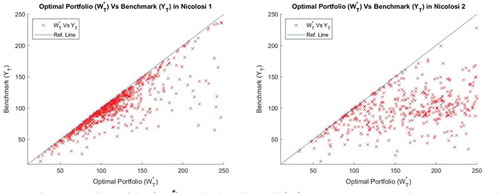
Results from Nicolosi 1 show that all of the final optimal portfolio values (730 portfolios) are better than that of the final benchmark values; meanwhile results from Nicolosi 2 show that 711 final optimal portfolio values (97.4% of the total) are better than that of the final benchmark values given the individual estimated risk aversion. This shows that following the optimal strategy of Nicolosi (in every t < T) is highly likely to end up with both payments (fixed and variable payments). In addition, the optimal portfolios return the higher utility than that of the actual portfolios—as shown in Figure .Footnote13 This is surely not surprising.
Figure 5. Optimal utility (u(Π*)) vs actual utility (u(Π*)) given the estimated risk aversion in Nicolosi 1 and Nicolosi 2.
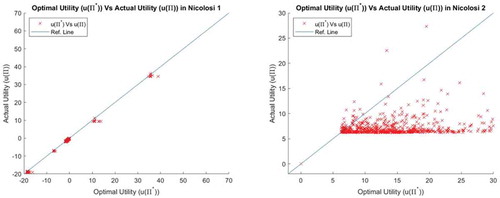
5.1. Nicolosi’s optimal strategy vs random and risk-neutral strategies
Now we move on to the first comparison between Nicolosi’s optimal strategy and the random and risk-neutral strategies. The concern is to find the best fitting strategy as the explanation of the individual behaviour in selecting the portfolio allocation between the asset and the money account with Nicolosi’s optimal strategy as the subject to test. I measure the goodness-of-fit by maximising the log-likelihood function, as specified in the EquationEquations (8)(8)
(8) and (Equation9
(9)
(9) ), but we need to correct the maximised log-likelihood for the number of parameters in each specification—Nicolosi’s optimal strategy has two estimated parameters while each of the random and risk-neutral choices has one estimated parameter. In particular, I simulate 100 times of the decisions in each problem to generate the dataset for both random choices (Random 1 and 2), then take its average log-likelihood.Footnote14
I use the Akaike Information Criterion (AIC) as the measure of the goodness-of-fit to find the best explanation for each subject. The details of the judgment can be seen in Appendix D and E. With the all-observation estimation—between Nicolosi 1, Random 1 and Risk Neutral 1—of all 73 subjects, 49 subjects are better explained with Risk Neutral 1 while the other 24 subjects are better explained with Nicolosi 1; Random 1 is always the worst. Nevertheless, with the average dataset estimation, 40 subjects are better explained with Nicolosi 2 while the other 33 subjects are better explained with Risk Neutral 2; Random 2 remains the worst. This finding obviously shows that subjects did not randomise their choice in allocating their portfolio—that they followed some specific strategies for this. In particular, averaging the dataset improves the goodness-of-fit of Nicolosi’s optimal strategy. This may be the evidence that subjects somehow follow the optimal strategy as in Nicolosi’s optimal strategy but having difficulties to be as precise as the theory.
So far it is obvious that the subjects did not randomise their choices, and that following the optimal strategy is highly likely to end up with a better portfolio than that of the benchmark. As it also has shown, Nicolosi’s optimal strategy receives the most empirical support on the average level. Nevertheless, the subjects might find it difficult to follow the optimal strategy of Nicolosi, which involves sophisticated dynamic programming, given his/her risk aversion—calculating and implementing as precise as the optimal strategy. Results from the estimated precision show that the subjects’ choices are noisy compared to the optimal strategy in both Nicolosi 1 and 2; with average estimated precisions are 1.3556 (or standard deviation 0.7377) and 1.4763 (or standard deviation 0.6774) respectively. However, they must respond to some variables shown on the screen to determine their choice.
5.2. Nicolosi’s optimal strategy vs the simple strategy
Building on the previous results, I try to explore the determinants of the subjects’ choice in a simple way using a regression model. Following Nicolosi, the portfolio allocation in the asset (θt) should not be constant, as in the Merton’s strategy (θM = βm/γ), when the managed portfolio value is lower than that of the benchmark during trading in order to increase the chance to beat the benchmark at maturity. In particular, θt tends to be low, during trading, when the portfolio value (Wt) is higher than that of the benchmark (Yt), vice versa. So I involve the difference between the managed portfolio and the benchmark (Wt − Yt) in the regression model; I denote this as ∆t. In addition, I also involve the asset price (St) and the benchmark value (Yt) since they were shown to the subjects in the experimental interface—I denote θ¯, S¯, Y¯ and ∆¯ for variables used in Simple 2. The regression results from Simple 1 and Simple 2 are as followsFootnote15:
I use percentage values for θ, t is time step and i is average time step. Overall results from both regression model above show that all independent variables are significant in determining the subjects’ choice—the signs of the independent variables are identical. Both the asset price and the difference between the managed portfolio and the benchmark have a negative effect on the subjects’ choice, meanwhile, the value of the benchmark has a positive effect on the subjects’ choice. These results are sensible and intuitive. Overall, subjects tended to buy the asset when its price was low and to sell the asset when its price was high; to some extent, it is commonly known as “buy low, sell high” strategy. This strategy is possibly the most famous adage in making profits from an asset market. Moreover, subjects tended to hold the asset as they saw the benchmark value was high. Lastly, subjects were consistent with the theoretical prediction in which they were unlikely to hold the asset when their portfolio value was relatively far above the benchmark.
Building on the regression results, I then run the regression model individually using the same structure as in EquationEquations (10)(10)
(10) and (Equation11
(11)
(11) ). This is to give a comparison of which model to have a better explanation for each subject between Nicolosi’s optimal strategy and the simple strategy using their measure of the goodness-of-fit; I compare between Nicolosi 1 and Simple 1, and between Nicolosi 2 and Simple 2. Since the models have different specifications, hence different degree of freedom, I calculate the AIC to correct for differing degrees of freedom.Footnote16 I compare them and have a conclusion accordingly for each subject.
Results from the two estimation procedures (using all observations and the average dataset) produce a slightly different AIC conclusion. With the all-observation estimation, 37 subjects are better explained with Nicolosi’s optimal strategy; 36 subjects are better explained with the simple strategy. Meanwhile, with the average-dataset estimation, 36 subjects are better explained with Nicolosi’s optimal strategy; 37 subjects are better with the simple strategy. The details of the judgment can be seen in Appendix F.
6. Discussion and conclusion
This paper examines Nicolosi (Citation2018) by investigating the subjects’ behaviour in order to follow the optimal strategy of Nicolosi in a controlled lab-experiment. Subjects act as if they are the hedge fund manager who takes a responsibility to manage the investor’s funds. The manager agrees on a contract, determined by the investor, who pays the manager with a two-term payment: the fixed payment and the variable payment, where the variable payment is a share-based on the over-performance with respect to the specific benchmark.
I follow Nicolosi’s design in which there are two markets for the manager to invest: the asset market and the money market. The asset price follows geometric Brownian motion and subjects were aware of all parameters used in the experiment. However, there are non-consequential and consequential differences in the experimental setup from the theoretical design. The former relates to the computer limitation to implement the continuous time in generating the asset price, hence I use the discrete approximation to the continuous time with an increment of 0.1 second. The latter restricts the maximum portfolio allocation between the asset and the money account, and that the subjects are not allowed to either borrow and lend money. This is addressed to prevent the subjects from a negative payoff from short-selling since it may have an unlimited loss.
To give a proper assessment of the empirical validity of Nicolosi (Citation2018), I compare its optimal choice, given the estimated subjects’ risk aversion, with two alternative strategies. First, I compare the optimal strategy of Nicolosi with random and risk-neutral choices. The random choice generates θ (portfolio allocation in the asset market) randomly following a normal distribution while the risk-neutral choice generates θ as if the subject was a risk-neutral agent; put everything on the asset if the asset price goes up, otherwise nothing if the asset price goes down. Second, I compare Nicolosi’s optimal strategy with the simple strategy, developed using a regression model. One obvious conclusion from the first assessment is that the subjects did not randomise their choice. They followed some specific strategies to maximise their utility from the experiment. Of all subjects, 24 subjects are better explained with Nicolosi’s optimal strategy while the other 49 subjects are better explained with risk-neutral choice using all the observations. We get a different conclusion if we use the average dataset. Of all subjects, 40 subjects are better explained with Nicolosi’s optimal strategy; the other 33 subjects are better explained with the risk-neutral choice.
Building on the previous results, I then develop a regression model to provide the simple strategy in which the subjects may plausibly have followed. With this simple strategy, one tends to hold the asset when its price is low and to sell the asset when its price is high. In addition, one manages its portfolio value depending on the benchmark value and the difference between the managed portfolio and the benchmark. I compare Nicolosi’s optimal strategy with this simple strategy individually—as with the previous analysis. The comparison sees that 37 subjects are better explained with Nicolosi’s optimal strategy, 36 others are better explained with the simple strategy, using all observations. If we use the average dataset, we get slightly different results: of all subjects, 36 subjects are better explained with Nicolosi’s optimal strategy, while 37 others are better explained with the simple strategy.
Although the optimal strategy of Nicolosi ensures a high possibility to end up with both fixed and variable payments, hence the maximum utility, the subjects found it difficult to follow. As it has shown, the subjects’ choices are noisy compared with the optimal strategy. One may argue that the subjects could have more precise computation if they were well accommodated in the experiment since Nicolosi’s optimal strategy involves sophisticated dynamic programming. For example, we could ask the subject to specify their own strategy to be implemented during trading at the beginning of each problem, and they are free to adjust their strategy at any time. Will it improve the empirical validity of the theory? I may not think so because it depends on how subjects understand the random process in the asset price, hence determining the benchmark value.
Alternatively, we could go further with other models within similar substantial framework of Nicolosi’s optimal strategy. Among these are Nicolosi et al. (Citation2018) and Herzel and Nicolosi (Citation2019). Both provide the optimal solution for the fund manager, similar to Nicolosi (Citation2018), who invests in one riskless asset and several risky assets. However the former assumes that there is no fixed payment, instead the manager is compensated with implicit incentives as shown in Chevalier and Ellison (Citation1997). By this the asset under management is multiplied if the manager performs well due to the inflow in the investor’s funds, otherwise a part of the asset under management is withdrawn. Other possibility is to model the subject’ choice assuming one preference function within either risk or ambiguity frameworks as shown in He and Zhou (Citation2011) and Ahn et al. (Citation2014), though they do not take into account the payment scheme for the fund manager; but they are not restricted only for the risk-averse agent case. Nevertheless, the optimal choice of Nicolosi (Citation2018) receives strong empirical support in explaining the subjects’ behaviour. In addition, subjects follow the intuitive prediction of Nicolosi’s optimal strategy where the difference between the managed portfolio and the benchmark determines the subjects’ choice.
Acknowledgements
I am thankful to John Hey, Marco Nicolosi, and participants at Gama ICEB 2019 and the 4th International ICNDBM Conference on New Directions in Business, Management, Finance and Economics for helpful comments and feedback. This paper was funded by Indonesia Endowment Fund for Education (Ref: S-4294/LPDP.3/2015). All errors and omissions are solely mine.
Additional information
Funding
Notes on contributors
Yudistira Permana
I am a staff at Vocational College, Universitas Gadjah Mada. I hold PhD in Economics from the University of York. My research interests are in Behavioural Economics and Experimental Economics and I have been actively conducting a laboratory experiment, particularly, in individual decision making under risk and under uncertainty. This paper tries to provide the empirical findings of the portfolio strategies where the theory can well-predict human complex thinking and behaviour in the particular setting. In the wider context, it should bring this study into a further exploration on why and how some fund managers may fail to manage the investor’s funds.
Notes
1. This benchmark can be either fixed or variable. The fixed benchmark usually is the expected return from the investment funds whereas the variable benchmark usually is the portfolio value at maturity following the investor’s portfolio allocation choice.
2. One may also refer this to as the “investment planning horizon”.
3. Despite this assumption, there may be various implementation to be taken for the case of under-performance considering that the investor pays a relatively high amount of payment for the manager. For example, a percentage deduction to the fixed payment depending on the magnitude of the under-performance.
4. Black-Scholes setting has following assumptions: a) there are two types of market, the asset market (risky) and the money market (risk-free), b) asset pays no dividend and there is no transaction cost in the market, c) asset price reflects all information in the asset market, d) asset price is exogenous to all agents, e) it is possible to borrow and lend cash at riskless rate as well as doing short-selling, f) the asset price change is random with known parameters, and g) it is possible to buy and to sell asset at any time. This assumption is important in the model in order to draw stochasticity of the asset price.
5. Wiener process (Vt) has the following properties: a) it is continuous, b) its change process is independent of the previous values, c) its increment process follows N(0, dt), d) V0 = 0.
6. See Appendix A for the specification of CRRA utility function.
7. State price density contains important information on the behaviour and expectations of the market (Hardle & Hlavka, Citation2009). It follows a log-normal distribution in the Black-Scholes setting.
8. EquationEquation (2)(2)
(2) is the implication of the martingale approach used in the model which decomposes a dynamic optimization problem
s.t. Wt into a static optimisation problem as in EquationEquation (2)
(2)
(2) . This determines the optimal condition at maturity. This approach was notably developed by Pliska (Citation1986), Karatzas et al. (Citation1987), and Cox and Huang (Citation1989) among others.
9. See Appendix C for the parameters used in the experiment.
10. One alternative is to have a separate experiment to elicit subjects’ risk aversion and calibrate only those risk-averse agents with Nicolosi’s optimal strategy. However Nicolosi (Citation2018) does not say anything about this in which one needs to check if the agent has to be risk averse to enter the game and that inferring risk aversion from the subjects’ decisions remains valid (see Zhou and Hey (Citation2018) for this issue).
11. I use pattersearch routine in Matlab to maximise the log-likelihood function in all specifications.
12. The average estimated risk aversion in Nicolosi 1 is 2.3353 compared to 0.5778 from the result in Nicolosi 2. Meanwhile, the average estimated precision in Nicolosi 1 is 1.3556 compared to 1.4763 from the result in Nicolosi 2; with θt is bounded between 0 and 1 whereas θt* is unbounded.
13. This sees 535 optimal portfolios (73.29% of the total) returns the better utility than that from the actual portfolios from results in Nicolosi 1; and 665 optimal portfolios (91.1% of the total) returns the better utility than that of the actual portfolios from results in Nicolosi 2.
14. The procedures for this are: i) draw the decisions in each problem using uniform distribution (to create values between 0 and 1) for 100 times, ii) fit the simulated decisions using a normal distribution (as in Equations (8) and (9)) to compute the likelihood, iii) take the average log-likelihood in each problem.
15. I use a simple linear procedure in both regression models. Standard errors are in parentheses and *, **, *** denote the significance at 1%, 5%, and 10% respectively. All coefficients are jointly not equal to zero in both regression model. Adjusted R2 in both models are 0.0094 and 0.0097 respectively, and the number of observations is 1,314,730 and 132,130 respectively.
16. The AIC is given by 2 k—2LL, where k is the number of estimated parameters and LL is the log-likelihood.
References
- Ahn, D. S., Choi, S., Gale, D., & Kariv, S. (2014). Estimating ambiguity aversion in a portfolio choice experiment. Quantitative Economics, 125(1), 40–20. https://doi.org/10.3982/QE243
- Browne, S. (1999). Beating a moving target: Optimal portfolio strategies for outperforming a stochastic benchmark. Finance and Stochastics, 3(3), 275–284. https://doi.org/10.1007/s007800050063
- Carpenter, J. N. (2000). Does option compensation increase managerial risk appetite? The Journal of Finance, 55(5), 2311–2331. https://doi.org/10.1111/0022-1082.00288
- Chevalier, J., & Ellison, G. (1997). Risk taking by mutual funds as a response to incentives. The Journal of Political Economy, 105(6), 1167–1200. https://doi.org/10.1086/516389
- Cox, J. C., & Huang, C.-F. (1989). Optimal consumption and portfolio policies when asset prices follow a diffusion process. Journal of Economic Theory, 49(1), 33–83. https://doi.org/10.1016/0022-0531(89)90067-7
- Gabih, A., Grecksch, W., & Richter, M. (2006). Optimal portfolio strategies benchmarking the stock market. Mathematical Methods of Operational Research, 64(2), 211–225. https://doi.org/10.1007/s00186-006-0091-3
- Guasoni, P., & Obloj, J. (2016). The incentives of hedge fund fees and high-water marks. Mathematical Finance, 26(2), 269–295. https://doi.org/10.1111/mafi.12057
- Härdle, W., & Hlávka, Z. (2009). Dynamics of state price densities. Journal of Econometrics, 150(1), 1-15. doi:10.1016/j.jeconom.2009.01.005
- He, X. D., & Zhou, Y. X. (2011). Portfolio choice under cumulative prospect theory: An analytical treatment. Management Science, 57(2), 315–331. https://doi.org/10.1287/mnsc.1100.1269
- Herzel, S., & Nicolosi, M. (2019). Optimal strategies with option compensation under mean reverting returns or volatilities. Computational Management Science, 16(1–2), 47–69. https://doi.org/10.1007/s10287-017-0296-3
- Hildebrand, P. M. (2005). Recent developments in the hedge fund industry. Swiss National Bank Quarterly Bulletin, 23-1(2005), 42–57. https://www.snb.ch/en/mmr/speeches/id/ref_20050204_pmh
- Hodder, J. E., & Jackwerth, J. C. (2007). Incentive contracts and hedge fund management. The Journal of Finance and Quantitative Analysis, 42(4), 811–826. https://doi.org/10.1017/S0022109000003409
- Karatzas, I., Lehoczky, J. P., & Shreve, S. E. (1987). Optimal portfolio and consumption decisions for a small investor on a finite horizon. SIAM Journal on Control and Optimization, 25(6), 1557–1586. https://doi.org/10.1137/0325086
- Merton, R. C. (1969). Lifetime portfolio selection under uncertainty: The continuous-time case. The Review of Economics and Statistics, 51(3), 247–257. https://doi.org/10.2307/1926560
- Merton, R. C. (1971). Optimum consumption and portfolio rules in a continuous-time model. Journal of Economic Theory, 3(4), 373–413. https://doi.org/10.1016/0022-0531(71)90038-X
- Nicolosi, M. (2018). Optimal strategy for a fund manager with option compensation. Decisions in Economics and Finance, 41(1), 1–17. https://doi.org/10.1007/s10203-017-0204-x
- Nicolosi, M., Angelini, F., & Herzel, S. (2018). Portfolio management with benchmark related incentives under mean reverting processes. Annals of Operations Research, 266(1–2), 373–394. https://doi.org/10.1007/s10479-017-2535-y
- Palomino, F., & Prat, A. (2003). Risk taking and optimal contracts for money managers. The RAND Journal of Economics, 34(1), 113–137. https://doi.org/10.2307/3087446
- Panageas, S., & Westerfield, M. M. (2009). High-water marks: High risk appetites? Convex compensation, long horizons, and portfolio choice. The Journal of Finance, 64(1), 1–36. https://doi.org/10.1111/j.1540-6261.2008.01427.x
- Pliska, S. R. (1986). A stochastic calculus model of continuous trading: Optimal portfolios. Mathematics of Operations Research, 11(2), 371–382. https://doi.org/10.1287/moor.11.2.371
- Zhou, W., & Hey, J. (2018). Context matters. Experimental Economics, 21(4), 723–756. https://doi.org/10.1007/s10683-017-9546-z
Appendices
Appendix A.
Specification of CRRA utility function
Nicolosi (Citation2018) assumes that the manager is utility maximiser specified with CRRA utility function. It can be written as:
The manager is assumed to be strictly risk-averse and the function is undefined when γ = 1. However I apply CRRA utility function so it is able to accommodate when γ = 1 as follows:
Appendix B.
Definitions to EquationEquation (6)
(6)
(6)
Solution in EquationEquation (6)(6)
(6) contains some components (C1, C2, C3, d1, d2 and d3) where they are defined as follows: