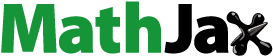
Abstract
The increased access and availability of information technology solutions have brought structural shifts in traditional business models, processes, and activities worldwide. As a result, the innovative ways of doing business and practices have disrupted the traditional businesses and technologies. This study investigates the impact of investment in data analytics on the financial performance of banks in Pakistan. A sample of 32 banks including commercial and microfinance banks for 2010 to 2019 was selected. Random effect panel estimation and instrumental variable two-stage least square were employed to quantify the impact of data analytics on firm performance. The results indicate that investment in data analytics increases the productivity of banks by 10%. However, the impact of investment in DA on profitability measures including return on assets, return on equity, and net interest income was negative, reflecting the “profitability paradox.” In the current era of digitalization, the banks need to invest in innovative technologies which have analytical capabilities to remain competitive and sustainable.
PUBLIC INTEREST STATEMENT
This study is motivated by the fact that digitalization has disrupted traditional business models particularly in the wake of pandemic Covid-19. Although the financial sector works as a growth engine for economic development, approximately 50% of Pakistan’s population is unbanked. The increased focus on digitalization in the country in general and the financial sector in particular is promising. This research seeks to establish a linkage between modern technologies that emerged through digitalization and firm performance in an emerging economy context. The research will help regulators and decision-makers understand the potential role of modern technologies driven by the banking industry. However, this study suggests that banks’ survival against disruptive business models is possible through investing in modern technologies with analytical and decision-making capabilities.
1. Introduction
The digital transformation is a current megatrend that has led to data deluge. The volume of data doubles every three years with an average of 2.5 quintillion bytes of data being generated every day (Analytics, Citation2016). Despite being aware of the potential value of data, many companies still waste more than half of the data they hold. Rose (Citation2016) argues that with further advancement in information technology (IT) it is now cheaper to keep data than delete it. Therefore, efficient utilization of data and analytics has become essential to the competitiveness of companies in today’s data-driven world. The digital transformation and the resultant data creation are forcing companies to invest in IT to create economic and business value for the users (Manyika et al., Citation2011; Mayer-Schönberger & Cukier, Citation2013). This has increased the demand for data analytics (DA) tools defined as “extensive use of data, statistical and quantitative analysis, explanatory and predictive models, and fact-based management to derive decisions and actions” (Davenport et al., Citation2007, p. 7).
Moreover, the increased access and availability of IT solutions have brought structural shifts in traditional business models, processes, and activities worldwide (SBP, Citation2020). The subsequent innovative ways of doing businesses and practices have disrupted the traditional business models. The digital ecosystem has been impressive in Pakistan too (SBP, Citation2015), particularly in the financial sector which is the major recipient of the data and information. There is a pressing need for digitalization and the availability of digital financial services during the Pandemic Covid-19 as the pandemic-induced lockdowns and disruptions demand the banks to be more digitally present than ever. This stressed the need to identify the business value of IT investment in the financial sector to promote digitalization and tackle issues related to the supply- and demand-side constraints (SBP, Citation2020).
Pakistan’s financial sector is the top recipient of data and information and it has been using IT-related banking solutions for more than two decades (SBP, Citation2015). However, big data analytics investment is yet to come in Pakistan’s banking sector due to the enormous cost of investing in big data (Gul & Ahsan, Citation2019). Laney (Citation2001) revolutionized the definition of big data and introduced three dimensions of big data; volume, velocity, and variety. While big data analytics have the capability to exploit unstructured data, most banks in Pakistan have invested in software with no analytical, forecasting or risk management capability. However, others are investing in data analytics (DA) which facilitate decision-making, visualization, predictions, and risk management without an ability to exploit unstructured data. We argue that investment in a technology that is not unique and available to everyone will not enhance the firm performance (Carr, Citation2003). Therefore, this paper attempts to identify how investment in data analytics (such as Oracle, Tableau, IBM, etc.) would affect banks’ financial performance in Pakistan.
Various academic and non-academic sources (e.g., Heudecker & Kart, Citation2014; Müller et al., Citation2018; Toon & Collins, Citation2015) confirm the business value of IT investment and how it affects organizational performance. Yet, the empirical evidence on the nexus between data analytics and firm performance in the financial sector is scarce. This nexus needs exploration in emerging economies like Pakistan so that policymakers and regulators may understand the significance of DA and develop policies to facilitate the smooth adoption of DA. Therefore, the main research question of this study is as follows: What is the impact of data analytics on the financial performance of banks in Pakistan?
We address the following issues in our study
Identify the investment in data analytics as per the data-enhanced business model definition.
Identify the measurements for productivity and profitability of the banking sector in Pakistan.
Investigate the impact of investment in data analytics on banks’ performance while controlling for IT expense.
Hilbig et al. (Citation2018) categorized digitalization and business models into three categories: low data business models, data-enhanced business models, and pure data-driven decision business models. On one extreme, low data business models hardly produce any digital data to exploit and on the other extreme, pure data-driven business models always rely on digital data (both structured and unstructured) in their key processes to generate value. The middle way is data-enhanced business models (DEBM) where “companies with DEBM offer physical products and/or services while enhancing given aspects of their business models using digital technologies and the exploitation of digital data to generate competitive advantages” (Hilbig et al., Citation2018, p. 12). Thus, taking together physical and digital products and services to generate value is the main key of data analytics investment.
This study makes three key contributions to the existing literature on digitalization and its implications for firm performance. First, it examines the impact of data analytics on productivity and profitability through econometric methods, whereas most of the studies have relied on surveys or interviews. Second, the existing research highlighted that digital data itself might not create value for companies; companies must have internal practices and methods suitable to put resources into value creating strategies in an uncertain environment. Third, it provides insight into an emerging economy, Pakistan, and shows how digitalization affects firm performance in the financial sector. The rest of the paper is structured as follows: the next section derives hypotheses based on a brief literature review. The materials and methods are discussed in section 3. The results are discussed in section 5 following the analysis of results in section 4. The final section concludes the paper.
2. Literature review and hypothesis development
When companies at small scale and countries at large scale adopt data perspective, they expect to create economic and social value (Fredriksson, Citation2015), which is measured in terms of cost minimization, efficient production processes, and maximized revenues, etc. (Manyika et al., Citation2011). Additionally, digital data facilitate continuous product and process innovation, organizational transparency, scientific customer and pricing segmentation, and informed decision-making (Fredriksson, Citation2015; Wamba et al., Citation2015). Only a few empirical studies have tried to quantify the impact of data analytics on business performance and value creation. Also, the financial value created through DA investment and measured through econometric models is scarce with the exception (Brynjolfsson et al., Citation2011; Müller et al., Citation2018; Tambe, Citation2014). On the other hand, most of the studies are either qualitative and provide only the challenges and opportunities of data analytics (e.g., Sodenkamp et al., Citation2015; Vom Brocke et al., Citation2014) and/or survey-based where the perceptual measures of value creation are used (such as Someh & Shanks, Citation2015). The Organization for Economic Co-operation and Development (OECD) report highlights that despite hype on a positive link between data analytics and productivity, the empirical evidence based on quantitative measures is scarce (OECD, Citation2014).
The literature on IT business value is huge with somewhat conclusive evidence (Koetter & Noth, Citation2013; Martín-Oliver & Salas-Fumás, Citation2008). However, the business value of big data analytics and its predecessors such as data analytics, decision support systems is lacking (Müller et al., Citation2018). Therefore, Abbasi et al. (Citation2016) argue that there is a dire need to conduct a critical assessment of the actual impact of DA and identify whether financial performance can be improved. Earlier, the empirical evidence on the business value of information technology was mixed as a payoff of IT investment was minimal, termed as “IT Productivity Paradox” (Brynjolfsson, Citation1993). Besides, measuring the business value of IT was another problem for the financial sector due to its different business structure. Therefore, most of the studies excluded the financial sector from their sample and focused on manufacturing sector only (see for example, Brynjolfsson & McElheran, Citation2019).
Of late, IT’s significant and positive business value in manufacturing sector is well documented in the literature (Brynjolfsson et al., Citation2011; Brynjolfsson & McElheran, Citation2019; Kohli & Devaraj, Citation2004; Müller et al., Citation2018) whereas the research on financial sector is still scarce. Also, the contribution of data analytics and digitalization to productivity remained limited to manufacturing sector due to the ambiguous definition of “output”. This problem is particularly persistent in the banking sector which is the focus of our study. To fill this gap, this study attempts to investigate the relationship between investment in DA and financial performance of banks in Pakistan. Based on the above discussion, the study develops the following hypotheses;
H1: Data analytics has a positive impact on the productivity of banks in Pakistan.
H2: Data analytics has a positive impact on the profitability of banks in Pakistan.
3. Materials and methods
3.1. Sources of data
A sample of commercial and microfinance banks operating in Pakistan is used for this study. In total, there are 33 commercial banks and 11 investment banks registered with the State Bank of Pakistan. Due to the small operation size, foreign banks were excluded from the sample. Four specialized banks were also excluded from the sample due to their operations and target market’s different nature. This left us with 36 banks. However, due to the non-availability of data of few banks, the final sample is composed of 32 banks; of which 10 are microfinance and 22 are commercial banks. The data were collected from Pakistan Stock Exchange, State Banks of Pakistan’s library, and respective banks’ annual reports. The sample period of our study is 10 years from 2010 to 2019.
3.2. Construction of variables
The variables used in this study are constructed as follows:
3.2.1. Data Analytics (DA)
The empirical literature on how to measure DA is scant. Tambe (Citation2014) measured Hadoop investment as number of technical workers who had Hadoop skills. Müller et al. (Citation2018) measured big data analytics (BDA) assets as binary assets; record 1 when the firms go live with BDA assets and the following years, and 0 before BDA investment. Following Müller et al. (Citation2018), we also measure DA as a dummy variable; 1 in the year and the following years when a bank goes live with data analytics and zero otherwise.
3.2.2. Productivity
For this paper, financial performance is measured through the productivity of the banks. The most widely used measure of performance is multifactor productivity in IT literature (Hitt et al., Citation2002). A firm’s output (sales) is related to input variables (labor, capital, and IT). Although various production functions can be used to estimate IT investment productivity, the most preferred is Cobb–Douglas (Brynjolfsson et al., Citation2011; Müller et al., Citation2018). Cobb and Douglas (Citation1928) modeled the growth of the American economy from 1899 to 1922. They offered a simplified view of the economy where production output is determined by the capital invested and the amount of labor involved. Their model proved to be remarkably accurate, though, additional variables were also added to the original model, the functional form of the Cobb–Douglas model is as follows:
P(L, K) = bLαKβ where b is the total factor productivity, α and β are the output elasticities of labor and capital, respectively.
3.2.3. Profitability
An alternative method of measuring firm performance is the profitability measured through ratio analysis. This approach has been widely used to investigate the impact of investment in IT assets on profitability of firms (Aral et al. Citation2006; Hitt et al., Citation2002). Return on assets and return on equity, and net interest income are used for profitability analysis consistent with (Kriebel & Debener, Citation2020; Mashal, Citation2006; Onay & Ozsoz, Citation2013).
3.3. Methodology
Panel data estimation is the most appropriate method for current study as it combines cross-sections and time series data acting as a rich source of information (Baltagi, Citation2008). Panel estimation considers individual groups, firms or countries heterogeneous and so it controls for individual heterogeneity. As the banks possess different ownership structures, types of operations, and risk exposure, it may lead to biased results if this heterogeneity is not controlled. Panel data reduce multicollinearity and offer more variability, degree of freedom, and efficiency among explanatory variables. However, panel data may suffer from endogeneity and reverse causality; therefore, we also employ instrumental variable two-stage least-squares regression (IV/2SLS). The statistical analysis is conducted in STATA 15.
3.4. Estimable models
We apply Cobb–Douglas production consistent with IT literature to quantify the impact of data analytics on firm performance. The Cobb–Douglas production function gives marginal effect of DA on the output of the firms after accounting for other input variables (labor, capital and deposits). Formally, the following regression model is developed to test first hypothesis:
Here, Y is the output of the Cobb–Douglas production function. As the banking sector has a different business structure, the output of banking sector remained a debatable issue in the literature. However, recently many authors used total loans and investments as the output of the banks (Koetter & Noth, Citation2013). We are also using total loans, lending to other institutes and investments as the output variable. Next, we identify DAit as a dummy variable, 1 for the firms who have adopted DA and the following years and 0 otherwise. K is the fixed capital and measured through fixed assets. L is the number of employees. D is the financial capital measured through deposits of banks. ITE is the information technology expense to control for the impact of transactional IT on productivity. ∑ꞵiXit represents matrix of control variables which include type of the banks and listing. Type of the bank is a dummy variable that carries 1 if a bank is a commercial bank and zero for microfinance banks. Listing on stock exchange imposes extra regulations yet they may enhance productivity of the banks through market exposure. Listing is also a dummy variable that carries 1 if the bank is listed on stock exchange and zero otherwise. Uit is a random error term.
Here, P is the profitability of the banks measured through ROA, ROE and net interest income. DAit is a dummy variable, 0 for the firms till the time they have not invested in DA and1 for the firms who have adopted DA and the following years. ITE is the information technology expense. ∑δiZit represents firm-specific variables that include non-performing loans to total loans (NPL to Loans), total equity to total assets ratio (TETA), and total assets (TA). ∑γiXit represents a matrix of control variables which include type of the banks and listing. Uit is a random error term.
4. Result analysis
The descriptive statistics of major variables used in the study are presented below in .
Table 1. Descriptive statistics
The results related to the Cobb–Douglas production function to estimate the impact of DA on the productivity of the firms are shown below in . Since we use the log transformation of a Cobb–Douglas production function, the coefficients of DA can be expressed as a percentage change in output variable due to investment in DA (Hitt et al., Citation2002). We employed three different estimators to test the first hypothesis, including ordinary least square, random effect panel estimation, and Instrumental Variable Two-Stage least square. The first column of presents OLS results and shows that firms’ productivity increases by 8.78% when investment in DA goes live. Next, we estimated the same model using random effect panel estimation to control for time-invariant firm-specific factors. The association between DA and output remained positive and significant, though the magnitude increased to 13.14%. Since the reverse causality and endogeneity remained a major issue in productivity studies; therefore, following Brynjolfsson and McElheran (Citation2019) and Müller et al. (Citation2018), we employed 2SLS/IV with random effect. The DA and the amount spent on software were considered endogenous. Therefore, we used the instrument (i.e. log form of software) to control potential biases arising from omitted variables or reverse causality. The coefficient of DA is significant at 5% and shows that the banks’ productivity is increased by 12.31% if they invest in data analytics. Thus, our empirical analysis fully supports the first hypothesis.
Table 2. Productivity estimates
The results of impact of DA on profitability are presented below in . The impact of DA on ROA is negative and significant. The coefficient of DA shows that ROA decreases by 0.8% when DA goes live. ROE also decreases by 6.4% when investment in DA goes live. Finally, the impact of DA on NII is also negative; it decreases by 11% the year DA goes live. These results are based on random effect panel estimation. The results are consistent with many studies focused on financial sector (Beccalli, Citation2007; Mashal, Citation2006; Onay & Ozsoz, Citation2013). The major justification of negative impact of DA on profitability is due to the huge cost associated with DA investment as when the cost increases the profits fall (Koetter & Noth, Citation2013). While DA causes a significant increase in productivity, it seems that the decline in return is due to the lack of skills and capabilities of human capital. We reject the second hypothesis and conclude that investment in DA causes a decline in profitability of banks.
Table 3. Performance estimate (RE panel estimate)
5. Discussion of results
We used three estimation methods to identify the impact of DA on productivity of banks. All three methods confirm the significant contribution of DA to the productivity of banks consistent with previous literature (Koetter & Noth, Citation2013; Müller et al., Citation2018). This suggests that information technology is an intermediate input for productivity studies in the banking sector. While empirical evidence is more conclusive for the link between productivity and investment in IT, the relation between profitability and investment in IT remained questionable (Koetter & Noth, Citation2013). We investigated the impact of data analytics on banks’ profitability, considering that DA is a unique resource and may show a positive return for banks. But the impact of DA on all performance measures related to profitability (i.e. ROA, ROE and NII) is negative. The increase in productivity but decline in return seems to be associated with lack of human capital skills and capabilities (Schilke, Citation2014). Other reasons of the decrease in profits seem to be due to the increased cost associated with DA investment and subsequent reduced profits (Ho & Mallick, Citation2010)
Carr (Citation2003) argued that information technology does not enhance firm performance as it is an ordinary resource freely available to everyone. Few other studies also confirm profitability paradox in the banking sector (e.g., Beccalli, Citation2007; Ho & Mallick, Citation2010). Thus, it shows that either the banks in Pakistan face the lack of expertise and skill to use DA as a new technology in a competitive manner (Koetter & Noth, Citation2013) or IT does not have a direct impact on performance measures (Hauswald & Marquez, Citation2003). The study argued IT works as an intermediary input to the performance measures and its direct impact on the performance is scarce. Moreover, the relation between IT and firm performance is endogenous by nature (Koetter & Noth, Citation2013; Müller et al., Citation2018). We argue that many studies which established positive relation between IT and profitability, had not used the lagged performance variable as an explanatory variable in the model (e.g., Brynjolfsson et al., Citation2011; Mehmood et al., Citation2015; Ogunyomi & Obi, Citation2016).
6. Conclusion
This paper investigates the impact of investment in DA on productivity and profitability of banks in Pakistan. Our results based on OLS, random effect, and IV-2SLS indicate that live DA is associated with 10% higher productivity on average. However, the impact of DA investment on performance measures including return on assets, return on equity and net interest income is negative. This paper is one of the first to quantify the impact of investment in DA on productivity and profitability for a large sample of banks. To the best of our knowledge, our study is the first study that relied on objective measurements of investment in DA in the financial sector whereas previous studies relied on the amount spent on software, hardware, etc. In addition, our study is the first to provide the productivity estimates of banks in Pakistan’s context by including IT as an additional input variable in the traditional production framework.
This study has profound implications for the firms and professionals including bank managers, policymakers, incumbents and consultants. First, this study offers an insight into the actual outcome of the investment in DA which is highly relevant to the policymakers and regulators to understand its significance and develop policies to facilitate its smooth adoption. Second, by offering the magnitude of productivity estimates, this study will facilitate the decision makers and incumbents to conduct the cost-benefit analysis before investing in data analytics. The incumbents would know exactly how much productivity can be increased if investment in DA is made. Finally, this study clarifies that current pandemic-induced lockdowns are pushing businesses to be online; therefore, the firms need to be at the forefront of digitalization to make their presence sustainable and competitive in the market.
This study has few limitations too. The major limitation of this study is that it focused on the banking sector; therefore, the generalizability to other sectors remains limited. Methodologically, due to the small sample size and panel estimation, we did not use dependent variables’ lagged values as explanatory variables in our models, which is another limitation. Therefore, we recommend that in future studies with larger data sets, lagged performance variables should be included to produce more robust estimates. Other aspects such as employees’ skills and capabilities and banks’ risk management should be studied to document a more rigorous view on the nexus of DA and firm performance.
Additional information
Funding
Notes on contributors
Raazia Gul
Raazia Gul is a PhD-candidate in the Department of Business Administration at Foundation University Islamabad, Pakistan. She has been working as a lecturer at various universities and business schools. Ms. Gul has also been working as a freelance researcher, writer, copy editor and proofreader for more than a decade. She has won gold-medal in MPhil and BSc programs. Her research interests include data analytics, intellectual capital, value creation and firm performance. She has presented in reputable conferences across the world.
References
- Abbasi, A., Sarker, S., & Chiang, R. H. (2016). Big data research in information systems: Toward an inclusive research agenda. Journal of the Association for Information Systems, 17(2), 3. https://doi.org/https://doi.org/10.17705/1jais.00423
- Analytics, M. (2016). The age of analytics: Competing in a data-driven world. Technical report, San Francisco: McKinsey & Company.
- Aral, S., Brynjolfsson, E., & Wu, D. (2006). Which came first, IT or productivity? The virtuous cycle of investment and use in enterprise systems. ICIS.
- Baltagi, B. (2008). Econometric analysis of panel data. John Wiley & Sons.
- Beccalli, E. (2007). Does IT investment improve bank performance? Evidence from Europe. Journal of Banking & Finance, 31(7), 2205–10. https://doi.org/https://doi.org/10.1016/j.jbankfin.2006.10.022
- Brynjolfsson, E. (1993). The productivity paradox of information technology. Communications of the ACM, 36(12), 66–77. https://doi.org/https://doi.org/10.1145/163298.163309
- Brynjolfsson, E., Hitt, L. M., & Kim, H. H. (2011). Strength in numbers: How does data-driven decisionmaking affect firm performance? . Technical report. Available at SSRN 1819486.
- Brynjolfsson, E., & McElheran, K. (2019). Data in action: Data-driven decision making and predictive analytics in US manufacturing. Rotman School of Management Working Paper, 3422397. https://doi.org/https://doi.org/10.2139/ssrn.3422397
- Carr, P. J. (2003). The new parochialism: The implications of the Beltway case for arguments concerning informal social control. American Journal of Sociology, 108(6), 1249–1291. https://doi.org/https://doi.org/10.1086/377517
- Cobb, C. W., & Douglas, P. H. (1928). A theory of production. The American Economic Review, 18(1), 139–165. https://www.jstor.org/stable/1811556
- Davenport, T. H., Harris, J. G., Jones, G. L., Lemon, K. N., Norton, D., & McCallister, M. B. (2007). The dark side of customer analytics. Harvard Business Review, 85(5), 37. https://hbr.org/2007/05/the-dark-side-of-customer-analytics
- Fredriksson, C. (2015). Knowledge management with big data creating new possibilities for organizations. The XXIVth Nordic Local Government Research Conference (NORKOM). Ireland.
- Gul, R., & Ahsan, A. (2019). Big data and analytics: Case study of good governance and government power. 10th European Conference on Intangibles and Intellectual Capital, ECIIC 2019 - Chieti-Pescara, Italy
- Hauswald, R., & Marquez, R. (2003). Information technology and financial services competition. The Review of Financial Studies, 16(3), 921–948.
- Heudecker, N., & Kart, L. (2014). Survey analysis: Big data investment grows but deployments remain scarce in 2014. Gartner.
- Hilbig, R., Etsiwah, B., & Hecht, S. (2018). Berlin start-ups–the rise of data-driven business models. ISPIM Innovation Symposium. Fukuoka, Japan.
- Hitt, L. M., Wu, D., & Zhou, X. (2002). Investment in enterprise resource planning: Business impact and productivity measures. Journal of Management Information Systems, 19(1), 71–98. https://doi.org/https://doi.org/10.1080/07421222.2002.11045716
- Ho, S. J., & Mallick, S. K. (2010). The impact of information technology on the banking industry. The Journal of the Operational Research Society, 61(2), 211–221. https://doi.org/https://doi.org/10.1057/jors.2008.128
- Koetter, M., & Noth, F. (2013). IT use, productivity, and market power in banking. Journal of Financial Stability, 9(4), 695–704. https://doi.org/https://doi.org/10.1016/j.jfs.2012.06.001
- Kohli, R., & Devaraj, S. (2004). Contribution of institutional DSS to organizational performance: Evidence from a longitudinal study. Decision Support Systems, 37(1), 103–118. https://doi.org/https://doi.org/10.1016/S0167-9236(02)00211-7
- Kriebel, J., & Debener, J. (2020). Measuring the effect of digitalization efforts on bank performance. Academy of Management Proceedings, 2020(1), 22004. https://doi.org/https://doi.org/10.5465/AMBPP.2020.22004abstract
- Laney, D. (2001). 3D data management: Controlling data volume, velocity and variety. META Group Research Note, 6(70), 1. https://www.scirp.org/(S(351jmbntvnsjt1aadkposzje))/reference/ReferencesPapers.aspx?ReferenceID=1611280
- Manyika, J., Chui, M., Brown, B., Bughin, J., Dobbs, R., Roxburgh, C., & Hung Byers, A. (2011). Big data: The next frontier for innovation, competition, and productivity. McKinsey Global Institute.
- Martín-Oliver, A., & Salas-Fumás, V. (2008). The output and profit contribution of information technology and advertising investments in banks. Journal of Financial Intermediation, 17(2), 229–255. https://doi.org/https://doi.org/10.1016/j.jfi.2007.10.001
- Mashal, A. (2006). Impact of information technology investment on productivity and profitability: The case of a leading Jordanian Bank. Journal of Information Technology Case and Application Research, 8(4), 25–46. https://doi.org/https://doi.org/10.1080/15228053.2006.10856099
- Mayer-Schönberger, V., & Cukier, K. (2013). Big data: A revolution that will transform how we live, work, and think. Houghton Mifflin Harcourt.
- Mehmood, B., Nisar, A., & Ur Rehman, H. (2015). Technology matters: Evidence from Pakistani banking sector using flexible transcendental logarithmic production function. Pakistan Economic and Social Review, 53(2), 203–224. https://www.jstor.org/stable/26153257
- Müller, O., Fay, M., & Vom Brocke, J. (2018). The effect of big data and analytics on firm performance: An econometric analysis considering industry characteristics. Journal of Management Information Systems, 35(2), 488–509. https://doi.org/https://doi.org/10.1080/07421222.2018.1451955
- OECD. (2014). Data-driven innovation for growth and well-being. Interim synthesis report.
- Ogunyomi, O. O., & Obi, E. (2016). Information and communication technology investment and firm productivity: Evidence from the Nigerian banking industry. Research Journal of Finance and Accounting, 7(8), 47–59. https://www.iiste.org/Journals/index.php/RJFA/article/view/29958
- Onay, C., & Ozsoz, E. (2013). The impact of internet-banking on brick and mortar branches: The case of Turkey. Journal of Financial Services Research, 44(2), 187–204. https://doi.org/https://doi.org/10.1007/s10693-011-0124-9
- Rose, D. (2016). Data science: Create teams that ask the right questions and deliver real value. Apress.
- SBP. (2015). Financial inclusion. State Bank of Pakistan. https://www.sbp.org.pk/Finc/finc.asp.
- SBP. (2020). Covid-19: An unprecedented global health crisis. State Bank of Pakistan. https://www.sbp.org.pk/FSR/2019/Overview.pdf
- Schilke, O. (2014). On the contingent value of dynamic capabilities for competitive advantage: The nonlinear moderating effect of environmental dynamism. Strategic Management Journal, 35(2), 179–203. https://doi.org/https://doi.org/10.1002/smj.2099
- Sodenkamp, M., Kozlovskiy, I., & Staake, T. (2015). Gaining is business value through big data analytics: A case study of the energy sector. Fort Worth: ICIS.
- Someh, A. I., & Shanks, G. (2015). How business analytics systems provide benefits and contribute to firm performance? .ECIS.
- Tambe, P. (2014). Big data investment, skills, and firm value. Management Science, 60(6), 1452–1469. https://doi.org/https://doi.org/10.1287/mnsc.2014.1899
- Toon, M., & Collins, J. (2015). Going beyond the data: Achieving actionable insights with data and analytics. KPMG. https://assets.kpmg/content/dam/kpmg/pdf/2015/04/going-beyond-data-and-analytics-v4.pdf
- Vom Brocke, J., Debortoli, S., Müller, O., & Reuter, N. (2014). How in-memory technology can create business value: Insights from the Hilti case. Communications of the Association for Information Systems, 34(1), 7. https://doi.org/https://doi.org/10.17705/1CAIS.03407
- Wamba, S. F., Akter, S., Edwards, A., Chopin, G., & Gnanzou, D. (2015). How ‘big data’can make big impact: Findings from a systematic review and a longitudinal case study. International Journal of Production Economics, 165(C), 234–246. https://doi.org/https://doi.org/10.1016/j.ijpe.2014.12.031