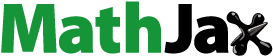
Abstract
This study investigates the price movement characteristics of banking issuers listed on the Indonesia Stock Exchange with macroeconomic indicators as an exogenous variable. By using the k-means clustering based on the monthly rate of return, banks are classified into three clusters, lower, middle, and upper clusters, with a cluster rank directly proportional to the average rate of return. Furthermore, each cluster formed is estimated using dynamic panel data regression with the generalized method of moment. This study shows that the price of banking issuers in the middle cluster is more reactive in dealing with interest rates and exchange rates. At the same time, the highest inflation coefficient exists in the upper cluster. The higher beta coefficient creates abnormal returns that allow for higher gains and line with the risk level. Meanwhile, the price effect in the previous period proved to be in line with the cluster level, indicating that higher clusters are more resilient. Therefore, referring to the possibility of abnormal returns and resilience to uncertainty, this study concludes that issuers in the middle cluster are more suitable for a short-term investment, while issuers in the upper cluster are for the long-term.
PUBLIC INTEREST STATEMENT
This study performs clustering of banking issuers on the Indonesian Stock Exchange based on the monthly rate of return. Bank issuers are divided into three cluster levels directly proportional to their average monthly returns, namely lower, middle and upper. This finding definitely shows that the higher the cluster level tends to be more resilient in challenging economic shocks. Furthermore, this study shows that the price of bank issuers in the middle cluster is more reactive in responding to changes in the interest rate and exchange rate—meanwhile, the upper cluster dealing with the highest inflation variable. Higher coefficient of macroeconomic variables cause volatility and provide a chance for high returns in the short term; however, it tends to be vulnerable to economic shocks. Therefore, this study recommends that issuers in the middle cluster are more suitable for short-term investment and issuers in the upper cluster for the long term.
1. Introduction
Hardouvelis (Citation1987) analyzed the response of financial stock prices on the New York Stock Exchange to macroeconomic information. Share prices respond sensitively to early monetary policy announcements. The result can implicitly state that the stock price reacts to the policies issued by the Central Bank. Similar research by Paul and Mallik (Citation2003) took the object of financial and banking stocks on the Australian Stock Exchange for the period 1980–1999; this study found that banking and financial stocks are cointegrated with macroeconomic variables, particularly GDP, inflation, and interest rates. GDP responded positively by share prices; meanwhile, interest rates and inflation are responded negatively significant.
Furthermore, Talla (Citation2013) uses monthly data from 1993 to 2012 to look at the impact of macroeconomic variables on the Stockholm stock exchange. The Granger Causality test and the ordinary least square regression estimate findings demonstrate that interest rates and exchange rates affect share price; positive inflation and share price are insignificant. Also, in the Singapore stock market, a small open economy with controlled exchange rate targeting, the asymmetric asset-price movements, exchange rates, and stock prices are analyzed using a monetary approach. The exchange rates of the Singapore dollar to developed countries’ currencies are negatively linked to stock prices. In contrast, the relationship between the exchange rate of the Singapore dollar and the Malaysian ringgit and stock prices is positive instead (Wu, Citation2001).
A lot of studies on the relationship between the macroeconomic and stock price movements have also been carried out in Indonesia. Utami et al. (Citation2015), through panel data analysis, found that inflation and interest rates had a significant negative effect on stock returns in the construction subsector, while the exchange rate had a significant positive effect. Other studies also show that only the GDP has a significant effect on the stock price index of the plantation sector, and variables such as interest rates, inflation rates, and the Rupiah exchange rate against the Dollar do not have a significant effect on the stock price index of the plantation sector (Safitri & Kumar, Citation2014). Recent literature on the impact of macroeconomics on the stock market in Indonesia can also be tracked through research by Augustpaosa Nariman (Citation2019), Bassar et al. (Citation2021), Lusiana (Citation2020), and Maharditya et al. (Citation2018)
However, specific studies on the relationship between macroeconomic variables and banking stocks in Indonesia are limited. One of the studies in the Indonesian banking issuers through the monthly price index for the period 2010–2015 show that inflation and exchange rates positively affect the stock price index while interest rates negatively affect it. Lower interest rates stimulate higher investment and better economic activity, which increases stock prices (Laduna & Sun’an, Citation2018). Meanwhile, the partial research on Indonesia’s banking share prices with large capitalization for the 2016–2018 period using the dynamic panel data regression found that gross domestic product and appreciation of the domestic currency against the US Dollar significantly positively affected the banking share price. At the same time, inflation has a negative effect (Zuhroh, Citation2020).
Several previous studies that discuss the effect of macroeconomic variables in stock prices are generally separate from the discussion of firm characteristics. In addition, research on the firm characteristics generally uses classical approaches such as capitalization, transaction volume, and firm size (Diantimala et al., Citation2021; Lim & Hooy, Citation2011; Widiatmoko et al., Citation2020; Yang & Pangastuti, Citation2016). Therefore, this study specifically applies a clustering approach to understand the pattern of stock price movements in response to changes in macroeconomic indicators. Furthermore, this study will provide a new perspective that optimizing investment returns can also be done by understanding the issuer’s characteristics, especially through k-means clustering based on the monthly rate of return. In general, this study contributes as alternative literature on investment portfolio management, specifically for in the investment time frame base on macroeconomic indicators. In particular, this study also expands the literature on Indonesian banking stocks that are exceedingly lacking.
The rest of this paper is organized in the following manner; Section 2 describes data, variable measurements, and research method; Section 3 presents results and a short discussion. Section 4 concludes the paper.
2. Data and methodology
2.1. Data
Adapting empirical studies by Hardouvelis (Citation1987), Laduna and Sun’an (Citation2018), Paul and Mallik (Citation2003), and Utami et al. (Citation2015), and Wu (Citation2001) and considering the availability of monthly data, this study takes four secondary data: a) monthly prices of 28 issuers listed on IDX in the 2016–2019 period (sp); b) the central bank reference rate of the Republic of Indonesia for the period 2016–2019 (ir); c) the USD exchange rate against the rupiah by exchange rate in December 2015 as the baseline (er); d) the inflation rate (inf) measured by the consumer price index (CPI). The CPI is a weighted average of prices for a basket of goods and services, such as transportation, food, and medical care. It’s calculated by average price changes across all items in a predetermined basket of goods. Meanwhile, monthly banking stock’s rate of return calculated by EquationEquation (1)(1)
(1) while
represent rate of return of banking share
in
month,
is the share price of Bank
, in
month.
2.2. k-means clustering
Clustering is one of the most common unsupervised learning methods for exploratory data analysis to gain insight into the data structure. The role of identifying subgroups in the data can be described in such a way that the data points in the same subgroup (cluster) are very similar, whereas the data points are different in each cluster. In other words, we try to find homogeneous subgroups within the data such that data points are as close as possible in each cluster according to a similarity measure (Guojun et al., Citation2007).
The research uses the -means clustering method. Formally, assuming a set of observations, where each observation is a real vector with d-dimension, k-means clustering aims to divide observations into
for every
where
is total data of observation. The set
thus minimizing the within-cluster sum of square-like variants. The purpose of minimizing this variant can be stated in EquationEquation (2)
(2)
(2) and equivalent to minimizing the paired square deviations of points in the same cluster (3). Also, the equivalence can be deduced from identity in EquationEquation (4)
(4)
(4) . Where
is the mean of points in
. When the total variance is constant, it is equivalent to maximizing the sum of the squared deviations between points in different clusters following the law of total variance (Guojun et al., Citation2007; Kriegel et al., Citation2017). In this study, we divided banking issuers into three clusters based on the average monthly rate of return.
2.3. Panel data regression
Arellano & Bover (Citation1995) and Blundell & Bond (Citation1998) introduced the Generalized Method of Moment (GMM) as one of the dynamic panel data processes. This approach is superior to alternative panel data estimators (e.g., pooled ordinary least squares, fixed effects, and GMM differences). It solves econometric issues in lagged dependent variable models induced by unobserved group-specific effects and endogeneity of explanatory variables. Also, the GMM’s estimators proved consistent in parameter estimation and unbiased (Hasan & Gan, Citation2009). Technically, dynamic panel data through GMM also avoids the singularity matrix in the regression process because the explanatory data in this study do not completely panel in nature. Because of this limitation, this study also provides a robustness test to increase the validity and reliability of the regression results.
The two-step GMM approach was chosen over the one-step procedure in this study cause, asymptotically, it looked to be more efficient (Blundell & Bond, Citation1998; Hasan & Gan, Citation2009). Furthermore, as Laporte and Windmeijer (Citation2005) indicated, the strong standard error is derived using sample correction down to the two-step covariance matrix. This study applies Arellano–Bond AR(2) test to identify autocorrelation and to check the validity of overidentifying is a clear application of the J-statistic; the J-statistic would be zero if the equation outside suspect instruments is precisely defined and this study utilizes an appropriate probability lower than 0.1 (Benchimol, Citation2020). The baseline equation was thus set as follows equation (5) Where represents of the cluster,
represent the cross-section unit of
, while t represents the time of the number of
and there are
independent variables on
. By clustering and adjusting for each variable, we propose corresponding equations as follows Equationequations (6)
(6)
(6) .
is the share price of Bank i, in t period, in cluster j;
is interest rate in
period;
is domestic exchange rates converted in USD in t period;
is an inflation in
period measured from the consumer price index;
is the share price of Bank i, in cluster j, in the previous period.
,
,
are successive variable coefficients for banking share prices in the previous period, interest rate, exchange rates and inflation in j cluster;
is the error term or residual term indicates the error of the assessment.
2.4. Robustness test
Robustness is an attribute of resilience that measures the behavior of the system under non-standard conditions. Robustness is characterized as the degree to which a system operates correctly in exceptional inputs or stressful environmental conditions. Since it might also crash the system in the worst-case scenario to cause robustness faults, detecting this form of fault is of utmost importance (Micskei et al., Citation2012). The uncertainty about the expected effect size increase of the robustness test model’s baseline model obtains various point estimates and/or gets larger standard errors. Robustness tests will improve the validity of inferences either way.
Robustness has been defined in various ways in the literature: a) as the same sign and meaning (Leamer); b) as the weighted average effect (Bayesian and Frequently Model Averaging); c) as stability of the effect (Neumayer & Plumper, Citation2017). This research assuming robustness as affecting stability and uses model variance tests to modify and replace model specifications with an alternative assumption. Specifically, change in the set of regressors through controlling variable is applied follows the combination rule. This rule can be modeled as well as formula (5) with the number of models as much as for each cluster, and finally, the EquationEquation (7)
(7)
(7) is obtained.
3. Results and discussion
The clustering results reveal that the lower, middle, and upper clusters, respectively, have a cluster center at 0.010, 0.014, and 0.178, as seen in and directly proportional to the average return. Meanwhile, the highest return variance is in the middle cluster, followed by the lower cluster, and lastly, the upper cluster. Furthermore, the matrix correlation is presented in . Accordingly, no pairs of exogenous variables have a higher value of 0.5 so that the model built is free from multicollinearity (Gujarati, Citation2011).
Table 1. Final cluster center
Table 2. Correlation matrix
The dynamic panel data regression results show that the model in each cluster show a probability value greater than 0.05 for the Arellano-Bond AR (2) test. These results indicate that the model obtained is free from autocorrelation symptoms. Besides, the J-statistic value in each cluster also shows the probability value carried 0.1. The low probability of the J-statistic indicates that the model is free from overidentifying. Based on the AR (2) and J-statistic tests, the model obtained in each cluster is declared valid and reliable.
3.1. Interest rate
Theoretically, lower interest rates are believed to be the trigger for growth. Consumers will spend more income so that consumption and production increase. Lower interest rates will allow the business to have a wide fiscal space of finance operations, acquisitions, and expansion at a cheaper cost, thereby increasing potential future income. Furthermore, the condition will encourage penetration of financing demand to banks and, in turn, lead to higher profit in the future. Conversely, when the Central Bank announces an increase in interest rates, both businesses and households will reduce spending, which leads to lower productivity and demand for financing. This condition is considered a negative sentiment by the market, which in turn pushes the share price.
shows the interest rate in each cluster has a negative and significant coefficient. Previous research conducted on banking issuers in Indonesia also showed the same result, an increase in interest rates negatively affected stock prices (Safitri & Kumar, Citation2014). In addition, Paul and Mallik (Citation2003) also reveals that interest rates negatively affect banking and finance stock prices in the Australian stock exchange. This result brings solid consensus that increasing interest rates negatively affect banking stocks, especially in the Indonesian stock market.
Table 3. GMM model’s estimation results
3.2. Exchange rate
In general, the results of data analysis can be concluded that the strengthening of the USD will increase the price of banking stocks in the middle-upper cluster (See ). This is in line with Wu (Citation2001), which show that the Singapore dollar’s weakening tends to be responded to positively by investors in the Singapore stock exchange. Meanwhile, investors in the lower clusters did not consider exchange rate movements to be valuable information. In contrast, research in Stockholm stock exchange shows that domestic currency depreciation has a significant negative effect on stock prices (Talla, Citation2013). The existence of clusters that do not respond significantly to the exchange rate also strengthens the argument, which states that macroeconomic variables such as exchange rates are not very significant for the capital market for developing countries (Lim & Hooy, Citation2011; Wu, Citation2001).
In terms of financing, when the local currency weakens, financing in foreign currency is certainly less attractive due to high-interest expenses and the cost of margin. Therefore, it is understandable that investors will tend to seek domestic financing. Likewise, if it is associated with global supply chains, when the local currency strengthens, producers can get a more competitive price for raw material through export, but this is not the case when the local currency weakens. There are many possibilities; one of them is looking for substitute local raw materials. The rotation of domestic businesses, in the end, provided local banks the opportunity to expand their financing. The increase in credit growth indicates increasing net profit, which is a positive sentiment for banking issuers.
However, share prices’ negative response to the domestic currency’s strengthening may only occur under normal conditions when the depreciation in extreme pressure, the domestic currency’s weakening makes production costs more expensive due to high economic integration. It will ultimately affect the aggregate supply and demand. High production costs tend to reduce purchasing power; companies will eventually tighten their finances and delay expansion. This will put pressure on the market and investment climate, and the banking industry is no exception. By diversifying their assets through sales, stock market investors reacted to this signal, resulting in a decline in stock prices. Based on the 2016–2019 observation range can see that the strengthening of the USD against the rupiah still has a positive impact in a middle-upper cluster; this is a strong indication that during that period, the weakening and strengthening of the rupiah were still within normal limits.
3.3. Inflation
shows the inflation negatively impacts the lower cluster, insignificant in the middle cluster, and positive significance in the upper cluster. The lower cluster results are in line with Hsing et al. (Citation2011) and Paul and Mallik (Citation2003), which indicate that inflation hurts stock prices. When inflation increases, there is a possibility that the interest rate will also increase and cause demand for bonds to increase; a higher rate of inflation reduces the ability of the government or company to issue debt securities. Therefore, it is more attractive for the private sector to raise funds by selling equity than to issue debt securities; this condition results in increased availability of the stock supply. An increase in stock supply and demand for bonds will ultimately lower stock prices (Quayes & Jamal, Citation2008).
On the other hand, a price increase is a positive signal for economic actors because it includes a profit incentive. A positive response from stock prices to inflation can also occur if the source of inflation is driven by stronger demand for the real sector; strengthening demand is a positive signal for economic growth, which an increase will follow in bank financing disbursements, so, naturally, rising inflation is considered a positive sentiment for investors in upper clusters. These findings in the upper cluster are in line with the finding by Talla (Citation2013), which states that inflation positively affects stock prices in the Stockholm Stock Exchange. Recent research from India also shows that inflation positively correlates with stock prices (Giri & Joshi, Citation2017).
The difference in the direction of the coefficients and the significance level indicates that banking investors in the Indonesian stock market still do not have a strong consensus. Therefore, investors need to understand the direction of inflation in drawing up the investment portfolio, especially in the banking issuers.
3.4. Cluster characteristics
The highest coefficient for the interest rate and exchange rate variables is definitely in the middle cluster, while the inflation variable, in the upper cluster. These imply that banking issuers in the middle cluster are more reactive in responding to the interest rate and exchange rate; meanwhile, issuers in the upper cluster dealing with inflationary shocks. The excessive response causes greater price pressures and increases the risk level when there is turmoil in macroeconomic variables. At the same time, the excessive response comes with volatility and provides opportunities for abnormal returns, which can be one of the strategies to optimize profits in the short term.
Furthermore, for the level of shock resistance to macroeconomic uncertainty, the price coefficient in the previous period can be a valuable indicator. shows that the price coefficient in the previous period directly proportional to the cluster level; the higher the cluster level, the greater the dependence on prices in the previous period. This indicates that higher clusters have better resilience to face macroeconomic shock. Also, keep in mind that the cluster level in line with the higher average rate of return. Based on these findings, we argue that issuers in the middle cluster are more suitable for short-term investments, especially by taking advantage of abnormal returns—meanwhile, banking issuers in the upper cluster for long-term investment projections.
3.5. Result of robustness test
The results of the robustness test, as shown in , show that the model in the upper cluster is consistent in each control variable, and the lower-middle cluster only resilience when the insignificant variable is removed from the model. The results of this robustness test indicate that the GMM model formed in the upper cluster is definitely robust; meanwhile, the lower-middle cluster still has vulnerabilities. However, the results of this robustness test also strengthen the finding that insignificant variables in the lower-middle cluster are truly no impact. In general, the robustness test strengthens the evidence that banking issuers in the upper cluster tend to be more resilient to extreme shocks that are more suitable for long-term investment.
Table 4. GMM model’s estimation results with control variable
4. Conclusions
This study reveals that k-means clustering based on a monthly rate of return after being tested with dynamic panel data regression is proven to reveal the unique characteristics of each cluster. These characteristics include direction and coefficient values, significance, and opportunities for optimizing returns by dividing the investment time frame based on the clusters formed.
This study shows that banking issuers in the middle cluster are more reactive in responding to the interest rate and exchange rate changes—meanwhile, the upper cluster excessive in the face inflation variable. The excessive response makes issuers more sensitive to macroeconomic pressures. However, it also allows for abnormal returns, which can optimize capital gains in the short term. In addition, based on the price coefficient in the previous period, it is clear that the higher clusters have better resilience to macroeconomic shocks. Based on these characteristics, issuers in the middle cluster are more suitable for a short-term investment, while issuers in the upper cluster are for long-term investment.
However, this study also shows that the cluster formed still has a high variance value; therefore, in further research to provide a more established and resilient cluster, the clustering process can be carried out by involving more parameters such as capitalization, transaction volume, and even financial ratios such as return on assets or return on equity. In addition, a wider sample and several cluster-based unsupervised learning methods such as Fuzzy C-means, Hierarchical clustering, and a Mixture of Gaussians need to be implemented as comparisons.
Conflicts of interest
The authors declare no conflict of interest.
Acknowledgements
We acknowledge The Center for Economics, Business and Entrepreneurship Development (PPEBK), Faculty of Economics and Business, Universitas Muhammadiyah Malang (UMM) for funding this research.
Disclosure statement
No potential conflict of interest was reported by the author(s).
Additional information
Funding
Notes on contributors
Idah Zuhroh
Idah Zuhroh has research interests in finance, banking, and Islamic economics. Currently, Idah Zuhroh works at the Faculty of Economics and Business, Universitas Muhammadiyah Malang as an Associate Professor.
Mochamad Rofik is a research fellow at the Center for Economics, Business and Entrepreneurship Development, Faculty of Economics and Business, Universitas Muhammadiyah Malang, with concern in Macroeconomics, Finance, and Labor Economics.
Abdelghani Echchabi is Assistant Professor of Finance, Higher Colleges of Technology, Dubai Men’s Campus, United Arab Emirates. His research scope covers banking, finance, and financial technology.
References
- Arellano, M., & Bover, O. (1995). Another look at the instrumental variable estimation of error-components models. Journal of Econometrics, 68(1), 29–51. https://doi.org/https://doi.org/10.1016/0304-4076(94)01642-D
- Augustpaosa Nariman, H. T. (2019). Faktor-Faktor yang mempengaruhi indeks harga saham gabungan. Jurnal Ekonomi, 24(1), 144. https://doi.org/https://doi.org/10.24912/je.v24i1.546
- Bassar, T. S., Effendi, N., Hidayat, A. K., & Budiono, B. (2021). The effect of inflation rate, exchange rate, The Certificate of Bank Indonesia (SBI) interest rate and sharia stock trading volume on sharia stock performance in companies listed on the Indonesian Sharia Stock Index (ISSI). International Journal of Multicultural and Multireligious Understanding, 8(3), 326. https://doi.org/https://doi.org/10.18415/ijmmu.v8i3.2494
- Benchimol, J. (2020). Memento on EViews output. Technical Guide, Mimeo. https://scholar.harvard.edu/files/jbenchimol/files/memento-eviews.pdf
- Blundell, R., & Bond, S. (1998). Initial conditions and moment restrictions in dynamic panel data models. Journal of Econometrics, 87(1), 115–143. https://doi.org/https://doi.org/10.1016/S0304-4076(98)00009-8
- Diantimala, Y., Syahnur, S., Mulyany, R., & Faisal, F. (2021). Firm size sensitivity on the correlation between financing choice and firm value. Cogent Business & Management, 8(1), 1926404. https://doi.org/https://doi.org/10.1080/23311975.2021.1926404
- Giri, A. K., & Joshi, P. (2017). The impact of macroeconomic indicators on Indian stock prices: An empirical analysis. Studies in Business and Economics, 12(1), 61–78. https://doi.org/https://doi.org/10.1515/sbe-2017-0005
- Gujarati, D. (2011). Econometrics by example. In Social indicators research. Basingstoke: Palgrave Macmillan.
- Guojun, G., Chaoqun, M., & Jianhong, W. (2007). Data clustering: Theory, algorithms and appications. In ASA-SIAM series on statistics and applied probability. ASA-SIAM. https://doi.org/https://doi.org/10.1137/1.9780898718348
- Hardouvelis, G. A. (1987). Reserves announcements and interest rates: Does monetary policy matter? The Journal of Finance, 42(2), 407–10. https://doi.org/https://doi.org/10.1111/j.1540-6261.1987.tb02574.x
- Hasan, B. A. S., & Gan, J. Q. (2009). Unsupervised adaptive GMM for BCI. In 2009 4th international IEEE/EMBS conference on neural engineering, NER ’09 (pp. 295–298). Antalya: IEEE. https://doi.org/https://doi.org/10.1109/NER.2009.5109291
- Hsing, Y., Budden, M. C., & Phillips, A. S. (2011). Macroeconomic determinants of the stock market index for a major latin American country and policy implications. Business and Economic Research, 2(1), 1. https://doi.org/https://doi.org/10.5296/ber.v2i1.1152
- Kriegel, H. P., Schubert, E., & Zimek, A. (2017). The (black) art of runtime evaluation: Are we comparing algorithms or implementations? Knowledge and Information Systems, 52(2), 341–378. https://doi.org/https://doi.org/10.1007/s10115-016-1004-2
- Laduna, R., & Sun’an, M. (2018). The effect of macroeconomic variables on banking stock price index in Indonesia stock exchange. Russian Journal of Agricultural and Socio-Economic Sciences, 73(1), 155–162. https://doi.org/https://doi.org/10.18551/rjoas.2018-01.20
- Laporte, A., & Windmeijer, F. (2005). Estimation of panel data models with binary indicators when treatment effects are not constant over time. Economics Letters, 88(3), 389–396. https://doi.org/https://doi.org/10.1016/j.econlet.2005.04.002
- Lim, K., & Hooy, C.-W. (2011). The delay of stock price adjustment to information: A country-level analysis. Business Valuation Review, 30(2), c1–c36. https://doi.org/https://doi.org/10.5791/0882-2875-30.2.c1
- Lusiana, O. A. (2020). Analisis Pengaruh Inflasi, SBI, Kurs Dan Indeks Global Terhadap Pergerakan Indeks Harga Saham Di BEI Pada Periode 2016-208. In Jurnal Ilmu Manajemen (JIM), 8(4), 1338–1352. https://doi.org/https://doi.org/10.26740/jim.v8n4.p1338-1352
- Maharditya, M. A., Layyinaturrobaniyah, L., & Anwar, M. (2018). Implication of macroeconomic factors to stock returns of Indonesian property and real estate companies. Jurnal Dinamika Manajemen, 9(1), 100–113. https://doi.org/https://doi.org/10.15294/jdm.v9i1.14656
- Micskei, Z., Madeira, H., Avritzer, A., Majzik, I., Vieira, M., & Antunes, N. (2012). Robustness testing techniques and tools. In Resilience assessment and evaluation of computing systems. Berlin, Heidelberg: Springer. https://doi.org/https://doi.org/10.1007/978-3-642-29032-9_16
- Neumayer, E., & Plumper, T. (2017). Robustness tests for quantitative research. In Robustness tests for quantitative research (Methodological tools in the social sciences). Cambridge: Cambridge University Press. https://doi.org/https://doi.org/10.1017/9781108233590
- Paul, S., & Mallik, G. (2003). Macroeconomic factors and bank and finance stock prices: The Australian experience. Economic Analysis and Policy, 33(1), 23–30. https://doi.org/https://doi.org/10.1016/S0313-5926(03)50002-9
- Quayes, S., & Jamal, A. (2008). Does inflation affect stock prices? Applied Economics Letters, 15(10), 767–769. https://doi.org/https://doi.org/10.1080/13504850600770871
- Safitri, I. R., & Kumar, S. (2014). The impact of interest rates, inflation, exchange rates and GDP on stock price index of plantation sector: Empirical analysis on BEI in the year of 2008-2012. In Trends in multidisciplinary business and economic research (pp. 55–61). Bangkok: Global Illuminators. https://www.globalilluminators.org/wp-content/uploads/2014/09/TMBER-14-121.pdf
- Talla, J. T. (2013). Impact of macroeconomic variables on the stock market prices of the stockholm stock exchange [Jönköping University]. In Jonkoping International Business School. http://www.diva-portal.org/smash/get/diva2:630705/FULLTEXT02
- Utami, W. R., Hartoyo, S., Nur, T., & Maulana, A. (2015). The effect of internal and external factors on stock return: Empirical evidence from the Indonesian construction subsector. Asian Journal of Business and Management, 03(05), 370–377. https://www.ajouronline.com/index.php/AJBM/article/download/3167/1661
- Widiatmoko, J., Indarti, M. G. K., & Pamungkas, I. D. (2020). Corporate governance on intellectual capital disclosure and market capitalization. Cogent Business and Management, 7(1), 1750332. https://doi.org/https://doi.org/10.1080/23311975.2020.1750332
- Wu, Y. (2001). Exchange rates, stock prices, and money markets: Evidence from Singapore. Journal of Asian Economics, 12(3), 445–458. https://doi.org/https://doi.org/10.1016/S1049-0078(01)00107-5
- Yang, A. S., & Pangastuti, A. (2016). Stock market efficiency and liquidity: The Indonesia stock exchange merger. Research in International Business and Finance, 36(1), 28–40. https://doi.org/https://doi.org/10.1016/j.ribaf.2015.09.002
- Zuhroh, I. (2020). How banking stock prices respond to gross domestic product, exchange rates and inflation: Empirical studies of Indonesia and Hong Kong. Jurnal Reviu Akuntansi Dan Keuangan, 10(1), 64–78. https://doi.org/https://doi.org/10.22219/jrak.v10i1.10539