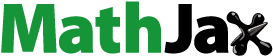
Abstract
As one of the most strategically relevant and financially promising developing industries, the requirements for autonomous driving (AD) systems´ reliability are dramatically higher than in the driver-based car industry. Using the analytic hierarchy process method, this study conducts a quantitative empirical study to prioritise the 15 critical success factors (CSFs) of total quality management (TQM) in the AD-ecosystem. The CSFs are derived from VDA and IATF 16949, two widely accepted TQM-frameworks in the car industry. Comparisons are made between Germany and China as two of the most important places in the world for strategic marketing for autonomous driving.
PUBLIC INTEREST STATEMENT
Autonomous Driving is a major impetus for future innovation in automobile industry. New players from different industries who must implement the complex technology require high level of reliability in these business ecosystems. A suitable total quality management system will guarantee automated and cross-companies coordination.
Germany and China, as two of the most important strategic markets, take leading roles in developing and establishing autonomous driving ecosystems. The present research provides a model that states the role of each member of an autonomous driving system. Then, a quantitative empirical study with 64 experts from Germany and China has been done to prioritise the current critical success factors of the AD-TQM with a comparison overview. Furthermore, several qualitative interviews have been finished to analyse the reasons of the prioritizing as well as to derive the further potential critical success factors of total quality management in this upcoming new industry.
1. Introduction
Total Quality Management (TQM) is a holistic concept for integrating quality as the core purpose for all levels of an organisation (company, institution, etc.). All processes are optimised continuously with the participation of all employees to ensure that the products and services are characterised by high quality (Musenze & Thomas, Citation2020; Rothlauf, Citation2014). TQM is therefore a management approach that aims to achieve a permanent optimisation of processes and procedures (Alanazi, Citation2020; Yusof & Aspinwall, Citation2000). According to a literature review by Aquilani et al. (Citation2017), p. 103 academic papers covering the critical success factors (CSF)s of TQM are described, which shows that the topic has been already developed with certain prescriptions over the past few decades.
It is generally accepted that autonomous driving (AD)-ecosystems will be a fundamental disruption in the car industry and that it is a major impetus for future innovation (Mauer et al., Citation2015; Wang & Meckl, Citation2020). The advantages achieved through innovation, such as increasing the efficiency and safety of the transport system, preventing traffic accidents, providing assistance (e.g., in the case of driver fatigue or driver impairment) to drivers as well as optimising the traffic flow, show the disruptive character of this new technology. In addition, the predicted sustainability of the AD ecosystem integrates not only technology aspects but also will influence and be influenced by non-technical factors such as environment, climate change as well as the use of space (AD will create efficiencies that require less cars on the road or parked on city streets) (Mauer etal., Citation2015, pp.151–73; Mora etal., Citation2020). Consequently,AD-ecosystems have proven to be the focus of research activities of the automotive industry and of public institutions, as well as in economic and academic circles (Mauer et al., Citation2015, p.4).
The necessity for a very high level of reliability within theAD-ecosystem is obvious (Wang & Meckl, Citation2020). In such a highly automated system, deficits in quality from the steering algorithm up to the sensor detection may cause severe damage to lives and material property. Wang and Meckl (Citation2020) have identified 15 CSFs of AD-TQM with a qualitative empirical study as a pre-test for identifying and adapting the relevant factors in this new field. The next step should be prioritising the CSFs to support the players in the AD-ecosystem in implementing a highly reliable and successful ecosystem (Chin et al., Citation2008). Therefore, in this paper, we aim to contribute to the prioritising of CSFs in an AD-TQM-system with a quantitative scale analysis to establish highly reliable technical systems for AD-ecosystems.
Several research studies have already posited that China has the potential to become the world’s largest market for AD vehicles (see e.g., Beiker et al., Citation2016; Pizzuto et al., Citation2019). With huge potential and excellent motivations, new high innovative technologies for AD ecosystem must be integrated in China because of the complex traffic environment and safety requirements (Pizzuto et al., Citation2019; West, Citation2016). Since Germany is already an innovative technology leader at the international level with an excellent image and market acceptance, especially for the automotive industry (Dilk et al., Citation2008; Keck, Citation1993), Germany will also take a leading role in developing and establishing AD-ecosystems (Kaltenhäuser et al., Citation2020). With the big market potential and the high innovation capacity of both countries, it is interesting from a research point of view to compare the strategies the relevant players are implementing in the two countries, also as a means to identify potential ways of cooperation, which may accelerate the success of AD-ecosystem (Schlobach & Retzer, Citation2018). Therefore, a comparison model for understanding the CSFs of AD-TQM between Germany and China will be implemented for advancing research results on that topic, which have been missing up to now.
2. The Autonomous Driving (AD) ecosystem
To identify the CSFs, it is necessary to define and describe a typical AD-ecosystem in its most relevant components. Lima et al. (Citation2016; also see Wang & Meckl, Citation2020) have stated that there are five factors which face the end users (in this case, the drivers) directly in an AD-ecosystem.
S-Car: The autonomous driving car that can communicate with other S-Cars and S-components with CPU, sensors, and driving robots. (Lima et al., Citation2016; Datta et al., Citation2017; Mauer et al., Citation2015, pp. 9–36).
S-Components: Other sentient components, such as motorcycles or the roads and traffic lights with CPU, sensors, and driving robots in the ecosystem which communicate with each other and actively react according to the received information (Lima et al., Citation2016; Datta et al., Citation2017; Mauer et al., Citation2015, pp. 9–36).
Road-Side Units (RSUs): RSUs implement the communication between S-Cars and roads. The data will be acquired and controlled to coordinate the necessary information for the S-Cars about the traffic situations. Near-real-time images of the state of traffic in an area can be generated (Lima et al., Citation2016; Datta et al., Citation2017; Mauer et al., Citation2015, pp. 9–36).
Trusted Authorities (TAs): TAs are public and private organisations such as the government, Technical Inspection Association (TÜV) and National Highway Traffic Safety Administration (NHTSA) for certifying the RSUs, S-Cars, and S-components (Lima et al., Citation2016).
Environment: everything else which is not interconnected in the ecosystem, such as non-autonomous cars, bicycles, and the physical environment itself, i.e., roads, weather, obstacles, etc. (Lima et al., Citation2016; Datta et al., Citation2017; Mauer et al., Citation2015, pp. 9–36).
Based on this definition of players in the ecosystem, the five layers presented in are identified:
Table 1. The five layers of an autonomous driving ecosystem (Lüers et al., Citation2017; Rauen et al., Citation2017; Datta et al., Citation2017; lima et al., Citation2016; Mauer et al., Citation2015, p. 9–36; Wang & Meckl, Citation2020)
As Layer 1, the cloud infrastructure providers implement the computing ability and storage capacity to process, store, and disseminate Big Data in near-real time (Datta et al., Citation2017; Lima et al., Citation2016; Lüers et al., Citation2017; Rauen et al., Citation2017).
The IoT platform providers (IoTPPs), as Layer 2, enable the digital connections of physical objects, as well as the transactions over the IoT via a coordinating platform (Lüers et al., Citation2017; Rauen et al., Citation2017).
The applications and software developers are located in Layer 3. They provide software services and solutions on the platform (Lüers et al., Citation2017; Rauen et al., Citation2017).
The trusted authorities as Layer 4 provide the institutional platform for autonomous driving and certify the components such as roadside units (RSUs), S-Cars, and S-components based on national laws and policies (Datta et al., Citation2017; Lima et al., Citation2016; Lüers et al., Citation2017; Rauen et al., Citation2017).
The producers that manufacture the S-Cars, S-components and RSUs with active sensors and actuators are located in Layer 5 (Lima et al., Citation2016; Lüers et al., Citation2017; Rauen et al., Citation2017, Citation2017).
shows the ecosystem of autonomous driving in a graph (Wang & Meckl, Citation2020).
Figure 1. AD-ecosystem (Wang & Meckl, Citation2020).
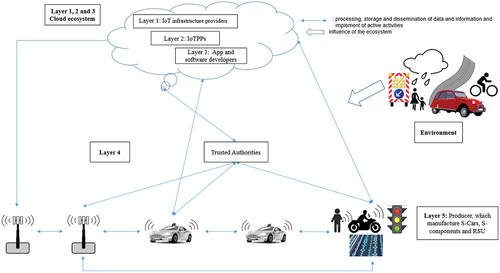
3. CSFs of AD-TQM
The CSFs of TQM have been discussed by many scholars, who have advocated certain prescriptions in different industries over the past few decades. Vimal Kumar and Sharma (Citation2014) have identified 36 CSFs of TQM. According to Aquilani et al. (Citation2017), ten CSFs in TQM have been defined as “most important”. The IATF has integrated seven of the most core CSFs for the automotive industry. Wang and Meckl (Citation2020) have summarized the resulting matrix as :
Table 2. Matrix of the quality principles of IATF 16949 and the CSFs of TQM (Wang & Meckl, Citation2020)
Based on the matrix table, also considering the integration of the German Association of the Automotive Industry (VDA), another relevant and integrated quality management system in the automotive industry, and using the 103 research papers about the CSFs of TQM by Aquilani et al. (Citation2017) as the basis and starting point, Wang and Meckl have developed 15 CSFs of AD-TQM (Franceschini et al., Citation2011; Qualitätsmanagementsystem-, Citation2016; VDA2, Citation2012; Wang & Meckl, Citation2020).
The 15 CSFs are first identified as 15 corresponding hypotheses using conceptual methodology without an empirical study (Arumugam et al., Citation2011; Idris & Zairi, Citation2006; Mandava & Bach, Citation2015; Mustafa & Abdul, Citation2012; Soltani et al., Citation2005; Talib & Rahman, Citation2010). A pre-test was conducted using qualitative cognitive expert interviews (Mellahi & Eyuboglu, Citation2001; Mensah et al., Citation2012; Niu & Fan, Citation2015), so that the 15 CSFs are generated (see ; for details on the CSFs see Wang & Meckl, Citation2020).
Table 3. 15 CSFs of AD-TQM (Wang & Meckl, Citation2020, pp. 21–22)
4. Methodology: quantitative analytic hierarchy process
To prioritise CSFs, the analytic hierarchy process (AHP) approach can be implemented (for details on AHP see e.g., Saaty). The AHP is “hierarchical” because the criteria that are used to solve a problem are always placed in a ranking, i.e., a hierarchical structure. The elements of a hierarchy can be divided into groups, whereby each group only influences one other (“higher”) group of hierarchy elements and is only influenced by one other (“lower”) group (Saaty, Citation1994; Golden et al., Citation1989). It is called “analytical” because it is suitable for comprehensively analysing a complex problem constellation in all its dependencies (Saaty, Citation1994; Golden et al., Citation1989). In addition, the AHP provides a procedural flow of how to prioritise the elements (Saaty, Citation1994; Golden et al., Citation1989), which is also the objective of this paper with the CSFs (“elements”) of an AD-ecosystem.
Due to these characteristics, the AHP has become a widely employed methodology for creating ranking lists. According to Wasil and Golden (Citation2003), thousands of AHP applications have been used to support the generation of strategies of ecosystems in more than 30 diverse areas. It has also been employed in research papers(see for example, Rezazadeh et al., Citation2012) for prioritising the general CSFs of TQM. The common approach, adapted to the research focus of this paper, should be executed as follows (Cheng & Li, Citation2002; Saaty, Citation1994; Lam & Chin, Citation2005; Golden et al., Citation1989):
Identifying the CSFs of AD-TQM
Defining the questionnaire as well as the profile of the experts
Collecting the data by pair-wise comparisons of the CSFs
Calculating the consistency ratio (CR) of the judgments
Representing the weights of the CSFs of AD-TQM
Implementing the comparison between Germany and China, using additional qualitative cognitive expert interviews.
Step 1 has been adopted from prior research, as explained above.
For Step 2, it is important that the questionnaire survey focuses on experts from all five layers. To guarantee high-quality answers, the experts must have direct project experience in connection with an AD-ecosystem. The quantity of the experts from each layer between Germany and China should be the same for a fair comparison. The referencing organisations by each layer, for example, Nokia in infrastructure, Baidu in IoTPPs, Fraunhofer Institute in app developers and software, the provincial government in trusted authorities as well as e.g., Audi in automotive OEMs should be included, so that the results are more convincing. Since AHP does not require the involvement of a large number of experts (Cheng & Li, Citation2001), all the participants interviewees have been reached by personal contacts of the authors, ensuring that the experts have the trust and readiness to express their real opinions of the new, unfamiliar, and sensitive theme instead of issuing statements made with a view to mere political correctness.
Therefore, eight experts in IoT infrastructure providers, 10 experts in IoTPPs, 10 experts in software and app developers, eight experts in trusted authorities, as well as 28 experts in automotive OEMs and system suppliers were sought out in Germany and China. The experts are either senior engineers who are deeply integrated in AD-ecosystem projects or senior managers (CXOs) who must focus on the business strategy of the whole ecosystem. The profile of the participants as well as their employers are reported in . Due to confidentiality, the names of the companies/institutions have been replaced by a description of institutions’ position in the ecosystem and their respective industry.
Table 4. The profile of the interviewees as well as their work organisations
Through a pair-wise comparison (procedure in detail see also Kou et al., Citation2016; Ramík & Korviny, Citation2010), the AHP can rate the CSFs quantitatively. Summing up Step 3, 64 questionnaires were sent to the participants, and all of them responded with the quantitative pairwise comparisons mainly because their permission to do so has been cleared beforehand. In the questionnaires, the responses should evaluate how important they think the 15 CSFs will be for the success of AD-TQM. The participants should assign points (the more points, the more important is the respective factor for the success of AD-TQM) to each factor. As a control condition, the points in total for the 15 CSFs must be 100. A side-document including an explanation of the method and giving background information proved useful since no follow-up inquiries from the participants were made.
To ensure that all the judgments were consistent, the consistency tests of each data set were calculated in the fourth step with the formal CR = CI/RI (see also Chu and Liu, 2002). The RI is the random index and should be defined as 1.58 based on n (CSFs of TQM) = 15 (Yap et al., Citation2018) in this paper. With the formal CI (consistency index) , that λmax is calculated by averaging the value of the consistency vector (procedure in detail also see Alonso & Lamata, Citation2006; Geoff, Citation2004). The consistency ratios CR of these 64 data sets are all below 0.1, which means these data are consistent and reliable (Saaty). The norming of the total points which could be assigned to 100 ensures a high consistency. The consistency indices of each data set are shown in (Appendix).
Therefore, the weighting of the 15 CSFs of AD-TQM can be implemented based on all the 64 questionnaires, which appear in in the Appendix (from highest to lowest weighting). The weighting from the German point of view based on the data of the 32 German experts appears in in appendix and from the Chinese point of view, based on the data of the 32 Chinese experts, in the Appendix shows the weights.
For Step 6, qualitative interviews were conducted as the main approach to an innovative, i.e., unstructured field of research (Bethann et al., Citation2019; Wang & Meckl, Citation2020; Willis, Citation2004, Citation2015). The same method was also integrated by other cited papers about the CSFs of TQM (Mellahi & Eyuboglu, Citation2001; Mensah et al., Citation2012; Niu & Fan, Citation2015). In this paper, this method has been used to interview some of the 64 experts from both Germany and China after the quantitative AHP. This supports the researchers in identifying the unrealised potential interdependencies as well as determining the main reasons for the differences in the prioritising of the CSFs of AD-TQM between Germany and China. The results were meant to highlight the reasons for some big differences in the evaluations of experts from both countries. Therefore, in the present paper, six experts from Germany and China were interviewed. Their evaluated points as well as the correlations are shown in (n/a is the abbreviation for “not available”).
Table 5. Evaluations of the six responses from the interviews
In addition, the interviews were a chance to discuss with the experts whether and why they want to add new factors to the CSF list of AD-TQM. The proposed new CSFs were discussed until both experts and authors agreed on the new ideas.
5. Results and discussion
We ranked the weights of the CSFs along four levels:
Very important (8% ≤ normalised priority weight)
Important (7% ≤ normalised priority weight < 8%)
Neutral (6% ≤ normalised priority weight < 7%)
Less important (normalised priority weight < 6%)
Taking the four levels, the German and Chinese experts have the same opinion on CSF 8 (Data and information exchange procedure). It is the only “very important” CSF of AD-TQM. Collecting, analysing, and exchanging the relevant data and information between the five layers to reach a conformity of products and services, customer needs and satisfaction, as well as the implementation and performance of the TQM system does make the most relevant contribution to a high level of an AD-TQM-system. As a consequence, the building up of such an exchange procedure should be one of the critical challenges in an AD-ecosystem. With players from very different backgrounds (see ), solving this task requires high capabilities in ecosystem governance and coordination.
Several previous research studies have already stated that the implementation of the Big Data has significant implications for sustainability with the excellent understanding of operations, determining the environment (energy) risks, optimising the resource implementation, as well as establishing better regulation (see e.g., Dubey et al., Citation2019;).The present research has proved that these considerations are also suitable for building up an AD-TQM system.
The six CSFs 15, 13, 10, 3, 5, and 14 are evaluated from both sides, Germany and China as “neutral” CSFs, which means these factors should be considered for building up the AD-TQM but are not the highest priorities.
From both Germany and China, it is stated that CSF 4, to involve and train all the employees for AD-TQM, seems to be a “less important” CSF, which is somehow surprising, regarding the high relevance of the human factor in traditional TQM-systems. Further interviews with the experts (Experts 2, 4, 5, and 6, whose points are all less than 6% by CSF 4) show that the eperts doubtthere are enough resources to train all the employees of every player because of the high complexity and innovation of the AD-ecosystem. In addition, the level of the automation of the AD ecosystem will be extremely high, which means that not all employees significantly influence the success of the AD-ecosystem. Some reference roles such as the technology and marketing are very important. Therefore, it makes more sense to train solely the target employees who are highly relevant for the performance of the ecosystem.
The enterprise resource management theory, especially the resource capacity, as well as resource prioritisation and allocation in sustainable development (see e.g., Hoch & Dulebohn, Citation2013; K. Kumar & van Hillegerberg, Citation2020; Parris & Kates, Citation2013), could be the theoretical basis for explaining the very surprising result that most resources should be planned and placed for the most important tasks, in this case, to train the target and relevant employees of the AD ecosystem with limited resources.
In further discussion with the interviewees, they all agreed that resource management could be a new important CSF of AD-TQM with the explanation that in practice, the relevant resources are always limited. With the integration of such considerations in the CSFs, the implementation of an AD-TQM can be planned and considered in a more practical way.
Therefore, a new CSF (CSF 16) of AD-TQM could be defined as:
16. The participants of all five layers should plan and prioritise their limited resources for the most important processes of AD-TQM.
Because of the massive difference between Germany and China, CSF 11 (legal framework and sharing of competences between public and private institutions) is discussed ambiguously. It is (only) ranked as a middle “neutral” CSF for Germany but scored as the highest “important” CSF for China. Because of the high scoring on the Chinese side, it is considered as the highest “important” (one level below the “very important”) CSF of AD-TQM in total. According to the further discussions with the experts referenced, the biggest difference is to define/integrate the role and responsibility of the government for the AD-ecosystem. German eperts recognise no priority and stay quite neutral on whether the government should be really integrated to realise AD technology. Chinese experts believe that one of the most important parts should be the legal framework which cannot be realised without the government. In addition, the government may take on the orchestrator role to coordinate other layers based on the law and policy aspects.
Several papers have already asserted that in general, a good government should develop and maintain strategies, policies, and programs to promote sustainability (Bell, Citation2002; Saha, Citation2009). Specifically for AD ecosystems, research studies have also discussed that the government may offer support to build up the necessary infrastructure as well as to define new laws and a regulatory framework (Mauer et al., Citation2015, pp. 151–73). This paper has contributed to further discussion, clarifying that the exact role and position of the government should be defined clearly and will be very different based on diverse countries with varying cultural backgrounds in AD-ecosystem.
CSF 9 (a standard and consistent release process for product and production in the ecosystem) is also in discussion because the evaluations are ranked as a “neutral” CSF by Germany but as an “important” CSF by China, which leads to an “important” CSF of AD-TQM in total. For German experts, this CSF is a support process to ensure that the integrated technologies from different layers are reliable. Therefore, the technologies should be most important instead of the release process itself. On the other hand, the Chinese experts prefer to have an AD-ecosystem in the market in which the technology is perhaps not so mature at first but can be developed continuously, based on the feedback from the market and Big Data analytics. Therefore, a standard release process to ensure the security of the AD-ecosystem is important.
A similar discussion can also be seen in CSF 2 (implemented emergency plan): the German viewpoint is a “neutral” but CSF 2 is defined as “important” by China, the same as the ranking in total. During the further interviews, the German experts have the similar statement that a mature technology of the AD-ecosystem (both products and production) should be developed, which means a complex emergency plan is not needed. In contrast, China prefers the same as CSF 9: to have an AD-system in the market first without the (“over required”) full maturity so that an emergency plan to ensure the security and reliability of the AD-ecosystem is needed. Therefore, it is judged as “important”.
The discussion of CSF 7 (predictive maintenance processing) is critical, since to the Germans it is “important” while it is ranked as a middle “neutral” CSF by China. It is considered to be as a “neutral” CSF of AD-TQM in total. Based on the further interviews of the experts, the different understandings (similar to CSFs 9 and 2) for “technology first” in Germany and “market first” in China are also the main reasons here. The German experts have stated that automatic predictive maintenance should be one of the innovative new technologies to ensure the matureness of the AD-ecosystem during the whole system life, which leads it to be an “important” CSF. On the other hand, the experts in China prefer to concentrate on the development of the AD-ecosystem itself instead of investing many resources to integrate an automatic predictive maintenance technology, which could slow the market entrance because of the high investment required and a too-long development period.
The CSF 12 (automated environment application) is ranked from the German side as “important”. From China, it is judged as the highest “neutral” CSF, where the CSF is also ranked as “neutral” in the total overview. A similar justification of this difference is also the “technology first” reasoning from Germany or “market first” from China. In German opinion, the start-stop function based on the suitable application environment of an AD-ecosystem should be one of the interesting new and innovative technologies to be implemented in the AD-ecosystem, whereas the Chinese experts agree with this statement but at the same time they also fear that the suitable application environment cannot be determined correctly. As a consequence, there could be too many “stops based on fake false environment”, so that the driver experience of the AD-ecosystem could be negatively influenced. Therefore, CSF 12 is ranked as “neutral”.
Because of the huge difference between Germany and China, the CSF 6 (digital curriculum vitae) is also in discussion. It is ranked as the lowest “less important” (5.72%) CSF for Germany but scored as a middle “neutral” (6.53%) CSF for China. In sum, it is judged as a “neutral” CSF of AD-TQM. During the further interviews, the German experts stated that the digital curriculum vitae for all the components of an AD-ecosystem is only an excellent theoretical idea. In practice, they cannot image which technologies can be integrated to realise such a complex innovative approach. In addition, there is uncertainty about whether it makes sense to invest so many resources regarding the risk that, due to the high complexity of such a system, the performance of the AD technology is not improved by a digital curriculum vitae. Therefore, it is ranked as the lowest “less important”. In contrast, the China experts have the opinion that such a technology could help to define the responsibility of the potential failures and improve the trust of the end users for the AD-ecosystem. Of course, to produce all the components with such a complex and expensive technology is not possible, but it can be implemented for the important parts (for example, the algorithm of AD software). It could be a huge advantage for the marketing side to show the industry’s innovation to the customers. Thus, it is weighted as a middle “neutral” CSF.
According to previous research (see e.g., Hall & Vredenburg, Citation2003; Kolk & Van Tulder, Citation2010; Lubin & Esty, Citation2010), it can be stated that both technological innovation and marketing development are the critical factors, which have close interactions (innovation could create a sustainable marketing profit and a sustainable marketing profit would encourage the continuous technical investments and motivations) for the sustainability of a business ecosystem. With regard to this phenomenon, the present paper has demonstrated the significat difference in the understanding and prioritisation of the two critical factors from Germany and China and opened a further discussion to analyse the reasons for the difference as well as to achieve a balance between the two factors to develop a reliable AD-TQM.
In further discussions with the interviewees, they have stated that the significant difference is because of the different understanding from Germany and China regarding new innovative business ecosystems. It should not be defined as a new CSF of AD-TQM because the cultural difference could influence many other aspects and can’t be considered as a separate factor.
For the CSF 1, the handling of complaints in the AD-ecosystem, the total rating is scored as “less important”. The ratings between Germany and China are different but both ratings (Germany: 6.00%; China: 5.94%) are at the borderline between “neutral” and “less important” with similar understandings.
The summary of the analysis is shown in .
Table 6. Weights of the CSFs of AD-TQM according to the four levels of importance in total and between Germany and China
6. Conclusions, limitations, and outlook
The purpose of this study is to prioritise the 15 CSFs of AD-TQM quantitatively and implement a comparison between Germany and China.
The AHP method was adopted. 64 experts from all five layers of an AD-ecosystem from Germany and China who are either senior experts of AD-projects or the senior managers who must focus on the strategy of the new ecosystem were selected. The 64 experts completed 64 quantitative pair wise questionnaires to weight the 15 CSFs of an AD-TQM-system. The consistency ratios are all below 0.1, which means that all the data sets are reliable for further analysis. Six experts were interviewed for the further explanations of the differences in results between Germany and China.
Four levels of the weights as “very important”, “important”, “neutral”, and “less important” were defined based on the scorings of the CSFs.
The CSF 8, data and information exchange and analysis in the AD-ecosystem, proved to be the only “very important” CSF for the success of the AD-TQM.As theoretical explanations, several previous research studies have already stated the theory of Big Data for sustainability. The CSFs 11, 9, and 2, to define the responsibilities of the public and private organisations to certify the layers in AD-ecosystem, define a release process of product and production, as well as to integrate an emergency plan of application, have been defined as the “important” CSFs of AD-TQM. The CSFs 7, 12, 15, 13, 10, 3, 5, 14 and 6 have been judged as the “neutral” CSFs. The CSF 1, handling of the complaints, as well as the CSF 4, involving and training of all the employees, have been ranked as the two “less important” CSFs. For the surprising evaluation of CSF 4, the enterprise resource management theory in sustainable development could be a theoretical basis for an explanation. Additionally, it has been selected as a new important CSF during the interviews for a practical reliable implementation of an AD-TQM.
Seven CSFs showed different evaluations between Germany and China. One of the biggest differences of understanding between Germany and China is the role and responsibilities of the government in the AD-ecosystem (CSF 11) which has been also discussed by other referenced research. The German experts stayed neutral and had no preference whether government, or generally speaking public authorities, should be tightly integrated in the ecosystem, whereas the Chinese experts determined that the participation of government authorities is a precondition for a successful AD-TQM, based on law and policy aspects. According to some Chinese experts interviewed (Expert 2, 4, and 6), the government should even take the orchestrator role to coordinate other participants to realise the AD-TQM. The basic result thus is that CSF 11, the responsibilities of the public organisations, does not correspond between Germany and China. The Germans ranked it as “neutral”, while the Chinese scored it as the highest “important” CSF.
The other big difference of understanding of how to develop the AD-ecosystem between Germany and China is the “technology first” attitude in Germany versus the “market first” attitude in China. The German experts interviewed (Expert 1, 3, and 5) preferred to have a mature technology before the market rollout, so that the applications of the AD-ecosystem could be secure and reliable for the long-term stable development of the industry. On the other hand, the Chinese experts interviewed (Expert 2, 4, and 6) had the idea to roll out the AD technology to the market without introducing “overly required” matureness. Afterwards, the AD-ecosystem can be developed and optimised continuously and efficiently based on the feedback from the customers and on Big Data analytics for the applications. Because of this basic difference, the CSFs 9, 2, 7, 12, 6 and 1 are differently ranked from the German and Chinese sides. These two factors (“technology first” and “market first”) have close interactions and should be realized with an feasible balance for a sustainable AD-TQM.
Furthermore, an important contribution of this study is in relation to sustainability and generally with compliance to ESG-related management. Our study contributes to promoting an environmentally important and socially desirable new system of mobility. Identifying and empirically validating CSFs are preconditions for establishing the future environment-central mobility system. It helps to efficiently operate this system and to ensure a long-lasting survival of an AD ecosystem that helps make mobility cheaper and easier to use.
The limitations of this approach may be found on the methodological level. In the AHP-approach, the subjective factor in filling out the questionnaires and thus determining the hierarchical structure are obvious (see also Kaliyamurthi, Citation2017; Oguztimur, Citation2011). What is more, the sample consisted of 64 experts as well as six interviewed experts from two different countries, which means that for each layer and each country, the number of experts may be too low to guarantee a generally valid result. Experts from all five layers, and also from other interesting countries such as the USA or Japan, should be integrated in future studies to reach a higher level of empirical verification. In this case, the present research can be defined as a starting basis and an official introduction toward beginning a research project for acquiring the experience of the experts in all five layers and all relevant countries without depending upon personal contacts . Furthermore, multi-level sample sizes are required since variations in results may occur depending on different criteria or different groups such as gender, age, ethnic group, etc. Therefore, the CSF list should be a dynamic construct with the idea that the CSFs have been defined and prioritised according to the current technology based on the present surveys. New CSFs with new prioritising resulting, e.g., from technological developments, could be stated with further quantitative research and interviews.
Future research should also focus on the analysis and implementation of the new CSF “resource management”. Moreover, an interesting result is the differing opinion on the role of the government in AD-ecosystems. Future research should develop types of AD-ecosystems with different levels of government involvement and evaluate the effects on TQM. Furthermore, it is also necessary to study the phenomenon and the associated meanings of the “technology first” approach in Germany and “market first” in China for building up a successful AD-TQM system in general. Such a study could help to define the orchestrators as well as their exact roles in order to see who will and should take which responsibilities (and how) to build up a reliable AD-TQM in the future. What is more, further relevant CSFs of AD-TQM should be integrated and prioritised continuously by further surveys with large quantitative data. In addition, the cooperation and coalition between Germany and China to build up an international standard AD-TQM could be implemented after analysing the differences in understanding for the complex innovative new ecosystem.
In summary, Wang and Meckl (Citation2020) have identified 15 CSFs of AD-TQM as the first step with a qualitative pre-test according to (only) four interviews of experts by only two layers (three by layer 5 and one by layer 3). As a further procedure, the present research has prioritised the 15 CSFs according to 64 quantitative empirical studies based on the data from all five layers as a critical further step. Moreover, six qualitative interviews have been conducted to analyse the reasons for the prioritising as well as to determine further potential new CSFs of AD-TQM. With this analysis, the understandings of the AD-TQM ecosystem have been implemented more comprehensively (all the layers have participated) and deeply (much more experts have participated with quantitative evaluations). The importance and sensitivity of the topic has been also widely extended from both the theoretical and practical side (several senior managers for example, the president, the CEO and the CMO of several very famous institutional organisations have been quantitative questioned and qualitative interviewed). Several further research directions such as discussing the orchestrators of the AD ecosystem and defining the exact role and responsibilities of the government in the AD ecosystem have been specified much more clearly.
Disclosure statement
No potential conflict of interest was reported by the author(s).
Additional information
Funding
Notes on contributors
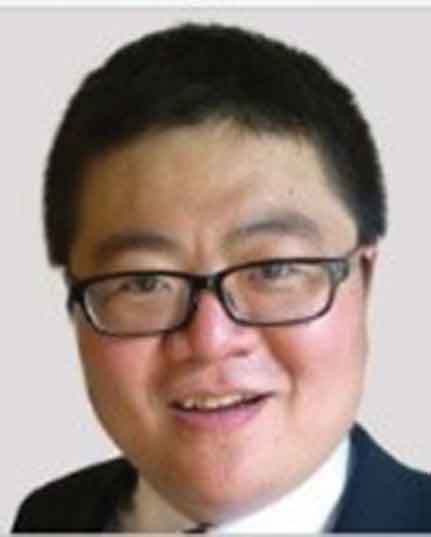
Zinan Wang
Zinan Wang, M.Sc., has been working for several years as the China CEO of a German hidden champion supplier in the automotive industry. He is a certificated VDA 6.3 quality auditor as well as a CQI 15 and CQI 17 quality auditor. At the same time, he is a research assistant at the Chair for International Management at the University of Bayreuth, Germany. His research interests include total quality management in the field of autonomous driving as well as the strategy of the internationalisation between Germany and China in the autonomous driving ecosystem.
Reinhard Meckl
Prof. Dr. Reinhard Meckl is Chair for International Management at the University of Bayreuth, Germany. His main research areas are international digital business models, cross border mergers and acquisitions, and German-Chinese business relations.
References
- Alanazi, M. (2020). The mediating role of primary TQM factors and strategy in the relationship between supportive TQM factors and organisational results: An empirical assessment using the MBNQA model. Cogent Business & Management, 7(1), S. 1771074. Jg., Nr. https://doi.org/10.1080/23311975.2020.1771074.
- Alonso, J., & Lamata, M. (2006). Consistency in the analytic hierarchy process: A new approach. International Journal of Uncertainty, Fuzziness and Knowledge-based Systems, 14(4), S. 445–459. Jg., Nr. https://doi.org/10.1142/S0218488506004114.
- Aquilani, B., Silvestri, C., Ruggiere, A., & Gatti, C. (2017). A systematic literature review on total quality management critical success factors and the identification of new avenues of research. The TQM Journal, 29(1), 184–25. https://doi.org/10.1108/TQM-01-2016-0003
- Arumugam, V. C., Mojtahedzadeh, R., & Malarvizhi, C. A. Critical success factors of total quality management and their impact on performance of Iranian Automotive Industry. In: International conference on innovation, management and service, IACSIT Press, Singapore. 2011. P. 312–316.
- Beiker, S., Hansson, F., Suneson, A., & Uhl, M. (2016). How the convergence of automotive and tech will create a new ecosystem. McKinsey & Company, Automotive & Assembly.
- Bell, D. A. V. I. D. V. J. The role of government in advancing corporate sustainability. 2002. Final draft. Sustainable Enterprise Academy, York University (Canada).
- Bethann, A., Buschle, C., & Reiter, H. (2019). Kognitiv oder qualitativ? In Natalja Menold, Tobias Wolbring, (Eds.).Qualitätssicherung sozialwissenschaftlicher Erhebungsinstrumente (pp. S. 159–193). Springer VS.
- Cheng, E., & Li, H. (2001). Analytic hierarchy process: An approach to determine measures for business performance. Measuring Business Excellence, 5(3), 30–37. https://doi.org/10.1108/EUM0000000005864
- Cheng, E., & Li, H. (2002). Construction partnering process and associated critical success factors: Quantitative investigation. Journal of Management in Engineering, 18(4), 194–202. Jg., Nr. https://doi.org/10.1061/(ASCE)0742-597X(2002)18:4(194)
- Chin, K., Chan, B., & P, L. A. M. (2008). Identifying and prioritizing critical success factors for coopetition strategy. Industrial Management & Data Systems, 108(4), 437–454. https://doi.org/10.1108/02635570810868326
- Chu, P., & J, L. I. U. (2002). Note on consistency ratio. Mathematical and Computer Modelling, 35(9–10), S. 1077–1080. Jg., Nr. https://doi.org/10.1016/S0895-7177(02)00072-9
- Datta, S., Haerri, J., Bonnet, C., & Da Costa, R. (2017). Vehicles as connected resources: Opportunities and challenges for the future. IEEE Vehicular Technology Magazine, 12(2), S. 26–35. Jg., Nr. https://doi.org/10.1109/MVT.2017.2670859.
- Dilk, C., Gleich, R., Wald, A., & Motwani, J. (2008). Innovation networks in the automotive industry: An empirical study in Germany. International Journal of Automotive Technology and Management, 8(3), 317–330. https://doi.org/10.1504/IJATM.2008.020215
- Dubey, R., Gunasekaran, A., Childe, S. J., Papadopoulos, T., Luo, Z., Wamba, S. F., & Roubaud, D. (2019). Can big data and predictive analytics improve social and environmental sustainability? Technological Forecasting and Social Change, 144, 534–545. https://doi.org/10.1016/j.techfore.2017.06.020
- Franceschini, F., Galetto, M., Maisano, D. A., & Mastrogiacomo, L. ISO/TS 16949: Analysis of the diffusion and current trends. Proceedings of the Institution of Mechanical Engineers, Part B: Journal of Engineering Manufacture, 2011, 225. SAGE. Jg., Nr. 5, P. 735–745.
- Geoff, C. (2004). The analytic hierarchy process (AHP). Practical Strategy. Open.
- Golden, B., Wasil, E., & Harker, P. T. (1989). The analytic hierarchy process. In Bruce L. Golden, Edward A. Wasil, & Patrick T. Harker, (eds.), Applications and Studies (pp. 2). Heidelberg. Jg.
- Hall, J. E. R. E. M. Y., & Vredenburg, H. A. R. R. I. E. (2003). The challenge of innovating for sustainable development. MIT Sloan Management Review, 45(1), S. 61 . Jg., Nr.https://sloanreview.mit.edu/article/the-challenges-of-innovating-for-sustainable-development/
- Hoch, J. E., & Dulebohn, J. H. (2013). Shared leadership in enterprise resource planning and human resource management system implementation. Human Resource Management Review, 23(1), 114–125. https://doi.org/10.1016/j.hrmr.2012.06.007
- Idris, M., & Zairi, M. (2006). Sustaining TQM: A synthesis of literature and proposed research framework. Total Quality Management and Business Excellence, 17(9), 1245–1260. Jg., Nr. https://doi.org/10.1080/14783360600750535.
- Kaliyamurthi, K. P. (2017). A Comparison of strength and weakness for analytical hierarchy process. International Journal of Pure and Applied Mathematics, 116(8), 29–33. http://www.ijpam.e
- Kaltenhäuser, B., Werdich, K., Dandl, F., & Bogenberger, K. (2020). Market development of autonomous driving in Germany. Transportation Research Part A: Policy and Practice, 132, 882–910. https://doi.org/10.1016/j.tra.2020.01.001
- Keck, O. T. T. O. (1993). The national system for technical innovation in Germany. In Richard R. Nelson, (Eds.), National innovation systems: A comparative analysis (pp. S. 115–157).
- Kolk, A. N. S., & Van Tulder, R. O. B. (2010). International business, corporate social responsibility and sustainable development. International Business Review, 19(2), S. 119–125. Jg., Nr. https://doi.org/10.1016/j.ibusrev.2009.12.003.
- Kou, G., Ergu, D., Lin, C., & Chen, Y. (2016). Pairwise comparison matrix in multiple criteria decision making. Technological and Economic Development of Economy, 22(5), S. 738–765. Jg., Nr. https://doi.org/10.3846/20294913.2016.1210694.
- Kumar, K., & van Hillegerberg, J. (2020). Enterprise resource planning: Introduction. Communications of the ACM, 43(4), 22–26. https://doi.org/10.1145/332051.332063
- Kumar, V., & Sharma, R. R. K. (2014). TQM implementation: Relating critical success factor to strategy of the firm. California Business Review, 2(1), S. 19–24. Jg., Nr. https://doi.org/10.18374/CBR-2-1.3.
- Lam, P., & Chin, K. (2005). Identifying and prioritizing critical success factors for conflict management in collaborative new product development. Industrial Marketing Management, 34(8), 761–772. Jg., Nr. https://doi.org/10.1016/j.indmarman.2004.12.006.
- Lima, A., Rocha, F., Völp, M., & Esteves-verissimo, P. Towards safe and secure autonomous and cooperative vehicle ecosystems. In: Proceedings of the 2nd ACM workshop on cyber-physical systems security and privacy. Vienna Austria: Association for Computing Machinery New York NY United States. 2016. S. 59–70.
- Lubin, D. A., & Esty, D. C. (2010). The sustainability imperative. Harvard Business Review, 88(5), 42–50. https://www.engema.org.br/22/wp-content/uploads/sites/10/2020/11/Lubin-Esty-HBR-SustainabilityImperative.2010.pdf
- Lüers, M., Becker, L., Walti, J., & Houta, H. (2017). Mastering the Industrial Internet of Things (IIoT). Roland Berger.
- Mandava, T., & Bach, C. (2015). Total quality management and its contributing factors in organizations. Total Quality Management, 2(12), 3504–3510. Jg., Nr.http://www.jmest.org/wp-content/uploads/JMESTN42351275.pdf
- Mauer, M., Gerdes, J. C., Lenz, B., & Winner, H, (Eds.). (2015). Autonomous driving: Technical. Legal and Social Aspects. https://doi.org/10.1007/978-3-662-48847-8
- Mellahi, K., & Eyuboglu, F. (2001). Critical factors for successful total quality management implementation in Turkey: Evidence from the banking sector. Total Quality Management, 12(6), 745–756. Jg., Nr. https://doi.org/10.1080/09544120120075352.
- Mensah, J., Copuroglu, G., & Fening, F. (2012). Total quality management in Ghana: Critical success factors and model for implementation of a quality revolution. Journal of African Business, 13(2), 123–133. Jg., Nr. https://doi.org/10.1080/15228916.2012.693444.
- Mora, L., Wu, X., & Panori, A. (2020). Mind the gap: Developments in autonomous driving research and the sustainability challenge. Journal of Cleaner Production, 275, 124087. https://doi.org/10.1016/j.jclepro.2020.124087
- Musenze, I., & Thomas, M. (2020). Development and validation of a total quality management model for Uganda’s local governments. Cogent Business & Management, 7(1), S. 1767996. Jg., Nr. https://doi.org/10.1080/23311975.2020.1767996.
- Mustafa, E., & Abdul, T. (2012). Role of top management leadership and commitment in total quality management in service organization in Malaysia: A review and conceptual framework. In P. Jg. (Ed.), Elixir Human Resource Management (Vol. 51, pp. 11029–11033).Elixir Journal.
- Niu, R., & Fan, Y. (2015). An in-depth investigation on quality management practices in China. International Journal of Quality & Reliability Management, 32. https://doi.org/10.1108/IJQRM-10-2013-0175
- Oguztimur, S. (2011). Why Fuzzy Analytic Hierarchy process Approach For Transport Problems? In 51st Congress of the European Regional Science Association: ”New Challenges for European Regions and Urban Areas in a Globalised World”, 30 August - 3 September 2011. Barcelona, Spain. Louvain-la-Neuve: European Regional Science Association.
- Parris, T. M., & Kates, R. W. (2013). Characterizing and measuring sustainable development. Annual Review of Environment and Resources, 28(1), 559–586. https://doi.org/10.1146/annurev.energy.28.050302.105551
- Pizzuto, L., Thomas, C., Wang, A., & Wu, T. (2019). HOW CHINA WILL HELP FUEL THE REVOLUTION IN AUTONOMOUS VEHICLES. McKinsey&Company.
- Qualitätsmanagementsystem-, I. A. T. F. (2016). Standard der Automobilindustrie: Anforderungen an Qualitätsmanagementsystem für die Serien. Und Ersatzteilproduktion in der Automobilindustrie. International Automotive Task Force.
- Ramík, J., & Korviny, P. (2010). Inconsistency of pair-wise comparison matrix with fuzzy elements based on geometric mean. Fuzzy Sets and Systems, 161(11), S. 1604–1613. Jg., Nr. https://doi.org/10.1016/j.fss.2009.10.011.
- Rauen, H., Zollenkop, M., Glatz, R., Lüers, M., Schnittler, V., Becker, L., Peters, K., & Schorak, M. (2017). Plattformökonomie im Maschinenbau: Herausforderungen- Chancen – Handlungsoptionen. Roland Berger.
- Rezazadeh, A., Najafi, S., Hatami-shirkouhi, L., & Miri-nargesi, S. (2012). Evaluating and prioritising critical success factors of TQM implementation based on fuzzy AHP. International Journal of Productivity and Quality Management, 9(1), S. 1–24. Jg., Nr. https://doi.org/10.1504/IJPQM.2012.044009.
- Rothlauf, J. (2014). Total quality management in Theorie und Praxis: Zum ganzheitlichen Unternehmensverständnis. Walter de Gruyter.
- Saaty, T. L. (1994). Fundamentals of decision making and priority theory with the analytic Hierarchy Process ( pp. 204–220). RWS Publications.
- Saha, D. E. V. A. S. H. R. E. E. (2009). Factors influencing local government sustainability efforts. State and Local Government Review, 41(1), S. 39–48. Jg., Nr. https://doi.org/10.1177/0160323X0904100105.
- Schlobach, M., & Retzer, S. (2018). Defining the future of mobility: Intelligent and Connected Vehicles (ICVs) in China and Germany. Opportunities, Challenges and the Road Ahead. GIZ, 46. http://icv.sustainabletransport.org/ICV_final.pdf
- Soltani, E., Lai, P., & Gharneh, N. (2005). Breaking through barriers to TQM effectiveness: Lack of commitment of upper-level management. Total Quality Management and Business Excellence, 16(8–9), 1009–1021. Jg., Nr. https://doi.org/10.1080/14783360500163201.
- Talib, F., & Rahman, Z. (2010). Critical success factors of TQM in service organizations: A proposed model. Services Marketing Quarterly, 31(3), 363–380. Jg., Nr. https://doi.org/10.1080/15332969.2010.486700.
- VDA2. (2012). Qualitätsmanagement in der Automobilindustrie- Sicherung der Qualität von Lieferungen: Produktionsprozess- und Produktfreigabe (PPF). Verband der Automobilindustrie.
- Wang, Z., & Meckl, R. (2020). Critical success factors of total quality management in autonomous driving business models. Cogent Engineering, 7(1), 1767018. Jg., Nr. https://doi.org/10.1080/23311916.2020.1767018.
- Wasil, E., & Golden, B. (2003). Celebrating 25 years of AHP-based decision making. Computers & Operations Research, 10(30), 1419–1420. Jg., Nr. https://doi.org/10.1016/S0305-0548(02)00184-3.
- West, D. A. R. R. E. L. L. M. (2016). Moving forward: Self-driving vehicles in China, Europe, Japan, Korea, and the United States. Center for Technology Innovation at Brookings.https://d1wqtxts1xzle7.cloudfront.net/52649783/DriverlessCars-with-cover-page-v2.pdf?Expires=1640348891&Signature=G-P-Xt4k~21wzgOf1wMKQgKdERGoqsNznAtdTrQDp2oLsyULAy6y7-J1su7jVFOKP6REkEMPx8sod2SySDXbSFwZi0FkPDJWGYMxrhdqw-DXGq-LN4GooVUwUPlqa~o6xZMMYZg53tunj2EJIFA7x67SHqK2AJojhz5OThO-QKmUcpevrMgJAk7eEgyBPf~kQ9Lu1BzomMLw-WE4zaO25~AR-8w7ubWfnDo8U-m96XWS7eo~36yP04c01nu9VQ9lwilH~a5MDdaPSg7GcXW1piuondTqlIlBQxjYcEyv6yNcCzlknaXDZGy4bJ3ir1QEWUbiEO3DhRNYx52DfW45Mg__&Key-Pair-Id=APKAJLOHF5GGSLRBV4ZA
- Willis, G. O. R. D. O. N. B. (2004). Cognitive interviewing: A tool for improving questionnaire design. sage publications.
- Willis, G. O. R. D. O. N. B. (2015). Analysis of the cognitive interview in questionnaire design. Oxford University Press.
- Yap, J., Ho, C., & Ting, C. Analytic Hierarchy Process (AHP) for business site selection. In: AIP Conference Proceedings. Penang, Malaysia. AIP Publishing LLC, 2018. S. 020151.
- Yusof, S., & Aspinwall, E. (2000). Critical success factors in small and medium enterprises: Survey results. Total Quality Management, 11(4–6), 448–462. Jg., Nr. https://doi.org/10.1080/09544120050007760.
Appendix
Table A1. Consistency ratios of the 64 quantitative empirical studies
Table A2. Weighting of 15 CSFs of AD-TQM (total)
Table A3. Weighting of 15 CSFs of AD-TQM (German)
Table A4. Weighting of 15 CSFs of AD-TQM (Chinese)