Abstract
The spread of new coronavirus (COVID-19) infections shows no signs of stopping. Therefore, we must consider how to deal with this disaster well. The practice of social distance is one of the powerful tools for that purpose. Therefore, in this paper, we analyzed the factors that influence physical proximity in the manufacturing industry, which has a large impact on the economy given the scale of employment. As the method, first, exploratory factor analysis is performed using the US occupation information site O*NET information, and the extracted 7 variables, sitting work, work conditions, information processing, task significance, interdependence, response to aggression, and autonomy, are used in the regression analysis. As a result, it was shown that interdependence and response to aggression, which are categorized as “social characteristics”, and work conditions and sitting work, which are categorized as “context characteristics”, showed a positive correlation with physical proximity.
PUBLIC INTEREST STATEMENT
Social distancing is important to prevent manufacturers from being infected with COVID-19. This is the first study to show that social distancing is more difficult in a manufacturing site that is interdependent, exposed to human aggression and a bad environment, and has a lot of standing work. However, whether or not the work is involved in information processing or the work's significance and autonomy are high were not related to the ease of social distancing although these factors are generally thought to be related to social distancing. These indicate that social distancing in the manufacturing industry requires different strategies from other industries and discussions involving diverse persons related to the industry.
1. Introduction
The threat of the new coronavirus (COVID-19) shows no signs of fading. At the time of writing, on 7 April 2021, there are 132,423,013 infected people worldwide (Johns Hopkins University & Medicine, Citation2021). Therefore, it will be necessary to continue to take the social distancing that is currently recommended by the WHO in practice at the workplace (World Health Organization, Citation2020). As a result, researchers have been working to analyze the factors that influence the feasibility of social distancing and remote working (Crowley & Doran, Citation2020; Dingel & Neiman, Citation2020; Kokubun & Yamakawa, Citation2021b; Koren & Pető, Citation2020).
However, these studies are aimed at all the industries, and as far as the author knows, there are no studies limited to specific industries. If the way people work differs depending on the industry, it is considered that the factors of social distancing also differ between industries. Moreover, many of these studies are not in line with previous studies in the vocational field, except for Kokubun and Yamakawa (Citation2021b). There are several types of professions, depending on their characteristics (Morgeson & Humphrey, Citation2006), so the scientific view of vocational social distance relationships cannot ignore achievements in the relevant research field.
Therefore, the purpose of this study is to find the work characteristics that influence social distancing (physical proximity as a proxy variable) using data available on O*NET, an occupational information site.
2. Literature review and hypotheses
Research on the prevention of coronavirus infection and its effect is increasing (e.g., Kokubun et al., Citation2022Citation2022; Kokubun & Yamakawa, Citation2021a). Relatedly, several studies have analyzed the factors that influence social distancing (Crowley & Doran, Citation2020; Dingel & Neiman, Citation2020; Kokubun & Yamakawa, Citation2021b; Koren & Pető, Citation2020). Among them, Kokubun and Yamakawa (Citation2021b) investigated the relationship between work characteristics and social distancing using data available on O*NET, an occupational information site. After extracting eight factors (work conditions, supervisory work, information processing, response to aggression, specialization, autonomy, interaction outside the organization, and interdependence) by performing an exploratory factor analysis, they showed that interdependence, response to aggression, and interaction outside the organization, which are categorized as ”social characteristics,” and information processing and specialization, which are categorized as “knowledge characteristics,” were associated with physical proximity through the result of multiple regression analysis.
However, as far as the author knows, there is no analysis specialized for a particular industry. Given that people work differently in different industries, it will be necessary to analyze the factors that influence social distancing in each industry. Among them, the manufacturing industry is of great significance for its research given its large impact on the national economy. For instance, in 2018, the number of workers employed in the manufacturing industry was 12,689 thousand, accounting for 7.9% of the number of workers in all industries in the United States (U.S. Bureau of Labor Statistics, Citation2020). Therefore, in this study, following the procedure of Kokubun and Yamakawa (Citation2021b), some factors common to the occupations in the manufacturing industry were extracted by performing an exploratory factor analysis. We showed factors that determine physical proximity by performing a multiple regression analysis using the constructed independent variables.
According to Morgeson and Humphrey (Citation2006), there are four types of work design characteristics: social, knowledge, task, and contextual characteristics. Of these, social characteristics involve relationships with people (interdependence, social support, etc.), which are expected to require physical proximity because “physical proximity has a tremendous impact on the ability to work together” (Kiesler & Cummings, Citation2002, p. 57). In the area of the manufacturing industries, for instance, a previous study results revealed that face-to-face social networks facilitate knowledge sharing, problem-solving, learning, and teaching (Al Saifi et al., Citation2016). In the same vein, another previous study showed that team proximity in software development correlates with teamwork quality (Hoegl & Proserpio, Citation2004). Thus, the following hypothesis was derived:
Hypothesis 1 (H1). Interdependence (or another relationship-related interaction which needs proximity to others such as responding to aggression) of social characteristics are positively related to physical proximity.
On the other hand, contextual characteristics (sitting works, work conditions, etc.) did not correlate with physical proximity in the analysis of all industries in the previous work (Kokubun & Yamakawa, Citation2021b), but are considered to correlate with physical proximity in the manufacturing industry. For example, repairing a machine is a standing work and sometimes involves physically bad conditions such as heat and light, and at the same time, it is often performed consulting with the person who handles the machine at the site maintaining physical proximity with him. Thus, the following hypothesis was derived:
Hypothesis 2 (H2). Contextual characteristics are related to physical proximity.
3. Method
We conducted an exploratory factor analysis to extract the factors of work characteristics. Then, we conducted a multiple regression analysis to identify the work characteristics that determine physical proximity. For that purpose, we discuss the source and nature of data in this section.
3.1. Samples and data collection
We used the responses to two surveys included in release 24.3 of the database administered by O*NET (https://www.onetonline.org/, accessed on 8 August 2020, leased in May 2020. The accessed data were Work Context: https://www.onetonline.org/find/descriptor/browse/Work_Context/, accessed on 8 August 2020 and Work Activities: https://www.onetonline.org/find/descriptor/browse/Work_Activities/, accessed on 8 August 2020), a program sponsored by the US Department of Labor to investigate the association between work characteristics and physical proximity. The first survey is called the Work Context Questionnaire and includes questions about the “physical and social factors that influence the nature of work” such as interpersonal relationships, physical work conditions, and structural job characteristics. The second survey is called the Generalized Work Activities Questionnaire and includes questions about the “general types of job behaviors occurring on multiple jobs” such as the input of information, interaction with others, mental processes, and work output. We used all 98 items recorded under these questionnaires. Importance and level are recorded under Work Activities, but in this study, importance was used according to previous research for optimum clarity. All items take a number from 0 to 100, indicating frequency and importance. The data are classified by 968 occupations of various industries, but we use the data classified by 153 manufacturing-related occupations only in the current research.
3.2. Measures
Physical proximity used as a dependent variable in this study was selected from “I don’t work near other people (beyond 100 ft),” “I work with others but not closely (e.g., private office),” “Slightly close (e.g., shared office),” “Moderately close (at arm’s length),” and “Very close (nearly touching)” for the question “To what extent does this job require the worker to perform job tasks in close physical proximity to other people?” It was assigned a value from 0 to 100 when totaling. Details of other questions and options are available on O*NET; therefore, they are omitted in this paper. However, to give an example, “Work Context—Spend Time Standing,” is “How much does this job require standing?” The options are “Never,” “Less than half the time,” “About half the time,” “More than half the time,” and “Continually or almost continually,” with 0, 25, 50, 75, and 100 points assigned to each, to calculate the average value for each occupation.
3.3. Analytical method
We performed an exploratory factor analysis (EFA) to extract items to construct variables for the regression analysis. EFA is a statistical method used to uncover the underlying structure of a relatively large set of variables (Norris & Lecavalier, Citation2009). The criterion for factor extraction was an eigenvalue of 1 or more, and the factor load was calculated after performing varimax rotation using the main factor method. After that, items with a factor load of less than 0.4 and of 0.4 or higher on a plurality of factors were excluded, and factor analysis was performed again using the same criteria. This process was repeated until there were no items whose factor loads were less than 0.4 and 0.4 or higher on plural factors. Here, we followed the idea of Stevens (Citation1992), who suggests using a cut-off of 0.4, irrespective of sample size, for interpretative purposes. After establishing the factor structure, which is composed of sitting work, work conditions, information processing, task significance, interdependence,response to aggression, and autonomy, we performed the regression analysis using the variables comprising each factor as the independent variable, and physical proximity as the dependent variable to analyze the factors influencing social distancing.
4. Analysis and results
4.1. Exploratory factor analysis
As a result of repeating the factor analysis 8 times by the above method, eight factors consisting of 44 items were extracted. However, the reliability coefficient of the factor consisting of two items of “Work Context-Importance of Being Exact or Accurate” and “Work Context-Time Pressure” was 0.544. This value is lower than the standard of 0.7 (Cortina, Citation1993) that many researchers show, or 0.6 (Taber, Citation2018; Van Griethuijsen et al., Citation2015), which is a slightly looser standard. Therefore, it was determined that it would be difficult to use as a variable for analysis. Therefore, as a result of continuing the same processes after removing these items, 7 factors consisting of 40 items as shown in Table were extracted in the 10th factor analysis. Note that the sentences listed are not the question text, but the content of the question text. Based on the contents of the included items, the factors were named as sitting work, work conditions, information processing, significant task, interdependence, response to aggression, and autonomy. For these factor names, the terminology of Morgeson and Humphrey (Citation2006) was used except for the sitting work and response to aggression, which were named by the authors as there was a lack of corresponding factor names. Among the factors, interdependence and response to aggression were categorized as “social characteristics,” as per the classification by Morgeson and Humphrey (Citation2006). Likewise, information processing was categorized as “knowledge characteristics” following the same classification. Task significance and autonomy may be categorized as “task characteristics,” and sitting work and work conditions may be categorized as “contextual characteristics” (Morgeson & Humphrey, Citation2006). Physical proximity was not included in any of these variables because it loaded on multiple factors in the first factor analysis. The difference in the number of factors (7) and types of work design characteristics (4) is because the former corresponds to the subdivision of the latter (Morgeson & Humphrey, Citation2006). Previous studies covering the entire industry, including manufacturing, have also shown such differences in numbers (Kokubun & Yamakawa, Citation2021b).
Table 1. Results of exploratory factor analysis
Prior to the analysis, variables were created based on the results of the factor analysis. The value of the variable was a simple average of the items constituting each factor. However, the signs of the four items “Work Context—Spend Time Sitting”, “Work Context—Electronic Mail”, “Work Context—Telephone”, and “Work Context—Letters and Memos” were reversed so that they match the factor names.
Table is descriptive statistics. Three variables, work conditions (r = 0.296), interdependence (r = 0.393), and response to aggression (r = 0.309) showed a statistically significant positive correlation with physical proximity at the 1% level. However, the remaining sitting work, information processing, task significance, and autonomy did not show a significant correlation with physical proximity even at the 5% level. In the following multiple regression analysis, we used three industries, Manufacturing Production Process Development (MPPD, N = 26), Maintenance, Installation & Repair (MIR, N = 24), and Production & Quality Assurance (PQA, N = 103), as the control variables after converting them into dichotomous values. Using these variables, hierarchical multiple regression analysis was performed. That is, first (1) only these control variables were input, then (2) only the seven main variables were input, and finally (3) all these variables were input.
Table 2. Descriptive statistics
α: Cronbach’s alpha coefficient.
4.2. Multiple regression analysis
Table shows the results of multiple regression analysis by the stepwise method. The first column, Model 1, shows the result of inputting only the dichotomous variables of three industries. MIR (β = 0.219, p < 0.01) was selected as the statistically significant positively correlated variable, whereas MPPD and PQA were not selected. The second column, Model 2, is the result of inputting only 7 variables. Like the results of single correlation analysis, work conditions (β = 0.157, p < 0.05), interdependence (β = 0.302, p < 0.01), response to aggression (β = 0.187, p < 0.05) were statistically significant and positive. This result supports H1 and H2. Also, as a result of controlling other variables, a significant negative correlation was shown in sitting work (β = −0.200, p < 0.01). This result also supports H2. The third column, Model 3, shows the results of an analysis conducted by adding three industrial-type variables to seven variables. Here, three variables of MIR (β = 0.330, p < 0.01), sitting work (β = −0.265, p < 0.01), and interdependence (β = 0.492, p < 0.01) are significant positive correlations at 1% level. On the other hand, other variables including work conditions and response to aggression did not become significant at the 5% level. For reference, the adjusted R-squared values for all three models in Table are 0.04 (Model 1), 0.22 (Model 2), and 0.27 (Model 3) from the left to the right. They exceed 0.02 (small level), 0.13 (moderate level), and 0.26 (large level) by the Cohen’s criteria (Cohen, Citation1988). Furthermore, there are small to moderate differences between the values of models. Therefore, the mixed model in Model 3 is useful for predicting the feasibility of social distancing more accurately. Among the variables, we can also notice that interdependence has the largest coefficient indicating the largest association with the physical proximity.
Table 3. Results of multiple regression analysis with physical proximity as the dependent variable
MPPD = Manufacturing Production Process Development
MIR = Maintenance, Installation & Repair
PQA = Production & Quality Assurance
Figure shows the correlation between interdependence and physical proximity. The box in the lower left indicates that both interdependence and physical proximity values are 0.5 standard deviations lower than the average value. The box in the upper right indicates that both interdependence and physical proximity values are 0.5 standard deviations higher than the average value. Appendix A1 and A2 are extracted from the occupations in the lower left and upper right boxes for each variable. A1 (occupation with low interdependence and low physical proximity) includes repairers of electronic/camera/medical/musical equipment, timing device assemblers/adjusters, computer-controlled machine tool operators, machine setters/operators/tenders of extruding/drawing/cutting/punching/press/welding/soldering/brazing, settlers/operators/tenders of textile bleaching/dyeing/knitting/weaving machine, etc. A2 (occupation with high interdependence and high physical proximity) includes first-line supervisors of mechanics/installers/repairers and production/operating workers, meat cutters, food cooking machine operators/tenders, forging/rolling machine settlers/operators/tenders, model/pattern makers, system operators of a nuclear power reactor, power distribution, chemical plant, and petroleum pump, etc.
Figure 1. Scatter plot showing the relationship between interdependence and physical proximity.
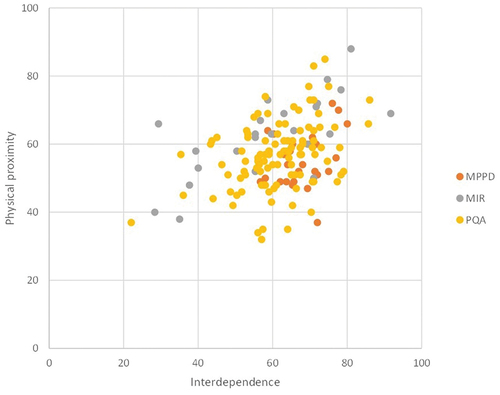
The former (A1) is characterized by the inclusion of repairers/assemblers/adjusters of some precision mechanical equipment and computer-controlled machine tool operators. It seems that interdependence and proximity are not required so much when working with craftsmanship, such as the repair or manufacturing of precision machinery, or when handling devices that are computer-controlled replacing human control. The latter (A2) is characterized by the inclusion of first-line supervisors and large-scale equipment system operators. It seems that interdependency and proximity are much required when dealing with people and huge devices. It is interesting to note that machine settlers/operators/tenders are included in both groups, even though they are of different types. This means that even when working with machines, there is a large difference in the degree to which interdependence and physical proximity are required.
5. Discussion
The purpose of this paper was to find variables that correlate with physical proximity at the manufacturing site based on an exploratory method. The scales extracted as a result of the factor analysis were sitting work, work conditions, information processing, task significance, interdependence, response to aggression, and autonomy. Of these, as a result of multiple regression analysis, context characteristics (work conditions, sitting work) and social characteristics (interdependence and response to aggression) were shown to have a significant correlation with physical proximity.
Furthermore, even in a model in which 7 main variables and 3 industry variables were input at the same time, interdependence showed a positive correlation and sitting work showed a negative correlation. By occupation, MIR (Maintenance, Installation & Repair) showed a statistically significant positive correlation with physical proximity, while PQA (Production & Quality Assurance) and HSD (Honestly Significant Difference) did not show a significant correlation. This means that the practice of social distancing is difficult for jobs that require interdependence, standing works, and jobs for Maintenance, Installation & Repair, etc.
Conversely, information processing (or knowledge characteristics) was not related to physical proximity. This job is in many cases considered to be the one that does not require physical contact with people (e.g., Althoff et al., Citation2020; Hatayama et al., Citation2020). Indeed, in the previous research, knowledge characteristics-related variables were inversely associated with physical proximity (Kokubun & Yamakawa, Citation2021b), because knowledge works normally does not require interaction with people. However, this may not always be true in the manufacturing industry. In the manufacturing field, a close relationship between developers or between developers and production workers is often required. Physical proximity would not be required so much if it is a one-step relationship in which the production method is converted into digital data and sent to the factory, and the production site supervisor who receives it informs the production workers to carry out the production. However, since the manufacturing industry is often based on two-way information exchange, knowledge characteristics do not always reduce the need for physical proximity, unlike the average tendency of all the industries shown by Kokubun and Yamakawa (Citation2021b). Therefore, whether knowledge characteristics require physical proximity depends on what kind of information is being exchanged, and it is considered that the manufacturing industry did not show a consistent tendency.
Similarly, task significance and autonomy of task characteristics were not related to physical proximity. Jobs that affect other members or require autonomy are mainly of management jobs and do not always require close cooperation with the field side. However, it is often pointed out that it is easier to exert influence and to perform creative work by engaging with the site face-to-face. For instance, it is suggested in a previous study using a sample of 53 innovation teams that the transformational leadership is not very effective in improving the performance of geographically highly distributed teams (Eisenberg et al., Citation2019). Therefore, it cannot be said unconditionally whether task characteristics seeks physical proximity.
To sum, context characteristics (work conditions, sitting work) and social characteristics (interdependence and response to aggression) were shown to have a significant correlation with physical proximity. On the contrary, knowledge characteristics (information processing) and task characteristics (task significance and autonomy) were not related to physical proximity.
6. Implication
In this paper, we analyzed the factors that influence physical proximity in the manufacturing industry by creating variables based on exploratory factor analysis and multivariate analysis using the information on questionnaire results recorded in O*NET, a job information website in the United States. As a result, it was shown that social characteristics (interdependence and response to aggression) and context characteristics (sitting work and work conditions) showed a significant correlation with physical proximity. Also, the results showed that the job of Maintenance, Installation & Repair also requires physical proximity. Social characteristics has been found indispensable to the innovation (e.g., Montes et al., Citation2005) and employee commitment (e.g., Kokubun, Citation2018) of the manufacturing industry, and it is unlikely that this fact will change rapidly. However, under the COVID-19 pandemic, it is required to secure social distancing as much as possible and continue production activities while preventing the spread of infection. For the occupations that require interdependence, the factor most significantly associated with physical proximity, and high physical proximity, as listed in Appendix Table A2, there is room to consider whether there is a method to secure social distancing by changing the way of working while implementing infection protection measures. Besides, the required strength and contents of interdependence are considered to vary greatly depending on the workplace within the same industry. Efforts to devise ways to secure social distancing, such as replacing existing face-to-face teamwork with virtual ones, are worth the effort. For example, some studies show that increasing employee trust affects virtual team performance more than face-to-face team performance (Breuer et al., Citation2016; Ford et al., Citation2017).
In this study, we identified variables that affect social distancing only in the manufacturing industry. In Kokubun and Yamakawa (Citation2021b), which targets all industries, social characteristics and knowledge characteristics were factors that influence social distancing. On the other hand, in this study, social and context characteristics were factors that influence social distancing. Therefore, social characteristics, including the relationships between members, can be said to be an important factor that influences social distancing regardless of whether it is a manufacturing industry or not. On the other hand, knowledge characteristics such as information processing generally have the effect of enabling social distancing, but does not have such an effect in the manufacturing industry. This is thought to be due to the difference in the quality of information. Information such as improvements in production methods used in the manufacturing industry often requires interaction with the persons at the field, so just because a person is engaged in information processing work does not mean that it is easy to take social distancing. On the other hand, context characteristics such as work conditions are generally not factors that influence social distancing, but they are factors that influence in the manufacturing industry. In the manufacturing industry, it means that people who are standing work or who are in a harsh environment regarding temperature, heat, etc. tend to require physical proximity between people. It has also been shown that MIR, in which such an environment is strongly observed, has a higher physical proximity than other MPPD and HSD.
7. Limitation
This paper exploratively extracted the factors that are the variables used in regression analysis, using the average values by the occupation of the attitude survey data recorded in the US occupation information site, O*NET. Therefore, if the primary data before being aggregated by occupation can be obtained and the results of this paper can be verified, its significance will be great. Besides, Physical Proximity used as the dependent variable of the analysis is a variable based on the questionnaire survey results and may differ from the actual proximity. It is also significant to verify the analytical model in this paper after measuring the actual proximity using GPS location information, etc.
8. Conclusion
The spread of new coronavirus (COVID-19) infections shows no signs of stopping. The practice of social distancing is one of the powerful tools for preventing that disaster. In previous research, analysis of the factors that influence social distancing has also been carried out using information from the US occupation information site O*NET. However, they targeted all industries, not specific industries. Therefore, in this paper, we analyzed the factors that influence Physical Proximity in the manufacturing industry, which has a large impact on the economy given the scale of employment. As the method, first, exploratory factor analysis is performed using O*NET information, and the extracted 7 variables, sitting work, work conditions, information processing, task significance, interaction, response to aggression, and autonomy, are used in the regression analysis. As a result, it was shown that interdependence, work conditions, and response to aggression showed a positive correlation and sitting work showed a negative correlation with physical proximity. By job type, maintenance, installation & repair tended to have higher physical proximity than manufacturing production process development, production & quality assurance.
Acknowledgement
An earlier version of this treatise is published on the following preprint server: https://doi.org/10.1101/2020.08.22.20179994 (accessed on 25 August 2020).
Disclosure statement
No potential conflict of interest was reported by the author(s).
Additional information
Funding
References
- Al Saifi, S. A., Dillon, S., & McQueen, R. (2016). The relationship between face to face social networks and knowledge sharing: An exploratory study of manufacturing firms. Journal of Knowledge Management, 20(2), 308–17. https://doi.org/10.1108/JKM-07-2015-0251
- Althoff, L., Eckert, F., Ganapati, S., & Walsh, C. (2020). The city paradox: Skilled services and remote work. CESifo Working Paper; CESifo: Munich, Germany. (accessed on 8 August 2020). https://ssrn.com/abstract=3744597.
- Breuer, C., Hüffmeier, J., & Hertel, G. (2016). Does trust matter more in virtual teams? A meta-analysis of trust and team effectiveness considering virtuality and documentation as moderators. Journal of Applied Psychology, 101(8), 1151–1177. https://doi.org/10.1037/apl0000113
- Cohen, J. (1988). Statistical power analysis for the behavioral sciences (2nd ed.). Routledge.
- Cortina, J. M. (1993). What is coefficient alpha? An examination of theory and applications. Journal of Applied Psychology, 78(1), 98–104. https://doi.org/10.1037/0021-9010.78.1.98
- Crowley, F., & Doran, J. (2020). COVID-19, occupational social distancing and remote working potential: An occupation, sector and regional perspective. Regional Science Policy & Practice, 12(6), 1211–1234. https://doi.org/10.1111/rsp3.12347
- Dingel, J. I., & Neiman, B. (2020). How many jobs can be done at home? Journal of Public Economics, 189, 104235. https://doi.org/10.3386/w26948
- Eisenberg, J., Post, C., & DiTomaso, N. (2019). Team dispersion and performance: The role of team communication and transformational leadership. Small Group Research, 50(3), 348–380. https://doi.org/10.1177/1046496419827376
- Ford, R. C., Piccolo, R. F., & Ford, L. R. (2017). Strategies for building effective virtual teams: Trust is key. Business Horizons, 60(1), 25–34. https://doi.org/10.1016/j.bushor.2016.08.009
- Hatayama, M., Viollaz, M., & Winkler, H. (2020). Jobs’ amenability to working from home: Evidence from skills surveys for 53 countries. World Bank Policy Research Working Paper, 9241. https://ssrn.com/abstract=3599548.
- Hoegl, M., & Proserpio, L. (2004). Team member proximity and teamwork in innovative projects. Research Policy, 33(8), 1153–1165. https://doi.org/10.1016/j.respol.2004.06.005
- Johns Hopkins University & Medicine (2021). COVID-19 Dashboard. https://coronavirus.jhu.edu/map.html (accessed on 7 April 2021)
- Kiesler, S., & Cummings, J. N. (2002). What do we know about proximity and distance in work groups? A legacy of research. Distributed work. In P. J. Hinds & S. Kiesler (Eds.), Distributed work (pp. 57–80). MIT Press.
- Kokubun, K. (2018). Education, organizational commitment, and rewards within Japanese manufacturing companies in China. Employee Relations, 40(3), 458–485. https://doi.org/10.1108/ER-12-2016-0246
- Kokubun, K., & Yamakawa, Y. (2021a). Social capital mediates the relationship between social distancing and COVID-19 prevalence in Japan. INQUIRY: The Journal of Health Care Organization, Provision, and Financing, 58, 00469580211005189. https://doi.org/10.1177/00469580211005189
- Kokubun, K., & Yamakawa, Y. (2021b). The impact of work characteristics on social distancing: Implications at the time of COVID-19. International Journal of Environmental Research and Public Health, 18(10), 5074. https://doi.org/10.3390/ijerph18105074
- Kokubun, K., Ino, Y., & Ishimura, K. (2022). Social and psychological resources moderate the relation between anxiety, fatigue, compliance and turnover intention during the COVID-19 pandemic. International Journal of Workplace Health Management, 15(3), 262–286. https://doi.org/10.1108/IJWHM-07-2021-0142
- Koren, M., & Pető, R. (2020). Business disruptions from social distancing. PLoS ONE, 15(9), e0239113. https://doi.org/10.1371/journal.pone.0239113
- Montes, F. J. L., Moreno, A. R., & Morales, V. G. (2005). Influence of support leadership and teamwork cohesion on organizational learning, innovation and performance: An empirical examination. Technovation, 25(10), 1159–1172. https://doi.org/10.1016/j.technovation.2004.05.002
- Morgeson, F. P., & Humphrey, S. E. (2006). The Work Design Questionnaire (WDQ): Developing and validating a comprehensive measure for assessing job design and the nature of work. Journal of Applied Psychology, 91(6), 1321–1339. https://doi.org/10.1037/0021-9010.91.6.1321
- Norris, M., & Lecavalier, L. (2009). Evaluating the use of exploratory factor analysis in developmental disability psychological research. Journal of Autism and Developmental Disorders, 40(1), 8–20. https://doi.org/10.1007/s10803-009-0816-2
- Stevens, J. P. (1992). Applied multivariate statistics for the social sciences (2nd edition) ed.). Erlbaum.
- Taber, K. S. (2018). The use of Cronbach’s alpha when developing and reporting research instruments in science education. Research in Science Education, 48(6), 1273–1296. https://doi.org/10.1007/s11165-016-9602-2
- U.S. Bureau of Labor Statistics (2020) Employment by major industry sector. (Last Modified Date: September 4, 2019). Accessed 20 Aug 2020 https://www.bls.gov/emp/tables/employment-by-major-industry-sector.htm
- van Griethuijsen, R. A., van Eijck, M. W., Haste, H., den Brok, P. J., Skinner, N. C., Mansour, N., Gencer, A. S., & BouJaoude, S. (2015). Global patterns in students’ views of science and interest in science. Research in Science Education, 45(4), 581–603. https://doi.org/10.1007/s11165-014-9438-6
- World Health Organization (2020) Coronavirus disease (COVID-19) advice for the public. Accessed 20 Aug 2020. https://www.who.int/emergencies/diseases/novel-coronavirus-2019/advice-for-public
Appendix
A1 Occupation with low Interdependence and low Physical Proximity
A2 Occupation with high Interdependence and high Physical Proximity