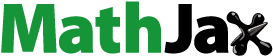
Abstract
Determinants of international capital inflow differ from one country to another, and as such country-specific studies are important in assisting each economy to understand cutting-edge policies which can be implemented to attain and retain desired capital inflow. The purpose of the present empirical effort is to examine the determinants of international capital inflow into the South African economy with special focus on the role of global uncertainty. The study investigated the determinants of portfolio inflow, direct capital inflow and total inflow using time series quarterly data from 1960(Q1) to 2021(Q3). The study employed the Dynamic Conditional Correlation (DCC GARCH) to investigate time-varying correlation among the variables in the light of more current data and Bayesian Linear Regression Model given its ability to deal with the problem of volatility. The overall picture that emerges from the study is that global uncertainty does not significantly correlate or affect portfolio inflow; while on the other hand, it negatively correlates and impacts direct capital inflow and total inflow. The implication is that government and South African policymakers should pay a closer attention to measures to mitigate global uncertainties in attempt to increase direct capital investment flow.
1. Introduction
The importance of international capital inflow in promoting and accelerating long-term growth has been emphasized and underscored by several theoretical and evidence-based studies (Adams, Citation2009; Khobai & Mavikela, Citation2018; Tiwari & Mutascu, Citation2011). Foreign capital inflow contributes to economic growth of the host nations through bridging the capital and resources inadequacies of the host nations, promotion of investments in productive and human capital, capacity improvements of the host and perhaps improved technology transfer. International capital inflow also contributes to widening and deepening of the financial market and enhancements of liquidity (Adams et al., Citation2017; Anetor, Citation2020). Meanwhile, excess and volatile capital inflows may also create distortions in economic activities and policy hiccups. For instance, excess capital inflows may hamper the intermediation process of the domestic financial systems and as such likely lead to undue credit creation that could create financial instability risks. Second, excessive capital inflows could cause currencies to appreciate that has a negative implication for export and growth performance. Notwithstanding, given the role and the importance of moderate international capital inflow in the development process, the question then is what determines the flow international capital flight in the domestic economy or what factors enhances the international flows of capital, especially with sub-Saharan Africa receiving a smaller proportion of the increased global flows. This question has occupied empirical finance-studies space consequent upon the pivotal roles of international foreign capital inflow in development attainments.
Studies have confirmed the role of external conditions in the financial markets as they argued that external economic conditions are important in determining the pattern of capital inflows to emerging and developing economies (Calvo et al., Citation1993; Fernandez-Arias, Citation1996). For instance, empirical literature argues that short term interest rates change by the apex banks could play an important role in triggering of capital inflows and outflows to emerging and developing economies through their impact on the interest rates spreads (Canova, Citation2005; Maćkowiak, Citation2007). Similarly, Vidal and Correa (Citation2007) confirmed the role of economic conditions in attracting capital inflow as they submit that both favorable political and economic environment are more likely to play prominent role in capital inflow. This is because economic conditions and factors are capable enough to impact the probable future returns from investments in the host countries Also, economic shocks such as insecurity, Covid-19 pandemic among others could orchestrate economic uncertainties and as such capable of affecting international capital inflows (Dornean et al., Citation2012; Ucal et al., Citation2010). Also, financial market deregulations, liberalization of capital market transaction and equity markets have been suggested as policy incentives to induce more international capital flows (Campion & Neumann, Citation2004; Caprio et al., Citation2007; Kaminsky & Schmukler, Citation2003; Prasad et al., Citation2006). Alfaro et al. (Citation2008) underscores the importance of internal structural characteristics such as financial openness, human capital or institutions in attracting capital inflow.
There is no agreement in the literature on the determinants of capital inflow as the extant studies do not favor any determinant playing a significant role over others. Determinants of international capital inflow vary from one country to another, and as such country-specific studies are important in helping each economy to understand cutting-edge policies to adopt to attain and retain desired capital inflow. For instance, Byrne and Fiess (Citation2011) argue that interest rates are an important determinant in United States. On the other hand, Forbes and Warnock (Citation2012) could not establish the significance of global interest rates or global liquidity international capital inflow in both advanced and emerging economies However, like some other studies, global risk aversion is found to be a significant and robust factor. Athari et al. (Citation2020) investigate the impact of global competitiveness on international capital inflow and find that competitiveness has a positive and statistically significant impact on international capital inflows. Huang (Citation2010) establishes very strong evidence linking the exchange rate to the inflow of capital, and while interest rates differential is also significantly linked to inflow of capital. Statistics show that South Africa has low level of savings coupled with high levels of poverty and inequality (SARB, Citation2022). Capital inflows are argued to be one of the ways to achieve high rates of growth given that they bridge the gap between demand and supply of loanable funds.
The global uncertainty is often caused by the external factors not envisaged, and generally has negative and undesirable effects on the various macroeconomic fundamentals. The most recent of such is COVID-19 outbreak, which led to a complete shutdown of economic activities in most nations of the world with South Africa inclusive. Apart from the negative implication of the recent global uncertainty as occasioned by Covid-19 pandemic on the health of people world-wide, the economic and financial consequences cannot be quantified as they are too enormous to recount. For instance, GDP contracted globally by 6% globally, while trade and FDI also fell significantly. Capital inflows across countries was expected to fall by 40%. In most of the cases, global uncertainty is often accompanied by unstable oil prices and exchange rates depreciation. For most of the emerging economies such as Brazil, South Africa among others, the currency depreciation greatly deepened between end-February and mid/end-March 2020. For instance, Ahiadorme (Citation2021) argued that global uncertainty significantly affects the macro-financial performance in emerging markets. Similarly, Bhattarai et al. (Citation2020) found that global uncertainty affects stock prices and exchange rates and significantly leads to capital outflows. This position is corroborated by Forbes and Warnock (Citation2012) and Passari & Rey (Citation2015). They argued that extreme fluctuation in capital flows is largely driven by global uncertainty. Hlaing and Kakinaka (Citation2019) submitted that global uncertainty worsens the unexpected reduction of portfolio investment in both advanced and developing economies. It is, however, found to increase the foreign direct investment in developed economies.
Studies on determinants of capital inflow have not attracted desired empirical efforts in Africa, and more importantly in South African economy especially in recent time. Among the available studies on the determinants of capital inflow are Fedderke and Liu (Citation2002) and Ahiadorme (Citation2021) among others. Fedderke and Liu (Citation2002) conclude that stable and transparent policy environment are significant in attracting foreign capital inflow. Similarly, Brafu-Insaidoo and Biekpe (Citation2014) examine the determinants of foreign capital flows in some of selected sub-Saharan African countries and found that liberalization of the domestic financial system and the domestic equity market exerts a positive and significant impact on international capital flows.
This study differs from Ahiadorme (Citation2021) as the current study specifically examines the role of global uncertainty on capital inflow while Ahiadorme (Citation2021) examined the role global uncertainty on macroeconomic aggregates such as inflation, interest rate, and credit allocated to private sector.
The current study contributes to the available work by examining the determinants of capital inflow utilizing Dynamic Conditional Correlation (DCC GARCH) to investigate time-varying correlation among the variables in the light of more current data and Bayesian Linear Regression Model. The time-varying correlation approach is superior to pairwise correlation coefficients which is a static correlation coefficient. The pairwise correlation coefficients are static as they could not account for the correlation changes which occur over time. It only presents the contemporaneous or instant relationship over a period while DCC approach enables us to see the changes in how variables co-move over time. This current empirical work confirms the popular position in the literature that global uncertainty significantly hampers capital inflows. The remaining discussion is as follows: review of empirical literature is contained in section 2, while section 3 discusses the empirical strategy. Section 4 contains the empirical results and concluding remark is contained in section 5.
2. Brief review of literature
Capital inflow drivers have long been a subject of discussion by both theoretical and empirical studies given the place of capital inflow in development process. Several factors such as macroeconomic variables performance and market size have been often considered when investigating determinants of FDI inflows (Noorbakhsh et al., Citation2001; Shah & Faiz, Citation2015). Byrne and Fiess (Citation2016) investigate the determinant of aggregate and disaggregate capital inflows in emerging market economies their studies found that long-run bond yields and commodity prices are important determinants of global capital flows. This is slightly consistent with Reinhart and Reinhart (Citation2009). Reinhart and Reinhart (Citation2009) conclude that economic growth, short-run interest rates and commodity prices are important determinants of capital inflow. However, global uncertainty and risks were identified as the major determinant of capital inflow by Forbes and Warnock (Citation2012). They argued that global factors, most especially global risk factors are a major driver of gross capital inflows. The VIX index was used to proxy global economic uncertainty. The findings conclude that increased global uncertainty suppress investments due to potential irreversibility. Similarly, quality of quality of institution prevailing in an economy has also being strongly linked to the capital inflow. Naudé and Krugell (Citation2007) submit that the quality of institution in form of stability of political system was found to be a significant capital inflow determinant in Africa. Their findings also corroborated Forbes and Warnock (Citation2012) as they also found global economic risks to be an important driver of capital inflow. This is consistent with Allen and Giovannetti (Citation2011). They specifically argued that several investment projects in countries like Democratic Republic of Cong, Zambia and were put on hold or cancelled during the global economic crisis. This evidence of importance of global economic uncertainty as a driver of capital inflow was rejected by Méon and Sekkat (Citation2012). They argued that capital flows are insensitive to global political risk or uncertainty. Most especially if the inflow volume is larger. By implication, they conclude that there and a positive correlation between global risk-taking and capital inflow.
Forbes and Warnock (Citation2012) and Sarno et al. (Citation2016) classified the determinants of capital inflow broadly into two which are push and pull factors. Accordingly, push factors are factors that drive capital flows from developed to developing countries such as global rate and this is generally measured by interest rate differential, global risk aversion and portfolio diversification among others. On the other hand, pull factors are internal economic factors that drive capital into a country and these are internal growth rate, exchange rate behaviour, debt-GDP ratio and trade openness among others (Sarno et al., Citation2016). On the other hand, Broner and Rigobon (Citation2004) argued that macroeconomic factors provided little explanations on the movement of capital inflow. In contrast Alfaro et al. (Citation2007) submit that the prevailing macroeconomic factors in conjunction with quality of institution are important determinants of capital flow volatility.
Using panel data from 1973 to 2000, Neumann et al. (Citation2009) conclude that different capital flow types responded differently to financial liberalization, with direct capital inflows showing significant increases in volatility for emerging markets. Broto et al. (Citation2011) examined the determinants of direct capital inflows, portfolio inflows and other debt inflows to emerging economies. Their findings show that developing countries could actually do little or nothing in driving capital inflow. In the same vein, Mercado and Park (Citation2011) employed a panel GMM estimator to investigate the determinants of FDI inflows, portfolio inflows among others to developing Asian economies. Their results show that quality of institution, trade openness, financial openness, and stock market capitalization are significant drivers of capital inflow.
In conclusion, within the empirical literature, most of the existing studies have been carried out within the panel framework. The findings from such analysis may not truly reflect the actual determinants of international capital inflow in each economy due to several differences between the countries. The current study exclusively focused on South African economy.
3. Empirical strategy
3.1. Data description and summary
Quarterly time series data were sourced from the South African Reserve Bank (SARB) and Federal Reserve Economic Data (FRED). More specifically, data on portfolio inflow (PI), direct capital inflow (DCI) and total inflow were obtained from SARB while data on gross domestic product, debt-output ratio, world uncertainty index, long-term government yield, interest rate differential and exchange rate from 1960(Q1) to 2021(Q3) were obtained from FRED. Interest rate differential is calculated as the difference between the South African interest and global interest rate. The global interest rate is proxied by United States interest rate. Interest differential is believed to be among major determinants of foreign inflow. Theoretically, potential investor looks at the difference between the interest rate prevailing in investment destination and United States interest rate. The study aims at examining the time-varying correlation among the variables using dynamic conditional correlation (DCC GARCH model) and Bayesian Linear Regression Model (BLR). The independent variables in the model are gross domestic product, debt-output ratio, world uncertainty index, long-term government yield, interest rate differential and exchange rate. While the dependent variables are portfolio inflow (PI), direct capital inflow (DCI) and total inflow (TI). Consequently, different estimation is done for each of the dependent variable against the independent variables. This is to enable us see the impacts of the independent variables on each inflow.
3.2. GARCH-DCC model
The GARCH-DCC is a multivariate model also known as Dynamic Conditional Correlation (DCC) model. The DCC model is adopted to examine the time varying correlations as against static correlation. The specified GARCH(p,q) model is estimated using Maximum Likelihood Estimation (MLE) techniques. It is represented by the subsequent equations where is a residual from fitted VAR equation.
is already standardised disturbance term as a result of mean removal from the VAR residual series. The log of volatility of is given as a function of its own lagged values and lagged standardised disturbance terms. s are the persistence of volatility and
s represent the GARCH effects. The standardized residual from the VAR equations is re-standardised. The following variant of DCC is used for estimation in R statistical package:
where is the Dynamic Conditional Correlation,
is the standardized residuals from GARCH’s.
,
are the persistence of correlation,
is the initial correlation matrix at
i.e .
. The obtained correlations would shed light on the time-varying contemporaneous relationships amongst the variables.
3.3. Bayesian linear regression model
The empirical approach employed in the study is Bayesian Linear Regression (BLR) model. The general multiple linear regression model can be written as:
where is a column matrix of the dependent variables which are portfolio inflow, direct capital inflow, and total inflow.
is a vector of independent variable which include the size of the economy proxied by GDP, debt-GDP ratio, global uncertainty proxied by global uncertainty index, exchange rate, long-term government bond yield and interest rate differential.
is a vector of regression model parameters
is a column vector of error terms
Bayesian linear regression obtains parameter estimation by means of prior, likelihood distribution and posterior distribution. Estimation of parameters is done through posterior distribution which is used to multiply both prior distribution and likelihood distribution. Linear regression model assumes error terms are normally distributed, and as such variables are assumed to be normally distributed. In Bayesian approach, probability density function of the variables can be stated as follows:
The likelihood function of the variables can be stated as follows:
Bayesian approach to regression analysis makes use of several prior distributions. Parameters estimation using Bayesian approach can be executed through iteration of the marginal posterior. Posterior distribution is obtained by multiplying both prior distribution and likelihood function.
The study makes use of MCMC (Markov Chain Monte Carlo) algorithm to obtain regression model parameters. Gibbs Sampling method of algorithms in MCMC is adopted. MCMC pack available in R statistical package.
4. Empirical results
4.1. Preliminary analysis
All the variables were expressed in their logarithmic forms. Table contains summary properties of the variables. The means and the standard deviations of all the variables are not close to zeros. This shows that the variables exhibit some degree of volatility as suggested by standard deviations. This further explains the adoption of DCC GARCH model to obtain time-varying correlation coefficients. Another important deduction from the descriptive statistics is that the null hypothesis of the Jacque Berra statistic is rejected for all the variables, signifying that all the variables are not normally distributed. This perhaps further justifies the use of Bayesian Linear Regression model as Bayesian approach to parameter estimation does not rely on the assumption of normality.
Table 1. Summary statistics of the variables
Table shows the pair wise correlation coefficients otherwise known as static correlation coefficient. Confirming the theoretical expectation, the correlation coefficients between each measure of inflow in the economy and the determinants are high with the exceptions of world uncertainty index and debt as a ratio of GDP. Nonetheless, these coefficients are somewhat static as they do not account for the correlation changes which take place over time. They only show the contemporaneous or instant relationship over a period of time. The study hence utilizes Dynamic Conditional Correlation (DCC GARCH) to investigate time-varying correlation among the variables.
Table 2. Correlation matrix
Table shows the results of time series properties of the series with and without trend using ADF and PP. We found the variables not to be stationary in their level form, but became stationary after differencing once. Variables in their differenced form were fitted into our models
Table 3. Time series properties
4.2. DCC GARCH results
All the variables are expressed in logarithmic form. The variables in their differenced form are then fitted to a DCC GARCH model. DCC with multivariate Skew Student-t Distribution (sstd) and DCC with multivariate Student-t Distribution (std) were modelled. DCC with multivariate Student-t Distribution yielded a lower Akaike Information Criteria, and was fitted for each measure of inflow into the economy; Portfolio Inflow (PI), Direct Capital Inflow (DCI) and total inflow which is the sum of both portfolio inflow and direct capital inflow.
The time-varying correlations between the portfolio inflow and the determinants are presented in in Figure . As displayed in Figure , the dynamic correlation between portfolio inflow and GDP was around 0.4 in 1960 and subsequently began to decline, almost reaching zero in early 70s but slightly went up hereafter to around 0.2 and became stable around 0.2 before it began to pick up in early 2020s going as far as 0.8. The dynamic conditional correlations between portfolio inflow and debt as a ratio of GDP are entirely in negative region with highest coefficient being very close to −0.9 around early 2020 and lowest correlation around −0.4 early 1960. On the average, the dynamic conditional correlation between the portfolio inflow and debt-GDP ratio during the study period is negative. This suggests that both portfolio inflow and debt-GDP ratio move in opposite direction.
The dynamic correlation coefficients between portfolio inflow and global economic shock proxied by world uncertainty index are entirely weak throughout the period under consideration, with coefficients being less than 0.05 for majority of the period under consideration. This suggests that portfolio inflow into South African economy and global economic uncertainty does not necessarily move in the opposite direction. In other words, portfolio inflow is largely influenced by endogenous factors prevailing in the South African economy. Also, the dynamic correlation coefficients between portfolio inflow and long-term government bond yield is also observed to be negative over the study period. However dynamic condition coefficients are quite low with the coefficients oscillating between −0.1 and −0.25. The implication is that larger composition of the foreign portfolio will probably be equity. Also, the dynamic conditional correlation coefficients between portfolio investment and interest rate differential are largely positive ranging between 0.1 and 0.4. The relationship is however not as strong as theoretically expected. The directions of relationship between the portfolio investment and the exchange rate are largely positive. This implies that increase in exchange rate which implies a depreciation of South African will probably attract foreign portfolio because foreign portfolio will become much cheaper since more units of foreign portfolio can be bought with the same amount of US dollar.
The time-varying correlations between the direct inflow and the determinants are presented in in Figure . As shown in Figure , the dynamic correlation between direct inflow and GDP was roughly the same with that of portfolio inflow and GDP. As observed under portfolio inflow, the dynamic conditional correlation coefficient was around 0.4 in 1960 and later began to decline, almost reaching zero in early 70s but slightly went up hereafter to around 0.2. It began to pick up in early 2020s reaching as far as 0.8. The dynamic conditional correlations between direct inflow and debt as a ratio of GDP are also entirely in negative region with highest coefficient being very close to −0.5 around early 2020 and lowest correlation being −0.01 around early 1960s. This shows that both direct inflow and debt-GDP ratio move in opposite direction under the study period. The dynamic correlation coefficients between direct inflow and world economic shock proxied by world uncertainty index are largely negative though not as strong as one would imagine. The overall picture is direct inflow seems to negatively affected by global uncertainty index. This suggests that direct inflow into South African economy, which are the real investments in factories, capital goods, land, and inventories and global economic uncertainty move in the opposite direction. Also, the dynamic correlation coefficients between direct investment and long-term government bond yield are seen to be oscillating between −0.05 and −0.25. This is not theoretically unexpected because an increase in bond yield will reduce direct inflow but however boost the portfolio inflow in the economy. Also, the dynamic conditional correlation coefficients between direct investment and interest rate differential are almost the same with the direction of relationship between portfolio investment and interest rate differential with coefficients oscillating between 0.1 and 0.4. The dynamic conditional correlation coefficients between the direct investment and the exchange rate are found to be very stable and positive. Unlike the relationships between portfolio investment and exchange rate that exhibit high volatile positive coefficients, the relationships between direct investment and exchange rate exhibit more stable positive coefficients. The implication is that direct investment into South Africa economy is not as sensitive to exchange rate movement as portfolio inflow is.
Figure 2. Time varying correlation coefficients between direct investment inflow and its determinants.
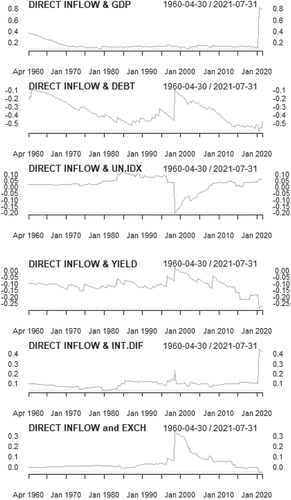
The dynamic conditional correlation coefficients between total inflow and the selected drivers are plotted in Figure . Similar patterns and behaviour movements are observed as in the case of time-varying correlations between the direct investment inflow and the determinants. This is not entirely unexpected as the direct investment inflow constitutes the greater proportion of total inflow into the South African economy.
4.3. BLR Results
The Gibbs Sampling algorithm approach using Markov Chain Monte Carlo (MCMC) method is employed in the Bayesian estimation process in order to obtaining the posterior distribution. Iteration used as many as 10000 with Burn in at 500 and thin of 1. Table shows the BLR results of the drivers of portfolio inflow in South African economy, with posterior distributions plot in Figure . From the results in Table , the effects of debt, world uncertainty index and yield are negative on portfolio inflow into the economy. However, the effect of world uncertainty index is approximately zero which confirms the DCC results that portfolio inflow is not driven by the global external shock but rather inherent macroeconomic stability. Based on the observed data, we see that there is a 95% likelihood that the portfolio inflow will reduce by 0.09 to 0.016 with one additional unit increment of debt as a percentage of GDP. GDP, interest rate differential and exchange rate depreciation are found to be positive drivers of portfolio inflow into South African economy. This is consistent with DCC estimation results.
Table 4. BLR results with portfolio inflow as dependent variable
Table shows the BLR results of the drivers of direct inflow in South African economy, with posterior distributions plot in Figure . From the Table , among the observed variables, only GDP and interest rate differential are found to be positive drivers of direct investment inflow into the South African economy. Based on the observed data, we believe there is a 95% possibility that the direct investment inflow will increase by 1.56 to 4.36 with additional growth rate in the economy, while it will increase by 0.02 to 0.06 with an increased positive interest rate differential. Long-term bond yield is observed to have a negative impact on direct investment inflow. In other words, increase in return on bonds, other things being equal, will motivate potential investors to invest in financial instruments such as bonds, and increase in exchange rate i.e. depreciation of Rand is found to be a negative driver of inflow. This is not entirely unexpected as most of the equipment and machinery that would be needed for real investment might need to be imported from much more developed economies. Also, unlike portfolio inflow, world uncertainty index is found to be an important negative driver of direct investment inflow. Based on the observed data, we believe there is a 95% chance that the direct investment inflow will reduce by 0.24 to 0.26 with increased uncertainty. While debt as ratio of GDP is also found to be a negative driver.
Table 5. BLR results with direct investment inflow as dependent variable
Table shows the BLR results of the drivers of total inflow in South African economy, with posterior distributions plot in Figure . From the Table , only GDP and interest rate differential are found to be significant positive drivers of total inflow into the South African economy. This is similar to the case of direct investment inflow. This uniform pattern of empirical findings is consistent with the results of dynamic conditional correlation. This is not unexpected as direct investment occupies the larger share of the total inflows. Given the observed data, we believe there is a 95% possibility that the total inflow will increase by 1.28 to 4.13 with additional growth rate in the economy, while it will increase by 0.02 to 0.06 with an increased interest rate differential. Other variables such as debt as a percentage of GDP, long-term bond yield, world uncertainty index and Rand depreciation are observed to have a negative impact on total inflow.
Table 6. BLR results with total inflow as dependent variable
From our empirical findings, the size of GDP is found to play a notable role in attracting capital inflow. This is consistent with some empirical findings in the literature. For instance, Reinhart and Reinhart (Citation2009) submit that economic growth is an important determinant of economic growth. Our findings also show that interest rate differential and exchange rate movement play positive role in attracting portfolio inflow and direct investment inflow into the domestic economy. This is consistent with Alfaro et al. (Citation2007) and Reinhart and Reinhart (Citation2009) among others. However, this finding could be said to be at variance with earlier study by Broner and Rigobon (Citation2004) as they argued that macroeconomic factors could not provide significant explanation on the sources of capital inflow. Our empirical findings also show that portfolio inflow and global economic uncertainty does not necessarily have impact on portfolio inflow while the world uncertainty index is found to have negative impact on direct investment inflow. This is in support of the findings by Forbes and Warnock (Citation2012) and Allen and Giovannetti (Citation2011). The conclusion corroborates out findings that global economic uncertainty affects direct capital inflow. This however at variance with earlier submission of Méon and Sekkat (Citation2012). Our findings also show that debt-GDP ratio is an important negative factor among international investors for both direct and portfolio inflows. This is consistent with so many empirical studies in the literature such as Sarno et al. (Citation2016), Mercado and Park (Citation2011), and Forbes and Warnock (Citation2012). The affirmed unfavourable macroeconomic conditions such as high debt-GDP ratio is a deterrent to capital inflow in whatever form.
5. Conclusions
The study presented empirical evidence on the determinants of international capital inflow into South African economy. International investment inflow can be broadly classified into portfolio investments and direct investments portfolio. Portfolio investments are financial assets denominated in a national currency while direct investment inflow are real investments in factories, capital goods among others. The study investigated the determinants of these broad classifications of total international capital inflow and the total inflow as well, using time series quarterly data from 1960(Q1) to 2021(Q3) with special consideration on the role of global uncertainty. The data were sourced from the South African Reserve Bank (SARB) and Federal Reserve Economic Data (FRED). Data on portfolio inflow (PI). direct capital inflow (DCI) and total inflow were obtained from the South African Reserve Bank (SARB) while data on gross domestic product, debt-output ratio, world uncertainty index, long-term government yield, interest rate differential and exchange rate were obtained from Federal Reserve Economic Data (FRED). This study apart from contributing to relatively scarce country-specific studies on determinants of capital inflow, is largely unique because it employs Dynamic Conditional Correlation (DCC GARCH) to investigate time-varying correlation among the variables. The study also makes use of Bayesian Linear Regression Model to investigate the determinants of international capital inflow. The findings of the study are as follows: (1) the dynamic conditional correlation between portfolio inflow and GDP as well as direct inflow and GDP were entirely positive throughout the study, going as far as 0.8 in early 2020s. The implication is that size of GDP has been playing dominant role in attracting both portfolio inflow and direct investment inflow in recent times; (2) interest rate differential is also observed to play positive role in attracting portfolio inflow and direct investment inflow as their dynamic conditional correlation coefficients range between 0.1 and 0.4 throughout the entire study. The depreciation of South African Rand is also found to positively impact portfolio inflow perhaps because domestic portfolio will become much cheaper to foreign investors since more units of the portfolio can be bought with the same amount of United State dollar, while direct investment into South Africa economy is less sensitive to exchange rate movement; (3) the dynamic correlation coefficients between portfolio inflow and world uncertainty index are entirely weak and this suggests that global economic uncertainty does not necessarily have impact on portfolio inflow while the world uncertainty index is however found to have negative impact on direct investment inflow; (4) the dynamic conditional correlations between portfolio inflow and debt as a ratio of GDP as well as direct investment inflow and debt as a ratio of GDP are entirely in negative region going as far as −0.9 in early 2020 and thereafter. The implication here is that debt-GDP ratio is an important negative consideration among international investors for both direct and portfolio inflows in recent time; and (5) total inflow is found to display similar patterns and behavioural movements are observed as in the case of time-varying correlations between the direct investment inflow and the determinants.
In the same way, the results of Bayesian Linear Regression Model (BLR) show that debt and bond yield impact negatively on portfolio inflow into the economy while the effect of world uncertainty index is approximately zero which confirms the DCC results that portfolio inflow is not driven by the global external shock but rather inherent macroeconomic stability. GDP, interest rate differential and exchange rate depreciation are found to be positive drivers of portfolio inflow into South African economy. This is consistent with DCC estimation results. On the other hand, only GDP and interest rate differential are found to be positive drivers of direct investment inflow and total inflow into the South African economy. Long-term bond yield, exchange rate, debt as a ratio of GDP and world uncertainty index are found to be important negative drivers of both direct investment inflow and total inflow. Overall, it is evident from the empirical findings that global uncertainty index does not significantly correlate or affect portfolio inflow; while global uncertainty index is found to negatively correlate and impact direct capital inflow and total inflow. Consequently, government and South African policy makers should pay a closer attention to measures to mitigate against global uncertainties in attempt to increase direct capital investment flow. Also, stable macroeconomic conditions must be maintained in terms of steady growth of the economy, stable external value of Rand among others to further attract foreign investments, especially direct capital inflow. The current increasing debt profile of the country needs to be brought low so as to keep the debt-GDP ratio within a reasonable range that could not deter the foreign investors.
Disclosure statement
No potential conflict of interest was reported by the author(s).
Additional information
Funding
References
- Adams, S. (2009). Foreign direct investment, domestic investment, and economic growth in Sub-Saharan Africa. Journal of Policy Modeling, 31(6), 939–20. https://doi.org/10.1016/j.jpolmod.2009.03.003
- Adams, S., Klobodu, E. K. M., & Lamptey, R. O. (2017). The effects of capital flows on economic growth in Senegal. Margin: The Journal of Applied Economic Research, 11(2), 121–142. https://doi.org/10.1177/0973801016687869
- Ahiadorme, J. W. (2021). On the aggregate effects of global uncertainty: Evidence from an emerging economy. South African Journal of Economics. https://onlinelibrary.wiley.com/doi/pdfdirect/10 .1111/saje.12309
- Alfaro, L., Kalemli-Ozcan, S., & Volosovych, V. (2007). Capital flows in a globalized world: The role of policies and institutions. In Capital controls and capital flows in emerging economies: Policies, practices, and consequences (pp. 19–72). University of Chicago https://www.nber.org/system/files/chapters/c0148/c0148.pdf
- Alfaro, L., Kalemli-Ozcan, S., & Volosovych, V. (2008). Why doesn’t capital flow from rich to poor countries? An empirical investigation. The Review of Economics and Statistics, 90(2), 347–368. https://doi.org/10.1162/rest.90.2.347
- Allen, F., & Giovannetti, G. (2011). The effects of the financial crisis on Sub-Saharan Africa. Review of Development Finance, 1(1), 1–27. https://doi.org/10.1016/j.rdf.2010.10.002
- Anetor, F. O. (2020). Human capital threshold, foreign direct investment and economic growth: Evidence from sub-Saharan Africa. International Journal of Development Issues. https://doi.org/10.1108/IJDI-01-2020-0014
- Athari, S. A., Shaeri, K., Kirikkaleli, D., Ertugrul, H. M., & Ozun, A. (2020). Global competitiveness and capital flows: Does stage of economic development and risk rating matter? Asia-Pacific Journal of Accounting & Economics, 27(4), 426–450. https://doi.org/10.1080/16081625.2018.1481754
- Bhattarai, S., Chatterjee, A., & Park, W. Y. (2020). Global spillover effects of US uncertainty. Journal of Monetary Economics, 114, 71–89. https://doi.org/10.1016/j.jmoneco.2019.05.008
- Brafu-Insaidoo, W. G., & Biekpe, N. (2014). Determinants of foreign capital flows: The experience of selected sub-Saharan African countries. Journal of Applied Economics, 17(1), 63–88. https://doi.org/10.1016/S1514-0326(14)60003-9
- Broner, F., & Rigobon, R. (2004). Why are capital flows so much more volatile in emerging than in developed countries? Available at SSRN 884381. https://repositori.upf.edu/bitstream/handle/10230/969/862.pdf?sequence=1
- Broto, C., Díaz-Cassou, J., & Erce, A. (2011). Measuring and explaining the volatility of capital flows to emerging countries. Journal of Banking & Finance, 35(8), 1941–1953. https://doi.org/10.1016/j.jbankfin.2011.01.004
- Byrne, J. P., & Fiess, N. (2011). International capital flows to emerging and developing countries: National and global determinants. https://core.ac.uk/outputs/287544560
- Byrne, J. P., & Fiess, N. (2016). International capital flows to emerging markets: National and global determinants. Journal of International Money and Finance, 61, 82–100. https://doi.org/10.1016/j.jimonfin.2015.11.005
- Calvo, G. A., Leiderman, L., & Reinhart, C. M. (1993). Capital inflows and real exchange rate appreciation in Latin America: The role of external factors. Staff Papers - International Monetary Fund, 40(1), 108–151. https://doi.org/10.2307/3867379
- Campion, M. K., & Neumann, R. M. (2004). Compositional effects of capital controls: Evidence from Latin America. The North American Journal of Economics and Finance, 15(2), 161–178. https://doi.org/10.1016/j.najef.2003.11.004
- Canova, F. (2005). The transmission of US shocks to Latin America. Journal of Applied Econometrics, 20(2), 229–251. https://doi.org/10.1002/jae.837
- Caprio, G., Laeven, L., & Levine, R. (2007). Governance and bank valuation. Journal of Financial Intermediation, 16(4), 584–617. https://doi.org/10.1016/j.jfi.2006.10.003
- Dornean, A., Işan, V., & Oanea, D. C. (2012). The impact of the recent global crisis on foreign direct investment. Evidence from central and Eastern European countries. Procedia Economics and Finance, 3, 1012–1017. https://doi.org/10.1016/S2212-5671(12)00266-3
- Fedderke, J. W., & Liu, W. (2002). Modelling the determinants of capital flows and capital flight: With an application to South African data from 1960 to 1995. Economic Modelling, 19(3), 419–444. https://doi.org/10.1016/S0264-9993(01)00071-2
- Fernandez-Arias, E. (1996). The new wave of private capital inflows: Push or pull? Journal of Development Economics, 48(2), 389–418. https://doi.org/10.1016/0304-3878(95)00041-0
- Forbes, K. J., & Warnock, F. E. (2012). Debt-and equity-led capital flow episodes (No. w18329). National Bureau of Economic Research.
- Hlaing, S. W., & Kakinaka, M. (2019). Global uncertainty and capital flows: Any difference between foreign direct investment and portfolio investment? Applied Economics Letters, 26(3), 202–209. https://doi.org/10.1080/13504851.2018.1458182
- Huang, Y. (2010). China’s Great Ascendancy and structural risks: Consequences of asymmetric market liberalisation. Asian‐Pacific Economic Literature, 24(1), 65–85. https://doi.org/10.1111/j.1467-8411.2010.01250.x
- Kaminsky, G. L., & Schmukler, S. L. (2003). Short-run pain, long-run gain: The effects of financial liberalization. National Bureau of Economic Research, Working Paper 9787, 1-63. https://www.nber.org/papers/w9787
- Khobai, H., & Mavikela, N. (2018). Investigating the link between foreign direct investment, energy consumption and economic growth in Argentina (No. 1808).
- Maćkowiak, B. (2007). External shocks, US monetary policy and macroeconomic fluctuations in emerging markets. Journal of Monetary Economics, 54(8), 2512–2520. https://doi.org/10.1016/j.jmoneco.2007.06.021
- Méon, P. G., & Sekkat, K. (2012). FDI waves, waves of neglect of political risk. World Development, 40(11), 2194–2205. https://doi.org/10.1016/j.worlddev.2012.03.022
- Mercado, R. V., Jr, & Park, C. Y. (2011). What drives different types of capital flows and their volatilities in developing Asia? International Economic Journal, 25(4), 655–680. https://doi.org/10.1080/10168737.2011.636628
- Naudé, W. A., & Krugell, W. F. (2007). Investigating geography and institutions as determinants of foreign direct investment in Africa using panel data. Applied Economics, 39(10), 1223–1233. https://doi.org/10.1080/00036840600567686
- Neumann, R. M., Penl, R., & Tanku, A. (2009). Volatility of capital flows and financial liberalization: Do specific flows respond differently? International Review of Economics & Finance, 18(3), 488–501. https://doi.org/10.1016/j.iref.2008.04.005
- Noorbakhsh, F., Paloni, A., & Youssef, A. (2001). Human capital and FDI inflows to developing countries: New empirical evidence. World Development, 29(9), 1593–1610. https://doi.org/10.1016/S0305-750X(01)00054-7
- Passari, E., & Rey, H. (2015). Financial flows and the international monetary system. The Economic Journal, 125(584), 675–698. https://doi.org/10.1111/ecoj.12268
- Prasad, E., Rajan, R. G., & Subramanian, A. (2006). Patterns of international capital flows and their implications for economic development. Proceedings of the 2006 Jackson Hole Symposium, Federal Reserve Bank of Kansas City (pp. 119–158).
- Reinhart, C. M., & Reinhart, V. R. (2009). Capital flow bonanzas: An encompassing view of the past and present. In NBER international seminar on macroeconomics (Vol. 5 9–62). The University of Chicago Press.
- SARB. (2022). Publications of South African Reserve Bank on Poverty and Inequality.
- Sarno, L., Tsiakas, I., & Ulloa, B. (2016). What drives international portfolio flows? Journal of International Money and Finance, 60, 53–72. https://doi.org/10.1016/j.jimonfin.2015.03.006
- Shah, M. H., & Faiz, M. (2015). Terrorism and foreign direct investment: An empirical analysis of SAARC countries. MPRA 82008, 1-22. https://mpra.ub.uni-muenchen.de/82008/8/MPRA_paper_82008.pdf
- Tiwari, A. K., & Mutascu, M. (2011). Economic growth and FDI in Asia: A panel-data approach. Economic Analysis and Policy, 41(2), 173–187. https://doi.org/10.1016/S0313-5926(11)50018-9
- Ucal, M., Özcan, K. M., Bilgin, M. H., & Mungo, J. (2010). Relationship between financial crisis and foreign direct investment in developing countries using semiparametric regression approach. Journal of Business Economics and Management, 11(1), 20–33. https://doi.org/10.3846/jbem.2010.02
- Vidal, G., & Correa, E. (2007). Outsourcing and FDI in developing countries: The case of the mexican economy. The Journal of Economic Asymmetries, 4(1), 111–122. https://doi.org/10.1016/j.jeca.2007.01.008