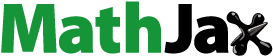
Abstract
We employ senior bank loan officers’ responses regarding actual and expected loan demand from enterprises linking successive surveys to determine the dominant expectation formation mechanism that best describes European senior bank loan officers’ expectations. Our findings suggest that the adaptive expectations mechanism is compatible with senior bank loan officers’ expectations for loan demand from enterprises. Our study contributes to the understanding of the formation of loan demand expectations, and hence our findings can be beneficial for monetary policy purposes.
PUBLIC INTEREST STATEMENT
A description of your paper of NO MORE THAN 150 words suitable for a non-specialist reader, highlighting/explaining anything which will be of interest to the general public.
Models are important tools that economists use to help them understand the behaviour of economic agents and of the economy as a whole. In this context, this study explores the formation of expectations of loan officers regarding the future loan demand from enterprises relative to the benchmark assumption of rational expectations. Understanding the way expectations are formed is of great importance especially when dealing with the design of credit conditions and the overall credit cycle of an economy which may affect future investment plans and hiring decisions of firms. However, the rational expectations assumption is a very strong one. This has led economists to investigate the effect of deviations from it, namely the “adaptive” and the “regressive” hypotheses. Our results qualify adaptive expectations as the mechanism that more adequately describes the formation of expectations of loan officers.
1. Introduction
The past decade has been shaped by a series of major and minor shocks which are followed by periods of high volatility (Baldovin et al., Citation2013). At the same time, markets experienced one of the longest and strongest bull markets in history. Such a controversy encapsulates the strong relation between agents’ expectations and the real macro and financial variables. Within this context, Assenza et al. (Citation2014), note that the economy is thought of as an expectation feedback mechanism in which expectations play a prominent role in shaping agents’ decisions, which in their turn affect the real economy.
In this context, fluctuations in the demand or supply for loans may affect market interest rates, inflation dynamics, investment, and recovery of firms’ aftershocks (especially for those that are bank loan dependent), among others. Ultimately, the effectiveness of monetary policy may be limited by these fluctuations and other frictions on the market for bank loans. Recent examples are the quantitative easing programs carried out by some of the most important central banks of developed countries: loans made were well below the availability of funds. To understand whether this gap was more a supply problem (banks not willing to lend) or a demand problem (firms not willing to borrow) is crucial. Therefore, studying how agents involved in the bank lending process form their expectations may shed some understanding of the success and failures of this type of unconventional monetary policies, and monetary transmission in general.
Indeed, the better understanding of the underlying loan decision-making process via the expectations’ formation mechanisms is crucial for the proper design of economic policies both for academics and policymakers. Specifically, senior bank loan officers’ expectations for loan demand from enterprises are of paramount importance to the extent that credit conditions are key to several economic outcomes for firms such as future investment plans and hiring decisions (e.g., Ferrado et al., Citation2019). Therefore, an increased (decreased) demand for loans, a core driver of bank income, influences bankers’ sentiment, that is, tend to increase optimism (pessimism) and, therefore, likely lead the loan officers to loosen (tight) loan provisions affecting the overall trend of economic activity.
Given thus the importance of loan officers’ expectations, a critical question is how agents shape their expectations on loan demand from enterprises. Understanding the loan officers’ expectations mechanism is a relevant issue for policy design in terms of credit cycle. This approach has been empirically investigated using three widely accepted theories: the “rational” expectations hypothesis (REH hereafter), the “adaptive” and the “regressive”. However, rational expectations is a simplifying assumption and at the same time, a very strong assumption; hence it is useful exploring the effects of deviating from it. In particular, if the actual outcome is the direct result of agents’ expectations, then the mechanism of the rational perceptions is confirmed. If not, then researchers should explore the pattern of the perception error. To this end, the mechanisms of Adaptive or Regressive expectations should be considered. Hence, any deviation from the rational expectations hypothesis which assumes zero error can affect the decision-making process. In addition, if a drift occurs, it would be very important for policymakers to know how quickly the agents adjust/correct their perception of error in order to allow the implementation of an optimal policy (Assenza et al., Citation2014).
Expectations so far have been modeled in an ad hoc manner by many previous studies. However, there is a rapidly increasing amount of research on the mechanisms that form the expectations employing survey dataFootnote1 (Dave, Citation2011; Dominitz & Manski, Citation1997; Drakos, Citation2008; Dutt & Ghosh, Citation1997; Fraser & MacDonald, Citation1993; Kim, Citation1997; Madsen, Citation1996; Miah et al., Citation2016; Pesaran & Weale, Citation2006; Prat & Uctum, Citation2011). The main benefit of using survey data is that by definition corresponds to expectations that the respondents state. As Manski (Citation2004) asserted, one of the best ways to assess both the accuracy and the correctness of expectations is to follow the respondents as time passes and then contrast their expectations with the real events they experience.
We utilize survey responses from successive surveys obtained from the Bank Lending Survey (BLS hereafter) to examine how bank loan officers’ expectations of future business loan demand are fulfilled when they are confronted with the realized outcomes. This study contributes to the literature of expectations in two distinct ways. First, we bring for the first-time evidence about the expectation formation mechanisms that govern Eurozone’s senior bank loan officers regarding future loan demand provided to enterprises. Second, our new evidence comes from country-level survey data on expectations for a large number of European countries, obtained from the BLS database. To the best of our knowledge, existing studies have not employed these data before, and we aim at filling this gap in the literature. An essential advantage of the BLS database is that the very nature of the data allows us to consider whether banks make correct predictions of the direction of future loan demand and if they change in a systematic manner. Our results qualify adaptive expectations as the mechanism that most adequately describes the formation of expectations. To the best of our knowledge, existing studies have not used these data before, something that adds value to the current empirical literature.
The remainder of the paper is structured as follows: Section 2 describes the empirical methodology. Section 3 describes the data and the variables we use. Section 4 presents the estimation results. Finally, Section 5 concludes.
2. A brief literature background
The idea of expectations in economic theory is not new and refers to the forecasts or views that decision makers hold about key economic variables. The importance of expectations is due to their often-substantial effect on the current choices of economic agents and hence the overall level of economic activity. First of all, Keynes (Citation1936) assigned people’s expectations about the future—which he called “waves of optimism and pessimism”—a central role in determining the business cycle. However, Keynes did not have an explicit model of how expectations are formed. In the 1950s and 1960s, expectations were introduced into practically every area of macroeconomics, including investment, consumption, demand for money and inflation using adaptive expectations. Koyck (Citation1954) and Cagan (Citation1956) are considered to be the founders of the theory of adaptive expectations. The root of this approach is based on a simple principle, the fact that past events shape the future agents’ expectations. Adaptive expectations differ from rational expectations (RE), which form a different approach to predicting an economical or financial future. Indeed, Muth (Citation1961) laid the foundations of a standard methodology for modelling expectations via the concept that agents are rational decision-makers. In particular, agents make decisions based not only on past information but also, they make use of all the available information of the economy and all underlying interdependencies along with their human rationality. As a result, economic agents are able to make unbiased predictions of future economic variables, that is, without systematic errors (e.g., Assenza et al., Citation2014). Rational Expectations Hypothesis was further developed by Lucas (Citation1972) and Sargent and Wallace (Citation1976) setting it as the benchmark paradigm in both micro and macroeconomics changing the way the policy is conducted (Dominitz & Manski, Citation1997; Goodwin & Sheffrin, Citation1982; Keane & Runkle, Citation1990, Citation1993; Pesaran, Citation1987). Finally, another strand of research has gone beyond Rational Expectations by developing Regressive Expectations models. Regressive expectations hypothesis was developed by Modigliani and Sutch (Citation1966), modelling the term structure of interest rates. Frenkel (Citation1975) has also applied the model to expectations of inflation rates. This approach essentially states that agents expect the variable of main interest to adjust or “regress” towards some long run “normal level.”
A set of studies have shown that the mainstream approach of Rational Expectations is often unrealistic; that is, agents do not have sufficient capabilities to make fully and accurate rational predictions (e.g., Sargent, Citation1993; Evans & Honkapohja, Citation2001; Branch, Citation2004; Assenza et al., Citation2014; Colasante et al., Citation2017 etc.). A major disadvantage of testing the REH is that it is based on ex post observed data, which is then used to form expectation errors. In this context, if our data are not compatible to the REH, it will not come as a surprise. Besides, REH does not propose any special expectation formation mechanism and should elucidate all persistent errors and allow agents to make use of all available information (e.g., Chow, Citation1989, Citation2011). The only way to overcome such a problem is to rely on survey data (e.g., Dave, Citation2011; Dominitz & Manski, Citation1997; Drakos, Citation2008; Dutt & Ghosh, Citation1997; Kim, Citation1997; Madsen, Citation1996; Miah et al., Citation2016; Pesaran & Weale, Citation2006; Prat & Uctum, Citation2011). Thus, directly observing market participants expectations allows the researcher to assess both the accuracy and the correctness of expectations as time passes and contrast them with the actual events (Manski, Citation2004).
The crucial question of the formation of expectations is then, which of the three widely accepted theories prevails? In this line, there are controversial empirical results about which model can dominate depending on the data used. There are studies which do find support regarding adaptive expectations as being superior to rational expectations (e.g., Moosa & Shamsuddin, Citation2004; Chow, Citation2011 etc.), while others rejecting adaptive expectations and the rational expectations hypothesis suggesting instead regressive expectations formation (e.g., Drakos, Citation2008; Dave, Citation2011 etc.). The way agents and in our case individual loan officers form their expectations is closely relevant to policy designs. Hence, the question in empirical studies of which approach is dominant becomes quite relevant to the policymakers in order to know the time effect of the, if any, adopted policy. In order to do so, there is a number of studies adopting the predictive ability of survey-based expectations against the model-based rational expectations in various markets showing that models perform better when agents’ stated expectations are taken into account (e.g., Lee & Shields, Citation2000; Mankiw & Reis, Citation2001; Mehra, Citation2002; Moosa & Shamsuddin, Citation2004; Pieroni & Ricciarelli, Citation2005; Boulier et al., Citation2006; Drakos, Citation2008; Prat & Uctum, Citation2011; Dave, Citation2011; Miah et al., Citation2016; Golitsis et al., Citation2022 etc.)
From an empirical viewpoint then the scope of this study is to explore the mechanism that more adequately describes the behaviour of expectations’ formation. Hence, we explicitly focus on survey data in order to test which of the three theories applies on the expectations of loan officers regarding the loan demand. In compliance to the Adaptive Expectation, economic agents adjust their forecasts in each period depending on the previous period’s expectation/forecasting error (Lovell, Citation1986). Chow (Citation1989, Citation2011) managed to provide econometric evidence which shows that Adaptive Expectations are better than Rational Expectations. Hence, we cannot rule out the fact that the adaptive expectation formation mechanism might be the best description of our data. Finally, the Regressive Expectations Theory enters conceptually as quite similar to adaptive expectations in the estimation. Indeed, as stated above in the case of adaptive expectations, the adjustments are made towards the time variable past formed expectation, while in regressive expectations it is made towards a time invariant mean.
3. Empirical methodology
The starting point of our empirical methodology is to test for the Rational Expectations Hypothesis (REH hereafter). Pursuant to the REH, agents do not make any systematic errors when they form their expectations because they take into consideration the whole set of available information.
Following Lovell (Citation1986) and Drakos (Citation2008) we examine the REH by employing the following model:
where i, t, A, and E denote country, time, actual LD, and expected LD, respectively. We express the expected LD from enterprises with a time lag at the right-hand side of the equation to signify that the expectations for loan demand have been shaped prior to the actual outcome.
In order to accept the REH, the following associated joint hypotheses of unbiasedness must not be rejected:
H0: α0 = 0, α1 = 1
Consistent with the adaptive expectations mechanism, senior bank loan officers modify their expectations in each period depending on the expectation (or forecasting) error of the previous period. If a zero-forecasting error existed (i.e., a perfect forecast in the previous period), it would suggest that the previous expectation would be maintained perpetually (Lovell, Citation1986).
Following Lovell (Citation1986), Moosa and Shamsuddin (Citation2004), and Drakos (Citation2008) we define the adaptive expectations model as:
where i, t, A, and E denote country, time, actual LD (Loan Demand), and expected LD (Loan Demand), respectively. Parameter θ is the coefficient of adaptation, showing the pace of adjustment to the previous period’s expectation error. In order to accept the adaptive expectations hypothesis, parameter θ must be statistically significant and lie in the interval (−1,0).
We test whether parameter θ is statistically significant, and we also test whether θ is different from its maximum theoretical value (−1):
According to the regressive expectations hypothesis, agents believe that the variable of interest shows a propensity to move towards its average value (Drakos, Citation2008). Following Pesaran and Weale (Citation2006), Drakos (Citation2008), and Dave (Citation2011) the regressive expectations mechanism can be specified as follows:
where i, t, A, E, Δ, , and
denote country, time, actual LD, expected LD, first differences, the sample mean of the actual LD and the adjustment parameter, respectively.
The regressive expectations hypothesis is consistent with the data if and only if parameter ψ is statistically significant, positive, and lies in the (0, 1) interval. In addition, apart from testing whether parameter ψ is different from zero, we also examine whether it is different from its maximum theoretical value (+1):
4. Data issues and econometric methodology
For both actual (realized) and expected loan demand from enterprises (LD), we employ data from the BLS. The BLS is a survey-based database updated four times a year (i.e., on a quarterly basis) by the European Central Bank (ECB hereafter), encompassing very useful information about the bank lending conditions in the Euro-area. BLS along with the U.S. Federal Reserve Board’s Senior Loan Officer Opinion Survey on Bank Lending Practices (SLOOS) are the best-known bank loan officer surveys.
In any given BLS issue, senior bank loan officers are invited to respond on the future (expected) loan demand (LD) from enterprises, as well as for the corresponding occurred (actual) loan demand (LD) from enterprises in the previous period. Consequently, by linking successive survey responses, we investigate whether senior bank loan officers’ expectations for LD from enterprises are formed rationally, and if deviations from rationality exist, we examine whether they conform to other widely known expectation formation mechanisms (i.e., adaptive and regressive expectations).
Our sample covers the period 2003Q1-2019Q4 for 14 Euro-area countries.Footnote2 This produces an unbalanced panel dataset of 769 observations, consisting of country-quarterly dimensions. Moreover, the data for LD are provided by the BLS as a diffusion index and not as the senior bank loan officers’ raw responses. According to the BLS GlossaryFootnote3 “[…] Regarding demand for loans, the diffusion index is defined as the difference between the weighted sum of the percentages of banks responding, “increased considerably” and “increased somewhat”, and the weighted sum of the percentages of banks responding, “decreased considerably” and “decreased somewhat”. Pursuant to the definition of the diffusion index, lower (greater) values signify decreased (increased) LD from enterprises.
In this study, we employed the Panel Corrected Standard Errors (PCSE hereafter) approach as our main estimation technique (Beck & Katz, Citation1995). This estimation simultaneously corrects autocorrelation, cross-sectional dependence, and heteroscedasticity to improve parameter efficiency (e.g., Sandow et al., Citation2021). Indeed, panel data are regarded as having repeated observations over time on some set of units, in our case the 14 EU countries. This typically leads to non-consistent results against the usual performed diagnostics. In this context then, Beck and Katz (Citation1995) developed the PCSE, which is robust as concern the possibility of non-spherical errors. Furthermore, the panel-corrected standard error (PCSE) technique is used when working with dynamic heterogeneous panel data (Bailey & Katz, Citation2011). All in all, the PCSE approach is generally a more appropriate approach for long panel data (T > N) than pooled least squares or Fixed/Random Effects with robust standard errors (in our case T = 68 quarters and N = 14 countries). Hence, the main benefit of the PCSE approach is that it allows the error term to be correlated over i and be heteroskedastic (Cameron & Trivedi, Citation2010). In contrary, other techniques such as the FGLS are not that appropriate as according to Beck and Katz (Citation1995) and yield coefficient standard errors that are rigorously underestimated.
5. Estimation results
Based on the PCSE estimation results in Table , it contains all the estimation results for each expectation formation mechanism along with the corresponding hypotheses tests. As far as the results of the REH are concerned, although we find that parameter a1 is statistically significant at the 1% level, we emphatically reject the null hypothesis that the parameter is equal to 1. This finding provides evidence against the unbiasedness hypothesis and consequently against rationality. Therefore, we conclude that senior bank loan officers do not form their expectations for loan demand from enterprises rationally. In other words, the REH is not consistent with our data.
Table 1. Estimation results for each expectation formation mechanism—PCSE methodology
We proceed now by exploring the process that more adequately describes the expectation formation mechanism. We observe that only for the adaptive expectations estimated slope parameters are consistent with their a priori expected signs. In particular, the slope parameter associated with the adaptive expectations is negative and lies in the interval (−1,0), indicating that if in the last period loan officers had overestimated (underestimated) the actual loan demand, they will adjust downwards (upwards) their expectations for the current period. On the contrary, the results for regressive expectations do not conform with the expected sign and value. Therefore, our results qualify the adaptive expectations as the mechanism that more adequately describes the formation of expectations of senior loan officers in line with e.g., Moosa and Shamsuddin (Citation2004); Chow (Citation2011) among others. Such a result suggests that loan officers systemically overestimate or underestimate the current situation, something that can substantially affect the loan market.
In some more detail, we document that the speed of adjustment θ is statistically significant at the 1% level (i.e., non-trivial). We also find that parameter θ is different from its maximum theoretical value of −1. The absolute magnitude of the point estimate of parameter θ suggests an adaptation rate of about 45.5%. Thus, 2.19 time periods are needed, on average, to cover the distance between the actual and expected LD. Parameter θ carries a negative sign, suggesting that if senior bank loan officers had overestimated (underestimated) the actual LD in the current period, they would then adjust downwards (upwards) their expectations for the next period. Moreover, we find that the point estimate parameter θ lies in the (0, 1) interval. Therefore, we document that adaptive expectations are consistent with our data.
Moving to the regressive expectations hypothesis (see, Table ), although we find that the adjustment parameter ψ is non-trivial in both estimation methods, its point estimates were found to be negative and statistically significant. Therefore, the regressive expectations mechanism is not consistent with our data.
Then, we conduct a sensitivity analysis re-estimating our models breaking our sample into two subgroups. Specifically, we break our sample into core and peripheral Euro-area countries. Following Anastasiou et al. (Citation2019), we define Austria, Belgium, Cyprus, Estonia, Germany, Latvia, Lithuania, Luxembourg, and Slovenia as core countries, while Greece, Ireland, Italy, Portugal, and Spain are considered peripheral ones. Therefore, we re-estimate each of our models twice, once for each country group.
In Table , we report the results for the core and peripheral countries. We find for both country groups that the adaptive expectations hypothesis is the dominant expectation formation mechanism.
Table 2. Estimation results for each expectation formation mechanism—Core_vs_Peripheral countries
Given that our sample covers the pre-crisis, the crisis, and the post-crisis period, it might be the case where the senior loan officers form their expectations in a different manner before and after the outburst of the recent financial crisis. To this end, we consider it imperative to demonstrate a second sensitivity analysis. We re-estimate the baseline specifications twice, once for the pre-crisis period (2003Q1-2007Q4) and once for the crisis period (2008Q1-2016Q1). We define the beginning of the recent financial crisis in Europe in the year 2008 (Gibson et al., Citation2016; Ivashina & Scharfstein, Citation2010; Lane, Citation2012). Furthermore, following Anastasiou et al. (Citation2019) we define the crisis period in the Euro-area to last until 2016Q1. The estimation results reported in Table are in line with our previous findings, which suggest that either before or after the 2008 financial crisis the European senior bank loan officers form their expectations for LD in an adaptive manner.
Table 3. Estimation results for each expectation formation mechanism—Crisis_vs_Pre-Crisis period
In addition, Cornille et al. (Citation2019) use 2013Q4 as the end of European sovereign crisis period, instead of 2016Q1 that Anastasiou et al. (Citation2019) suggested. Hence, as an additional robustness test, we re-estimate our regression models with the last point in time of the sovereign debt crisis being 2013Q4, to make sure the findings are not driven by the crisis definition. Once again, our findings remain robust as above (see ).
Table 4. Estimation results for each expectation formation mechanism—Crisis_vs_Pre-Crisis period
As a final robustness test, we re-estimate our specifications employing the Driscoll and Kraay’s (Citation1998) covariance matrix estimator. By relying on large-T asymptotics, Driscoll and Kraay (Citation1998) demonstrate that the standard nonparametric time-series covariance matrix estimator can be modified such that it is robust to general forms of cross-sectional as well as temporal dependence. Driscoll and Kraay’s approach loosely applies a Newey-West–type correction to the sequence of cross-sectional averages of the moment conditions. Adjusting the standard error estimates in this way guarantees that the covariance matrix estimator is consistent, independently of the cross-sectional dimension N (i.e., also for N → ∞). Therefore, Driscoll and Kraay’s approach eliminates the deficiencies of other large-T—consistent covariance matrix estimators. Once again, our findings reported in remain robust, and the Adaptive expectations is the dominant expectations formation mechanism.
Table 5. Estimation results for each expectation formation mechanism—Driscoll and Kraay (Citation1998) standard errors
6. Conclusions
We utilize survey data from the BLS for the period 2003Q1-2019Q4 and for 14 Euro-area countries to explore the performance of diverse competing expectation formation mechanisms for senior bank loan officers’ expectations for loan demand. We study the three primary expectation formation mechanisms, the rational, adaptive, and regressive expectations mechanisms. Our results indicate that senior bank loan officers do not shape their expectations for loan demand from enterprises rationally. We find evidence in favor of the adaptive expectations mechanism being the best description of the data. Our results are consistent for every country group we examined (all Euro-area, core, and peripheral countries). We also document that either before or during the 2008 financial crisis, the European senior bank loan officers form their expectations for loan demand in an adaptive manner. Hence, all in all, loan officers using their knowledge together with the actual data they observe, they form the expectations about loan demand for the next period, and they make according to their belief’s optimal decisions. This simple deviation from rational expectations, since information structure is not perfect, allows for a dynamic between expected and realised demand for loans. The way the loan officers correct their predictions of future loan demand if these do not coincide with the actual output is of great importance.
Our findings provide important policy implications. Such a situation where the European senior bank loan officers form their expectations for loan demand in an adaptive manner implies that they systemically overestimate or underestimate the current situation. This, in turn, may yield two unfavorable effects: (a) a potential surge of the discouraged bank borrowers and (b) cause adverse effects through the bank lending channel of the monetary policy transmission mechanism, to the extent that firms are bank loan dependent. Indeed, for bank-based economies in Eurozone, this can lead to a shrinkage of both loan provision and demand affecting the overall economic activity.
In order for policymakers to minimize such a disturbance in credit markets, they should closely follow the link between the formed expectations and the subsequent reality. If expectations and reality do not coincide each other as time passes, then policy designers should take into account this time gap and learn how quickly the adjustment to reality will take place. This is of vital importance as it allows the policymakers to decide the optimal timing of policy implementation in order the market to become more efficient. This requires though availability of information in the credit market so as to avoid a possible mismatch between demand and supply or a misjudgement from loan officers.
Finally, we need to note that the recent economic developments cannot be ignored and offer a great example of how the market can operate within a vulnerable environment. Especially, as monetary policy is becoming less accommodative due to unfavourable developments in global economy, banks face higher risk perceptions and show decreased risk tolerance. Within this context, the way the loan officers form their expectations becomes extremely important as a potential perception mismatch between supply and demand is rather negative. This highlights the necessity of feasible information across economic agents so as such errors to be controlled. In this sense, the monetary actions should work towards the direction of a stable credit cycle via supportive mechanisms in Eurozone countries like sharing information, quantitative easing, minimization of NPLs, supportive schemes of credit lines etc.
Disclaimer
The views and opinions expressed in this paper are those of the authors and do not reflect their respective institutions.
Disclosure Statement
No potential conflict of interest was reported by the authors.
Additional information
Funding
Notes on contributors
Dimitris Anastasiou
Dimitris Anastasiou works as a Research Economist at Alpha Bank and as a Researcher at the Athens University of Economics and Business. He holds a Ph.D. in Banking and Finance from the Athens University of Economics and Business. He has published extensively in international academic journals such as the Journal of Economic Behavior and Organization, the International Review of Financial Analysis, and the International Journal of Finance and Economics.
Christos Kallandranis is currently Assistant Professor in Finance at the University of West Attica in Greece. He has also taught at Regent’s University London in the UK, at Marconi University in Rome Italy, at the Hellenic Open University and at University of Patras. He holds a PhD in Economics from the University of Patras and an MSc in Finance & Economics from the Manchester Business School. He has published extensively in reputable international academic journals such as Journal of Economic Behavior and Organization, Journal of Common Markets, Finance Research Letters etc.
Khudoykulov Khurshid Khurramovich
Khudoykulov Khurshid Khurramovich is (DSc) is a Professor at the department of finance from the Tashkent State University of Economics (TSUE), Tashkent. His research interests focus on asset pricing, capital markets, investment, and the impact of geopolitical uncertainty on various macroeconomic and financial variables. He has published extensively in reputable international academic journals such as Economic Analysis and Policy, Economic Research, Cogent Economics and Finance so on.
Christos Kallandranis
Christos Kallandranis is currently Assistant Professor in Finance at the University of West Attica in Greece. He has also taught at Regent’s University London in the UK, at Marconi University in Rome Italy, at the Hellenic Open University and at University of Patras. He holds a PhD in Economics from the University of Patras and an MSc in Finance & Economics from the Manchester Business School. He has published extensively in reputable international academic journals such as Journal of Economic Behavior and Organization, Journal of Common Markets, Finance Research Letters etc.
Notes
1. A significant number of studies have also shown that macroeconomic models demonstrate better performance when survey-based expectations are employed rather than model-constructed expectations (see, for e.g., Batchelor, Citation1986; Lee & Shields, Citation2000; Madsen, Citation1996).
2. In order to assure a more homogeneous group of countries, in terms of institutional factors and economic status we choose to include only the Eurozone countries. BLS covers 14-euro area counties for the under-examination period since its first release in 2003.
3. Source: Glossary of the Bank Lending Survey of the ECB: (https://www.ecb.europa.eu/stats/pdf/ecbblsglossary.en.pdf).
References
- Anastasiou, D., Louri, H., & Tsionas, M. (2019). Non-performing loan in the euro-area: Are core-periphery banking markets fragmented? International Journal of Finance and Economics, 24(1), 97–13. https://doi.org/10.1002/ijfe.1651
- Assenza, T., Bao, T., Hommes, C., & Massaro, D. 2014. Experiments on expectations in macroeconomics and finance. In Experiments in macroeconomics (Research in Experimental Economics) (Vol. 17, pp. 11–70). Emerald Group Publishing Limited.
- Bailey, D., & Katz, J. N. (2011). Implementing panel corrected standard errors in R: The PCSE package. Journal of Statistical Software, 42(CS1), 1–11. https://doi.org/10.18637/jss.v042.c01
- Baldovin, F., Camana, F., Caraglio, M., Stella, A. L., & Zamparo, M. (2013). Aftershock prediction for high-frequency financial markets’ dynamics. In F. Abergel, B. Chakrabarti, A. Chakraborti, & A. Ghosh (Eds.), Econophysics of systemic risk and network dynamics. new economic windows (pp. 49-58). Springer.
- Batchelor, R. (1986). Quantitative vs. qualitative measures of inflation expectations. Oxford Bulletin of Economics and Statistics, 48(2), 99–120. https://doi.org/10.1111/j.1468-0084.1986.mp48002001.x
- Beck, N., & Katz, J. N. (1995). What to do (and not to do) with time-series cross-section data. American Political Science Review, 89(3), 634–664. https://doi.org/10.2307/2082979
- Boulier, B., Stekler, H., & Amundson, S. (2006). Testing the efficiency of the national football league betting market. Applied Economics, 38(3), 279–284. https://doi.org/10.1080/00036840500368904
- Branch, W. A. (2004). The theory of rationally heterogeneous expectations: Evidence from survey data on inflation expectations. Economic Journal, 114(497), 592_621. https://doi.org/10.1111/j.1468-0297.2004.00233.x
- Cagan, P. (1956). The monetary dynamics of hyperinflation. In Studies in the quantity theory of money. University of Chicago Press.
- Cameron, A. C., & Trivedi, P. K. (2010). Microeconometrics using stata (Vol. 2). Stata press.
- Chow, G. (1989). Rational versus adaptive expectations in present value models. Review of Economics and Statistics, 71(3), 376–384. https://doi.org/10.2307/1926893
- Chow, G., (2011). “Usefulness of adaptive and rational expectations in economics”, CEPS Working Paper No. 221.
- Colasante, A., Palestrini, A., Russo, A., & Gallegati, M. (2017). Adaptive expectations versus rational expectations: Evidence from the lab. International Journal of Forecasting, 33(4), 988–1006. https://doi.org/10.1016/j.ijforecast.2017.06.003
- Cornille, D., Rycx, F., & Tojerow, I. (2019). Heterogeneous effects of credit constraints on SMEs’ employment: Evidence from the European sovereign debt crisis. Journal of Financial Stability, 41, 1–13. https://doi.org/10.1016/j.jfs.2019.02.001
- Dave, C. (2011). Are Investment Expectations Rational, adaptive or regressive? Economic Inquiry, 49(1), 212–225. https://doi.org/10.1111/j.1465-7295.2010.00305.x
- Dominitz, J., & Manski, C. (1997). Using expectations data to study subjective income expectations. Journal of the American Statistical Association, 92(439), 855–867. https://doi.org/10.1080/01621459.1997.10474041
- Drakos, K. (2008). Efficiency and formation of expectations: Evidence from the European investment survey. Applied Economics, 40(8), 1015–1022. https://doi.org/10.1080/00036840600771189
- Driscoll, J. C., & Kraay, A. C. (1998). consistent covariance matrix estimation with spatially dependent panel data. The Review of Economics and Statistics, 80(4), 549–560. https://doi.org/10.1162/003465398557825
- Dutt, S., & Ghosh, D. (1997). Are experts’ expectations rational? A multicurrency analysis. Applied Economics, 29(6), 803–812. https://doi.org/10.1080/000368497326723
- Evans, G. W., & Honkapohja, S. (2001). Learning and expectations in macroeconomics. Princeton University Press.
- Ferrado, A., Gannoulis, I., & Preuss, C. (2019). Firms’ expectations on the availability of credit since the financial crisis. ECB Working Series, No. 2341.
- Fraser, P., & MacDonald, R. (1993). The efficiency of CAC expectations: A survey based perspective. Revue Economique, 44, 991–1000.
- Frenkel, J. (1975). Inflation and the formation of expectations. Journal of Monetary Economics, 1(4), 403–421. https://doi.org/10.1016/0304-3932(76)90011-8
- Gibson, H. D., Hall, S. G., & Tavlas, G. S. (2016). How the euro-area sovereign-debt crisis led to a collapse in bank equity prices. Journal of Financial Stability, 26, 266–275. https://doi.org/10.1016/j.jfs.2016.07.010
- Golitsis, P., Khudoykulov, K., & Palanov, S. (2022). Determinants of non-performing loans in North Macedonia. Cogent Business and Management, 9(1). https://doi.org/10.1080/23311975.2022.2140488
- Goodwin, T., & Sheffrin, S. (1982). Testing the Rational Expectations hypothesis in an agricultural market. Review of Economics and Statistics, 64(4), 558–567. https://doi.org/10.2307/1923950
- Ivashina, V., & Scharfstein, D. (2010). Bank lending during the financial crisis of 2008. Journal of Financial Economics, 97(3), 319–338. https://doi.org/10.1016/j.jfineco.2009.12.001
- Keane, M., & Runkle, D. (1990). Testing the rationality of price forecasts: New evidence from panel data. American Economic Review, 80, 714–735.
- Keane, M., & Runkle, D. (1993). Are analysts’ forecasts rational? Manuscript, Federal Reserve Bank of Minneapolis.
- Keynes, J. M. (1936). The general theory of employment, interest and money. MacMillan.
- Kim, S. J. (1997). Testing the rationality of exchange rate and interest rate expectations: An empirical study of Australian survey-based expectations. Applied Economics, 29(8), 1011–1022. https://doi.org/10.1080/000368497326408
- Koyck, L. M. (1954). Distributed lags and investment analysis. North- Holland.
- Lane, P. R. (2012). The European sovereign debt crisis. Journal of Economic Perspectives, 26(3), 49–68. https://doi.org/10.1257/jep.26.3.49
- Lee, K., & Shields, K. (2000). Expectations formation and business cycle fluctuations: An analysis of actual and expected output in UK manufacturing industries, 1975–1993. Oxford Bulletin of Economics and Statistics, 62(4), 463–490. https://doi.org/10.1111/1468-0084.00183
- Lovell, M. (1986). Tests of rational expectations hypothesis. American Economic Review, 76(1), 110–124.
- Lucas, R. (1972). Expectations and the neutrality of money. Journal of Economic Theory, 4(2), 103–124. https://doi.org/10.1016/0022-0531(72)90142-1
- Madsen, J. (1996). Formation of inflation expectations: From the simple to the rational expectations hypothesis. Applied Economics, 28(10), 1331–1337. https://doi.org/10.1080/000368496327877
- Mankiw, G., & Reis, R. (2001). “Sticky information versus sticky prices: A proposal to replace the New Keynesian Phillips Curve,” NBER Working Paper No. 8290.
- Manski, C. F. (2004). Measuring Expectations. Econometrica, 72(5), 1329–1376. https://doi.org/10.1111/j.1468-0262.2004.00537.x
- Mehra, Y. (2002). Survey measures of expected inflation: Revisiting the issues of predictive content and rationality. Economic Quarterly, 88, 17–36.
- Miah, F., Saifur, R., & Khalid, A. (2016). Rationality of survey based inflation expectations: A study of 18 emerging economies’ inflation forecasts. Research in International Business and Finance, 36, 158–166. https://doi.org/10.1016/j.ribaf.2015.09.029
- Modigliani, F., & Sutch, R. (1966). Innovations in interest rate policy. The American Economic Review, Papers and Proceedings, 56(1966), 169–180.
- Moosa, I., & Shamsuddin, A. (2004). Expectation formation mechanisms, profitability of foreign exchange trading and exchange rate volatility. Applied Economics, 36(14), 1599–1606. https://doi.org/10.1080/0003684042000217977
- Muth, J. (1961). Rational expectations and the theory of price movements. Econometrica, 29(3), 315–335. https://doi.org/10.2307/1909635
- Pesaran, H. (1987). The limits to rational expectations. Basil Blackwell.
- Pesaran, H. M., & Weale, M. (2006). Survey Expectations. In G. Elliott, C. W. J. Granger, & A. Timmermann (Eds.), Handbook of economic forecasting. North-Holland.
- Pieroni, L., & Ricciarelli, M. (2005). Testing rational expectations in primary commodity markets. Applied Economics, 37(15), 1705–1718. https://doi.org/10.1080/00036840500173205
- Prat, G., & Uctum, R. (2011). Modelling oil price expectations: Evidence from survey data. The Quarterly Review of Economics and Finance, 51(3), 236–247. https://doi.org/10.1016/j.qref.2011.03.003
- Sandow, J. N., Duodu, E., & OtengAbayie, E. F. (2021). Regulatory capital requirements and bank performance in Ghana: Evidence from panel corrected standard error. Cogent Economics & Finance, 9, 1, 2003503. https://doi.org/10.1080/23322039.2021.2003503
- Sargent, T. (1993). Bounded rationality in macroeconomics. Oxford University Press Inc.
- Sargent, T., & Wallace, N. (1976). Rational expectations and the theory of economic policy. Journal of Monetary Economics, 2(2), 169–183. https://doi.org/10.1016/0304-3932(76)90032-5