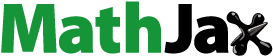
Abstract
Nowadays, the ESG-oriented portfolios are very popular. This study aims to study the performance of cross-asset portfolios between eco-friendly stocks (represented by Sri-Kehati index) with cryptocurrencies, bonds and gold. The data used in the study were the daily return of each instrument from January 2019 to December 2021, which was then analyzed using the DCC-GARCH analysis technique. The results show that adding Ripple, gold and bonds into a portfolio consisting of Sri-Kehati stocks can reduce the investment risk. The findings imply that investment managers and investors who have portfolios consisting of eco-friendly stocks such as Sri-Kehati stocks and bonds may reduce investment risk and balance their portfolios by adding gold or cryptocurrencies separately.
1. Introduction
Sustainable and responsible investment has become increasingly popular thanks in part to the growth in public awareness regarding the importance of investing in eco-friendly business sectors. The UN-pioneered Sustainable Development Goals (SDGs), which consist of 17 goals, also help support the development of sustainable and responsible investment. In a global context, these investments are often referred to as environmental, social, and governance (ESG)-oriented investments or green investments (Indriastuti et al., Citation2021; Ouchen, Citation2021).
In Indonesia, one of the guides that can be used by investors to invest in eco-friendly business sectors is the SRI-Kehati stock index, which was introduced by the Indonesia Stock Exchange in collaboration with Yayasan Keanekaragaman Hayati Indonesia (Yayasan KEHATI; Kurniatama et al., Citation2021; Robiyanto et al., Citation2021; Zulkafli et al., Citation2017). The SRI-Kehati index consists of 25 stocks that meet various criteria; the first criteria include total assets, price-to-earnings ratio (PER), and free float ratio. Meanwhile, the second one relates to several aspects such as Environmental, Community, Corporate Governance, Human Rights, Business Behavior, Labor Practices, and Decent Work (Salvatori et al., Citation2020).
Some studies have been carried out on investing in environmental- and social-friendly stocks; a study carried out by Utomo et al. (Citation2018) found that environmental- and social-friendly stocks tend to perform better than others, Zulkafli et al. (Citation2017) found that stocks included in SRI-Kehati index performed relatively better than other stocks in Jakarta Composite Index (JCI); the thesis is further supported by Robiyanto (Citation2017). A study on ESG-oriented stocks by Ouchen (Citation2021) found that those stocks were relatively more stable than non-ESG stocks.
Studies on ESG-oriented portfolios have been carried out by Naffa et al. (Citation2020) and Robiyanto et al. (Citation2021). While Naffa et al. (Citation2020) focused on the formation of ESG-based portfolio, Robiyanto et al. (Citation2021) conducted a study on the formation of a cross-asset portfolio between gold instruments and SRI-Kehati stocks using dynamic methods such as DCC-GARCH. Also, there are empirical evidences, such as one provided by Frensidy (Citation2016), Frensidy et al. (Citation2017), and Raza et al. (Citation2019), which show that cross-asset portfolios tend to perform better over time.
The reason for using the dynamic method is that stocks tend to fluctuate over time, so the usage of traditional portfolio formulation methods which tend to use constant correlation is not appropriate meaning that dynamic approach will help researchers simulate real-life situations better according to the capital market dynamics (see, Eimer (Citation2011); Katzke (Citation2013); Ogata (Citation2012); Zinecker et al. (Citation2016)). Some studies also using DCC as an input for their portfolios’ formulation, i.e. Arouri et al. (Citation2015), Kumar (Citation2014), and Robiyanto et al. (Citation2017), are very important because correlation is very important in financial management and portfolio formulation (Engle, Citation2002). DCC-GARCH is used in this study because it has proven that it can be successively estimated for time-varying covariance matrices (Filis et al., Citation2011) and very useful to formulate dynamic portfolio (Kumar, Citation2014; Putra et al., Citation2018).
Various studies on the formation of cross-asset portfolios using dynamic approach have been carried out before. Arouri et al. (Citation2015), Kumar (Citation2014), and Robiyanto et al. (Citation2017) used stocks and gold; Susilo et al. (Citation2020) used stocks and cryptocurrencies, and Robiyanto et al. (Citation2019) used stocks and fixed income instruments. Robiyanto et al. (Citation2021) specifically studied the formation of cross-asset portfolios between eco-friendly stocks and gold. Gold and other instruments such as bonds and cryptocurrencies were chosen due to their potential to become hedge and safe-haven instruments. Baur and Lucey (Citation2010) pioneered the study on gold as hedge and safe-haven following the Global Financial Crisis (GFC) in 2008. Some studies found that gold may act as safe-haven (Agyei-Ampomah et al., Citation2013; Akhtaruzzaman et al., Citation2021; Bahloul et al., Citation2021; Baur & McDermott, Citation2012), and so do bonds (Baur & Lucey, Citation2010; Robiyanto, Citation2018) and cryptocurrencies (Conlon et al., Citation2020; Shahzad et al., Citation2019; Susilo et al., Citation2020; Vukovic et al., Citation2021; Yuhanitha & Robiyanto, Citation2021).
Different from those previous studies, this study will scrutinize the potential of formulation of portfolios which consist of eco-friendly stocks with cryptocurrencies, gold and bonds. To the best of the author’s knowledge, there has been no research on the formation of cross-asset portfolios between eco-friendly stocks and cryptocurrencies or bonds. This research will fill the gap, particularly in the formation of a dynamic portfolio between eco-friendly stocks (represented by the SRI-Kehati index) and cryptocurrencies, gold and bonds.
This study found that adding some cryptocurrencies and gold into eco-friendly stocks such as Sri-Kehati stocks and bonds may reduce investment risk and enhance its performance. This study will contribute to the cross-assets portfolio formation’s literatures in terms of the potential mix of stock and other asset classes could produce better performance and also the importance of dynamic portfolio formulation.
The remainder of this paper is organized as follows. Section 2 discusses the review of literature. Section 3 presents data. Section 4 presents the methodology. Section 5 discusses the results. Finally, the last section concludes.
2. Literature review
2.1. Hedge and safe haven
Baur and Lucey (Citation2010) developed a more applicable and operational definition of hedge & safe-haven. At that time, the world’s economy suffered from Global Financial Crisis in 2008, which triggered massive flights to safety and quality, with gold considered as one of the “safe-haven” According to them, hedge may be defined as assets that have negative correlations with other assets or instruments, while safe havens are assets that have negative correlations with other assets or instruments during extreme conditions. Baur and Lucey (Citation2010)’s definition has been widely accepted by other researchers in the field.
Arouri et al. (Citation2015) stated that the study of the market for gold and other precious metals had received great attention from researchers and practitioners in the financial sector, since investors are willing to hedge using other assets (including precious metals) to reduce their investment risk. Gold and other precious metals are getting increasingly popular as attractive alternative investment instruments due to their volatility and weak correlation of returns. Meanwhile, Gunawan and Anggono (Citation2021) stated that cryptocurrencies could not be used as safe-haven assets for JCI-listed stocks.
3. Data
The data used in this study were the daily closing price data for Sustainable and Responsible Investment Index on the Indonesia Stock Exchange (SRI-Kehati index), bond indexes such as the Indonesian Corporate Bond Index (ICBI), gold prices on international markets, cryptocurrency prices such as Bitcoin, Ripple Coin and Ethereum as well as yield on one-year bonds from January 2019 to December 2021 (634 observation days). All data were obtained from Bloomberg Terminal. To calculate the return of each instrument, the following formula was used:
Pricei,t was the closing price of instrument i at period t, and Pricei,t-1 was the closing price of instrument i at period t-1. This study also considers average trading costs imposed by brokers in Indonesia. The average trading costs for buy and sell in Indonesia around 0.4% in total (0.15% for buy and 0.25% for sell).
4. DCC-GARCH
Engle (Citation2002) introduced a dynamic correlation model known as Dynamic Conditional Correlation-Generalized Autoregressive Conditional Heteroskedasticity (DCC-GARCH). This model is a development of the GARCH model previously introduced by Bollerslev (Citation1986). The DCC-GARCH model accommodates the time-varying conditional correlation matrix with the following formula:
Pt = (diag(Qt))−1/2 Qt(diag(Qt))−1/2 /
Qt = () is a positive symmetric definite matrix based on the following formula:
In the equation, α and β are non-negative scalars like α + β < 1, while is the unconditional correlation matrix (2 X 2) from standard errors ηt. Conditional variance is determined along with the GARCH process (1,1). Engle (Citation2002) suggested that the DCC specification did not encounter any problem in model estimation.
The DCC-GARCH model will produce DCC, conditional variance, and conditional covariance that can be used as input in portfolio calculations. To calculate optimal proportions in portfolios and hedge ratios as suggested by Kroner and Ng (Citation1998), Robiyanto et al. (Citation2017), and Susilo et al. (Citation2020), the following formula was used:
=
is the conditional variance of the return of precious metals,
is the conditional variance of the return of the stock market and
is the conditional covariance between the return of the precious metal and the return of the stock market in t.
The calculation of hedging effectiveness (HE) was performed with the formula first introduced by Ku et al. (Citation2007) and had been used in a research carried out by Robiyanto et al. (Citation2021):
HE =
Variancehedged is the return variance of precious metal-stock portfolio and Varianceunhedged is the return variance of stock portfolio. The higher the HE, the better the portfolio risk-decreasing will be, implying that the strategy has better hedging ability.
Meanwhile, the optimal hedge ratio was calculated using the following formula:
is optimal hedge ratio, while
and
are conditional volatility of precious metal returns and conditional covariance between precious metal returns and stock market returns in t.
To calculate the return, the following formula was used:
Returnt =
The calculation of risk-adjusted return of the portfolio was carried out with the Sharpe ratio using the following formula introduced by Sharpe (Citation1966):
Sharpe Ratio =
and Treynor Ratio using the following formula:
Treynor Ratio =
Sortino ratio was calculated using the following formula:
is the downside deviation of the return rate of the stock market within a certain period of time. The formula to calculate
is as follows:
Finally, the omega ratio was calculated using the following formula:
F(x) = cumulative probability distribution
= probability of return above the threshold
= probability of return below the threshold
5. Results & discussion
5.1. Descriptive statistics and correlations
The descriptive statistics of return of Bitcoin, Ethereum, Ripple, gold, Sri-Kehati index and bonds, which were used as samples of this research and are presented in Table .
Table 1. Descriptive statistics
Table shows that Ethereum has the highest average return (0.674%), and Sri-Kehati Index has the lowest (0.000%). While Table shows constant correlations among instruments used in this study.
Table 2. Constant correlation among instruments
Table shows that mostly constant correlation among instruments with different asset classes relatively low, i.e. Sri-Kehati with cryptocurrencies, Sri-Kehati with gold (XAU), and Sri-Kehati with bond (ICBI). Similar condition also apply for bond with other instruments. This show that portfolio formulation eco-friendly stocks with other instruments from different asset classes is appropriate because they low correlated, the same also apply for bond. Some cryptocurrencies produce high correlations, it is very normal because they are in similar asset class, while this study do not formulate portfolios inter-cryptocurrencies.
Table shows dynamic correlation among portfolios’ instruments. In order to validate dynamic correlation among portfolios’ instruments, it is necessary to compare constant correlation and dynamic correlation among those instruments. Both average dynamic and constant correlations for instruments in formulated portfolios only slightly differ in minor differences or only minor discrepancies occurred, so dynamic correlations’ calculations results could be validated. Regarding DCC GARCH model specification, McCloud and Hong (Citation2011) stated for the high correlated data, the time-varying conditional correlations may have underestimated. However, data used in this study are low correlated data, so this problem has been eliminated.
Table 3. Constant and Dynamic Correlation among Portfolios’ Instruments
5.2. DCC-GARCH Analysis
The results of calculations using the DCC-GARCH analysis on the return of Sri-Kehati index combined with Bitcoin, Ethereum, Ripple, gold and bonds combined with Bitcoin, Ethereum, Ripple, and gold are presented in Table . While Figure shows the DCC between Sri-Kehati Index, Cryptocurrencies and Gold with their time-varying portfolio weight; and Figure shows the DCC between Bonds and Bitcoin, Ethereum, Ripple, Gold and with their time-varying portfolio weight.
Table 4. DCC-GARCH summary
Figure 1. DCC between Sri-Kehati Index, Cryptocurrencies and Gold with Their Time-Varying Portfolio Weight.
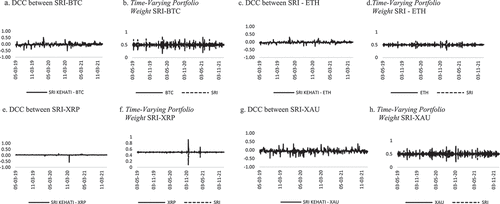
Figure 2. DCC between Bonds and Bitcoin, Ethereum, Ripple, Gold with Their Time-Varying Portfolio Weight.
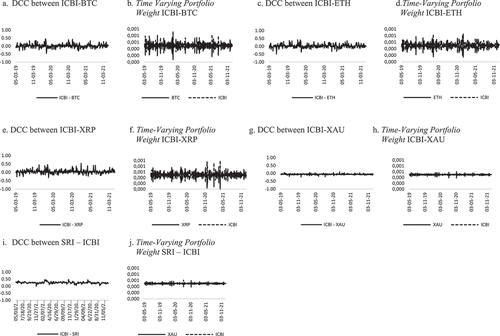
Table shows that the average value of dynamic portfolios containing Sri-Kehati index ranged from −0.064% to 0.032%; the lowest value was found in SRI-XAU, and the highest in SRI-XRP. Meanwhile, the average value of dynamic portfolio containing bonds ranged from −0.061% to 0.044%; ICBI-X AU has the lowest value, and ICBI-XRP has the highest. The results show that forming a dynamic portfolio between the Sri-Kehati index and Bitcoin, Ethereum, Ripple, gold and bonds with bitcoin, ethereum, ripple, and gold is the right decision. Due to the rapid changes in prices, investors need to diversify their assets to reduce risk and obtain optimal returns.
5.3. Optimal hedge ratio, hedging effectiveness and portfolio performance
The results of calculations of optimal hedge ratio, hedging effectiveness and portfolio performance are presented in Table .
Table 5. Optimal hedge ratio and hedging effectiveness
5.4. Optimal hedge ratio
Table shows that the average optimal hedge ratio of the dynamic portfolio between Sri-Kehati index, cryptocurrencies, and gold was −1.763%. SRI-XAU has the lowest average (−6.468%), and SRI-XRP has the highest (3.287%). It means that when investors own Sri-Kehati stocks, they must also purchase gold with a ratio of IDR 1 (stock): IDR 0.064 (gold). Meanwhile, when investors purchase Sri-Kehati stocks, they must also sell XRP with a ratio of IDR 1 (stock): -IDR 0.032 (XRP).
Table also shows that the average optimal hedge ratio of the dynamic portfolio between ICBI, cryptocurrencies, and gold was 5.490%; ICBI-XAU has the lowest average (−6.071%), and ICBI-SRI has the highest (24.701%). It means that when investors purchase bonds, they must also purchase gold with a ratio of IDR 1 (stock): IDR 0.060 (gold). Meanwhile, when investors purchase bonds, they must also sell Sri-Kehati stocks with a ratio of IDR 1 (stock): -IDR 0.247 (stocks). Adding trading costs in portfolio do not affect its hedging effectiveness and optimal hedge ratio, but trading cost will decrease portfolio return.
5.5. Hedging effectiveness
Table shows that the hedging effectiveness value of the dynamic portfolio between Sri-Kehati index, cryptocurrencies and gold ranged from 48.154% to 95.559%. SRI-XRP has the lowest value, which means that when adding XRP to a portfolio consisting of Sri-Kehati stocks, the risk can be reduced by 48.154%. Meanwhile, SRI-XAU has the highest value, which means that when adding gold to a portfolio consisting of Sri-Kehati stocks, the risk can be reduced by as much as 95.559%.
The hedging effectiveness value of the dynamic portfolio between bonds, cryptocurrencies and gold ranged from −47.206% to 98.441%. ICBI-SRI has the lowest value (−47.206%), which means that when investors purchase bonds, they must sell their Sri-Kehati stocks to reduce the investment risk by −47.206%; if they choose to add Sri-Kehati stocks to their bond portfolio, their investment risk will increase by 47.206%. While the highest optimal hedging effectiveness value of 98.441% is found in the ICBI-XAU portfolio, which means that adding gold to a portfolio consisting of bonds will help reduce the investment risk by as much as 98.441%. This matches the results of Yuliana and Robiyanto (Citation2022), which found that gold can be used as safe haven instrument. Meanwhile, the fact that bonds had hedging effectiveness value >0 showed that bonds could be considered low-risk fixed income instruments, supporting the results of Robiyanto et al. (Citation2019). In other words, investors may reduce their investment risk by combining bonds with gold in their portfolios rather than cryptocurrencies.
5.5. Portfolio performance comparison
The comparison between the performance of the Sri-Kehati index and bonds portfolio, combined with cryptocurrencies and gold, is presented in Table .
Table 6. Portfolios’ performance (Sri-Kehati index/bonds combined with cryptocurrencies/gold)
The comparison between the performance of portfolios consisting of the Sri-Kehati index and cryptocurrencies, gold and bonds is presented in Table .
Table 7. Summary of portfolios comparison
The results show that most of the portfolios hedged with cryptocurrencies, gold and bonds performed better than unhedged portfolios. It also shows that when calculated using the Sharpe ratio, Sortino ratio, Treynor ratio and Omega ratio, the dynamic portfolio between the Sri-Kehati index and cryptocurrencies has the highest value, while there is relatively no significant difference between the Jensen ratios of unhedged and hedged portfolio. In other words, combining Sri-Kehati index with cryptocurrencies in a portfolio will help reduce its investment risk, further supporting the results of Gunawan and Anggono (Citation2021); Susilo et al. (Citation2020), which found that cryptocurrencies could be used as hedging instruments. So, in term of to boost portfolio performance, investor should add either cryptocurrency or gold in their portfolio. This study also found that trading costs due to trading actively could affect portfolio performance, this is inline with Barber and Odean (Citation2000).
6. Conclusion and recommendation
The study aims to review the performance of cross-asset portfolios between eco-friendly stocks (like those constituting Sri-Kehati index in Indonesia) with cryptocurrencies and bonds by using the dynamic portfolio formulation method. The results of this study indicate that investors may reduce investment risk by adding Ripple and gold into a portfolio consisting of Sri-Kehati stocks. In addition, this study also found that adding gold into a portfolio consisting of bonds may reduce its investment risk by 98.441%. Moreover, adding gold into either Sri-Kehati or bond portfolio can help reduce investment risk since gold is a safe-haven asset. However, investors should not add Sri-Kehati stocks into their bond portfolio since it may increase investment risk by 47.206%.
The findings also imply that investment managers and investors who have portfolios consisting of Sri-Kehati stocks and bonds may reduce investment risk and balance their portfolios by adding gold or cryptocurrencies separately, including Ripple (XRP), because each instrument could become a diversifier which enhance stock portfolio. Portfolio formulated in this study require investment managers and investors trade actively, so trading costs is considered in this study. The results show that including trading costs in portfolio do not affect its hedging effectiveness and risk, but affect portfolios’ performance.
However, it should be noted that from 2020 to mid-2021, the global economy suffered from the impact of the COVID-19 pandemic, which may affect the data somewhat. So, it is recommended that future research may dig deeper and analyze the data from before the pandemic, during the pandemic and after the pandemic. The data characteristic should also be considered to choose the best portfolio formulation method.
Availability of data and materials
The datasets used and/or analysed during the current study are available on Bloomberg Terminal.
Authors’ contributions
All the authors have made an equal contribution to this study.
Ethics approval and consent to participate
Not Applicable
Consent for publication
Not Applicable
Acknowledgements
The authors would like to thanks Direktorat Riset dan Pengabdian Masyarakat, Kementerian Pendidikan, Kebudayaan, Riset dan Teknologi (Directorate of Research and Community Service, Ministry of Education, Culture, Research and Technology), Indonesia for funding this study by Grant Number: 072/E5/PG.02.00.PT/2022 and 001/LL6/PL/AK.04/2022.
Disclosure statement
No potential conflict of interest was reported by the authors.
Additional information
Funding
Notes on contributors
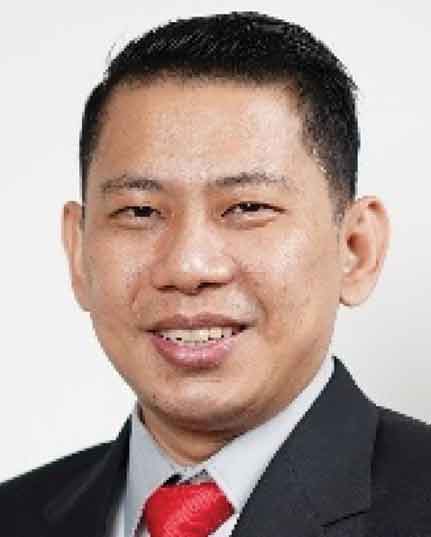
Robiyanto Robiyanto
Robiyanto Robiyanto is an Associate Professor in the Department of Management, Faculty of Economics and Business, Satya Wacana Christian University, Salatiga, Indonesia. He is active as an academic researcher; his research interests are capital market, commodity market, market efficiency, corporate governance, and portfolio management.
Andrian Dolfriandra Huruta
Andrian Dolfriandra Huruta is an Assistant Professor in the Department of Economics, Faculty of Economics and Business, Satya Wacana Christian University, Salatiga, Indonesia. His field of research is in macroeconomics, microfinance, applied econometrics, advanced econometrics, and international trade.
Budi Frensidy
Budi Frensidy is a Full Professor in Capital Market from Universitas Indonesia, Depok, Indonesia. His research interests are capital market, corporate governance, and corporate finance.
Ashalia Fitri Yuliana
Ashalia Fitri Yuliana is a Research Associate at PT Samuel Sekuritas Indonesia, Jakarta, Indonesia. Her research interests are capital market, and corporate finance.
References
- Agyei-Ampomah, S., Gounopoulos, D., & Mzaouz, K. (2013). Does gold offer a better protection against sovereign debt crisis than other metals. Paper presented at the EFMA.
- Akhtaruzzaman, M., Boubaker, S., Lucey, B. M., & Sensoy, A. (2021). Is gold a hedge or a safe-haven asset in the COVID–19 crisis? Economic Modelling, 102, 102, 105588. https://doi.org/10.1016/j.econmod.2021.105588
- Arouri, M. E. H., Lahiani, A., & Nguyen, D. K. (2015). World gold proces and stock return in China: Insights for hedging and diversification strategies. Economic Modelling, 44, 273–14. https://doi.org/10.1016/j.econmod.2014.10.030
- Bahloul, S., Mroua, M., & Naifar, N. (2021). Are islamic indexes, bitcoin and gold, still “safe-haven” assets during the COVID-19 pandemic crisis? International Journal of Islamic and Middle Eastern Finance and Management. https://doi.org/10.1108/IMEFM-06-2020-0295
- Barber, B. M., & Odean, T. (2000). Trading is hazardous to your wealth: The common stock investment performance of individual investors. The Journal of Finance, 55(2), 773–806. https://doi.org/10.1111/0022-1082.00226
- Baur, D. G., & Lucey, B. M. (2010). Is gold a hedge or a safe haven? An analysis of stocks, bonds and gold. The Financial Review, 45(2), 217–229. https://doi.org/10.1111/j.1540-6288.2010.00244.x
- Baur, D. G., & McDermott, T. K. J. (2012). Safe haven assets and investor behaviour under uncertainty. Working Paper. UTS, School of Business, Finance Discipline Group. Sydney.
- Bollerslev, T. (1986). Generalized autoregressive conditional heteroscedasticity. Journal of Econometrics, 31(3), 307–327. https://doi.org/10.1016/0304-4076(86
- Conlon, T., Corbet, S., & McGee, R. J. (2020). Are cryptocurrencies a safe haven for equity markets? An international perspective from the COVID-19 pandemic. Research in International Business and Finance, 54, 101248. https://doi.org/10.1016/j.ribaf.2020.101248
- Eimer, S. (2011). Adaptive portfolio theory: Hedging against correlations. Paper presented at the The 2011 Industrial Engineering Research Conference, Norcross.
- Engle, R. F. (2002). Dynamic conditional correlation: A simple class of multivariate generalized autoregressive conditional heteroskedasticity models. Journal of Business & Economic Statistics, 20(3), 339–350. https://doi.org/10.1198/073500102288618487
- Filis, G., Degiannakis, S., & Floros, C. (2011). Dynamic correlation between stock market and oil prices: The case of oil-importing and oil-exporting countries. International Review of Financial Analysis, 20(3), 152–164. https://doi.org/10.1016/j.irfa.2011.02.014
- Frensidy, B. (2016). The performance of undiversified portfolio in Indonesia stock exchange. Journal of Applied Business Research (JABR), 32(4), 1041–1048. https://doi.org/10.19030/jabr.v32i4.9720
- Frensidy, B., Utama, S., & Prijadi, R. (2017). An analysis of diversification practice of domestic individual investors in the Indonesia stock exchange. International Journal of Applied Business and Economic Research, 15(19), 137–148. https://serialsjournals.com/abstract/25463_9.pdf
- Gunawan, M., & Anggono, A. H. (2021). Cryptocurrency safe haven property against Indonesian stock market during COVID-19. Journal of Economics, Business, & Accountancy Ventura, 24(1), 121–138. https://doi.org/10.14414/jebav.v24i1.2661
- Indriastuti, M., Chariri, A., McMillan, D., & McMillan, D. (2021). The role of green investment and corporate social responsibility investment on sustainable performance. Cogent Business & Management, 8(1), 1–21. https://doi.org/10.1080/23311975.2021.1960120
- Katzke, N. (2013). South African sector return correlations: Using DCC and DCC multivariate GARCH techniques to uncover the underlying dynamics. Working Paper 17/2013, Stellenbosch University, Department of Economics.
- Kroner, K. F., & Ng, V. K. (1998). Modelling asymmetric comovements of assets returns. Review of Financial Studies, 11(4), 817–844. https://doi.org/10.1093/rfs/11.4.817
- Ku, Y.-H.-H., Chen, H.-C., & Chen, K.-H. (2007). On the application of the dynamic conditional correlation model in estimating optimal time-varying hedge ratios. Applied Economics Letters, 14(7), 503–509. https://doi.org/10.1080/13504850500447331
- Kumar, D. (2014). Return and volatility transmission between gold and stock sectors: Application of portfolio management and hedging effectiveness. IIMB Management Review, 26(1), 5–16. https://doi.org/10.1016/j.iimb.2013.12.002
- Kurniatama, G. A., Robiyanto, R., Sasongko, G., & Huruta, A. D. (2021). Determinants of corporate social responsibility: Empirical evidence from sustainable and responsible investment index. Quality - Access to Success, 183(22), 55–61. https://www.srac.ro/calitatea/en/arhiva/2021/QAS_Vol.22_No.183_Aug.2021.pdf
- McCloud, N., & Hong, Y. (2011). Testing the structure of conditional correlations in multivariate garch models: A generalized cross-spectrum approach. International Economic Review, 52(4), 991–1037. https://doi.org/10.1111/j.1468-2354.2011.00657.x
- Naffa, H., Fain, M., & Trinidad Segovia, J. E. (2020). Performance measurement of ESG-themed megatrend investments in global equity markets using pure factor portfolios methodology. PLoS ONE, 15(12), e0244225. https://doi.org/10.1371/journal.pone.0244225
- Ogata, H. (2012). Optimal Portfolio Estimation for Dependent Financial Returns with Generalized Empirical Likelihood. Advances in Decision Sciences, 1–8. https://doi.org/10.1155/2012/973173
- Ouchen, A. (2021). Is the ESG portfolio less turbulent than a market benchmark portfolio? Risk Management. https://doi.org/10.1057/s41283-021-00077-4
- Putra, M. P. S., Atahau, A. D. R., & Robiyanto, R. (2018). Cross–asset class portfolio between gold and stocks in Indonesia. Economic Journal of Emerging Markets, 10(1), 69–81. https://doi.org/10.20885/ejem.vol10.iss1.art8
- Raza, N., Ali, S., Shahzad, S. J. H., Rehman, M. U., & Salman, A. (2019). Can alternative hedging assets add value to Islamic-conventional portfolio mix: Evidence from MGARCH models. Resources Policy, 61, 210–230. https://doi.org/10.1016/j.resourpol.2019.02.013
- Robiyanto, R. (2017). Performance evaluation and risk aversion rate for several stock indices in Indonesia stock exchange. Jurnal Manajemen Dan Kewirausahaan, 19(1), 60–64. https://doi.org/10.9744/jmk.19.1.60-64
- Robiyanto, R. (2018). Testing of the Gold’s role as a safe haven and hedge for sharia stocks in Indonesia. Al-Iqtishad: Jurnal Ilmu Ekonomi Syariah, 10(2), 255–266. https://doi.org/10.15408/aiq.v10i2.6527
- Robiyanto, R., Ernayani, R., & Ismail, R. S. (2019). Formulation of a dynamic portfolio with stocks and fixed-income instruments in the Indonesian capital market. Organizations and Markets in Emerging Economies, 10(1), 132–146. https://doi.org/10.15388/omee.2019.10.00007
- Robiyanto, R., Nugroho, B. A., Huruta, A. D., Frensidy, B., & Suyanto, S. (2021). Identifying the role of gold on sustainable investment in Indonesia: The DCC-GARCH Approach. Economies, 9(3), 119. https://doi.org/10.3390/economies9030119
- Robiyanto, R., Wahyudi, S., & Pangestuti, I. R. D. (2017). The volatility–variability hypotheses testing and hedging effectiveness of precious metals for the Indonesian and Malaysian capital markets. Gadjah Mada International Journal of Business, 19(2), 167–192. https://doi.org/10.22146/gamaijb.26260
- Salvatori, E. G., Robiyanto, R., & Harijono, H. (2020). An analysis of the relationship between earnings and corporate taxes on dividend policy of companies in SRI-KEHATI Index. Journal of Management and Entrepreneurship Research, 1(1), 1–12. https://doi.org/10.34001/jmer.2020.6.01.1-1
- Shahzad, S. J. H., Bouri, E., Roubaud, D., Kristoufek, L., & Lucey, B. (2019). Is Bitcoin a better safe-haven investment than gold and commodities? International Review of Financial Analysis, 63, 322–330. https://doi.org/10.1016/j.irfa.2019.01.002
- Sharpe, W. F. (1966). Mutual Fund Performance. Journal of Business, 39(S1), 119–138. https://www.jstor.org/stable/2351741
- Susilo, D., Wahyudi, S., Pangestuti, I. R. D., Nugroho, B. A., & Robiyanto, R. (2020). Cryptocurrencies: Hedging opportunities from domestic perspectives in southeast Asia emerging markets. Sage Open, 10(4), 215824402097160. https://doi.org/10.1177/2158244020971609
- Utomo, M. N., Wahyudi, S., Muharam, H., & Taolin, M. L. (2018). Strategy to improve firm performance through operational efficiency commitment to environmental friendliness: Evidence from Indonesia. Organizations and Markets in Emerging Economies, 9(1), 62–85. https://doi.org/10.15388/omee.2018.10.00004
- Vukovic, D., Maiti, M., & Grigorieva, E. (2021). COVID-19 pandemic: Is the crypto market a safe haven? The impact of the first wave. Sustainability, 13(15), 15. https://doi.org/10.3390/su13158578
- Yuhanitha, N., & Robiyanto, R. (2021). Cryptocurrencies as a hedge and safe haven instruments during Covid-19 Pandemic. Journal of Accounting and Strategic Finance, 4(1), 13–30. https://doi.org/10.33005/jasf.v4i1.129
- Yuliana, A. F., & Robiyanto, R. (2022). Revisit the dynamic portfolio formation between gold and stocks in Indonesia in the period before and during the COVID-19 pandemic. Journal of Accounting and Strategic Finance, 5(1), 1–21. https://doi.org/10.33005/jasf.v5i1.161
- Zinecker, M., Balcerzak, A. P., Faldzinski, M., Pietrzak, M. B., & Meluzin, T. (2016). Application of DCC-GARCH model for analysis of interrelations among capital markets of poland, czech republic and Germany. Paper presented at the Proceedings of the International Scientific Conference Quantitative Methods in Economics Multiple Criteria Decision Making XVIII, Vratna. Institute of Economic Research. http://econpapers.repec.org/bookchap/pesecchap/12.htm
- Zulkafli, A. H., Ahmad, Z., & Muttaqin, E. E. (2017). The performance of socially responsible investments in Indonesia: A study of the Sri Kehati Index (SKI). Gadjah Mada International Journal of Business, 19(1), 59–76. https://doi.org/10.22146/gamaijb.17959