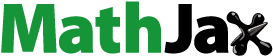
Abstract
Microfinance institutions must understand what influences their clients’ choice of credit source in order to design and provide adequate and appropriate credit facilities to their clients. This paper explores the determinants of the choice of credit source among beneficiaries of microfinance systems in the Upper West Region of Ghana. An interview guide and a questionnaire were used to collect data. The study employed the multinomial probit model to analyse the data. The study revealed that gender, arable crop farming, household size, dependency ratio, access to microfinance information, repayment period, group size, interest rate, distance, loan amount, borrowing experience, household assets, and household members employed were the main determinants of the choice of credit source among beneficiaries. The study recommends that the Bank of Ghana, through the Apex Bank, standardise repayment periods and procedures such that banks compete on innovative methods of sourcing borrowers, which could result in efficiency in the lending industry.
1. Introduction
Households obtain credit through formal and informal lenders. Formal loans are almost entirely for production and asset accumulation, while informal loans are used for consumption smoothening (Barslund & Tarp, Citation2008). While formal sources of credit are often preferred and recommended over indigenous sources, the latter is more convenient. This difference accounts for the existence of the indigenous financial sector in even developed nations (Fridell, Citation2007). To meet an unfulfilled demand for financial services by the poor and micro-entrepreneurs, a range of microfinance institutions (MFIs) has emerged over time in Africa. These MFIs are designed on two forms of indigenous financial mediation in poor communities—local savings groups and moneylenders. These institutions do this by expanding, formalising, and modernising the services and transactions offered by them (Harper, Citation1998). Some of these institutions focus only on making credit available, others are involved in both deposit and credit facilities, and some are interested only in deposits from their clients.
Members of low-income communities desiring to have credit for developing or starting income-generating activities are offered access to financial and non-financial services from microfinance institutions. As the name signifies, the savings and loans of the individual poorer clients are small. Microfinance came into being from the appreciation that micro-entrepreneurs and some poorer clients can be “bankable”; that is, they can repay both principal and interest on time and also make savings, provided financial services are tailored to suit their needs (Ramkumar et al., Citation2015). In addition, microfinance is being advocated as a development strategy to help alleviate poverty in many developing countries (Ferdous, Citation2021). The term microfinance comprises two words: micro and finance, which signify small credit. However, empirically, the concept of microfinance goes beyond the provision of small credit to poor people. Microfinance provides financial services to the economically active poor hitherto un-served by the mainstream financial service providers. Microcredit is commonly defined in terms of loan amount as a percentage of average per capita income (Abiola, Citation2011). The Bank for International Settlements (Citation2010) regards microfinance as the provision of financial services in limited amounts to low-income persons and small informal businesses. Microfinance is generally an umbrella term that refers to a broad range of services such as deposits, loans, payment services, money transfers and insurance to poor and low-income households and their micro-enterprises (Christen, Citation1997; Khawari, Citation2004; Otero, Citation1999; Robinson, Citation2001).
Poor people living in both rural and urban settings are vastly unable to obtain financial services from the formal financial sector for some reasons (Littlefield & Rosenberg, Citation2004). These reasons include; lack of collateral by the poor, limited debt capacity of the poor, inadequate information regarding the use of loans by the poor, limited ability to repay loans, and the high transaction costs associated with servicing many poor clients with small loans. Thus, formal financial institutions usually target the rich because they have greater capacity and propensity to repay loans and maintain savings. This evidence is supported by Kilic et al. (Citation2017), who indicated that almost 90% of borrowers in developed countries access credit from formal financial institutions, whilst about 60% of borrowers access credit from the indigenous financial institutions in developing countries. In 2019, global estimates revealed that merely 15.7% of individuals claimed to have gotten credit from a formal financial institution in the previous 12 months (Alliance for Financial Inclusion, Citation2022). Credit to micro and small businesses is on the low side as only small numbers (3.4%) sourced credit from formal commercial banks and (1.1%) from semi-formal financial institutions (Osei-Assibey et al., Citation2012). Microfinance appears to overcome these barriers by providing adequate financial services to disadvantaged clientele such as rural farmers and small business owners. A range of methods, including Rotating Savings and Credit Associations (ROSCAs), traditional kinship networks to NGOs and development projects, and funded by the formal and indigenous financial sectors, and international and domestic donors have been used in the microfinance sector in Africa (Bank of Ghana, Citation2007).
The factors that influence the demand for credit by households, farmers, and small and medium-sized firms (SMEs), among others, have been discussed in previous studies (Anang et al., Citation2015; Asiamah et al., Citation2021; Baffoe & Matsuda, Citation2015; Dlamini & Mohammed, Citation2018; Hananu et al., Citation2015). A few other studies have examined the determinants of credit source among farm households and owners of SMEs (Dlamini & Mohammed, Citation2018; Fufa, Citation2016). These studies divulge fascinating determinants such as borrower characteristics, environmental factors and lender attributes (Asiamah et al., Citation2021). The current study differs from previous studies by examining the determinants of choice of credit sources (i.e., formal, semi-formal and indigenous) among microfinance beneficiaries. The findings reveal that the common factors influencing the choice of formal and semi-formal credit sources among beneficiaries of MFIs are gender, household size, repayment period, dependency ratio, group size, interest rate, distance, loan amount, borrowing experience, household assets, and employment status of household members. Differences in the determinants of beneficiaries’ choice of credit source are discovered, as arable crop farming influences formal credit, while access to microfinance information drives the semi-formal credit source. The findings of this study will have significant implications for designing and delivering suitable and appropriate credit facilities to microfinance beneficiaries.
The remainder of this paper proceeds as follows: the subsequent section reviews literature on microfinance systems in Ghana and theoretical and empirical works on the subject. Section 3 presents the research methodology, which includes the sampling procedure, sample size determination, data collection techniques and model specification. Section 4 discusses descriptive statistics and empirical results. The final section concludes by highlighting the policy implications of the findings, suggestions for further research and the study limitation.
2. Review of the literature
2.1. Microfinance systems: the Ghanaian context
In Ghana, microfinance services are provided to the urban and rural poor through indigenous, semi-formal, and formal financial institutions (Gallardo, Citation2002). These financial institutions differ in how they operate, the services they offer, and the people they serve (Aryeetey, Citation2008). Formal financial institutions are those that have been incorporated under the Companies Code 1963 (Act 179) and subsequently licensed by the Bank of Ghana (BOG) under either the Financial Institutions (Non-Banking) Law 1993 (PNDCL 328) or the Banking Law 1989 (PNDCL 225). These institutions provide financial services under Bank of Ghana regulations (Bank of Ghana, Citation2007). The credit reporting Act, 2007 (Act 726) has also given the Bank of Ghana the authority to monitor the activities of the financial institutions and microfinance institutions to ensure compliance with the prescribed standards and requirements (Bank of Ghana, Citation2017).
Credit unions and Non-Governmental Organisations (NGOs) are part of the semi-formal system since, despite being technically registered, they are unlicensed by the Bank of Ghana. The poverty focus of NGOs leads them to comparatively deeply reach out to poor clients using microfinance methodologies, though to a greater extent on a limited scale. They often use external or donor funds for microcredit since they are not licensed to take deposits from the public. On the other hand, credit unions are registered by the Department of Cooperatives as cooperative thrift societies that can grant loans and accept deposits from only their members (Bank of Ghana, Citation2007). The indigenous financial sector is commonly associated with the various institutions of finance that operate outside the scope of conventional banking and government regulation. It covers a range of activities known as Susu, including Rotating Savings and Credit Associations, individual savings collectors, and savings and credit “clubs” run by the local communities. Other stakeholders in this sector include trade creditors, moneylenders, self-help groups, and personal loans from friends and relatives. Not too long ago, the activities of indigenous financial service providers seemed too remote and irrelevant to many experts. However, as the importance of these indigenous financial service providers is recognised, opinions are quickly changing (Tuffour, Citation2002).
2.2. Financial inclusion and household welfare
Financial inclusion has generally been identified as one of the crucial determinants of household economic activity and pro-poor growth in developing countries. When households have access to credit, they may be able to acquire productive capital, which could improve their capacity to generate income, save and invest for better welfare, as argued by Beck and Demirguc-Kunt (Citation2005). There is thus a broad consensus that financial inclusion is a pre-requisite for growth, poverty reduction and reduction of income inequality. It facilitates savings mobilisation, increases efficiency of investment, and allows households to accumulate both human and physical assets (Pande et al., Citation2012; Swamy, Citation2014). Therefore, theoretically it is possible to identify the various channels through which access to credit could lead to a reduction in poverty or an improvement in welfare. First, access to credit could improve the welfare of households through an increase in saving opportunities which could also increase households’ savings. An increase in savings will potentially raise the number of investible resources, which can positively impact household welfare (Ashraf et al., Citation2006). Second, financial inclusion can improve credit penetration when the aggregate level of savings increases, thus increasing the probability of excluded households getting access to credit which could impact the households’ welfare since they can invest in education, health and nutrition. Third, by improving the availability of loanable funds, financial inclusion may more generally lead to a diversification of the loan portfolios of financial intermediaries, reducing the average credit risk of loans, which then enhances the recycling of funds. This situation will lead to an increase in overall economic activity and hence an improvement in household welfare.
In the empirical literature, it has been established that financial inclusion can improve household savings, education, investment, healthcare, and food security, and thus the improvement in welfare (Baidoo et al., Citation2020; Iddrisu & Danquah, Citation2021; Machili, Citation2021; Omar & Inaba, Citation2020; Sakyi‐Nyarko et al., Citation2022; Sekabira & Qaim, Citation2017; Wieser et al., Citation2019).
2.3. Empirical literature
Empirical studies on the determinants of credit access are numerous and diverse. Some previous research examines the determinants of credit access for varied economic units, including households, farmers, and small and medium enterprises (SMEs), among others (Anang et al., Citation2015; Asiamah et al., Citation2021; Baffoe & Matsuda, Citation2015; Dlamini & Mohammed, Citation2018; Hananu et al., Citation2015). Methods commonly employed include logit, probit, OLS regression and Heckman Selection model models to study the determinants of credit access and loan amount. By carefully examining the previous studies, we can divide them into three categories. The first body of literature examines factors that affect credit access generally without highlighting different sources of credit. Using household level data and the probit model, Baffoe and Matsuda (Citation2015) acknowledged the inadequate credit access in the rural areas in developing countries. Their analysis revealed that livelihood diversification, household productivity, savings accounts and household size were significant factors influencing households’ ability to access credit in Ehiaminchini in the Fanteakwa district in the Eastern region of Ghana. In another study conducted in Ghana by Sekyi (Citation2017), gender, age, farming and trading occupations, credit history, and household income were revealed as significant drivers of rural households’ access to credit. Additionally, the loan amount was significantly predicted by gender, education, marital status, trading activity, employment in the formal sector, distance, and credit source. At the farm level, Anang et al. (Citation2015) investigated the determinants of smallholder farmers’ access to credit in Northern Ghana. Their study revealed that gender, household income, farm capital, improved technology adoption, contact with extension, the location of the farm and awareness of lending institutions are the factors influencing access to credit by smallholder farmers in the Northern region of Ghana. The authors further found gender, household size, farm capital, cattle ownership and improved technology adoption as significant factors influencing loan size. Hananu et al. (Citation2015) focused on factors influencing farm households’ demand for agricultural credit. The findings of the logistic regression analysis showed that factors including age, education, group participation, and credit source significantly influence demand for credit positively. Ahmad et al. (Citation2022) investigated the effects of entrepreneurial-specific traits on SME access to financing. The study observed that, having entrepreneurial personality traits is crucial for securing financing from Pakistani banks and other financial institutions. Age and educational attainment were shown as crucial factors for obtaining bank financing. On the other hand, having SME skills and being a woman did not significantly affect an entrepreneur’s ability to obtain bank financing. The study also demonstrates how entrepreneurs’ personality influences their ability to obtain bank financing and the necessity of developing strategies and policies to strengthen these traits through education, development, and personality development for the effective operation of the SME sector in Pakistan.
The second strand of literature investigates the factors that influence credit access by distinguishing between the various credit sources. The first group under this category examines formal, semi-formal or informal credit sources solely. For instance, in South Africa, poor households’ access to formal credit was examined by Biyase and Fisher (Citation2017). The Heckman Selection model results revealed factors such as the household head’s age, race, educational attainment, gender, employment, and geographic area influenced a household’s propensity to borrow. Kiros and Meshesha (Citation2022) investigated the factors determining farmers’ access to formal credit in Ethiopia. The findings indicate that farmers’ access to formal finance is significantly influenced age, sex of household head, family size, extension contacts, off-farm income, interest rate, lending procedure, group lending and rapid repayment period. In a study conducted in Pakistan, Chandio et al. (Citation2020) discovered that smallholder farmers’ demand for formal credit was positively and significantly influenced by formal education, farming experience, size of landholdings, road access, and extension contacts. According to Mamuye (Citation2021), age of the household head, family size, prior experience using formal credit, total livestock ownership, farmers’ perceptions of group formation, and the distance that households travelled to the lending institution were all significant determinants of smallholder farmers’ participation in formal credit in the Mojana Wodera District, Ethiopia. Departing from much of the prior literature, Sekyi et al. (Citation2014) utilised bivariate probit model to account for potential unobserved heterogeneity in SMEs’ choice of credit sources. The study found evidence that the unobserved factors influencing participation and credit source preference was positively correlated. The findings showed that age and its squared term and education significantly influenced entrepreneurs’ preference for formal credit sources. Age, household size, education, income, and wealth were also found to have a significant impact on entrepreneurs’ decision to participate in the credit market. Mukhwami et al. (Citation2022) conducted research in Kenya on the factors that influence rural farm households’ semi-formal credit participation. The authors found that factors such as farmers experience, the occupation of the household head, group membership, distance from the source of the credit, distance to the closest market, and access to financial training had a significant impact on household participation in semi-formal credit. Regarding informal credit source, Adugna and Heidhues (Citation2000) investigated the determinants of farm households’ access to informal credit in Ethiopia. Their study showed that informal credit access was more likely to be influenced by the resources farm households own (land, oxen and small ruminants), their health condition, dependency rate and land tenure arrangements. In another study of an informal financial institutions, Mwonge and Naho (Citation2021) looked into the factors that affect smallholder farmers’ demand for financing from micro financial institutions. Their research showed that factors such as the respondents’ age, gender, number of years of schooling, household size, distance, awareness, collateral, type of crops, farm size, contact with extension services, membership to economic farm groups, location of the farm, and interest rate affected smallholder farmers’ access to agricultural credit positively. However, the respondent’s gender, location, collateral, and interest rate had negative effects on smallholder farmers’ decisions to request and obtain agricultural financing from micro financial institutions.
The final group of studies under this review distinguish between the various credit sources and examines the determinants of credit access for at least two credit sources. These empirical works mostly employed multinomial logit or probit models. Kumar et al. (Citation2007) studied the performance of rural credit and factors affecting the choice of credit sources in India. The study reported that age significantly affects borrowing from institutional sources positively and negatively influences borrowing from non-institutional sources, gender positively affects borrowing from both institutional and non-institutional sources, male-headed households, larger household size and a higher level of education, increase the probability of having loans from the institutional sources. Fufa (Citation2016) investigated the determinants of credit access and choice of credit source using cross-sectional data on Ethiopian entrepreneurs. The author grouped the factors that determined credit choices into three categories, namely external environment (access to market, work premises, firm’s location, and business information), owner characteristics (risk, marital status) and business characteristics (firm size, firm capital, and business plan). The author identified credit sources in his study as formal, informal, NGO and internal financing. They found that firm size, firm age, corruption and firm locations were significant factors in determining the choice of credit source. Dlamini and Mohammed (Citation2018) studied the determinants of credit source choice among SMEs in the agricultural subsector using secondary data in Eswatini. The results indicated that keeping financial records, capital size required to start a business, the size of business, age of the business owner, and interest rates are significant factors that influence the choices of agricultural SME owners between informal, semi-formal and formal credit providers. Also, they found that entrepreneurs who did not keep records were more likely to use informal sources of credit. Odu et al. (Citation2011) used data on rice farmers in Nigeria to study the determinants of rice farmers’ access to credit in Niger state. They categorised the source of credit into formal and informal sources. Using multinomial logit models, they found that access to formal sources of credit was determined by factors including experience in rice farming, expenses on fertiliser input and rice income, while for the informal credit source, factors which influenced their access were gender, duration of village residency, experience in rice farming and expenses on fertiliser input. Brewer et al. (Citation2019) used data from the Kansas Farm Management Association to study farmers’ choice of credit among the farm credit system, commercial banks and non-traditional leaders. They found that the age of the farmer, debt/asset ratio, inverse current ratio, return on assets, number of dependents, wage income, rent and royalties were significant determinants of the choice of credit source.
A core issue that arises from the literature on credit access is the fact that no attempt, to the best of our knowledge, has been made to model the determinants of choice of credit source among clients of microfinance systems. Few studies have looked at the determinants of credit source among entrepreneurs (Dlamini & Mohammed, Citation2018) and (Fufa, Citation2016). Investigating the factors that influence clients of microfinance systems’ credit source preferences are crucial in the development of appropriate policies for the microfinance subsector. Thus, this study attempts to fill this gap in the body of literature.
3. Methodology
3.1. Sampling approach/procedure
The study employed a multistage sampling procedure to draw a representative sample. The technique consists of selecting districts and municipalities, microfinance institutions and beneficiaries. In the first stage, the Sissala East District, Wa Municipal, and the Lawra District were selected through simple random sampling out of the eleven districts and municipalities in the region. In the second stage, Nandom Rural Bank, Sissala Rural Bank and the Wa branch of the Nandom Rural Bank were selected as formal MFIs. The Wa Co-operative Credit Union and the Sissala East Co-operative Credit Union were semi-formal MFIs selected, while Susu associations were selected as indigenous MFIs. The rationale for using these institutions was based on the fact that they are the predominant microfinance systems in the selected districts and municipalities. In the third and final stage, simple random sampling was employed to choose beneficiaries of microfinance systems. Because the target population is the beneficiaries of formal, semi-formal, and indigenous microfinance systems, a simple random selection technique was employed to choose 120 people from each system. The application of the simple random sampling to the beneficiaries was relatively simple since a detailed list of these beneficiaries was obtained at the various institutions.
3.2. Sample size determination
This study used the Krejcie and Morgan (Citation1970) table to estimate the sample size for a known population. From the table, the sample size corresponding to a population of 5,000 is 357. Data were collected from 360 beneficiary households comprising 120 beneficiaries drawn from each of the three microfinance systems. Beneficiaries of the informal microfinance system were used as the control group or base category.
3.3. Sources and techniques of data collection
This study made use of primary data. Respondents were selected from clients or beneficiaries of indigenous, formal and semi-formal microfinance systems in the Upper West Region of Ghana. The use of these three groups was to make possible a comparative analysis of the three major microfinance systems operating in the region. A household survey was conducted to collect data from the beneficiaries of the three microfinance systems using a semi-structured questionnaire. Ten per cent of the study population participated in a pilot research to test for the survey instrument’s readability and comprehensibility. The questionnaire was updated after pretesting. The authors oversaw and monitored the data-gathering process, regularly checking all completed questionnaires for consistency and missing information. Each completed questionnaire was examined to ensure all the questions were correctly answered to guarantee the validity and reliability of the data. The study employed Cronbach alpha to test for the reliability of the survey instruments. We obtained an alpha coefficient of 0.82, suggesting that the variables had relatively high internal consistency.
3.4. Model specifications
The econometric properties of the variable of interest, such as the distribution of the dependent variable, have an enormous role in deciding which analytical method to use. The linear models are used when response variable are continuous variables. Many statisticians believe that when response variable are categorical, non-linear models such as logit, probit, multinomial regression models are most appropriate (El-Habil, Citation2012). The categorical response variable in the binary logit and probit regression models is assumed to have just two values, typically 1 for success and 0 for failure. The multinomial (logit or probit) regression models are used where the response variable is composed of more than two levels or categories. In other words, multinomial regression models are an extension of binary regression models allowing more than two categories of the dependent or outcome variable. By simultaneously estimating the log odds of three or more contrasts, the multinomial regression model allows for the comparison of several contrasts (Garson, Citation2009). This discussion reveals that application of linear models and non-linear models such as binary probit are inappropriate for this study. The use of the multinomial probit model for the analysis of the determinants of the choice of credit source of beneficiaries is informed by the fact that the dependent variable is categorical, representing the choices of beneficiaries of the three microfinance systems. The multinomial probit model is popular because it does not require the assumption of Irrelevant Alternative Independence.
The decision under this situation is the choice beneficiaries of the microfinance systems make between the microfinance systems that provide microfinance services to households. The decisions are unordered and are therefore motivated by the random utility model. This is the case where the consumer faces J number of choices. This is illustrated as follows (Cameron & Trivedi, Citation2005; Greene, Citation2003):
Given that yields the maximum utility to the consumer, the probability model for the choice is:
The utility obtained can be decomposed into observed and unobserved components expressed as:
Where is the utility of the
individual choosing alternative
,
is the deterministic component of the utility and
is the error term. Following from Green (2003), the conditional probability of the multinomial probit is specified as:
Where and
The base category (which is the indigenous microfinance source) is used to compare other choices by restricting the parameters of the base category to zero. The other microfinance sources are semi-formal and formal. This is because the J parameter vector is required to estimate J + 1 probability.
The J log-odd ratios are computed with the relation:
Based on Equationequation 5(5)
(5) the empirical model to be estimated is specified as:
Where is the outcome variable,
, vector of parameters to be estimated;
, vector of explanatory variables;
, random disturbance term. Estimation of the multinomial probit is most often done by the maximum likelihood method. The log-likelihood function:
The dummy variable takes the value of 1 if an
individual has chosen alternative
and 0 otherwise.
Taking the first order derivative of Equationequation (7)(7)
(7) yields:
The marginal effect is specified as:
This implies that a unit change in will change the probability of demand for a
source of microfinance system by
. Table presents the measurement and descriptive statistics, whereas Table shows the expected signs of the variables used in the analysis.
Table 1. Variables, measurement and descriptive statistics
Table 2. A priori expectations of variables used in the study
4. Results and discussion
4.1. Descriptive statistics
The mean of the dependent variable is 0.333, implying that 33.3% of the respondents were selected from the three beneficiaries’ microfinance systems (see, Table ). This is because the three types of microfinance had an equal representation of 120 beneficiaries under the study. The mean value of gender is 0.383, meaning 38.3% and 61.7% of the sampled beneficiaries are males and females, respectively. The mean amount of the loan is 1,160.40 and the age of the beneficiaries is 44 years. For household size and dependency ratio the averages were 5 and 1.25, respectively while years of schooling, group size, repayment period of current loan, and interest rate are 3.683, 13.922, 5.006 and 59.99, respectively. The results further reveal that 95.3% of the beneficiaries have access to microfinance information. Finally, the means for distance and estimated beneficiary income are 2.186 and 4,808.66, respectively.
4.2. Empirical results
Multinomial probit model is used in situations where the dependent variable has more than two categories. Each category is compared to the reference group in this study, the indigenous microfinance system. The result of the Wald test indicates that the model is statistically not equal to a null model. Thus, we reject the null hypothesis of all coefficients being equal to zero. All variables used in the model are jointly significant at 1% level. The results show that the probability of households accessing credit from a microfinance system is influenced to a great extent by gender, arable crop farming, household size, dependency ratio, group size, interest rate, access to microfinance information, repayment period, distance, loan amount, borrowing experience, household asset, and household members employed as shown in Table .
Table 3. Multinomial probit results of determinants of choice of microfinance system
The coefficient for gender is negative for the probability that males will choose to access credit from formal microfinance institutions but positive in the case of semi-formal institutions relative to the indigenous credit institution. It is statistically significant at 10% and 1% for formal and semi-formal microfinance systems, respectively. This result means that male beneficiaries are less likely to access credit from the formal microfinance system relative to the indigenous microfinance system. Specifically, the marginal effect predicts that males are 12.1 percentage points less likely to access credit from formal microfinance systems relative to the indigenous microfinance system. This finding may be explained by the fact that the majority of formal microfinance systems in Ghana frequently give preference to women when extending credit, decreasing the availability and accessibility of formal credit to men. This revelation contrasts the widely held view in the empirical literature that male beneficiaries often have a more risk-bearing ability that influences their relatively higher demand for credit from formal financial institutions (Kiros & Meshesha, Citation2022; Mpuga, Citation2010). However, male beneficiaries are more likely to opt for credit from semi-formal microfinance systems compared to indigenous microfinance systems. The marginal effect predicts that males are about 18.5 percentage points more likely to access credit from semi-formal microfinance systems compared to indigenous microfinance systems. This result can be linked to the semi-formal systems’ employment of the solidarity group lending approach, their proximity to rural communities, and the comparatively low-interest rates they charge.
The primary occupation of household members also influences the choice of credit. This variable coefficient is negative and statistically significant. Thus, beneficiaries whose primary occupation is arable crop farming are less likely to access credit from the formal microfinance system relative to the indigenous microfinance system. Arable crop farming is a risky venture, especially in rural settings that practice rain-fed agriculture. Borrowers who are arable crop farmers, therefore, self-exclude themselves from the formal credit market as they will perceive themselves to be risky and therefore do not meet the requirements for selection should they apply. Also, almost all arable crop farmers are small-scale farmers located in rural areas where informal financing is more prevalent, and this could lead them to patronize the informal finance schemes.
The estimated coefficient of household size is positive and statistically significant at a 10% level for the probability of households choosing formal microfinance systems relative to the indigenous microfinance system. The finding implies that as the household size increases, members are more likely to go in for loans from the formal microfinance system relative to the indigenous microfinance system. The marginal effect predicts that as household size increases by a person, the likelihood that a beneficiary household will access credit from a formal institution will increase by 13.4 percentage points relative to the choice of an indigenous microfinance system. This may be because larger households usually have some of their members freed from the normal daily activities to go through the long process of loan disbursement associated with formal microfinance institutions. Hence, larger households can access larger loans from the formal microfinance system. This revelation can also be credited to the fact that loan sizes are relatively more oversized in the case of formal credit institutions than indigenous sources. Since larger households may require larger loans to cater for their needs, they would prefer to access loans from the formal source relative to indigenous sources, where loans are usually smaller. The result is consistent with Silong and Gadanakis’s (Citation2020) findings. Also, household size is a negative determinant of the probability of choosing a semi-formal microfinance system as against an indigenous microfinance system. This result implies that beneficiaries will prefer loans from the indigenous microfinance system relative to the semi-formal microfinance system as household size increases. The marginal effect predicts that the probability of accessing credit from the semi-formal microfinance system decreased by 18.7 percentage points compared to the indigenous microfinance system. A plausible reason for this finding could be that as household size increases, members can readily form groups to access loans from indigenous sources, which thrive so much on group solidarity. However, Silong and Gadanakis (Citation2020) argue that increasing household size increases the probability of choosing to obtain credit from a semi-formal credit system.
The dependency ratio is positive and statistically significant for semi-formal microfinance systems. This result implies that given that the dependency ratio increases, households are more likely to choose a semi-formal microfinance system relative to indigenous loans. This finding contrasts with the work of Balogun and Yusuf (Citation2011), who found that households with a high dependency ratio have low demand for credit from NGOs and government agencies (predominantly semi-formal). However, our finding corroborates Mpuga (Citation2010). The author argued that households with a higher dependency ratio require more investment resources to keep the family going, compelling them to access loans from the NGOs and other semi-formal institutions. The marginal effect predicts that households are approximately 36 percentage points more likely to seek loans from semi-formal sources relative to an indigenous microfinance system if the dependency ratio increases by a unit. Also, the dependency ratio variable is negative and statistically significant at 1% for the probability of choosing a formal microfinance system relative to the base category. This finding implies that the likelihood of people sourcing loans from formal microfinance systems decreases as the dependency ratio increases. The marginal effect shows that the probability of households seeking loans from formal microfinance systems decreases by 31.1 percentage points for a unit increase in the dependency ratio relative to the indigenous system. A possible explanation for this finding is that households with a higher dependency ratio are more likely to be poor, hence unable to meet the collateral requirements for formal loans, forcing them to turn to the indigenous microfinance system.
Most people, especially in rural areas, form or join groups or associations to have a collective voice in their activities, including credit access. Group size (the number of people in the group) is significant at 1% level and negatively related to a household’s decision to access credit from the formal microfinance system relative to the indigenous microfinance system. This result implies that as the group size increases, a member is less likely to access loans from the formal microfinance system but more likely to access loans from the indigenous microfinance system. This outcome is understandable because, in rural areas, people depend on group solidarity in accessing credit. So as the group size increases, members are more likely to go in for loans from the indigenous microfinance system where there is group solidarity. Hence, group lending is a dominant criterion for credit disbursement. The probability that households will opt for loans from the formal microfinance system will decrease by 3.7 percentage points, given that the group size increases by a person. The factor is also positively related to the semi-formal microfinance system compared to the indigenous sources. Thus, as the group size expands, the probability of members accessing credit from the semi-formal microfinance system increases relative to the indigenous microfinance system. The marginal effect predicts that the probability of accessing credit from the semi-formal microfinance system increases by 2.7 percentage points relative to the indigenous system, given that the number of people in a group increases by a person.
The time it takes to repay the current loan is also a significant factor influencing households’ choice of a microfinance system. The repayment period is statistically significant at 1% for a household’s decision to choose both formal and semi-formal microfinance systems. It has a negative effect on households’ decision to opt for a formal microfinance system but a positive effect on their choice of a semi-formal microfinance system. The marginal effects show that the probability that households will choose loans from formal microfinance systems will decrease by 6.1 percentage points and increase by 4.1 percentage points in the semi-formal microfinance system relative to the indigenous microfinance system if the repayment period increases by a month. Comparatively, the indigenous microfinance system has a longer repayment time. Therefore, any additional increase in the repayment period may discourage beneficiaries from borrowing from this source since the commutative interest on the principal will be very high.
The interest rate is positive and statistically significant at 1% for the likelihood that households will choose a formal microfinance system relative to the base category. The marginal effect predicts that as the interest rate increases by a per cent, a household’s choice for formal microfinance system over the indigenous microfinance system is more likely to increase by 5.3 percentage points. This result is consistent with Owusu-Antwi and Antwi (Citation2010) and Balogun and Yusuf (Citation2011). These authors reported that as the interest rate increase, the demand for a loan from the indigenous sectors diminishes. This finding is understandable because the interest rate charged under the indigenous microfinance system is far higher than the rate charged by the formal credit lending institutions. This result is supported by our data, with the indigenous, semi-formal, and formal financial institutions charging an average interest rate of 107%, 38.3% and 34.67%, respectively. So, given that the interest rate increases, beneficiaries will therefore continue to favour the formal microfinance system since it has a substantially lower interest rate.
The information beneficiaries have on microfinance institutions and credit is a negative factor influencing households’ choice of a microfinance system. This result implies that, as beneficiaries acquire more information regarding the operations of microfinance systems, the less likely they will go in for semi-formal loans relative to the indigenous microfinance system. The marginal effect predicts that given that a household has information about a microfinance system, their likelihood of choosing a semi-formal relative to the indigenous microfinance system will decrease by 27.6 percentage points. Unless under targeted schemes, the loan market in developing economies tends to be biased towards formal workers, who have a constant source of income. This practice tends to be prevalent in semi-formal and formal credit financial institutions. This situation could explain why such requirements needed by the semi-formal institutions are made available to borrowers and more likely trigger self-exclusion from the semi-formal and formal credit sources. Borrowers will then tend to request credit from indigenous sources that they think might be easier for them to meet the requirements.
Borrowing experience has a significant positive effect on households’ probability of sourcing loans from formal microfinance systems. This finding implies that as beneficiaries gain more experience in borrowing, they are more likely to borrow from the formal microfinance system compared to the indigenous microfinance system. The marginal effect predicts that beneficiaries are 10.5 percentage points more likely to choose formal credit institutions, given that experience of borrowing increases by a year relative to the indigenous microfinance system.
The loan amount is a significant factor influencing the choice of a formal microfinance system. The coefficient is positive and statistically significant at a 1% level. The marginal effect predicts that if the current loan amount increases by a cedi, households are about 0.001 per cent more likely to access credit from the formal microfinance system than accessing it from the indigenous system.
Distance is a positive driver of beneficiaries’ choice of formal and semi-formal microfinance systems. These results imply that the faraway the credit institution is to the beneficiary, the more likely they will opt for formal and semi-formal microfinance systems relative to the indigenous microfinance system. The marginal effects predict the probability of choosing a formal and semi-formal microfinance system increases by 4.8 and 7.4 percentage points, respectively relative to the indigenous microfinance system. This finding contrasts the findings of Etonihu et al. (Citation2013), who observed a negative relationship between distance and the choice of credit outlet. The farther away the credit source is from the beneficiaries, the less likely they will access credit from that source. This discrepancy might be because, in the study area, the interest rate is relatively higher under the indigenous system. Hence, any beneficiary who has the means to travel to the district or regional capital where these formal and semi-formal credit institutions are located is more likely to choose formal and semi-formal credit sources which are predominantly located in these capitals and grant relatively larger loans at lower interest rates than their indigenous counterparts. Per the survey, the average annual interest on loans from the indigenous, semi-formal, and formal financial institutions are 107%, 38.3% and 34.67%, respectively. The disparities in the interest rate could explain why households will still opt for formal and semi-formal sources even as distance increases.
The effect of assets value on the probability of choosing a semi-formal microfinance system is negative and statistically significant at a 1% level. This result implies that the likelihood of households accessing credit from the semi-formal system reduces as asset value rises relative to the indigenous microfinance system. The result is consistent with the findings of Brewer et al. (Citation2019), who indicated that as the asset ratio of the farm increases, they are less likely to choose a semi-formal lender.
The number of household members employed also influences the household’s decision in accessing a loan from a microfinance system. The coefficient of the variable is negative and statistically significant at a 1% level for the probability that a person will seek a loan from a formal microfinance system but positive with the probability of accessing it from a semi-formal microfinance system relative to the indigenous microfinance system. These results imply that as the number of employed household members increases, they are less likely to source loans from the formal microfinance system, but more likely to source loans from the semi-formal system relative to the base category. The marginal effects predict that the probability of a household sourcing credit from the formal and semi-formal microfinance systems decreased and increased by 37.3 and 45.7% percentage points, respectively, relative to the indigenous system. The income of the primary beneficiary has a negative relationship with the likelihood of accessing credit from a formal microfinance system but is positively related to accessing it from a semi-formal microfinance system. These results are statistically significant at a 1% level in both cases. This result implies that as the primary beneficiary income increases, households are less likely to access credit from the formal microfinance system relative to the indigenous system. However, they are more likely to opt for a semi-formal microfinance system as their income increases relative to the indigenous system. Silong and Gadanakis (Citation2020) had similar findings for both formal and informal credit. However, the results of their study are not statistically significant.
5. Conclusion
The choice of credit source among beneficiaries of MFIs in the Upper West Region is influenced by gender, arable crop farming, household size, dependency ratio, group size, interest rate, distance, loan amount, borrowing experience, access to microfinance information, repayment period, household assets, and household members employed
When choosing a source of credit, a respondent’s access to financial information is a crucial determinant. Individuals should have financial extension services access to expand their understanding of credit sources and interest. Since the informal credit source setup in practice appears to be more suitable for rural clients, more information will enhance people’s awareness of it and increase access to credit. Regulating organisations for financial institutions should establish uniform repayment terms and practices for all sources of credit. A standardised setup will ensure that various credit sources compete with other competitive and efficient ways of sourcing for borrowers. As a result, both individuals and businesses will have easier access to finance.
The fact that men and women use different credit sources is a concern that calls for policy attention. It is vital to ensure effective policy and information communication to clients to ensure that there is no incidence or suggestion of discrimination between genders in granting loans across the various MFIs. The Ministry of Food and Agriculture should establish and strengthen policy initiatives to include arable crop farmers into formal credit schemes. Due to the risky nature of their farming, special programmes could be developed to encourage crop farmers to use formal credit sources. Also, government schemes which target farmers’ inclusion in the credit market should be concentrated in the indigenous financial institutions since they are the preferred credit sources for most arable farmers. Also, government efforts to achieve formal financial inclusion should entice arable crop farmers with appealing packages at formal institutions.
Like any other study, this study has some limitations. The study could not include financial literacy information in explaining the determinants of credit source choice, as suggested by some current literature. Also, interviews are vital tools for obtaining in-depth information from respondents. Therefore, it is recommended that future studies broaden the scope of their analysis to include qualitative data from respondents, such as the factors that influenced their choice of credit sources. Additional significant variables identified in current literature, such as financial literacy, could be included to broaden the range of the variables employed.
Correction
This article has been corrected with minor changes. These changes do not impact the academic content of the article.
Disclosure statement
No potential conflict of interest was reported by the author(s).
Additional information
Funding
Notes on contributors
Paul Bata Domanban
Paul Bata Domanban is a Senior Lecturer and the Dean of Students at the Simon Diedong Dombo University of Business and Integrated Development Studies (SDD-UBIDS), Ghana. He holds a PhD in Endogenous Development (University for Development Studies, Ghana). This current study ties in with his research interests in microfinance, poverty and development studies.
Fauster Agbenyo
Fauster Agbenyo is a Senior Lecturer and the Head of the Planning Department at the SDD-UBIDS, Ghana. He holds a PhD in Social Administration from University for Development Studies, Ghana. His research interests are theoretical, conceptual and empirical social and spatial development planning and management.
Samuel Sekyi
Samuel Sekyi is a Senior Lecturer and the Head of Department for the Department of Economics at the SDD-UBIDS, Ghana. He is a PhD (Economics) graduate from the University of South Africa. His main areas of expertise are Microeconometrics, health economics, development economics and agricultural economics.
References
- Abiola, B. (2011). Impact analysis of microfinance in Nigeria. International Journal of Economics and Finance, 3(4), 217–19. https://doi.org/10.5539/ijef.v3n4p217
- Adugna, T., & Heidhues, F. (2000). Determinants of farm households access to informal credit in Lume District, Central Ethiopia. African Review of Money Finance and Banking, 24(4), 27–46. https://www.jstor.org/stable/23026282
- Ahmad, S., Tayachi, T., Haq, S. G., Wang’ombe, W., & Ahmad, F. (2022). Entrepreneurial-specific characteristics and access to finance of SMEs in Khyber Pakhtunkhwa, Pakistan. Sustainability, 14(16), 10189. https://doi.org/10.3390/su141610189
- Alliance for Financial Inclusion. (2022). Policy catalogue: Women-led MSME access to financing. https://www.afi-global.org/wp-content/uploads/2022/08/Policy-Catalogue-Women-led-MSME-Access-to-Financing-24082022.pdf
- Anang, T. B., Sipiläinen, T., Bäckman, S., & Kola, J. (2015). Factors influencing smallholder farmers’ access to agricultural microcredit in Northern Ghana. African Journal of Agricultural Research, 10(24), 2460–2469. https://doi.org/10.5897/AJAR2015.9536
- Aryeetey, E. (2008). From informal finance to formal finance in Sub-Saharan Africa: Lessons from linkage efforts. Paper presented at the high level seminar on African finance for the 21st Century. IMF and Joint Africa Institute, Tunis, Tunisia, March 4–5. https://www.slideshare.net/DrLendySpires/from-informal-finance-to-formal-finance-in-sub-saharan-africa
- Ashraf, N., Karlan, D., & Yin, W. (2006). Tying Odysseus to the mast: Evidence from a commitment savings product in the Philippines. The Quarterly Journal of Economics, 121(2), 635–672. https://doi.org/10.1162/qjec.2006.121.2.635
- Asiamah, T. A., Steel, W. F., & Ackah, C. (2021). Determinants of credit demand and credit constraints among households in Ghana. Heliyon, 7(10), e08162. https://www.cell.com/heliyon/pdf/S2405-8440(21)02265-9.pdf
- Baffoe, G., & Matsuda, H. (2015). Understanding the determinants of rural credit accessibility: The case of Ehiaminchini, Fanteakwa district. Ghana. Journal of Sustainable Development, 8(6), 183–195. https://www.ccsenet.org/journal/index.php/jsd/article/view/47599
- Baidoo, S. T., Yusif, H., Ayesu, E. K., & Mensi, W. (2020). Improving loan repayment in Ghana: Does financial literacy matter? Cogent Economics & Finance, 8(1), 1787693. https://doi.org/10.1080/23322039.2020.1787693
- Balogun, O. L., & Yusuf, S. A. (2011). Determinants of demand for microcredit among the rural households in South-Western States, Nigeria. Journal of Agriculture & Social Sciences, 7(2), 41–48. http://www.fspublishers.org/published_papers/53847_.pdf
- Bank for International Settlements. (2010). Microfinance activities and the core principles for effective banking supervision. https://www.bis.org/publ/bcbs175.htm
- Bank of Ghana. (2007). A note on microfinance in Ghana. Working Paper No. WP/BOG-2007/01. Bank of Ghana Research Department, Accra, Ghana. https://www.findevgateway.org/sites/default/files/publications/files/mfg-en-paper-a-note-on-microfinance-in-ghana-2007.pdf
- Bank of Ghana. (2017). Credit referencing activity annual report.
- Barslund, M., & Tarp, F. (2008). Formal and informal rural credit in four provinces of Vietnam. Journal of Development Studies, 44(4), 485–503. https://doi.org/10.1080/00220380801980798
- Beck, T., Demirguc-Kunt, A., & Levine, R. (2005). SMEs, growth, and poverty: Cross-country evidence. Journal of Economic Growth, 10, 199–229.
- Biyase, M., & Fisher, B. (2017). Determinants of access to formal credit by the poor households. Studia Universitatis Babes-Bolyai. Economica, 62(1), 50–60. https://doi.org/10.1515/subboec-2017-0004
- Brewer, B. E., Bergtold, J. S., Featherstone, A. M., & Wilson, C. A. (2019). Farmers’ choice of credit among the farm credit system, commercial banks, and nontraditional lenders. Journal of Agricultural and Resource Economics, 44(2), 362–379. https://ageconsearch.umn.edu/record/287984
- Cameron, A. C., & Trivedi, P. K. (2005). Microeconometric methods and applications. Cambridge University Press.
- Chandio, A. A., Jiang, Y., Rehman, A., Twumasi, M. A., Pathan, A. G., & Mohsin, M. (2020). Determinants of demand for credit by small holder farmers’: A farm level analysis based on survey in Sindh, Pakistan. Journal of Asian Business and Economic Studies, 28(3), 225–240. https://ideas.repec.org/p/ags/aeas18/284776.html
- Christen, R. (1997). Banking services for the poor: Managing for financial success, an expanded and revised guidebook for microfinance institutions. Somerville.
- Dlamini, T., & Mohammed, M. (2018, September). Determinants of choice of credit sources by Eswatini SMEs: A focus on the Agriculture Sector. In 2018 Annual Conference, September 25-27, Cape Town, South Africa (No. 284776). Agricultural Economics Association of South Africa (AEASA).
- El-Habil, M. A. (2012). An application on multinomial logistic regression model. Pakistan Journal of Statistics and Operation Research, 8(2), 271–291. https://doi.org/10.18187/pjsor.v8i2.234
- Etonihu, K., Rahman, S. A., & Usman, S. (2013). Determinants of access to agricultural credit among crop farmers in a farming community of Nasarawa State, Nigeria. Journal of Development and Agricultural Economics, 5(5), 192–196. https://doi.org/10.5897/JDAE11.126
- Ferdous, J. (2021). Microfinance as a panacea for poverty reduction. In W. Leal Filho, A. M. Azul, L. Brandli, A. Lange Salvia, P. G. Özuyar, & T. Wall (Eds.), No poverty. Encyclopedia of the UN sustainable development goals (pp. 546–557). Springer, Cham. https://doi.org/10.1007/978-3-319-95714-2_125
- Fridell, M. (2007). Exploring the roles of informal, formal and semiformal microcredit in Jordan. [Unpublished dissertation], Lund University.
- Fufa, F. G. (2016). Determinants of access to credit and credit source choice by micro, small and medium enterprises in Nekemte, Ethiopia. International Journal of African and Asian Studies, 28, 11–27. https://core.ac.uk/download/pdf/234690228.pdf
- Gallardo, J. (2002). A framework for regulating microfinance institutions: The experience in Ghana and the Philippines. Research working paper series; No. WPS 2755. World Bank, Washington, DC. https://openknowledge.worldbank.org/handle/10986/15748
- Garson, D. (2009). Logistic Regression with SPSS. North Carolina State University, Public administration Program. https://www.dedoose.com/publications/logistic%20regression.pdf
- Greene, W. H. (2003). Econometric analysis (4th) ed.). Pearson Education Inc.
- Hananu, B., Abdul-Hanan, A., & Zakaria, H. (2015). Factors influencing agricultural credit demand in Northern Ghana. African Journal of Agricultural Research, 10(7), 645–652. https://doi.org/10.5897/AJAR2014.9330
- Harper, M. (1998). Profit for the poor, cases in microfinance. Intermediate Technology Publications.
- Iddrisu, A. M., & Danquah, M. (2021). The welfare effects of financial inclusion in Ghana: An exploration based on a multidimensional measure of financial inclusion (No. 2021/146). WIDER Working Paper.
- Khawari, A. (2004). Microfinance: Does it hold its promises? A survey of recent literature. HWWA discussion paper no. 276. Homburg Institute of International Economics, Homberg, Germany. https://papers.ssrn.com/sol3/Delivery.cfm/SSRN_ID556213_code357559.pdf?Abstractid=556213&type=2
- Kilic, T., Serajuddin, U., Uematsu, H., & Yoshida, N. (2017). Costing household surveys for monitoring progress toward ending extreme poverty and boosting shared prosperity. World bank policy research paper no. 7951, World Bank, Washington, DC, USA. https://documents.worldbank.org/en/publication/documents-reports/documentdetail/260501485264312208/costing-household-surveys-for-monitoring-progress-toward-ending-extreme-poverty-and-boosting-shared-prosperity
- Kiros, S., & Meshesha, G. B. (2022). Factors affecting farmers’ access to formal financial credit in Basona Worana district, north showa zone, Amhara regional state, Ethiopia. Cogent Economics & Finance, 10(1), 2035043. https://doi.org/10.1080/23322039.2022.2035043
- Krejcie, R. V., & Morgan, D. W. (1970). Determining sample size for research activities. Educational and Psychological Measurement, 30(3), 607–610. https://doi.org/10.1177/001316447003000308
- Kumar, A., Singh, D. K., & Kumar, P. (2007). Performance of rural credit and factors affecting the choice of credit sources. Indian Journal of Agricultural Economics, 62(3), 297–313. https://cgspace.cgiar.org/handle/10568/821
- Littlefield, E., & Rosenberg, R. (2004). Microfinance and the poor (Breaking down walls between microfinance and formal finance). Finance and Development. https://www.imf.org/external/pubs/ft/fandd/2004/06/pdf/littlefi.pdf
- Machili, T. (2021). “Financial inclusion and food security nexus: Evidence from the Southern African development community”. SA-TIED Working Paper No. 198. Southern Africa – Towards Inclusive Economic Development, September.
- Mamuye, W. (2021). Determinants of smallholder farmers participation in formal credit and challenges faced by institutions: The case of Mojana Wodera District, Amhara Region, Ethiopia. International Journal of Finance and Banking Research, 7(1), 9–20. https://doi.org/10.11648/j.ijfbr.20210701.12
- Mpuga, P. (2010). Constraints in access to and demand for rural credit: Evidence from Uganda. African Development Review, 22(1), 115–148. https://doi.org/10.1111/j.1467-8268.2009.00230.x
- Mukhwami, J. T., Gathungu, E. W., & Kalio, A. M. (2022). Determinants of semi-formal credit participation among rural farm households in Kakamega County, Kenya. Journal of Agribusiness and Rural Development, 63(1), 71–79. http://dx.doi.org/10.17306/J.JARD.2022.01510
- Mwonge, L. A., & Naho, A. (2021). Determinants of credit demand by smallholder farmers in Morogoro, Tanzania. African Journal of Agricultural Research, 17(8), 1068–1080. https://doi.org/10.5897/AJAR2020.15382
- Odu, O. O., Okoruwa, V. O., Adenegan, K. O., & Olajide, A. O. (2011). Determinants of rice farmer’s access to credit in Niger State, Nigeria. Journal of Rural Economics and Development, 20(1), 8–20. https://ageconsearch.umn.edu/record/206865/?ln=en
- Omar, M. A., & Inaba, K. (2020). Does financial inclusion reduce poverty and income inequality in developing countries? A panel data analysis. Journal of Economic Structures, 9(1), 1–25. https://doi.org/10.1186/s40008-020-00214-4
- Osei-Assibey, E., Bokpin, G. A., & Twerefou, D. K. (2012). Microenterprise financing preference: Testing POH within the context of Ghana’s rural financial market. Journal of Economic Studies, 39(1), 84–105. https://www.emerald.com/insight/content/doi/10.1108/01443581211192125/full/html
- Otero, M. (1999). Bringing developments back, into microfinance. Journal of Microfinance, 1(1), 8–19. https://scholarsarchive.byu.edu/cgi/viewcontent.cgi?article=1115&context=esr
- Owusu-Antwi, G., & Antwi, J. (2010). The analysis of the rural credit market in Ghana. International Business and Economics Research Journal, 9(8), 45–56. https://clutejournals.com/index.php/IBER/article/view/611
- Pande, R., Cole, S., Sivasankaran, A., Bastian, G., & Durlacher, K. (2012). Does poor people’s access to formal banking services raise their incomes?–A systematic review. In DFID Systematic Review EPPI-Centre. London: Social Science Research Unit, Institute of Education, University of London.
- Ramkumar, R., Supriyo, R., & Kaushik, K. (2015). Role of microfinance for improving quality of life by providing financial services to Low-income group: A critical review. International Journal of Applied Engineering Research, 10(55), 4136–4142. https://www.ripublication.com/Volume/ijaerv10n55spl.htm
- Robinson, M. (2001). The microfinance revolution: Sustainable finance for the poor. World Bank, Washington DC, USA. https://openknowledge.worldbank.org/handle/10986/28956
- Sakyi‐Nyarko, C., Ahmad, A. H., & Green, C. J. (2022). Investigating the well‐being implications of mobile money access and usage from a multidimensional perspective. Review of Development Economics, 26(2), 985–1009. https://onlinelibrary.wiley.com/doi/abs/10.1111/rode.12848
- Sekabira, H., & Qaim, M. (2017). Can mobile phones improve gender equality and nutrition? Panel data evidence from farm households in Uganda. Food Policy, 73, 95–103. https://doi.org/10.1016/j.foodpol.2017.10.004
- Sekyi, S. (2017). Rural households’ credit access and loan amount in Ghana: Empirical evidence from Wa municipality. International Journal of Economics and Financial Issues, 7(1), 506–514. https://www.econjournals.com/index.php/ijefi/article/view/2858
- Sekyi, S., Nkegbe, P. K., & Kuunibe, N. (2014). Participation in the credit market by small-scale enterprises in Ghana: Evidence from Wa municipality. African Journal of Business Management, 8(9), 292–299. https://doi.org/10.5897/AJBM2013.7313
- Silong, A. K. F., & Gadanakis, Y. (2020). Credit sources, access and factors influencing credit demand among rural livestock 10 farmers in Nigeria. Agricultural Finance Review, 80(1), 68–90. https://www.emerald.com/insight/content/doi/10.1108/AFR-10-2018-0090/full/html
- Swamy, V. (2014). Financial inclusion, gender dimension, and economic impact on poor households. World Development, 56, 1–15. https://doi.org/10.1016/j.worlddev.2013.10.019
- Tuffour, J. A. (2002). Forging linkages between formal and informal financial sectors: Emerging practices in Ghana. African Finance Journal, 4(1), 1–31. https://journals.co.za/doi/pdf/10.10520/EJC33795
- Wieser, C., Bruhn, M., Kinzinger, J. P., Ruckteschler, C. S., & Heitmann, S. (2019). The impact of mobile money on poor rural households: Experimental evidence from Uganda. World Bank Policy Research Working Paper, (8913). https://doi.org/10.1596/1813-9450-8913