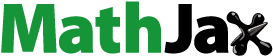
Abstract
Significant changes have been brought about in consumer behaviour as a result of the COVID-19 pandemic. Digital consumption has attracted a large number of new consumers during the pandemic. However, there are few academic studies on the determinants of these crucial changes in consumer behaviour. Addressing this gap, this study investigates consumers’ channel-switching behaviour during the COVID-19 pandemic. Using a sample of about 2,640 respondents collected after the outbreak, this study aims to define the key drivers of the changes in consumers’ shopping channel decisions. The study results show that several factors significantly affected consumers’ decisions to change their shopping habits after the pandemic broke out, including marital status, price, quality, convenience, and overall satisfaction with current and new shopping channels. More importantly, this study is one of the few to investigate the differences in determining factors regarding consumers’ choices of online and traditional channels in the post—COVID-19 pandemic era. The level of convenience, the time spent making purchases, technology competency, the abundance of product information, the ability to check product quality, and income significantly impact purchasing channel decisions between online and traditional channels.
1. Introduction
In December 2019, COVID-19 was reported by officials in Wuhan City, China. Subsequently, it has been spreading continuously on a global scale. Because of the rapid spread of the virus and the absence of an effective and safe treatment or vaccine, non-pharmaceutical interventions (NPIs) are the most important recommendations for preventing and controlling the transmission and spread of COVID-19 adopted by many governments (Iezadi et al., Citation2020). As a result, consumers have been spending more time online because of the lockdowns. According to the e-Conomy SEA 2020 report, the COVID-19 pandemic has led to significant shifts across Southeast Asia (SEA). This pandemic has been claimed to change the behaviour of some nature of consumers in this region. Approximately 40 million new users utilized digital consumption for the first time in 2020 (Iezadi et al., Citation2020). Vietnam is considered to be one of the fastest-growing Internet economies in SEA, with a growth rate of 36% in 2020. At that time, electric commerce (e-commerce) revenue amounted to approximately US$11.8 billion, accounting for approximately 5.5% of the total retail sales of goods and services in Vietnam.
Online shopping is a form of e-commerce in which customers can reach products or services and sellers can reach customers via the Internet through online platforms, which means that people can make sales and purchases at any time from any place. Apart from buying and selling products and services, e-commerce provides other services, such as transferring funds, money, data, and information related to commercial transactions through the Internet using information and communication technology (ICT). Improvements in technology in this era and the coming of the COVID-19 outbreak have contributed to changes in online shopping behaviour to some extent.
Although several studies have been made in the context of the COVID-19 pandemic, few studies have examined consumers’ channel-switching behaviour in the post—COVID-19 pandemic period (Ngoh & Groening, Citation2022; Youn et al., Citation2021). This study explores the channel-switching behaviour of consumers as a result of the COVID-19 outbreak. Using a sample of over 2,640 respondents, this study attempts to identify the key determinants of customers’ changing shopping channel choices. Several criteria—including marital status, price, quality, convenience, and overall happiness with existing and new shopping channels—strongly influence customers’ choices to modify their shopping habits following the pandemic outbreak. Service quality appears to significantly influence customers’ channel-switching decisions, followed by price and convenience. In addition, satisfaction with the existing channel influences whether customers transfer channels. In the post—COVID-19 pandemic age, this research also illustrates the contrasts between the variables that affect customers’ selection of the Internet and conventional channels. In general, this study attempts to address issues concerning (i) defining factors that significantly affect consumers’ channel-switching behaviour after the outbreak of the COVID-19 pandemic and (ii) defining factors that significantly affect consumers’ decision to prioritize online purchasing and conventional (e.g. traditional markets and supermarkets) channels during the COVID-19 pandemic.
This study has five sections. Following the introduction, the remainder of this research is divided into the following four sections. The second section provides a synthesis of relevant literature. In the third section, both the methodology and the data are broken down. In the fourth section, the study goes through the findings in detail. Finally, the conclusions are presented in the fifth section.
2. Literature review
2.1. Theoretical framework
In the literature, numerous theories have been applied to explain and predict multidimensional individual behaviour, which could lay the foundation for behavioural change. The most widely accepted theories can be divided into the following categories: (1) belief—attitude theories (i.e. protection motivation theory, the health belief model, the theory of reasoned action (TRA), the theory of planned behaviour (TPB)); (2) control-based theories (i.e. self-determination theory); (3) decision-making theories (Prochaska & DiClemente, Citation1983); and (4) competence-based theories (i.e. social cognitive theory (SCT), originated by Bandura and Walters (Citation1977)). In this study, to explain consumers’ channel-switching behaviour, we mainly used belief—attitude theories given that customers usually formulate their behaviours in daily purchasing using available beliefs and concurrent stimuli from the environment (Han & Ryu, Citation2012; Jung et al., Citation2016).
2.1.1. Theory of planned behaviour
The TPB is the extension of the self-efficacy theory (SET) and the TRA. Ajzen introduced the TPB by adding the component “perceived behavioural control”. In this way, he extended the TRA to better predict actual behaviour.
Perceived behavioural control refers to the degree to which a person believes that he or she can perform a given behaviour. It is concerned with an individual’s impression of his or her own ability to accomplish the behaviour. In other words, perceived behavioural control is tied to a certain behaviour or goal. This perception varies according to environmental circumstances and the behaviour involved. The TPB suggests that people are much more likely to carry out certain behaviours when they believe that they can do them successfully.
Compared to the TRA and TAM, the TPB is considered one of the most comprehensive and validated theories in the exercise domain for understanding and predicting exercise behaviour.
2.1.2. Expectation confirmation theory
Expectation confirmation theory (ECT) (Figure ) is a cognitive theory developed by Oliver (Citation1977) and applied to study consumer satisfaction and post-purchase behaviour. ECT has been widely applied to examine both pre-behaviour (expectation) and post-behaviour (perceived performance) variables rather than only pre-behaviour variables. Figure 4 illustrates the key factors and relationships in ECT.
Figure 1. Expectation confirmation theory.
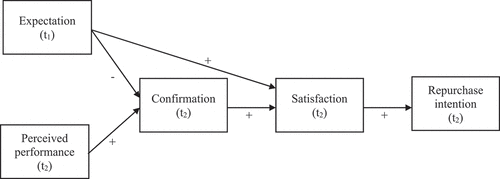
According to ECT, consumers form their initial expectations about a specific product or service based on their existing knowledge (Zeithaml & Berry, Citation1990) or interactions with different members of the communication channels (Rogers, Citation1995) before a purchase decision is made. After consuming that product or service, consumers establish perceptions about the performance of the product or service based on their consumption experiences (called perceived performance). Then a comparison is made between the perceived performance and the initial expectation to determine the expectation (confirmation). Pre-purchase expectations may be confirmed or contradicted by apparent performance. Positive confirmation is expected to occur if the product performs better than expected (i.e. perceived performance exceeds expectation). Alternatively, negative confirmation occurs if the consumers’ appraisal of the product or service is worse than expected (i.e. perceived performance is lower than expected). Finally, there is a positive link between expectation and satisfaction. At the same time, the confirmation of expectations leads to consumer satisfaction with the product or service. When expectations were either high or confirmed, there was a higher level of satisfaction. Consequently, satisfied customers are more likely to purchase the product or service again than those who are unsatisfied.
2.1.3. The unified framework of the planned and confirmation theory
This study proposes a unified framework of the planned and confirmation theory to explain channel-switching behaviour in two stages (Figure ). In the first stage, an individual’s intention to purchase products via a channel is determined by their perceived behavioural control regarding the channel (e.g. convenience, time spent, price, product quality) and their subjective norms regarding the purchase and channel (e.g. abundance of information and safety). The expectation components from the ECT can be used to explain how an individual’s expectations influence their attitude towards it (e.g. trust). If an individual has positive expectations, they will be more likely to have a positive attitude towards the channel and be more likely to engage with the channel. In the second stage, an individual’s decision to reuse the channel is determined by their satisfaction with the channel. The confirmation component of the ECT can be used to explain how an individual’s experiences with the channel influence their satisfaction with it. If an individual’s expectations of the channel are met or exceeded, they will be more likely to be satisfied with the channel and be more likely to reuse it.
2.2. Research questions
Davis (Citation1993) suggested that consumers’ attitude towards Internet shopping first depends on the direct effects of relevant online shopping features, which can be either the consumers’ perceptions of functional and utilitarian dimensions, such as “ease of use” and “usefulness”, or their perceptions of emotional and hedonic dimensions such as “enjoyment” (Menon & Kahn, Citation2002; Childers et al., Citation2001; Mathwick et al., Citation2001). Moreover, the literature shows that attitudes towards online shopping and the intention to shop online are not only affected by ease of use, usefulness, and enjoyment but also by exogenous factors such as consumer traits, situational factors, product characteristics, previous online shopping experiences, and trust in online shopping. Relevant exogenous factors in this context are “consumer traits” (Burke, Citation2002; Dabholkar & Bagozzi, Citation2002; Brown et al., Citation2001; Eastin & LaRose, Citation2000), “situational factors” (Wolfinbarger & Gilly, Citation2001; Avery, Citation1996), “product characteristics” (Grewal et al., Citation2002; Elliot & Fowell, Citation2000), “previous online shopping experiences” (Shim et al., Citation2001; Eastlick & Lotz, Citation1999), and “trust in online shopping” (Yoon, Citation2002; Lee & Turban, Citation2001). Furthermore, Rogers, Citation1995) asserted that perceived innovation qualities—such as compatibility, relative advantage, and complexity—may be predictors of online consumer adoption.
According to the literature, various factors constrain consumers’ adoption of new purchasing channels, including transactional barriers such as slow load times, the inability to discover items, inadequate information, the absence of human interaction, missed or late deliveries (Kaufman-Scarborugh & Lindquist, Citation2002), ease of use (difficulties with site navigation and complex procedures), and security (Elliot & Fowell, Citation2000). This study expands this body of literature by investigating the factors that determine consumers’ channel-switching behaviour in the post—COVID-19 pandemic era. The following three research questions are proposed in this study:
After the outbreak of the COVID-19 pandemic, which factors significantly affected consumers’ channel-switching behaviour?
During the COVID-19 pandemic, which factors have significantly affected consumers’ decisions to prioritize online purchasing channels?
In the COVID-19 pandemic era, which factors significantly affect consumers’ decisions to prioritize traditional purchasing channels (market or supermarket)?
3. Data and methodology
3.1. Data
This study employs a survey technique with a structured questionnaire as the data collection method to answer the three research questions. The questionnaire comprised a number of questions (Table ) developed following the theoretical background of the planned and confirmation theory framework to survey the variables used for data analysis. The five-point Likert measurement scale was used to structure the questions and collect the respondents’ perceived opinions. From December 2021 to March 2022, more than 5,000 individuals in Vietnam were contacted and received a structured questionnaire via email and follow-up phone calls if necessary. The sample was selected using stratified random selection to ensure sufficient data for each purchasing channel. This approach provides a highly representative level of the sample regarding purchasing channel choices in Vietnam. The inputs of irrelevant and inconsistent replies are removed, edited, rectified, rephrased, and refined, among other qualifying and refining procedures. These approaches lowered the number of qualified questionnaires needed for further data analysis to 2,647 valid answers, resulting in a response rate of approximately 56%. According to the descriptive statistics of the demographic variables in Table , this study’s sample largely reflects the main characteristics of the Vietnamese population. For instance, most of the respondents in the sample range from 22 to 50 years of age, which is consistent with the fact that Vietnam is a young country, with most of the population ranging from 25 to 54 years of age. The same conclusions can be drawn from income and education statistics. Vietnam is still a developing country with a GDP per capita of marginally over US$4,000 per annum, which is consistent with the major proportion of respondents’ income in the sample, ranging from 10 to 25 million VND.
Table 1. Factors and measurement items
Table 2. Descriptive analysis
3.2. Methodology
First, given the exploratory nature of this study, descriptive statistics were used to explore the impact of the COVID-19 outbreak on consumers’ channel-switching behaviour in the post—COVID-19 pandemic era.
Then a logistic regression model was employed to answer the research questions as the channel-switching behaviours and channel choices after the pandemic outbreak were constructed as binary variables. In this case, the switching channel behaviours and online and traditional channel selections are the dependent variables. The explanatory variables can be separated into different main groups: (1) respondents’ profile (education, age, income, gender, marital status, and technological competence), (2) channel benefits and characteristics’ perceptions (convenience, price, service quality, and general satisfaction), and (3) channel selection barriers (time, trust, safety, range of selection, information, technology, and freshness of goods). We also follow the usual practices to treat our ordinal independent variables as equally spaced continuous variables in running logistics models (Lenth & Dobler, Citation2005). The conceptual logistics models can be written as follows:Let
4. Analysis and results
4.1. Descriptive analysis
Figure depicts the proportion of respondents’ choice of purchasing channels before and after the COVID-19 pandemic broke out. Before the pandemic, traditional and small markets dominated consumers’ choice of purchasing channels (1,296 respondents, 49%). Supermarkets were the second most popular choice (637 respondents, 24%). Convenience stores (370, 14%) and online (344, 13%) channels were chosen the least frequently. However, after the COVID-19 pandemic broke out, online channels became the most popular choice (827, 31%). The traditional market (703, 27%) and supermarket (430, 16%) channels are still popular, even in the post-pandemic era. Convenience and grocery stores (294, 11%) became the least popular choice after the pandemic.
Regarding specific switching channel behaviours, Table presents the consumers’ changes from the pre-COVID-19 purchasing channels to the alternative channels after the pandemic broke out. Notably, consumers who used the market and supermarket channels before the pandemic tended to switch dramatically to online channels after the outbreak. Even though the pandemic has been appropriately controlled in Vietnam since the end of 2021, consumers have been switching to online channels, indicating the significant impact of the COVID-19 pandemic on consumers’ purchasing behaviours.
Table 3. Consumers’ channel-switching behavior after the COVID-19 pandemic broke out
4.2. Analysis and discussion
4.2.1. Drivers of the consumers’ channel-switching behaviour in the post—COVID-19 pandemic era
To determine which factors significantly affect consumers’ channel-switching behaviour after the COVID-19 pandemic outbreak, the consumers were asked to compare the old and new channels of purchasing based on multiple criteria. Based on the results in Table , different key factors affecting consumers’ switching behaviours regarding purchasing channels were identified, including marital status, price, service quality, convenience, and overall satisfaction.
Table 4. Factors driving the consumers’ channel-switching behavior in the post-COVID-19 pandemic era
4.2.1.1. Service quality
The results show a positive correlation between product or service quality and shopping channel shifts. More clearly, a strong shift occurs when perceived product quality and service quality are higher in the new channel than in the old one. Andreti et al. (Citation2013), when determining the reason for the constant increase in the number of convenience stores, proved that price, the form of promotion, and quality of service are factors that attract customers to the convenience store. Satit et al. (Citation2012) also provided similar results when proving that product quality and price strongly impact consumers’ final decisions.
4.2.1.2. Convenience
At the 1% significance level, convenience is positively correlated with the respondents’ decisions to change shopping channels. This result is similar to those of Irawan (Citation2018), who examined factors affecting online purchasing decisions in the Tangerang Selatan area, according to which trust, convenience, and quality service quality have a positive and significant impact on consumers’ online purchasing decisions. Moeller et al. (Citation2009) found clues about the significant impact of decision convenience and convenient access on purchase behaviour by looking at convenience across different dimensions (decision, access, search, transaction, and after-sales convenience). Khalifa and Liu (Citation2007) also showed that the driving forces of online shopping behaviour are transaction efficiency, security, convenience, and cost savings.
4.2.1.3. Price levels
Satit et al. (Citation2012), when researching marketing strategy and customer decision making in the travel industry, showed that price and product quality have a strong impact on attracting customers. Customers tend to compare prices and product quality during product selection as well as shopping channel selection. To examine the emotional processes of changing brand prices, Somervuori and Ravaja (Citation2013) found that lower prices and national brands generate higher positive emotions than products with low prices; thus, positive emotions are associated with greater purchase intention. This study shows the importance of studying emotions when examining prices and consumer buying behaviour.
4.2.1.4. Overall satisfaction with shopping channels
The results showed that the overall level of satisfaction had a positive impact on the change in shopping channels. Accordingly, when the respondents have a high level of satisfaction with the new purchase channel, they tend to change their purchase channel choice. Mokhtarian and Tang (Citation2013), when researching clothing shopping behaviour on two channels (stores and the Internet) of people in northern California, showed that the overall level of satisfaction after purchasing is a factor that positively affects purchase channel choice at the 5% significance level.
4.2.1.5. Tech competency
Technology knowledge can be seen as the foundation of online shopping. Consumers with strong online capabilities are known as competent customers and are sophisticated in distinguishing websites that are safe and reliable to manipulate. Moreover, with their information search skills, they become smart shoppers in evaluating the quality of products and services. Raman and Pashupati (Citation2005), when distinguishing the level of self-perceived technological competence (SPTC) that influences people’s online behaviour through concern for customer privacy, revealed that people with low tech capabilities are more concerned with the risk to their privacy that the Internet brings to them and more likely to follow offline interaction, while those with high SPTC, who also know about and use the security features of online systems (such as security locks), have a higher level of risk acceptance when interacting online and feel more comfortable with the online transaction. In addition, online purchasing expenditure is more affected by customers’ technological competence than trust and privacy issues (Y. M. Kim, Citation2011). Similarly, a study by Mummalaneni et al. (Citation2016) in China revealed a positive relationship between customers’ technology readiness and purchase intention in both direct and indirect dimensions through perceived e-service quality. In Malaysia, Ling et al. (Citation2011) supported this view when they concluded that perceived technology is a focal element affecting online purchase intention, mediated by online trust.
4.2.1.6. Marital status
The results also reveal the significant impact of a demographic variable (marital status) that affects the decision to change shopping channels after the pandemic. Specifically, married people tend to change shopping channels more than unmarried individuals. In other words, single people tend to adhere to their current shopping habits. Deepak and Velaudham (Citation2019) examined the relationship between marital status and buying behaviour and found that the shopping decisions of married people are significantly different from those of single people. The results of this study show that the shopping decision making of married people is more influenced by factors such as consumer demand, product awareness, search information, and the evaluation of substitute products. In addition, price has an inverse effect on the change from the old channel to the new channel, with 95% confidence—that is, the lower the price of goods when buying in the new channel, the more the respondents choose the new channel to replace the current shopping channel.
4.2.2. Drivers of the consumers’ decisions on purchasing channels in the post—COVID-19 pandemic era
Table reveals a number of different elements driving online channel and traditional channel (market and supermarket) purchasing behaviour (Models (1) and (2)) in the post—COVID-19 pandemic period, including online channel characteristics (convenience, price level, price compared to other channels, product quality, service quality, time, information for selections, freshness of goods or products) and the respondents’ profiles (income, technology competence).
Table 5. Drivers of the consumers’ decisions on purchasing channels in the post-COVID-19 pandemic era
The results suggest that the respondents who are more advanced in their income (i.e. full-time vs. part-time jobs) tend to make more purchases on virtual platforms. This finding is consistent with those of Jusoh and Ling (Citation2012). However, this effect is not valid for the decision to choose the market or supermarket channels for purchasing. This could be because traditional purchasing channels are not very sensitive to income levels (Suwanvijit & Promsa-Ad, Citation2009; Tian et al., Citation2021).
In terms of the price level, we employ two variables: the perceived price when consumers experience online purchasing and the price levels of the channels compared to those of the other channels. The results reveal that the more reasonable the perceived price of goods when buying online and through traditional channels, the higher the potential for purchasing behaviour to be performed. Additionally, the competitive price levels of a channel compared with those of other purchasing channels encourage consumers to choose the current channel (Wolk & Ebling, Citation2010). For example, even though the prices of products are very similar across channels, online purchasing could result in higher total costs because of the high shipping cost, and the choice to buy online is much less attractive to consumers.
The results indicate that higher perceived convenience drives a higher probability of online shopping behaviour. Convenience is also a key driver of customer satisfaction in online shopping (Duarte et al., Citation2018). In contrast, if consumers pay more attention to the convenience of purchasing channels, traditional channels are not likely to be chosen. In addition, as one of the most important resources, the time spent on different channels for purchasing is important to consider. The results show that the more time is needed to make a purchase on a channel, the less attractive it is. This effect is not significant in online channels because of their convenience. However, the time spent negatively and significantly affects the choice of traditional purchasing channels.
Regarding consumers’ technological competence, the results show that once customers do not struggle with a concurrent technological problem and feel easy and comfortable manipulating technology to buy an item online, they prefer to purchase more using virtual platforms. Consumers with limited knowledge of technology and less understanding of how it works as well as the nature and benefits of online shopping are less likely to shop online (Cheung and Liao, Citation2003). Shopping experience is an important factor when studying consumers’ online shopping behaviour. Unlike traditional shopping, online shopping lacks social interaction. This makes many people with little online shopping experience hesitant to buy on an Internet platform. This was discovered by Khalifa and Liu (Citation2007), H. B. Kim et al. (Citation2009), and Dai et al. (Citation2014).
Product quality assessment is another important aspect to consider when deciding on a purchasing channel. The results indicate that consumers tend not to use online channels when they cannot touch the product and assess its quality. Consumers expect to see, touch, and inspect goods before purchasing them. This issue has been extensively discussed in the literature, such as in the studies by Kiran et al. (Citation2008), Goldsmith and Goldsmith (Citation2002), and M. J. Kim et al. (Citation2011). Moreover, in an empirical survey on consumer buying behaviour when shopping online, Ahuja et al. (Citation2003) showed that 4% of the research sample agreed that one of the reasons buyers do not choose online channels is that they lack inclination towards the product.
In addition to product quality, service quality plays a critical role in deciding which channels to use to purchase goods and products. According to the findings of this study, there is a positive relationship between service quality and both the choice of online channels and traditional channels. Interestingly, trust is not a significant factor affecting consumers’ choices of either online or traditional channels. This finding contrasts with those of Chung et al. (Citation2012), who investigated consumers’ attitudes and behaviours towards agricultural products and found that trust is one of the biggest factors in purchasing channel decisions.
Table reveals that these respondents value the freshness of the goods, one of the drivers for choosing traditional channels over online platforms for purchasing food or agricultural products. Moreover, abundant information for selection is an advantage of online shopping and significantly improves the chance of selecting this channel. These findings support those of Li and Houston (Citation2001), who studied the factors affecting consumers’ preferences of shopping channels in buying food in Taiwan with the six shopping channels mentioned. The authors showed that in most shopping channels, age, education level, and abundance of information for goods or product selection influence the frequency of shopping in the channels.
5. Discussion
First, the study contributes to the existing literature on consumer behaviour by proposing a new framework to explain channel-switching behaviours. The TPB and ECT can be merged to create a comprehensive framework of behaviour, called the planned and confirmation theory framework. In the first stage, this framework considers the individual’s intentions and expectations as well as their beliefs about certain behaviours. In the second stage, it considers the individual’s past experiences, which shape their decision to avoid or repeat the behaviour. This model could be used to better understand which factors influence and predict how individuals are likely to behave in the first place and then continue their actions in the future. For example, this model can be used to identify the factors that influence an individual’s decision to engage in a certain behaviour, such as purchasing, smoking, or drinking. The model could also be used to understand how an individual’s expectations and performance in a situation can shape their tendency to repeat the same behaviours or change them. For instance, if an individual expects to be judged negatively for a certain behaviour, they may be more likely to avoid it than repeat it.
Second, this study contributes to the existing literature by defining the key determinants leading to channel-switching behaviours after the outbreak of the COVID-19 pandemic, which have been ignored by scholars in the consumer behaviour field (Youn et al., Citation2021). Given that the COVID-19 pandemic has dramatically changed many aspects of life (Lin et al., Citation2021), people’s shopping methods have changed fundamentally. This study found multiple factors related to purchasing channels that affect people’s decisions to use online channels or conventional channels (traditional market or supermarket). Specifically, online channel characteristics (convenience, price level, price compared to other channels, product quality, service quality, time, information for selections, and freshness of goods or products) and respondents’ profiles drive online channel and traditional channel purchasing behaviour in the post—COVID-19 pandemic period (income, technology competence). These findings confirm the results of existing studies on the decision to choose online or traditional channel purchasing before the COVID-19 pandemic (Jusoh & Ling, Citation2012; Suwanvijit & Promsa-Ad, Citation2009). This suggests that these are fundamental factors for switching channel decisions and remain in effect regardless of the change in the external environment.
Third, another fundamental factor that managers in online businesses should pay attention to is people’s technological competency. The results of this study confirm previous findings on this matter (Dai et al., Citation2014; H. B. Kim et al., Citation2009), demonstrating that consumers buy more products or services online when they do not have technology issues and are comfortable using technology. Tech-illiterate shoppers are less likely to shop online (Cheung & Liao, Citation2003). Moreover, because online purchasing lacks socialization, consumer Internet purchase behaviour depends on the individual’s shopping experience, which makes technological literacy essential. Managers running online businesses should pay attention to this issue by making their online platforms much more user-friendly or have the initiative to educate consumers on technological literacy.
Finally, this study provides insights into how e-commerce and traditional operators can use different management methods to boost shoppers’ purchasing intentions within the framework of a pandemic. Specifically, this research suggests that operators should focus on the factors service quality, convenience, price range, overall satisfaction, tech proficiency, and marital status to increase their sales. Additionally, the existing channel’s customer satisfaction factor is a critical determinant influencing shopping channel conversion behaviour. Other factors—such as convenience, time spent, product quality assessment, price level, abundance of information, freshness of goods or products, and education level—were found to influence the choice of a particular purchasing channel.
6. Conclusion
This study found a significant increase in the number of consumers purchasing on virtual platforms after the outbreak of the COVID-19 pandemic. In addition, this research indicates and measures the impact of factors affecting consumer behaviour through two aspects: switching behaviour and consumption channel selection behaviour. Furthermore, the study shows that service quality, convenience, price range, overall satisfaction, tech proficiency, and marital status influence shopping channel conversion behaviour after the COVID-19 pandemic. Among these, the existing channel’s customer satisfaction factor is a critical determinant. The results also indicate that a number of factors—including convenience, service quality, good quality, time spent, product quality assessment, price level, price level compared to other channels, abundance of information, freshness of goods or products, tech competency, and education level—influence the choice of a particular purchasing channel after the pandemic. Interestingly, there was no significance in terms of trust factors in deciding on purchasing channels. The findings offer insights and feedback for e-commerce and traditional operators in developing different management methods to boost shoppers’ purchasing intentions within the framework of a pandemic.2003
Acknowledgments
This research is funded by University of Economics Ho Chi Minh City, Vietnam (UEH).
Disclosure statement
No potential conflict of interest was reported by the author(s).
Additional information
Funding
References
- Ahuja, M., Gupta, B., & Raman, P. (2003). An empirical investigation of online consumer purchasing behavior. Communications of the ACM, 46(12), 145–16. https://doi.org/10.1145/953460.953494
- Andreti, J., Zhafira, N. H., Akmal, S. S., & Kumar, S. (2013). The analysis of the product, price, place, promotion and service quality on customers’ buying decision of convenience store: A survey of a young adult in Bekasi, West Java, Indonesia. International Journal of Advances in Management and Econ, 2(6), 72–78.
- Avery, S. (1996). The interactive marketplace: Business-to-business strategies for delivering just-in-time, mass-customized products. Harvard Business Press.
- Bandura, A., & Walters, R. H. (1977). Social learning theory (Vol. 1). Englewood cliffs.
- Brown, J. J., Reingen, P. H., & Ganesan, S. (2001). Does type of product involvement matter? The role of consumer-level variables in predicting responses to brand extensions. Journal of the Academy of Marketing Science, 29(2), 149–164.
- Burke, R. R. (2002). Technology and the customer interface: What consumers want in the physical and virtual store. Journal of the Academy of Marketing Science, 30(4), 411–432.
- Cheung, M. & Liao, Z. (2003). Supply-side hurdles in internet B2C e-commerce: an empirical investigation. IEEE Trans. Eng. Manage., 50(4), 458–469. https://doi.org/10.1109/TEM.2003.819649
- Childers, T. L., Carr, C. L., Peck, J., & Carson, S. (2001). Hedonic and utilitarian motivations for online retail shopping behavior. Journal of Retailing, 77(4), 511–535.
- Chung, S. Y., Kim, G. H., Yang, S. B., Oh, S. H., Hwang, D. Y., Kim, Y. C., & Lee, S. W. (2012). A study of consumer attitude and purchasing behavior toward agricultural products using social network service. The Korean Journal of Food and Nutrition, 25(3), 650–655. https://doi.org/10.9799/ksfan.2012.25.3.650
- Dabholkar, P. A., & Bagozzi, R. P. (2002). An attitudinal model of technology-based self-service: Moderating effects of consumer traits and situational factors. Journal of the Academy of Marketing Science, 30(3), 184–201.
- Dai, H., Milkman, K. L., & Riis, J. (2014). The fresh start effect: Temporal landmarks motivate aspirational behavior. Management Science, 60(10), 2563–2582. https://doi.org/10.1287/mnsc.2014.1901
- Davis F. D. (1993). User acceptance of information technology: system characteristics, user perceptions and behavioral impacts. International Journal of Man-Machine Studies, 38(3), 475–487. https://doi.org/10.1006/imms.1993.1022
- Deepak, K. A., & Velaudham, C. (2019). Marital differences towards consumer buying behaviour. Ajanta, 8(2), 36–45.
- Duarte, P., E Silva, S. C., & Ferreira, M. B. (2018). How convenient is it? Delivering online shopping convenience to enhance customer satisfaction and encourage e-WOM. Journal of Retailing and Consumer Services, 44, 161–169. https://doi.org/10.1016/j.jretconser.2018.06.007
- Eastin, M. S., & LaRose, R. (2000). Internet self-efficacy and the psychology of the digital divide. Journal of Computer-Mediated Communication, 6(1), JCMC617.
- Eastlick, M. A., & Lotz, S. L. (1999). Profiling potential adopters of an interactive shopping service: The early years make a difference. Journal of Retailing, 75(3), 303–329.
- Elliot, S., & Fowell, S. (2000). Expectations versus reality: A snapshot of consumers' experiences with online grocery shopping. Journal of Interactive Marketing, 14(1), 55–62.
- Goldsmith, R. E., & Goldsmith, E. B. (2002). Buying apparel over the Internet. Journal of Product & Brand Management, 11(2), 89–102. https://doi.org/10.1108/10610420210423464
- Grewal, D., Monroe, K. B., & Krishnan, R. (2002). The effects of price-comparison advertising on buyers' perceptions of acquisition value, transaction value, and behavioral intentions. Journal of Marketing, 66(2), 46–59.
- Han, H., & Ryu, K. (2012). The theory of repurchase decision-making (TRD): Identifying the critical factors in the post-purchase decision-making process. International Journal of Hospitality Management, 31(3), 786–797. https://doi.org/10.1016/j.ijhm.2011.09.015
- Iezadi, S., Khosravi, A., & Hassani, M. (2020). COVID-19 pandemic: Impacts, roles, and strategies for sustainable development. Environmental Science and Pollution Research, 27(32), 40603–40608.
- Irawan, I. A. (2018). Effect of trust, convinience, security and quality of service on online purchase decision (consumer case study in tangerang selatan area). Jurnal Ekonomi, 23(1), 114–122. https://doi.org/10.24912/je.v23i1.337
- Jung, H. J., Kim, H., & Oh, K. W. (2016). Green leather for ethical consumers in China and Korea: Facilitating ethical consumption with value–belief–attitude logic. Journal of Business Ethics, 135(3), 483–502. https://doi.org/10.1007/s10551-014-2475-2
- Jusoh, Z. M., & Ling, G. H. (2012). Factors influencing consumers’ attitude towards e-commerce purchases through online shopping. International Journal of Humanities and Social Science, 2(4), 223–230.
- Kaufman-Scarborough, C., & Lindquist, J. D. (2002). Dimensions of internet anxiety: An empirical examination of their effects on consumer behavior. Journal of Public Policy & Marketing, 21(3), 312–324.
- Khalifa, M., & Liu, V. (2007). Online consumer retention: Contingent effects of online shopping habit and online shopping experience. European Journal of Information Systems, 16(6), 780–792. https://doi.org/10.1057/palgrave.ejis.3000711
- Kim, Y. M. (2011). Consumers’ knowledge: The missing element in online purchasing expenditures. Journal of Information & Knowledge Management, 10(02), 159–168. https://doi.org/10.1142/S0219649211002900
- Kim, M. J., Chung, N., & Lee, C. K. (2011). The effect of perceived trust on electronic commerce: Shopping online for tourism products and services in South Korea. Tourism Management, 32(2), 256–265. https://doi.org/10.1016/j.tourman.2010.01.011
- Kim, H. B., Kim, T. T., & Shin, S. W. (2009). Modeling roles of subjective norms and eTrust in customers’ acceptance of airline B2C eCommerce websites. Tourism Management, 30(2), 266–277. https://doi.org/10.1016/j.tourman.2008.07.001
- Kiran, R., Sharma, A., & Mittal, K. C. (2008). Attitudes, preferences and profile of online buyers in India: Changing trends. South Asian Journal of Management, 15(3), 55–73.
- Lee, M. K., & Turban, E. (2001). A trust model for consumer Internet shopping. International Journal of Electronic Commerce, 6(1), 75–91.
- Lenth, R., & Dobler, C. (2005). Regression Models for Categorical Dependent Variables Using Stata (rev.). The American Statistician.
- Li, H. S., & Houston, J. E. (2001). Factors affecting consumer preferences for major food markets in Taiwan. Journal of Food Distribution Research, 32(856–2016–57730), 97–109.
- Ling, K. C., Daud, D. B., Piew, T. H., Keoy, K. H., & Hassan, P. (2011). Perceived risk, perceived technology, online trust for the online purchase intention in Malaysia. International Journal of Business and Management, 6(6), 167. https://doi.org/10.5539/ijbm.v6n6p167
- Lin, C. L., Jin, Y. Q., Zhao, Q., Yu, S. W., & Su, Y. S. (2021). Factors influence students’ switching behavior to online learning under COVID-19 pandemic: A push–pull–mooring model perspective. The Asia-Pacific Education Researcher, 30(3), 229–245. https://doi.org/10.1007/s40299-021-00570-0
- Mathwick, C., Malhotra, N., & Rigdon, E. (2001). Experiential value: Conceptualization, measurement and application in the catalog and Internet shopping environment. Journal of Retailing, 77(1), 39–56.
- Menon, S., & Kahn, B. E. (2002). Cross-category effects of induced arousal and pleasure on the internet shopping experience. Journal of Retailing, 78(1), 31–40
- Moeller, S., Fassnacht, M., & Ettinger, A. (2009). Retaining customers with shopping convenience. Journal of Relationship Marketing, 8(4), 313–329. https://doi.org/10.1080/15332660903344644
- Mokhtarian, P. L., & Tang, W. L. (2013). Trivariate probit models of pre-purchase/purchase shopping channel choice: Clothing purchases in northern California. In Hess, S., & Daly, A. (Eds.), Choice Modelling (pp. 243–273). Edward Elgar Publishing.
- Mummalaneni, V., Meng, J., & Elliott, K. M. (2016). Consumer technology readiness and e-service quality in e-tailing: What is the impact on predicting online purchasing? Journal of Internet Commerce, 15(4), 311–331. https://doi.org/10.1080/15332861.2016.1237232
- Ngoh, C. L., & Groening, C. (2022). The effect of COVID-19 on consumers’ channel shopping behaviors: A segmentation study. Journal of Retailing and Consumer Services, 68, 103065. https://doi.org/10.1016/j.jretconser.2022.103065
- Oliver, R. L. (1977). Effect of expectation and disconfirmation on postexposure product evaluations: An alternative interpretation. Journal of applied psychology, 62(4), 480.
- Prochaska, J. O., & DiClemente, C. C. (1983). Stages and processes of self-change of smoking: Toward an integrative model of change. Journal of Consulting and Clinical Psychology, 51(3), 390. https://doi.org/10.1037/0022-006X.51.3.390
- Raman, P., & Pashupati, K. (2005). Online privacy: Consumer concerns and technological competence. In Krishnamurthy, S. (Ed.), Contemporary Research in E-Marketing (Vol. 1, pp. 200–225). IGI Global.
- Rogers, E. M. (1995). Diffusion of innovation. Free Press.
- Satit, R. P., Tat, H. H., Rasli, A., Chin, T. A., & Sukati, I. (2012). The relationship between marketing mix and customer decision-making over travel agents: An empirical study. International Journal of Academic Research in Business and Social Sciences, 2(6), 522 Omics, 2(6), 72–78.
- Shim, S., Eastlick, M. A., Lotz, S. L., & Warrington, P. (2001). An online prepurchase intentions model: The role of intention to search. Journal of Retailing, 77(3), 397–416.
- Somervuori, O., & Ravaja, N. (2013). Purchase behavior and psychophysiological responses to different price levels. Psychology & Marketing, 30(6), 479–489. https://doi.org/10.1002/mar.20621
- Suwanvijit, W., & Promsa-Ad, S. (2009). The insight study of consumer life-styles and purchasing behaviors in Songkhla province, Thailand. International Journal of Marketing Studies, 1(2), 66. https://doi.org/10.5539/ijms.v1n2p66
- Tian, D., Hao, S., Mu, W., Shi, J., & Feng, J. (2021). Chinese consumers’ selection of wine purchasing channels: Influence of demographic characteristics, perceived value factors, social factors and wine knowledge. British Food Journal, 124(11), 3522–3539. https://doi.org/10.1108/BFJ-07-2021-0789
- Wolfinbarger, M., & Gilly, M. C. (2001). Shopping online for freedom, control, and fun. California Management Review, 43(2), 34–55.
- Wolk, A., & Ebling, C. (2010). Multi-channel price differentiation: An empirical investigation of existence and causes. International Journal of Research in Marketing, 27(2), 142–150. https://doi.org/10.1016/j.ijresmar.2010.01.004
- Yoon, C. (2002). The antecedents and consequences of trust in online-purchase decisions. Journal of Interactive Marketing, 16(2), 47–63.
- Youn, S. Y., Lee, J. E., & Ha-Brookshire, J. (2021). Fashion consumers’ channel-switching behavior during the COVID-19: Protection motivation theory in the extended planned behavior framework. Clothing and Textiles Research Journal, 39(2), 139–156. https://doi.org/10.1177/0887302X20986521
- Zeithaml, V. A., & Berry, L. L. (1990). Delivering quality service: Balancing customer perceptions and expectations. Free Press.