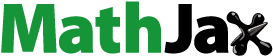
Abstract
Over the recent years, blockchain, a digitalization phenomenon, has leveraged its superior features to remodel the relationships of logistics partners. This cutting-edge technology has brought a faster, more transparent, and cost-effective logistics industry. This study, therefore, aims to investigate the behavioral intention to use the blockchain of individuals who work in logistics companies in Ho Chi Minh City (HCMC), Viet Nam, through the Unified Theory of Acceptance and Use of Technology (UTAUT) and its extended factors. Accordingly, non-probability sampling with convenience sampling has been chosen. A questionnaire was used to collect data from logistics workers before exploring and clarifying factors affecting the users’ intention, namely performance expectancy (PE), effort expectancy (EE), social influence (SI), and facilitating conditions (FC). In addition, experience (EXP) was also expected to influence the relationships. Therefore, a multi-analytical hybrid structural equation modeling-artificial neural network (SEM-ANN) approach was used to evaluate the gathered data empirically. The expert panel examined the established questionnaire through face validity and content validity to ensure the validity and reliability of the survey instrument. The findings revealed the different positive impacts of factors on the intention to use blockchain. While the result of the PLS-SEM technique is a descending order impact of PE, FC, EE, SI, and EXP was found that have no meaningful effect on the relationships, the ANN approach produces a surprising conclusion when SI ranks first in the magnitude of influence.
1. Introduction
Over the past couple of years, logistics—the flow and storage of goods, services, and associated information—had attracted widespread attention when global logistics were disrupted by the COVID-19 pandemic, which led to the lack of materials in production. Logistics directly connects manufacturing processes throughout the supply chain, affecting production efficiency and organizational coordination (X. Wang et al., Citation2015). For this reason, improving and innovating logistics procedures and functions is necessary to protect the continuity of trade activities and the global economic system. In line with this, technological advancements have pervaded and affected every aspect of production and business activities where standards and productivity are enhanced markedly. Indeed, one of the most recent tremendous technological advances that profoundly influences business management is the blockchain. By using cryptographic techniques and distributed consensus algorithms, blockchain could record transactions automatically in an immutable and believable manner (Tapscott & Tapscott, Citation2016). Thus, blockchain is a combined technology between computer science, cryptography, and economics. It allows businesses to provide a new distribution channel in addition to the ones they already have and eliminate the intermediaries. Information and communication technology systems also have automated logistics network business processes (S. E. Chang et al., Citation2019). The logistics business is also moving toward a decentralized, digital structure. The digital logistics system demands new financial transaction and data exchange technologies and complicated hardware systems. Distributed logistics marketplaces offer financial transparency and mature supply chain networks from decentralized and digitalized logistics systems. Blockchain technology, a new distributed ledger technology, facilitates peer-to-peer trading in these distributed freight logistics marketplaces, helping decentralized and digital logistics systems overcome their challenges (Schuetz & Venkatesh, Citation2020). Consequently, blockchain is expected to disrupt inefficient models, remodel the logistics relationships (S. E. Chang et al., Citation2019; Schuetz & Venkatesh, Citation2020), and bring a new digitally transformed world of security, transparency, authenticity, and efficiency.
Several studies have researched blockchain and its benefits in many sectors and countries. While most papers have focused on the empirical investigation of some reasons to using blockchain and its benefits for enterprises (Toufaily et al., Citation2021), other studies have concentrated on the specific usage of blockchain in many fields, including supply chain (L. W. Wong et al., Citation2020; Lu & Xu, Citation2017), finance (Chen & Bellavitis, Citation2020; L. W. Wong et al., Citation2019), energy systems (T. Wang et al., Citation2022), business management (Pal et al., Citation2021), and even medical information data management (Qu, Citation2021). The studies that were mentioned above identify specific areas for improvement in management operations, including personnel sufficiency, maintenance, training, and motivation, all the way up to goal attainment. Nevertheless, three significant issues motivate this study.
Firstly, virtually all the articles have mainly concentrated on the empirical examination of specific grounds for implementing blockchain and its influences on businesses rather than examining the variables influencing individuals’ intentions to use it. Indeed, a current study by (Garg et al., Citation2021) has measured the perceived benefits of implementing blockchain technology in the banking sector, in which there is an affirmation that, in the banking business, blockchain technology has the potential to reduce operational costs and improve customer experience.
Secondly, fewer studies have paid attention to the use of blockchain in the logistics sector than in other sectors. As can be seen, logistics is less well-known than the supply chain and finance sector since it is the back of the production and the consumption which control the flow of materials and goods. Therefore, previous papers investigate blockchain usage in the big picture of the products and services that include logistics in operation. For example, L. W. Wong et al. (Citation2019) (L. W. Wong et al., Citation2019) have researched Malaysian SMEs’ usage of blockchain in operations and supply chain management, and Garg et al. (Citation2021) (Garg et al., Citation2021) have concentrated on the impact of blockchain on the banking sector.
Thirdly, in the context of Viet Nam, since blockchain is such a new concept with many people, namely technology users, white- or blue-collar workers, and even entrepreneurs, the application of this technology is not popular recently. Moreover, there are few research articles on blockchain in logistics in this country, particularly in Ho Chi Minh City—a major economic center. Therefore, based on those mentioned above three significant gaps, it is necessary to find out the intention to use the blockchain of people who will use it in the future, as well as why businesses are now hesitant to adopt this technology while it is well-known worldwide. Consequently, this study aims to investigate factors impacting the behavioral intention to adopt blockchain in logistics companies in Ho Chi Minh City (HCMC), where many domestic and foreign logistics enterprises are concentrated, and the forefront of changes in digital technology are deployed. Following this direction, the Unified Theory of Acceptance and Use of Technology (UTAUT), comprised of eight prior foundational theories, is an appropriate approach for learning about blockchain adoption in the logistics industry. According to Venkatesh et al. (Citation2003), the UTAUT is a reliable model, with 56 percent of variation explained by behavioral intention and 40 percent by technology use. Indeed, many existing studies use the UTAUT model in various contexts (Jadil et al., Citation2021; Rahi et al., Citation2019). As can be seen, the UTAUT is recently the most popular model which is used to research human behavior in technology adoption. Hence, there is a need to develop a conceptual framework grounded in the fundamental knowledge from this model. As a result, the research question is, “What factors influence the intention to use blockchain in logistics companies in HCMC?”.
This research is intended to enrich the literature on blockchain adoption in the logistics sector. Through this, scholars and practitioners will understand more clearly the factors influencing the behavioral intention of individuals to use an emerging technology like blockchain in the logistics sector in developing countries. Furthermore, this study also proposes the expected influences of blockchain on the development of the Vietnamese logistics sector in the future when it is still in its infancy in Viet Nam. Just in time—one of the essential orientations that all logistics companies need to enhance day by day, and with blockchain, logistics companies could connect all stages in time by updating precisely the status of goods, cash and payment flows during the transportation. This advantage helps relevant partners have an ability to solve arising problems promptly and reduce operation time as well as cost because there are many unexpected situations when shipping goods from material to products. In addition, implementing blockchain requires significant financial costs, so companies need to be prepared for this situation. As a result, this paper not only helps logistics enterprises enhance the comprehension of blockchain adoption for the best preparation but also provides some blockchain aspects which need to be improved for application developers.
This study is divided into seven sections. The first section is on the introduction of this research. Following this, section 2 briefly overviews the relevant literature, the underlying definitions of blockchain, and the technology acceptance theories. Section 3 discusses our study framework before the research methodology is derived in Section 4, and the findings are emphasized in Section 5. As for the remainder, Section 5.6.6.1 discusses such findings, while some practical managerial and theoretical implications to practitioners and researchers are also provided. Finally, Section 6 concentrates on the research limitation and future direction for future studies.
2. Literature review
2.1. Unified Theory of Acceptance and Use of Technology (UTAUT)
The Unified Theory of Acceptance and Use of Technology (Venkatesh et al., Citation2003) seems to be a popular model used in many sectors, particularly in recent economic papers. The UTAUT aims to explain user intentions to use information technology and subsequent usage behavior. The UTAUT does not only base on similar factors of previous models, such as the Theory of Reasoned Action (TRA) (Ajzen, Citation1991), Theory of Planned Behavior (TPB), Technology Acceptance Model (TAM), Model of PC Utilization (MPCU) (Thompson et al., Citation1991), Innovation Diffusion Theory (IDT), Combined TAM and TPB (C-TAM-TPB) (Taylor & Todd, Citation1995), Social Cognitive Theory (SCT) (Bandura, Citation1986), and Motivational Model (MM) (Davis et al., Citation1992), but also recognizes that broader relevant components may encourage or reject innovation acceptance in technology (Charness & Boot, Citation2015).
In 2003, Venkatesh et al. (Citation2003) proposed the UTAUT, developed through eight prominent theories mentioned above, creating a more robust new basement and a more complex model to explore user acceptance of new information technology (Yu, Citation2012). Since TAM lacks external factors like social influence, user training, features of systems, and other factors, it has some limitations in explaining the technology adoption (Taherdoost, Citation2018). However, everything is more explicit with UTAUT, the latest combined model of many previous models. Indeed, according to (Kripanont, Citation2007), from a theoretical perspective, UTAUT provides a refined view of why people determine their behavioral intention. In other words, it analyzes the relationship between causes and human decisions through acceptance or rejection. In line with this, from UTAUT, performance expectancy describes the usefulness that people believe can make a better performance, in other words, whether it can increase the efficiency of employee performance and the company’s operation.
On the other hand, effort expectancy is how users believe new technology is accessible and combined with other appliances. Therefore, effort expectancy is approximately as same as perceived ease of use (TAM) and complexity (DOI, MPCU) (Rahi et al., Citation2019). Another factor in this model that impacts technology adoption is social influence, the degree that people are impacted by surrounding people who want an individual to use a new information system. Other people can be families, colleagues, friends, or anyone they believe in. Finally, facilitating conditions are defined as the degree to which a person accepts that an organizational and specialized foundation exists to support the implementation of the new technology framework. The facilitating condition is similar to perceived behavioral control and compatibility (Dang et al., Citation2022; Rahi et al., Citation2019). By contrast, according to this model, PE, EE, FC, and SI not only directly influence behavioral intention but also have a different impact on the intention depending on different moderators, such as gender, age, voluntariness, and the experience of users.
From a theoretical perspective, UTAUT offers a more detailed look at how the factors influencing desire and behavior change over time. For instance, Jure Erjavec and Anton Manfreda (Citation2022) (Erjavec & Manfreda, Citation2022) have used UTAUT to identify factors that impact the behavioral intention of adopting online shopping during the COVID-19 pandemic and social isolation. In other words, people’s behavior is influenced by many factors which can change over time and living conditions. Similarly, Chang et al. (Citation2022) (M. Chang et al., Citation2022) have learned about the behavioral intention of using a new technology—a virtual tourism cryptocurrency—implemented in Jeju, Korea, tourism industry via UTAUT because this model gives SMEs meaningful information about the deployment of blockchain technology. Therefore, UTAUT highlights the most critical individual-level factors influencing technology adoption and discusses the contingencies that could intensify or limit these beneficial effects. As a result, there is a reason to affirm that UTAUT is the appropriate model for this study.
2.2. Blockchain and logistics
Blockchain is introduced as a new step forward in payment and transaction technology which has many unique features that bring a new digitally transformed world of security, transparency, authenticity, and efficiency (H. Nguyen & Nguyen, Citation2021). By allowing numerous participants in logistics to see the digital footprint of a (digitized) product at any point in its lifecycle (from production to distribution to retail), blockchain technology enables the creation of transparent logistics. Customers are more likely to believe in a product’s authenticity when using blockchain technology because of its immutability (Ozdogru, Citation2019). In addition, arguments and disagreements may be avoided over time-sensitive problems by using timestamping (the act of defining a chronological order among sets of events) in the blockchain to verify the presence of specific data at a moment in time. As blockchain can store various information, including ownership, location, product specification, and pricing, information completeness may also be improved (Y. Wang, Citation2021). These advantages are aspects in which the logistics industry is very interested in making the value chain more efficient. Being first released in the bitcoin protocol as a distributed ledger technology, blockchain can eliminate the dependence on the third party in any transactions. Thus, blockchain can reduce the time of financial transactions among different partners in the network during the operation via remittances and online payments. Furthermore, with blockchain, every transaction and every stage will be executed automatically after the smart contract is signed through the information system with the Internet. Indeed, smart contracts significantly impact network data sharing of logistics when it collects and transmits data in nearly real-time to enhance the speed of decision-making, financial arrangements, and process management. All along the logistics, documents such as purchase orders, invoices, change orders, receipts, ship notifications, and other trade-related papers, as well as data on available stock, may and should cascade with smart contracts (Ozdogru, Citation2019). In conventional database architecture designs, “stored procedures” are used to automate contractual terms. When executed in a blockchain environment, smart contracts vary from traditional contracts in that their outcomes may be independently audited and verified by anybody in the network. When the customer receives the cargo, the smart contract might trigger the fund transfer to the supplier. With GPS tracking, returned goods might be recorded in real-time, with the position recorded in the blockchain and a signal sent for instant reimbursements (Y. Wang, Citation2021). That is why it can reduce time operation and narrow payment gaps of logistic partners by integrating delivery and payments (L. W. Wong et al., Citation2020). In other words, according to Kamble et al. (Citation2019), finance procedures are made more straightforward, and logistics management and regulation are more efficient through smart contracts (Casado-Vara et al., Citation2019).
On the other hand, transparency is one of blockchain’s most significant advantages to users. When a block is created, the transactions are marked in chronological order. Then they are connected within a chain after being verified that it is a valid transaction by the parties. Once the system has recorded the information, the blockchain will release a compilation of unchangeable past transaction documents to the public at nearly the same time as the creation. Hence during the procedure (Hawlitschek et al., Citation2018), users can add new information instead of editing a block in a chain. As a result, partners could share information promptly and respond quickly to reduce fraud risks and increase trust among parties in business operations (L. W. Wong et al., Citation2020). Information flows are the root of the logistics industry to perform every stage in operation with no trouble, from forecasting the data to classifying feedback. Hence, blockchain technology allows for more secure tracking since once data is stored in the blockchain, it is secured and prevented from unauthorized access (Y. Wang et al., Citation2019). Collectively, this offers a more reliable network for enterprises and helps them raise the products’ integrity which could gain consumer trust (Montecchi et al., Citation2019). Recently, many multinational corporations have adopted blockchain to enhance operation efficiency and achieve speed, risk, quality requirements, and cost reduction. Nevertheless, blockchain acceptance still has been in its infancy in Viet Nam and some countries, even though it could bring more value to enterprises and meet the high requirement.
3. Hypotheses development
In order to choose a model to analyze and explain the behavioral intention of adopting blockchain in logistics companies, in which its almost typical factors are studied in many papers, UTAUT is chosen to be the primary model and basic theory to propose in this study. Following some previous studies in this sector, and according to UTAUT, performance expectancy, effort expectancy, facilitating conditions, and social influence were found as predominant factors which impact blockchain technology acceptance. Moreover, this paper will propose a mediator to extend the influence of experience on the relationship among variables. However, the usage behavior should not be mentioned in the model since blockchain is a brand-new technological advance and is not implemented widely throughout Viet Nam.
3.1. Performance expectancy
Performance expectancy is the extent to which an individual believes that using innovative technology will help him or her to have a better job performance (Venkatesh et al., Citation2012). In many previous studies, PE was shown to be a key reason for adoption intentions for crypto-currency (Jung et al., Citation2019) and supply chain (Casado-Vara et al., Citation2019; Y. Wang, Citation2021), which is consistent with other literature (L. W. Wong et al., Citation2021; Venkatesh et al., Citation2003). In the context of this paper, performance expectancy refers to the ability that employees in logistics companies will use blockchain technology if they improve their productivity (Queiroz & Fosso Wamba, Citation2019). Indeed, it reflects the perception of the new system’s advantages for employees’ job routines. Therefore, blockchain improves waste, paperwork delay, and the convenience and speed of working and identifying troubles. On the other hand, it also suggests that they will adopt when they believe that blockchain contributes to the enhance firm’s performance and profits. One of the significant considerations that specifically influences blockchain acceptance is expected to be performance expectation (Hawlitschek et al., Citation2018; Montecchi et al., Citation2019). Thus, the following hypothesis is formulated:
H1.
Performance expectancy (PE) positively influences the behavioral intention (BI) to use blockchain.
3.2. Effort expectancy
Effort expectancy (EE) refers to “the extent of ease connected with the use of a system” (Venkatesh et al., Citation2003). In other words, it shows the decision of potential users if they realize that new technology is easier for them to learn so that they do not need to spend more effort (C. H. Wong et al., Citation2015). In the logistics industry, people will be willing to use blockchain if it is simple and does not need to spend too much time and effort to learn to use. Many enterprises have applied blockchain and gained more benefits than others. The fact proves that blockchain is not difficult for logistics workers to understand and implement. Therefore, it is anticipated that the easier the blockchain technology to use, the higher the users’ behavioral intention (Alalwan et al., Citation2017). Moreover, users will accept more quickly with simple requirements from modern technology, such as blockchain. Therefore, we hypothesize:
H2.
Effort expectancy (EE) positively influences the behavioral intention (BI) to use blockchain.
3.3. Facilitating condition
Facilitating conditions are defined as the extent to which people believe existing technical infrastructures could support them using a new information system (Venkatesh et al., Citation2003). Following previous literature, many things impact the intention of using technology, such as operating systems, internet speed, and integration with other existing systems (Francisco & Swanson, Citation2018; Garg et al., Citation2021; Yee-Loong Chong et al., Citation2015). Particularly in the logistics sector, transactions and contracts are stored and enforced in the cloud, called the blockchain. Hence, an important mission is to prepare and improve the information technology system. Therefore, for this study, facilitating conditions refers to an individual’s perception of having specific services, such as blockchain infrastructure and technical expertise, available to them when considering (L. W. Wong et al., Citation2020). If employees find that their firms have the available infrastructure and the ability to meet the requirements to support blockchain usage, they will be ready to adopt blockchain technology. Hence, we assume the following:
H3.
Facilitating condition (FC) positively influences the behavioral intention (BI) to use blockchain.
3.4. Social influence
Social influence (SI) is defined as “the degree to which an individual perceives that important other people believe he or she should use the new system” (Venkatesh et al., Citation2012). In this study context, social influence refers to how the employees clearly understand the recommendation to use blockchain from people they believe. In fact, at the individual level, some existing papers have highlighted that users are more impacted by the perspectives and experiences of friends, colleagues, and family members (Irani et al., Citation2017). As mentioned above, social influence is the same as the subjective norm in TAM2 and the social norms in the TRA model. Therefore, it is anticipated that social influence has a positive impact on the behavioral intention to adopt blockchain (Chong, Citation2013; Hawlitschek et al., Citation2018; L. W. Wong et al., Citation2021), so this study proposes the hypothesis:
H4.
Social influence (SI) positively influences the behavioral intention (BI) to use blockchain.
3.5. Experience
The expression “moderating variable” refers to a term that appears when the interaction among constructs is weak, contradictory, or non-existent, as stated by Sekaran & Bougie (2010) (Alalwan et al., Citation2017). Whereby in order to eliminate or reinforce the relationships, the moderator variables are the factors that researchers usually use in two forms (Baron & Kenny, Citation1986):
Qualitative, like gender, culture, and others
Quantitative, such as age, weight, and others.
In this research, the desire to adopt a new technology system like blockchain seems affected by three moderators: age, sex, and voluntaries. In many cases, the moderator voluntaries are more subjective when people may accept using blockchain without reason. Furthermore, age and sex also do not impact the intention to use a blockchain because they do not belong to reason but nature. People can choose a thing to buy depending on their age and sex; however, they cannot decide to adopt blockchain for a similar reason. Therefore, experience, one of four moderators developed by Venkatesh et al. (Citation2003) (Ozdogru, Citation2019), is put in the framework to moderate and clarify its influence on the direct relationship between PE, EE, FC, SI, and Behavior intention of using blockchain. Experience is understood as the number of years individuals use a particular information technology (Venkatesh et al., Citation2003). According to Venkatesh & Bala (2008), experience is believed to significantly affect an individual’s reaction toward adopting blockchain technology. Several studies comparing experienced users to other users have explored that the factors influencing the purpose of using blockchain differ between them (Taylor & Todd, Citation1995). In the context of this study, the ability to perform tasks is different among logistics workers with different experiences. People with more experience will be more familiar with papers, tools, technologies, and logistics procedures; thus, they will be willing to use blockchain sooner than the less experienced others. Moreover, some extensive papers suggest that users could change their behavioral intention after having an experience in using modern technologies (Liao & Lu, Citation2008). Furthermore, Venkatesh et al. (Citation2003) stated that the influence of facilitating conditions on behavioral intention was only significant when examined in conjunction with the moderating effect of experience. As a result, it is anticipated that experience plays a crucial role in moderating the relationship among factors impacting the usage and the behavior intention of using blockchain in logistics, so the following hypothesis is formulated:
H5a.
Experience moderates the influence of Performance Expectancy (PE) on the Behavioral intention (BI) to use blockchain.
H5b.
Experience moderates the influence of Effort Expectancy (EE) on the Behavioral intention (BI) to use blockchain.
H5c.
Experience moderates the influence of Facilitating Conditions (FC) on the Behavioral intention (BI) to use blockchain.
H5d.
Experience moderates the influence of Social Influence (SI) on the Behavioral intention (BI) to use blockchain.
4. Research methodology
4.1. Target population
The target population of this research is people who work in logistics companies in Ho Chi Minh City (HCMC), where over 70 percent of domestic enterprises and subsidiaries of global corporations in the logistics field are located in Viet Nam. HCMC was also selected for this study since it has converged nationwide with diversified knowledge, experience, and opinion. However, due to the absence of a sampling frame of logistics companies in HCMC, and the list of employees, this study uses non-probability sampling. In addition, judgmental sampling was engaged in this study because, based on some previous papers, the researchers chose the time-and cost-effective samples in judgmental sampling.
4.2. Measures, questionnaire design, and data collection
In the context of this study, surveys were used to collect data. Since surveys provide research data based on real-world observation, it becomes more appropriate than the other types in studying individuals’ behavior (Kelley et al., Citation2003). The current study employed an electronic questionnaire provided through Google Forms. All items in the questionnaire were modified and updated from previous research based on the results of an extensive literature review to ensure content validity. Then, an expert panel would evaluate the chosen items to validate their content validity. First, the questionnaire was modified from previous research that was performed in English. After that, it was translated from English to Vietnamese before being conducted in Vietnamese, the official language and the language used by Vietnamese logistics staff the most, and then back to English to ensure translation equivalence. In detail, performance expectancy (PE) is adopted from (Venkatesh et al., Citation2003), effort expectancy (EE) is adopted from (L. W. Wong et al., Citation2019; Venkatesh et al., Citation2003), social influence (SI) is adopted from (Venkatesh et al., Citation2003, Citation2012), facilitating conditions (FC) is adopted from (Venkatesh et al., Citation2003, Citation2012), and behavioral intention (BI) is modified from (Venkatesh et al., Citation2003) (Appendix). Furthermore, the 7-point Likert scale was chosen to measure because of its advantages, including the dispersion increase and the neutral-response decrease; the range from (1) strongly disagree to (7) strongly agree. As recommended by the PLS literature, in a research model, a sample size of ten times the most complex relationship is requested (J. Hair et al., Citation2017). As a result, the minimum sample size required is 10 × 8 = 80. On the other hand, according to the statistical software G*Power version 3.1, for an effect size, = 0.15, probability of error, α = 0.05 and power level, (1- β) = 0.8 and the number of predictors = 8, a minimum sample size of 109 is recommended.
4.3. Data analysis strategy
Component-based SEM (PLS route modeling) was chosen to address the shortcomings of covariance-based SEM with regard to distributional properties, sample size, model complexity, measurement level, identification, and factor indeterminacy. Model validation commonly uses covariance-based SEM, which necessitates a sizable data set (Tenenhaus, Citation2008).
CB-SEM (such as AMOS, LISREL) is appropriate if theory testing is the study’s goal, while VB-SEM (such as SmartPLS, PLS-Graph) is appropriate if theory development and relationship prediction are the goals of the investigation. In addition, VB-SEM can handle a complicated model (six or more constructions and/or twenty or more items (Hew et al., Citation2017)). Our research delves into what influences logistics company employees to take action regarding blockchain. Therefore, PLS is the best appropriate method for our study since it is the prediction-focused SEM approach and can handle model complexity.
The factors influencing behavioral intention to use blockchain were validated using an ANN. The relevance of the predictors produced by SEM was also ranked using ANN. Non-linear and non-compensatory correlations, which PLS-SEM cannot discover, can be identified using the ANN model. Nevertheless, ANN can detect both linear and non-linear associations (L. T. Nguyen et al., Citation2022) and is robust against violations of multivariate assumptions. As a result, the unique approach has improved the model’s predictive ability and made it more resistant to noise.
5. Data analysis and results
5.1. Statistical analysis
This study surveyed 414 HCMC-based logistics industry professionals for their insights. Table shows that females comprise 71.74 percent of the sample, while men account for 28.26 percent. Additionally, the percentage of working-age women in Vietnam is one of the highest of any country in the top 15 (T. Nguyen, Citation2022). In addition to this, the global logistics business is seeing a shift in the gender gap as a result of more women applying for jobs, particularly in Europe (Whitehouse, Citation2020). Eighty-four point one percent of the population is between the age bracket of 22–30, while only 11.59 percent falls into the age bracket of 31 and above. Companies with a disproportionately high number of women and young people in management positions might benefit significantly from innovative technologies like blockchain in logistics (Gligor et al., Citation2022). Among those who responded, 82.37 percent have bachelor’s degrees or above, but just 17.63 percent have master’s or doctoral degrees. Moreover, 77.72 percent of individuals have experience ranging from one to two years, 17.92 percent of participants have experience ranging from three to four years, and 4.36 percent of respondents have experience ranging from five years or more.
Table 1. Descriptive statistics
5.2. Common method biases
Due to the data for the exogenous and endogenous variables being gathered from a single source, common method bias can emerge. In order to address this issue, both procedural and statistical procedures were used in this research data analysis (Leong et al., Citation2018; Teck Soon & Sharifah, Citation2017). Firstly, procedural-wise, there was insurance that respondents’ identities remained anonymous, and the responses would not be judged as true or false; thus, they only needed to answer candidly to all the questions. Secondly, Harman’s single-factor analysis was conducted on the statistical aspect, and the finding showed that a sole component explained only 47.9 percent of the total variance. This is less than the threshold of 50 percent, so there is no CMB issue (C. H. Wong et al., Citation2015). Consequently, the insignificant effect of CMB on the results has been verified.
5.3. Assessing the outer measurement model
Before testing the hypotheses in the inner model, it is necessary to verify the assessment of the outer model (measurement model) (structural model). First, Table shows that the values for internal consistency reliability, a method for measuring construct dependability, are all over the suggested threshold value of 0.70 (T. Nguyen, Citation2022). Composite dependability values are shown to be more than the 0.70 threshold set by Hair Jr. et al., as shown by the findings in the same table (2016). Thus, the
and composite reliability tests confirm substantial dependability across all constructions.
Table 2. Loading, composite reliability, Dijkstra Henseler, and average variance extracted
The validity of the measurement model is next checked for convergent and discriminant factors. Many items with similar ideas are evaluated using “Convergent Validity” (CV). It is advised to evaluate the CV using the average variance extracted (AVE) and the value of the outer loading, as Hair Jr et al. (2016) suggested. For AVE to be validated, its value must be larger than 0.50; for the CV to be considered validated, the outside loadings must be bigger than 0.70. Table shows that all AVEs are rather big, with values more than 0.50, and all outer loadings have values greater than 0.70. The reliability of this work is therefore shown. Nevertheless, Discriminant Validity (DV) ensures that the relevant items load heavily on the construct of interest while loading poorly on irrelevant constructs. Non-parametric bootstrapping was used to calculate the Heterotrait-Monotrait (HTMT) inference ratio of correlations to evaluate DV (J. Hair et al., Citation2017). All values were less than one, and the 95th percentile confidence interval was presented as indicated in Table . This result shows statistically significant differences between each item in the sample. Also, the “Fornell-Larcker criteria test” (Fornell & Larcker, Citation1981) for discriminant validity reveals, as shown in Table , that the correlation coefficients are lower than the square root of AVE. Table also includes an examination of cross-loadings, which were found to be universally high for the connected constructs and low for the unrelated ones (Henseler et al., Citation2014). Given this result, the DV is ascertained (Ab Hamid et al., Citation2017).
Table 3. Hetero-Trait-Mono-Trait (HTMT inference)
Table 4. Cross loadings
Table 5. Fornell-Larker criterion
5.4. Inspecting the inner structural model
In the next stage, to assess the global goodness of appropriateness of both the estimated and saturated models, the Standardized Root Mean Square Residual (SRMR) was deployed (Henseler et al., Citation2016). It was found that the saturated and estimated models all had a value of 0.041 (Table ). This finding is lower than 0.08, demonstrating that the model was well-fitting (Hu & Bentler, Citation1999). Additionally, the collinearity test was used to examine the presence of highly connected components before evaluating the inner structural model. Since the variance inflation factors (VIF) values for all constructs were lower than the recommendation of 5.0, the issues from multicollinearity do not exist (G. W. H. Tan & Ooi, Citation2018).
Table 6. Outcome of the structural model examination
The strength of a path is evaluated by its p-value when testing the hypothesis. A p-value is considered significant when it is less than 0.05. The path coefficients, t-values, and p-values for the structural model are shown in Figure and Table . 4 out of 8 hypotheses were supported. Overall, H1, H2, H3 and H4 were supported, while H5a, H5b, H5c, H5d were the unsupported hypotheses. As can be seen, PE (β = 0.397, p < 0.000), EE (β = 0.164, p < 0.004), FC (β = 0.271, p < 0.000), and SI (β = 0.082, p < 0.024) have a crucial positive impact on the behavioral intention to use blockchain. Conversely, the influence of EXP on relationships between independent variables and BI was found to be a non-significant effect when all p-values of H5 were much greater than 0.05. In other words, experience does not substantially impact the intention to use blockchain. In addition, Table illustrates that all of the Q2 values for BI are positive and greater than 0, demonstrating that the model has acceptable forecasting ability (Enaizan et al., Citation2020; L. T. Nguyen et al., Citation2022). By contrast, while the root mean squared error (RMSE) indices in the PLS-SEM model of almost items do not exceed those in the linear model benchmark, the BI1‘s one is an exception. The model offers a medium level of predictability.
Table 7. PLS predict
5.5. Predictive relevance and effect size
Hair Jr et al. (2016) proposed that cross-validated redundancy be utilized to determine Stone-Geisser’s Q2 when measuring predictive relevance (J. F. Hair et al., Citation2016). Table indicates that the Q2 value for BI, EE, FC, PE, and SI are 0.633, 0.596, 0.742, 0.564, and 0.746, respectively. All the Q2 values of constructs are greater than zero; hence, it is asserted that the model has predictive relevance. On the other hand, Cohen’s f-square () is the common measurement to measure effect size, which measures an independent variable’s relative importance or influence on the dependent variable (Cohen, Citation2013). Moreover, a small effect size is recommended to have a value of
from 0.02 to 0.149, a medium effect size
from 0.15 to 0.349, and a large effect size of
from 0.35 and beyond. Therefore, it can be seen from Table that SI does not affect BI with
0.013, while EE and FC on BI have a small effect size with
= 0.027 and
= 0.111, respectively. Finally, PE on BI has a medium effect size with
= 0.174.
Table 8. Predictive relevance,
Table 9. Effect Size,
5.6. Importance-performance map analysis
The outcomes of the PLS-SEM study are supplemented by an examination of the importance-performance maps (IMPA). IMPA supports the identification of key target constructs with a significant aggregate effect but poor performance, allowing strategic decisions to be made. Table depicts that the most significant precursors of BI to use blockchain are PE (0.405), FC (0.231), EE (0.161), and SI (0.065). On the other hand, for a performance level, PE (65.529) and EE (65.424) are the most predictive of BI to use blockchain, followed by FC (59.633) and SI (44.069). One point worth noting is that although FC is more important than EE, the performance of EE is more significant than FC’s one.
Table 10. Importance of performance map analysis
5.7. Artificial neural network analysis for blockchain adoption
The neural network method is often used to forecast IT/IS behavioral intention factors in mobile commerce (Leong et al., Citation2018), mobile payment (L. T. Nguyen et al., Citation2022), and supply chain management (Lim et al., Citation2021). Past research used this strategy to predict the influence of adoption factors in diverse scenarios. A good neural network is needed to determine input variables. The SEM inputs to the neural network are necessary and dependable (G. W. H. Tan et al., Citation2014).
As a new technology version, blockchain may not provide enough information for enterprises to recognize crucial behavioral intention factors and allocate resources effectively. SMEs must evaluate the factors that are most significant before allocating resources. The selected intention variables found using SEM-PLS are not prioritized, so they may not have offered enough information for enterprises to allocate their resources for blockchain adoption. SEM can only find linear associations, simplifying the intention to use blockchain. The neural network can recognize linear and non-linear interactions without distribution assumptions (Lim et al., Citation2021). The neural network method is more robust and provides higher prediction accuracy than SEM (L. W. Wong et al., Citation2021). To solve these issues, SEM-ANN was used. Thus, Smart PLS variables were used to further neural network analysis. The input segment included four distinct SEM parameters; the outcome component included behavioral intention.
This study analyzed networks with 1–10 hidden nodes to find unseen nodes (Lim et al., Citation2021). A 10-fold cross-validation technique was used for neural network analysis because it was complicated enough for dataset building without introducing errors. This research created the neural network model using SPSS version 25, a simple and effective software for academics and scientists. We calculated their Root Mean Squared Error (RMSE) to measure ANN’s prediction capacity. shows that the mean RMSE for the training data (0.840) and the testing data (0.787) is relatively small. The result indicates high predicted accuracy and good data fit for the ANN model developed in Figure . Tables shows how the number of hidden neurons with non-zero synaptic weight can be used as a proxy for the importance of reflective independent variables in ANN models. Table displays the results of a sensitivity study that ordered the relative importance of each significant predictor. The results showed that SI was the most significant predictor of sustainable performance (with a normalized relative relevance of 100%), followed by FC, EE, and PE. The PLS-SEM and ANN rankings are based on the studied variables’ Path Coefficient and Normalized Relative Importance, respectively. The results are interesting, as PE ranks fourth in ANN but first in PLS-SEM when considering the magnitude of its impact. This may be because ANN can capture linear and non-linear connections between concepts (Albahri et al., Citation2022). Considering the non-linear connection between SI and BI, SI is found to be more crucial than FC, EE, and PE. The value of the two-stage analysis approach (PLS-ANN) in this research is demonstrated by the fact that this truth would be obscured by using only the first step of analysis (i.e., PLS-SEM).
Table 11. RMSE values for independent variables
Table 12. Sensitivity analysis with normalized importance for independent variables
6. Discussion
By using UTAUT, this paper aims to empirically analyze the determining factors of blockchain adoption in logistics enterprises in HCMC. Fortunately, existing literature on technology adoption includes many excellent studies on blockchain adoption by utilizing the UTAUT model and its current variation from America to Asia. Accordingly, this study presents a construct that may help predict factors that affect the behavioral intention of using blockchain in these logistics companies. Some hypotheses were established by the relationships mentioned in the original model, namely the influences of PE, EE, FC, and SI on BI. In general, these factors were found to have different impacts on BI. Indeed, the influence of PE and FC was significant on BI, whereas EE and SI were found to have an insignificant impact on the BI of blockchain adoption. Moreover, this study also explores that there is no establishment of the moderating effect of EXP on the relationship between variables. By understanding the influence of these factors on BI, decision-makers can create the best strategy for their employees to learn because they know which aspects need to enhance and vice versa.
6.1. The effect of performance expectancy on behavioral intention of using blockchain
The results showed that performance expectancy (PE) substantially positively influences the behavioral intention (BI) to use blockchain, although it has different ranks in the two techniques. Therefore, this is consistent with the prior literature on adoption considerations (Hew et al., Citation2017; L. T. Nguyen et al., Citation2022) and the positive impact (Qu, Citation2021; T. Nguyen, Citation2022). Some researchers suggested that blockchain would become the game changer in the future because of its efficiency and support changes in the operation of the logistics sector with cryptocurrency (Pilkington, Citation2016). Adopting blockchain lets enterprises access and share information in real-time by every network partner, eliminating the complicated procedure of third parties and the processing time. In addition, the ability to manage operations automatically will lead to more efficient material transportation. As a result, every stage in the process will be quicker and earlier. By understanding and developing these advantages, logistics companies, which adopt blockchain soon, will gain more chances than others in the same sector. Indeed, it is clear that the implementation of blockchain depends on the clear proposition of benefits that the suppliers and partners could receive. Such findings align with previous papers using this variable (Gligor et al., Citation2022; Jung et al., Citation2019). In order to assert it again, Zou et al. (2019) stated that this performance expectancy is the main reason for selecting blockchain. The higher the effectiveness of blockchain, the greater the behavioral intention to use this technology.
6.2. The effect of effort expectancy on behavioral intention of using blockchain
The study shows that effort expectancy (EE) considerably positively impacts the behavioral intention (BI) to use blockchain. These findings are consistent with previous research on mobile banking adoption (Alalwan et al., Citation2017). Even though mobile banking is different from blockchain, these two have some similarities. Firstly, they are both new technologies and secondly, mobile banking is also a new system in the finance sector, similar to blockchain, a new information system in logistics. Therefore, the EE considerably impacts the behavioral intention of both these technologies. In other words, people will decide to adopt earlier when they do not need too much effort to learn how to use it. Indeed, according to L. W. Wong et al. (Citation2019) (Queiroz & Fosso Wamba, Citation2019), technology adoption was also shown to be significantly inhibited by the complexity of the new information system, which leads to the user will be reduced because of the fear of using complicated blockchain technology. Earlier findings of (Swan, Citation2015) and (Tsai et al., Citation2013) have also validated this. Therefore, the easier the blockchain is, the higher the percentage of the behavioral intention to use this technology would be.
6.3. The effect of facilitating conditions on behavioral intention of using blockchain
The findings indicated that facilitating conditions significantly positively affect the behavioral intention to use blockchain. Similarly, this result also agrees with the results observed in the past literature about blockchain (L. W. Wong et al., Citation2020). The requirement of understanding and planning before adopting blockchain is essential to implement widely in a firm because of its specification (Saberi et al., Citation2019). Therefore, an organization needs to clear some internal barriers by providing the knowledge, expertise, and tools essential to transfer to a new system like blockchain. Regarding Viet Nam, where the developing economy is not comprehensive since some companies’ working conditions have not widely installed modern technology systems, white-collar workers may reject accepting blockchain. Sometimes, the unequal development between companies also creates difficulties in implementing blockchain. As a result, the thorough preparation would motivate employees to be ready to use blockchain in their work and, at the same time, increase the proportion of success in adopting blockchain in the whole company. In the context of this paper, facilitating conditions play an essential role in pushing blockchain adoption, even if blockchain is complicated to use.
6.4. The effect of social influence on behavioral intention of using blockchain
There was a surprise when social influence is an excellent predictor of behavioral intention in the result of the ANN technique. However, it has a minor positive influence on the PLS-SEM approach. In other words, ANN’s result is consistent with Venkatesh et al. (Citation2012), while the results of this study by PLS-SEM have a slight impact on blockchain adoption. Furthermore, the findings contradict a study on mobile banking adoption that social influence does not affect behavioral intention (Alalwan et al., Citation2017). Nevertheless, they are consistent with those of recent research on the adoption of blockchain in the Indian supply chain (Queiroz & Fosso Wamba, Citation2019) and on mobile payment (Chong, Citation2013; Hawlitschek et al., Citation2018; L. W. Wong et al., Citation2021). In the context of Viet Nam, it is clear that most Vietnamese families are extended families, so their behavioral intention is impacted deeply by many members in their homes. Additionally, asking for other opinions when deciding is a typical behavior that may change the prior intention. Therefore, the results of this research suggest that family members, colleagues, and friends have a specific impact on the decision to use blockchain in the user’s work.
6.5. The effect of experience on the relationships among influencing factors and the behavioral intention of using blockchain
Lastly, the study’s results indicated that experience has no substantial impact on the association between factors and the intention to use the blockchain of people who work in logistics. The findings contradict the study of Venkatesh et al. (Citation2003), which asserts that experience influences the intention to use technology (Ozdogru, Citation2019). In line with this, these results are also opposite to the results obtained by (Taylor & Todd, Citation1995), where the new technology use intention was stronger with people with more experience. In the context of this study, the reason why people do not want to use technology is independent of their experience. According to some previous studies, although employees with more experience will find technology simple to use, the ability to use blockchain was the same with every employee because it is a new tool. Indeed, with a new system, if all employees, who have not had expertise about blockchain before, are trained to use it, they will have the same basement of knowledge. Therefore, the experience cannot significantly affect the intention to use this new technology. Additionally, due to the nearly identical basement of knowledge about blockchain, they have almost the same responses to other influencing factors of the intention of usage, namely the efficiency of their operating performance, the conditions of infrastructures in their organization, and the impact of people’s recommendations around them. On the other hand, in many Vietnamese enterprises and even multinational organizations, employees may have no choice but to accept any compulsory changes when their companies implement a new technology system. It cannot be denied that managers usually make decisions without asking their employees, specifically in strategies that enhance companies’ performance and profit. That is also why the experience has not substantially impacted the intention to use blockchain.
7. Conclusion and implications
Ying et al. (Citation2018) (Ying et al., Citation2018) argue that the current status of blockchain research, which is primarily exploratory, is in dire need of empirical data. Therefore, this study proposed a model through which the behavioral intention of individuals in using an emerging technology like blockchain in the logistics sector would be understood better and explained in detail. Considering the potential outcomes of blockchain technology, our study contributes significantly to the fields of information technology behavioral intention in logistics, and specifically blockchain. This is one of the earliest uses of the UTAUT model to analyze potential users’ attitudes regarding blockchain technology in the logistics industry. Despite the increasing focus on the potential uses of blockchain technology, the majority of the earlier studies have either advocated (Schuetz & Venkatesh, Citation2020) or done blockchain research from a TAM viewpoint (Kamble et al., Citation2019) or has mainly focused on isolated elements of blockchain, such as transparency (Flovik et al., Citation2021) or trust (Shin & Hwang, Citation2020). In this study, the critical adoption theory component is drawn from the UTAUT to delve more deeply into the perspectives of the businesses studied. In response to the research question, the findings of this study show that PE, EE, SI, and FC are significant and positive predictors of behavioral intention to use blockchain. Furthermore, among the significant predictors, both PLS-SEM and ANN show that EE and FC have the second and third ranks in the most influential factors in BI. Moreover, this study contributes to the expected blockchain influences on the development of the logistics field in the future. The paper’s research model, UTAUT, was inspired by a previously established, widely-used model. As a result, adapting the constitution’s theoretical framework into practice will be straightforward, and its trustworthiness will increase. On the other hand, the previous studies were mentioned during the research process to bring important insight to scholars and practitioners and help them comprehend how individuals behave when adopting blockchain. In this manner, this paper is intended to contribute to the increasing literature on blockchain adoption and offer variety to the research on adoption models for new technologies through the theoretical lens of the UTAUT model and the proof of experiment from logistics companies in HCMC. Empirical results from a statistically validated model imply that UTAUT may be sufficient for predicting behavioral intention to embrace new technologies, and this study is one of few to use a structural equation modeling artificial neural network (SEM-ANN) technique. Results from the model are promising and could inform future studies and practical applications of BC technology in the logistics sector.
Concerning the practical implications, this research would increase our understanding of blockchain adoption in logistics organizations by illuminating the elements that influence individuals’ behavioral intentions and allowing us to work to change those intentions from the outset of the transition. Consequently, businesses should invest in the necessary infrastructure to support the adoption of blockchain technology in logistics work and prioritize raising employee awareness and education about blockchain usage by studying the key constructs of behavioral intention. Indeed, it can be seen from the results of this paper that FC has a significant direct influence on the BI of using blockchain with the indicator of path coefficient equal to 0.271. A firm with the proper infrastructures, resources, and awareness support will enhance the desire to adopt blockchain of employees and any innovative technology. On the other hand, the findings here help extend the comprehension of blockchain application developers; through this, they will take relevant factors into account while developing such applications, namely, the usefulness and ease to use of the apps. As mentioned in Table , PE and EE substantially impact BI of using blockchain with path coefficients of 0.397 and 0.164, respectively. It is implied that an enterprise that wants to adopt blockchain must be able to deal with a wide range of challenges and establish and encourage many facets of implementing blockchain technology into existing ecosystems. When implementing a blockchain system, they must cope with obstacles and opportunities.
Blockchain technology could help logistics firms streamline their shipping and delivery processes. Productivity may be increased significantly, supply networks can be fortified, and delivery times and costs can be reduced thanks to this approach of monitoring products. Blockchain’s distributed ledger system eliminates inefficiencies in the financial services business by cutting out the middleman (Yusr et al., Citation2022). In addition to its benefits to the economy, blockchain technology also makes it possible to track and verify the authenticity of any product at every stage of the supply chain. This transparency ensures product quality, adherence to anticipated production requirements, compliance with environmental regulations, and fraud prevention (Mahyuni et al., Citation2020). Therefore, the purpose of this article is to dispel doubts about the pros and cons of implementing blockchain technology into business operations by providing relevant details. It also describes the numerous benefits to the logistics industry and associated economic sectors.
A substantial number of studies that used UTAUT and its expansion in the context of blockchain were carefully analyzed to determine the most frequently occurred factors prior to the proposal and discussion of four factors of the UTAUT model (namely PE, EE, FC, SI) and how they affect the BI of using blockchain systems in the logistics field. As a result, we anticipate that this study will yield some important findings for the field. However, some caveats in this paper necessitate further study. First, it is essential to note that this study’s conclusions may not generalize to circumstances outside of HCMC’s logistics industry. Therefore, future research could either provide a new model with more improved technology or reproduce this framework to further confirm in other sectors and nations. Second, as blockchain is still in its infancy, researchers have enough opportunities to learn new things about its widespread adoption. Future academics may argue for or against its adoption based on its features and characteristics, such as its interoperability, transaction speed, prices, legality, and others. The impact of experience as one of many potential modifiers on the studied connection was also investigated. As a result, other moderators and their relationship with one another may be the subject of further study. Finally, future research needs to consider organizational culture when comparing and contrasting organizations with different cultures. Not all businesses are equally open to exchanging information and resources, investing in technological infrastructure upgrades, or training employees using such upgrades. Therefore, it could cause disagreements in the decision-making process. Given these viewpoints, this field has a vast opportunity for additional study.
Disclosure statement
No potential conflict of interest was reported by the authors.
Additional information
Funding
References
- Ab Hamid, M. R., Sami, W., & Mohmad Sidek, M. H. (2017). Discriminant validity assessment: Use of fornell & larcker criterion versus HTMT Criterion. https://doi.org/10.1088/1742-6596/890/1/012163
- Ajzen, I. (1991). The theory of planned behavior. Organizational Behavior and Human Decision Processes, 50(2), 179–24. https://doi.org/10.1016/0749-5978(91)90020-T
- Alalwan, A. A., Dwivedi, Y. K., & Rana, N. P. (2017, Jun). Factors influencing adoption of mobile banking by Jordanian bank customers: Extending UTAUT2 with trust. International Journal of Information Management, 37(3), 99–110. https://doi.org/10.1016/J.IJINFOMGT.2017.01.002
- Albahri, A. S., Alnoor, A., Zaidan, A. A., Albahri, O. S., Hameed, H., Zaidan, B. B., Peh, S. S., Zain, A. B., Siraj, S. B., Masnan, A. H. B., & Yass, A. A. (2022). Hybrid artificial neural network and structural equation modelling techniques: A survey. Complex & Intelligent Systems, 8(2), 1781–1801. https://doi.org/10.1007/s40747-021-00503-w
- Bandura, A. (1986). Social foundations of thought and action: A social cognitive theory (pp. 617). Prentice Hall.
- Baron, R. M., & Kenny, D. A. (1986, Dec). The moderator–mediator variable distinction in social psychological research: Conceptual, strategic, and statistical considerations. Journal of Personality & Social Psychology, 51(6), 1173–1182. https://doi.org/10.1037/0022-3514.51.6.1173
- Casado-Vara, R., González-Briones, A., Prieto, J., & Corchado, J. M. (2019). Smart contract for monitoring and control of logistics activities: Pharmaceutical utilities case study. Advances in Intelligent Systems and Computing, 771, 509–517. https://doi.org/10.1007/978-3-319-94120-2_49
- Chang, S. E., Chen, Y. C., & Lu, M. F. (2019, Jul). Supply chain re-engineering using blockchain technology: A case of smart contract based tracking process. Technological Forecasting & Social Change, 144, 1–11. https://doi.org/10.1016/j.techfore.2019.03.015
- Chang, M., Walimuni, A. C. S. M., Cheol Kim, M., & Soon Lim, H. Acceptance of tourism blockchain based on UTAUT and connectivism theoryVol. 71(July) Elsevier Ltd, 2022. https://doi.org/10.1016/j.techsoc.2022.102027
- Charness, N., & Boot, W. R. (2015). Technology, Gaming, and Social Networking. Handbook of the Psychology of Aging: Eighth Edition. https://doi.org/10.1016/B978-0-12-411469-2.00020-0
- Chen, Y., & Bellavitis, C. (2020, Jun). Blockchain disruption and decentralized finance: The rise of decentralized business models. Journal of Business Venturing Insights, 13, e00151. https://doi.org/10.1016/j.jbvi.2019.e00151
- Chong, A. Y. L. (2013, Feb). Predicting m-commerce adoption determinants: A neural network approach. Expert Systems with Applications, 40(2), 523–530. https://doi.org/10.1016/J.ESWA.2012.07.068
- Cohen, J. (2013). Statistical power analysis for the behavioral sciences. https://doi.org/10.4324/9780203771587
- Dang, D. T. V., Nguyen, L., & Nguyen, A. H. D. (2022). Extending UTAUT2 in Mobile money adoption and actual use behavior: An empirical research in Vietnam during the Covid-19. Industrielle Beziehungen Zeitschrift für Arbeit, Organisation und Management, 10(4). https://doi.org/10.53384/inbe.101390943.2779.1862003510
- Davis, F. D., Bagozzi, R. P., & Warshaw, P. R. (1992). Extrinsic and intrinsic motivation to use computers in the workplace. Journal of Applied Social Psychology, 22(14), 1111–1132. https://doi.org/10.1111/j.1559-1816.1992.tb00945.x
- Enaizan, O., Eneizan, B., Almaaitah, M., Al-Radaideh, A. T., & Saleh, A. M. (2020, Jan). Effects of privacy and security on the acceptance and usage of EMR: The mediating role of trust on the basis of multiple perspectives. Informatics in Medicine Unlocked, 21, 100450. https://doi.org/10.1016/J.IMU.2020.100450
- Erjavec, J., & Manfreda, A. (2022, Mar). Online shopping adoption during COVID-19 and social isolation: Extending the UTAUT model with herd behavior. Journal of Retailing & Consumer Services, 65, 102867. https://doi.org/10.1016/J.JRETCONSER.2021.102867
- Flovik, S., Moudnib, R. A. R., & Vassilakopoulou, P. (2021, Jan). Determinants of blockchain technology introduction in organizations: An empirical study among experienced practitioners. Procedia computer science, 181, 664–670. https://doi.org/10.1016/J.PROCS.2021.01.216
- Fornell, C., & Larcker, D. F. (1981). Evaluating structural equation models with unobservable variables and measurement error. JMR, Journal of Marketing Research, 18(3), 382–388. https://doi.org/10.2307/3151312
- Francisco, K., & Swanson, D. (2018, Jan). The supply chain has no clothes: Technology adoption of blockchain for supply chain transparency. Logistics, 2(1), 2. https://doi.org/10.3390/LOGISTICS2010002
- Garg, P., Gupta, B., Chauhan, A. K., Sivarajah, U., Gupta, S., & Modgil, S. (2021, Feb). Measuring the perceived benefits of implementing blockchain technology in the banking sector. Technological Forecasting & Social Change, 163, 120407. https://doi.org/10.1016/J.TECHFORE.2020.120407
- Gligor, D., Russo, I., & Maloni, M. J. (2022). Understanding gender differences in logistics innovation: A complexity theory perspective. International Journal of Production Economics, 246, 108420. August 2021. https://doi.org/10.1016/j.ijpe.2022.108420.
- Hair, J., Hollingsworth, C. L., Randolph, A. B., & Chong, A. Y. L. (2017). An updated and expanded assessment of PLS-SEM in information systems research. Industrial Management & Data Systems, 117(3), 442–458. https://doi.org/10.1108/IMDS-04-2016-0130
- Hair, J. F., Hult, G. T. M., Ringle, C. M., & Sarstedt, M. (2016). A Primer on Partial Least Squares Structural Equation Modeling (PLS-SEM) (2nd ed.). Sage Publications.
- Hawlitschek, F., Notheisen, B., & Teubner, T. (2018, May). The limits of trust-free systems: A literature review on blockchain technology and trust in the sharing economy. Electronic Commerce Research and Applications, 29, 50–63. https://doi.org/10.1016/J.ELERAP.2018.03.005
- Henseler, J., Hubona, G., & Ray, P. A. (2016, Feb). Using PLS path modeling in new technology research: Updated guidelines. Industrial Management & Data Systems, 116(1), 2–20. https://doi.org/10.1108/IMDS-09-2015-0382
- Henseler, J., Ringle, C. M., & Sarstedt, M. (2014). A new criterion for assessing discriminant validity in variance-based structural equation modeling. J Acad Mark Sci, 43(1), 115–135. https://doi.org/10.1007/s11747-014-0403-8
- Hew, J. J., Tan, G. W. H., Lin, B., & Ooi, K. B. (2017). Generating travel-related contents through mobile social tourism: Does privacy paradox persist? Telematics and Informatics, 34(7), 914–935. https://doi.org/10.1016/j.tele.2017.04.001
- Hu, L., & Bentler, P. M. (1999). Cutoff criteria for fit indexes in covariance structure analysis: Conventional criteria versus new alternatives. Structural Equation Modeling: A Multidisciplinary Journal, 6(1), 1–55. https://doi.org/10.1080/10705519909540118
- Irani, Z., Dwivedi, Y. K., & Williams, M. D. (2017). Understanding consumer adoption of broadband: An extension of the technology acceptance model. The Journal of the Operational Research Society, 60(10), 1322–1334. https://doi.org/10.1057/JORS.2008.100
- Jadil, Y., Rana, N. P., & Dwivedi, Y. K. (2021, Aug). A meta-analysis of the UTAUT model in the mobile banking literature: The moderating role of sample size and culture. Journal of Business Research, 132, 354–372. https://doi.org/10.1016/j.jbusres.2021.04.052
- Jung, K. J., Park, J. B., Phan, N. Q., Bo, C., & Gim, G. Y. (2019). An international comparative study on the intension to using crypto-currency. Studies in Computational Intelligence, 788, 104–123. https://doi.org/10.1007/978-3-319-98370-7_9
- Kamble, S., Gunasekaran, A., & Arha, H. (2019, Apr). Understanding the Blockchain technology adoption in supply chains-Indian context. International Journal of Production Research, 57(7), 2009–2033. https://doi.org/10.1080/00207543.2018.1518610
- Kelley, K., Clark, B., Brown, V., & Sitzia, J. (2003). Good practice in the conduct and reporting of survey research. International Journal for Quality in Health Care, 15(3), 261–266. https://doi.org/10.1093/INTQHC/MZG031
- Kripanont, N. (2007). Examining a technology acceptance model of internet usage by academics within Thai business schools. Journal of Business Systems, Governance and Ethics, 1(2). https://doi.org/10.15209/jbsge.v1i2.72
- Leong, L. Y., Jaafar, N. I., & Ainin, S. (2018). Understanding Facebook commerce (f-commerce) actual purchase from an artificial neural network perspective. JECR-Journal of Electronic Commerce Research, 19(1), 75–103. http://www.jecr.org/sites/default/files/19_1Paper5.pdf
- Liao, H. L., & Lu, H. P. (2008, Dec). The role of experience and innovation characteristics in the adoption and continued use of e-learning websites. Computers & Education, 51(4), 1405–1416. https://doi.org/10.1016/J.COMPEDU.2007.11.006
- Lim, A. F., Lee, V. H., Foo, P. Y., Ooi, K. B., & Wei–Han Tan, G. (2021). Unfolding the impact of supply chain quality management practices on sustainability performance: An artificial neural network approach. Supply Chain Management-An International Journal, 27(5), 611–624. https://doi.org/10.1108/SCM-03-2021-0129
- Lu, Q., & Xu, X. (2017, Nov). Adaptable blockchain-based systems: A case study for product traceability. IEEE Software, 34(6), 21–27. https://doi.org/10.1109/MS.2017.4121227
- Mahyuni, L. P., Adrian, R., Darma, G. S., Krisnawijaya, N. N. K., Dewi, I. G. A. A. P., Permana, G. P. L., & Foroudi, P. (2020). Mapping the potentials of blockchain in improving supply chain performance. Cogent Business & Management, 7(1), 1788329. https://doi.org/10.1080/23311975.2020.1788329
- Montecchi, M., Plangger, K., & Etter, M. (2019, May). It’s real, trust me! Establishing supply chain provenance using blockchain. Business Horizons, 62(3), 283–293. https://doi.org/10.1016/J.BUSHOR.2019.01.008
- Nguyen, T. (2022). Gender equality and prevailing challenges in the workplace in vietnam. Vietnam Briefing. Retrieved March 11, 2023. https://www.vietnam-briefing.com/news/vietnams-gender-equality-and-prevailing-challenges-in-the-workplace-in-vietnam.html/
- Nguyen, L. T., Dwivedi, Y. K., Tan, G. W. H., Aw, E. C. X., Lo, P. S., & Ooi, K. B. (2022). Unlocking pathways to mobile payment satisfaction and commitment. Journal of Computer Information Systems, 00(00), 1–18.
- Nguyen, H. and Nguyen, L. (2021). Factors influence blockchain adoption in supply chain management among companies based in Ho Chi Minh City,” in Conference Towards ASEAN Chairmanship 2023 (TAC 23 2021), Indonesia, 198, 1–13. https://www.atlantis-press.com/proceedings/t-a-c-23-21/125965535
- Ozdogru, U. (2019). Impact of exponential technologies on global supply chain management. Elsevier Inc. https://doi.org/10.1016/B978-0-12-815956-9.00003-X
- Pal, A., Tiwari, C. K., & Haldar, N. (2021, Nov). Blockchain for business management: Applications, challenges and potentials. Journal of High Technology Management Research, 32(2), 100414. https://doi.org/10.1016/J.HITECH.2021.100414
- Pilkington, M. Blockchain technology: Principles and applications. Res. Handbooks Digit. Transform. Sep. 2016. https://doi.org/10.4337/9781784717766.00019
- Qu, J. (2021, Aug). Blockchain in medical informatics. Journal of Industrial Information Integration, 25, 100258. https://doi.org/10.1016/J.JII.2021.100258
- Queiroz, M. M., & Fosso Wamba, S. (2019). Blockchain adoption challenges in supply chain: An empirical investigation of the main drivers in India and the USA. International Journal of Information Management, 46, 70–82. https://doi.org/10.1016/j.ijinfomgt.2018.11.021
- Rahi, S., Ghani, M. A., & Hafaz Ngah, A. (2019). Integration of unified theory of acceptance and use of technology in internet banking adoption setting: Evidence from Pakistan. Technology in Society, 58, 101120. https://doi.org/10.1016/j.techsoc.2019.03.003
- Saberi, S., Kouhizadeh, M., Sarkis, J., & Shen, L. (2019, Apr). Blockchain technology and its relationships to sustainable supply chain management. International Journal of Production Research, 57(7), 2117–2135. https://doi.org/10.1080/00207543.2018.1533261
- Schuetz, S., & Venkatesh, V. (2020, Jun). Blockchain, adoption, and financial inclusion in India: Research opportunities. International Journal of Information Management, 52, 101936. https://doi.org/10.1016/J.IJINFOMGT.2019.04.009
- Shin, D., & Hwang, Y. (2020, May). The effects of security and traceability of blockchain on digital affordance. Online Information Review, 44(4), 913–932. https://doi.org/10.1108/OIR-01-2019-0013
- Swan, M. (2015). Blockchain: Blueprint for a New Economy (pp. 194). O’Reilly Media.
- Taherdoost, H. (2018). A review of technology acceptance and adoption models and theories. https://doi.org/10.1016/j.promfg.2018.03.137
- Tan, G. W. H., & Ooi, K. B. (2018). Gender and age: Do they really moderate mobile tourism shopping behavior? Telematics and Informatics, 35(6), 1617–1642. https://doi.org/10.1016/j.tele.2018.04.009
- Tan, G. W. H., Ooi, K. B., Leong, L. Y., & Lin, B. (2014). Predicting the drivers of behavioral intention to use mobile learning: A hybrid SEM-Neural Networks approach. Computers in Human Behavior, 36, 198–213. https://doi.org/10.1016/j.chb.2014.03.052
- Tapscott, D., & Tapscott, A. (2016). How blockchain will change organizations. MIT Sloan Management Review.
- Taylor, S., & Todd, P. (1995). Assessing IT usage: The role of prior experience. MIS Quarterly, 19(4), 561. https://doi.org/10.2307/249633
- Teck Soon, H., & Sharifah, S. L. (2017). The drivers for cloud-based virtual learning environment: Examining the moderating effect of school category. Internet Research, 27(4), 942–973. https://doi.org/10.1108/IntR-08-2016-0256
- Tenenhaus, M. (2008). Total quality management & business component-based structural equation modelling component-based structural equation modelling. Total Quality Management & Business Excellence, 19(7–8), 871–886. https://doi.org/10.1080/14783360802159543
- Thompson, R. L., Higgins, C. A., & Howell, J. M. (1991). Personal computing: Toward a conceptual model of utilization. MIS Quarterly, 15(1), 125–142. https://doi.org/10.2307/249443
- Toufaily, E., Zalan, T., & Ben Dhaou, S. (2021, Apr). A framework of blockchain technology adoption: An investigation of challenges and expected value. Information & Management, 58(3), 103444. https://doi.org/10.1016/j.im.2021.103444
- Tsai, M. C., Lai, K. H., & Hsu, W. C. (2013, Jan). A study of the institutional forces influencing the adoption intention of RFID by suppliers. Information & Management, 50(1), 59–65. https://doi.org/10.1016/J.IM.2012.05.006
- Venkatesh, V., Morris, M. G., Davis, G. B., & Davis, F. D. (2003). User acceptance of information technology: Toward a unified view. MIS Quarterly, 27(3), 425–478.
- Venkatesh, V., Thong, J. Y. L., & Xu, X. (2012). Consumer acceptance and use of information technology: Extending the unified theory of acceptance and use of technology. MIS Quarterly: Management Information Systems, 36(1), 157. https://doi.org/10.2307/41410412
- Wang, Y. (2021). Blockchain applications in logistics. International Encyclopedia of Transportation, 136–142. https://doi.org/10.1016/b978-0-08-102671-7.10233-7
- Wang, T., Hua, H., Wei, Z., & Cao, J. (2022, Feb). Challenges of blockchain in new generation energy systems and future outlooks. International Journal of Electrical Power & Energy Systems, 135, 107499. https://doi.org/10.1016/J.IJEPES.2021.107499
- Wang, X., Liu, X., Shi, L., Li, J., & Xie, J. (2015, Feb). Characteristic and formation mechanism of matt surface of double-rolled copper foil. Journal of Materials Processing Technology, 216, 463–471. https://doi.org/10.1016/j.jmatprotec.2014.10.015
- Wang, Y., Singgih, M., Wang, J., & Rit, M. (2019). Making sense of blockchain technology: How will it transform supply chains? International Journal of Production Economics, 211, 221–236. https://doi.org/10.1016/j.ijpe.2019.02.002
- Whitehouse, L., “Promoting gender balance in the logistics industry | CBI,”|“Promoting gender balance in the logistics industry | CBI,” The CBI, 2020. https://www.cbi.org.uk/articles/promoting-gender-balance-in-the-logistics-industry/ (Retrieved March 11, 2023).
- Wong, L. W., Leong, L. Y., Hew, J. J., Tan, G. W. H., & Ooi, K. B. (2019). “Time to seize the digital evolution: Adoption of blockchain in operations and supply chain management among Malaysian SMEs. International Journal of Information Management, 52, 101997.
- Wong, L. W., Tan, G. W. H., Lee, V. H., Ooi, K. B., & Sohal, A. (2020). Unearthing the determinants of Blockchain adoption in supply chain management. International Journal of Production Research, 58(7), 2100–2123. https://doi.org/10.1080/00207543.2020.1730463
- Wong, L. W., Tan, G. W. H., Lee, V. H., Ooi, K. B., and Sohal, A., “Psychological and system-related barriers to adopting blockchain for operations management: An artificial neural network approach,” IEEE Trans. Eng. Manag., 2021, https://doi.org/10.1109/TEM.2021.3053359.
- Wong, C. H., Tan, G. W. H., Tan, B. I., & Ooi, K. B. (2015, Nov). Mobile advertising: The changing landscape of the advertising industry. Telematics and Informatics, 32(4), 720–734. https://doi.org/10.1016/J.TELE.2015.03.003
- Yee-Loong Chong, A., Liu, M. J., Luo, J., & Keng-Boon, O. (2015, Jan). Predicting RFID adoption in healthcare supply chain from the perspectives of users. International Journal of Production Economics, 159, 66–75. https://doi.org/10.1016/J.IJPE.2014.09.034
- Ying, W., Jia, S., & Du, W. (2018, Apr). Digital enablement of blockchain: Evidence from HNA group. International Journal of Information Management, 39, 1–4. https://doi.org/10.1016/J.IJINFOMGT.2017.10.004
- Yu, C. S. (2012). Factors affecting individuals to adopt mobile banking: Empirical evidence from the utaut model. JECR-Journal of Electronic Commerce Research, 13(2), 104–121. http://www.jecr.org/sites/default/files/13_3_p01_0.pdf
- Yusr, M. M., Aliyu, O. A., Khattak, A., Salimon, M. G., & Muhammad, S. (2022). Determinants of SMEs’ product innovation performance in Malaysia: An extended model. Cogent Business & Management, 9(1). https://doi.org/10.1080/23311975.2022.2152649
Appendix
Appendix: Questionnaire
Factors impact the behavioral intention of adopting Blockchain in Logistics companies in HCMC
Part 1: Demographic characteristics
Part 2: Measurement scale