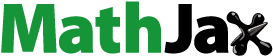
Abstract
Grape farming is a significant part of agricultural production in the central region of Dodoma and contributes tremendously to improving the livelihood of farmers. Smallholder farmers in Tanzania have been encouraged to use mobile phones for agricultural purposes. Regrettably, the involvement does not replicate how the use of mobile phones influences access to agricultural marketing information. It is, therefore, imperatively important to examine factors influencing the use of mobile phones for accessing agricultural marketing information by grape smallholder farmers in Dodoma. The study used a descriptive cross-sectional research design and a sample size of 400 grape smallholder farmers. A well-structured questionnaire and Focus Group Discussions (FGDs) were employed to solicit primary data. Descriptive, binary logistic regression, and thematic approaches aided data analysis. The most commonly accessed agricultural marketing information were buyers and price information. Socio-demographic factors such as sex, age, marital status, and education influenced the use of mobile phones for accessing agricultural marketing information by grape smallholder farmers. The study therefore, recommends that training programs be tailored to creating more knowledge on the use of mobile phones for accessing agricultural marketing information.
Public Interest Statement
Tanzania has ever been promoting the uptake and use of modern ICTs including mobile phones in the agricultural sector. Such efforts are expected to increase farmers access to agricultural information. Despite the immense efforts, access and use of mobile phones is still low among many smallholder farmers. The current study argues that effective use of mobile phone technologies is dependent upon context-specific socio-demographic factors which have not been previously studied among grape smallholder farmers. Addressing these factors may provide insightful information to policy makers initiatives on the supply of agricultural marketing information. The study empirically emphasizes the consideration of these factors when designing the system for disseminating agricultural marketing information to grape smallholder farmers.
1. Introduction
The use of mobile phones has become an integral part of smallholder farmers’ lives in both developed and developing countries. The rapid increase in the uptake and use of mobile phones among smallholder farmers has been attributed to a fall in cost, increased connectivity, and awareness (Balogun et al., Citation2022; Birner et al., Citation2021). This has changed how smallholder farmers access and use agricultural marketing information. The potential of mobile phones is estimated to empower smallholder farmers to make high-quality decisions. According to Yao et al. (Citation2022) and Khan et al. (Citation2022), the use of mobile phones has demonstrated efficiency in cost savings, improved bargaining power, reduced barriers to market participation, and helped farmers travel to better- paying markets. Abdul‐Rahaman and Abdulai (Citation2022) and Krell et al. (Citation2021) argue that mobile phones have transformative potential to reach many smallholder farmers in the shortest time and at the lowest cost. Quy et al. (Citation2022) and Kassem et al. (Citation2021) posit that mobile phones are continuously being utilised in the agricultural sector to improve access to agricultural marketing information. With the arrival of mobile phone technology, there is a compelling need for smallholder farmers to use mobile phones for accessing agricultural marketing information.
Agricultural marketing information is crucial for most smallholder farmers to make the right decisions. The importance of agricultural marketing information is related to the decision on what to produce, where to sell, when to sell, and the price to charge (Donkor et al., Citation2021). However, smallholder farmers are greatly challenged with access to agricultural marketing information, despite the existence of mobile phone technologies. Smallholder farmers are still using middlemen to access agricultural marketing information and end up exploited (Chelanga et al., Citation2021; Tamirat & Zeleke, Citation2021). In the grape farming context, Mlay (Citation2021) and Kulwijila et al. (Citation2018) highlight that grape smallholder farmers in Dodoma are still using friends, fellow farmers, neighbours, and relatives as their predominant sources of agricultural marketing information and end-up selling at farm get prices. Regrettably, Ndimbo et al. (Citation2023) and Mushi et al. (Citation2022) reported that smallholder farmers in Tanzania are still evolving to newer mobile phone technologies for agricultural marketing information access. This specifies that smallholder farmers’ need for reliable and timely agricultural marketing information can be met with the use of mobile phones.
Despite, the significance of mobile phones in accessing agricultural marketing information, there is still low mobile phone usage in accessing agricultural marketing information. The adoption and use of mobile phones is complex and influenced by interrelated factors, such as performance expectancy, effort expectancy, social influence, and facilitating conditions (Ivanova & Kim, Citation2022; Peng, Citation2022; Rahmiati et al., Citation2022). While these factors are moderated by the socio-demographic characteristics of respondents, little attention has been paid to such factors Rode et al. (Citation2021) and Tamar et al. (Citation2021) assert that moderators can potentially increase the predictive power of models. Moreover, in Tanzania, no study has been done on socio-demographic factors influencing the use of mobile phones for accessing agricultural marketing information by grape smallholder farmers. Studies on grape farming focused mainly on marketing challenges, post-harvest losses, input demand responses, and factors affecting marketing performance (Kalimangasi et al., Citation2021; Kulwijila, Citation2021; Mlay, Citation2021). This leaves a gap in what would explain the influence of socio-demographic factors on mobile phone use for accessing agricultural marketing information. This forms the bottom line for the study, which examines the influence of socio-demographic factors influencing the use of mobile phones for accessing agricultural marketing information. Specifically, the study intended to ascertain mobile phone ownership status, describe mobile phone use in accessing agricultural marketing information, and analyse the influence of socio-demographic factors on mobile phone use for accessing agricultural marketing information. The study was further guided by the following research question: -
RQ: What socio-demographic factors influence the use of mobile phones for accessing agricultural marketing information by grape smallholder farmers?
2. Literature Review and Hypotheses Development
Studies on socio-demographic factors and mobile phone use for accessing agricultural marketing information remain inconclusive, and understanding such differences remains significant. The reviewed literature demonstrates that smallholder farmers’ use of mobile phones is influenced by socio-demographic factors, and thus several hypotheses were developed.
2.1. Sex and use of mobile phones for accessing agricultural marketing information
Sex is among the common variables for predicting the adoption and use of mobile phones for accessing agricultural marketing information. Zheng and Ma (Citation2023) showed that women were less likely to use mobile phones for accessing agricultural marketing information. Similar findings were reported by Krell et al. (Citation2021) and Khan et al. (Citation2019), indicating that men were inclined towards mobile phones for accessing agricultural marketing information. Zheng and Ma (Citation2023) and Dagar et al. (Citation2021) showed that female farmers were less likely to adopt and use mobile phones for accessing agricultural marketing information. Yaseen et al. (Citation2021) and Kansiime et al. (Citation2019) showed that men and women differed significantly in the use of mobile phones for accessing agricultural marketing information. Therefore, the study hypotheses that: -
H1:
Sex is not related to the use of mobile phones for accessing agricultural marketing information.
2.2. Age and use of mobile phones for accessing agricultural marketing information
Age has often been negatively associated with the use of mobile phones for accessing agricultural marketing information. Dagar et al. (Citation2021) and Rahman et al. (Citation2020) attributed age differences to mobile phone use for accessing agricultural marketing information. Nwali et al. (Citation2022) and Haile et al. (Citation2019) cited age as a significant factor in mobile phone use for accessing agricultural marketing information. Studies by Islam et al. (Citation2021) and Moshe et al. (Citation2021) proved that younger farmers were more likely to use mobile phones for accessing agricultural marketing information than older farmers. Nwali et al. (Citation2022) and Benard et al. (Citation2020) emphasized that older farmers were constrained in their use of mobile phones for accessing agricultural marketing information. Kabirigi et al. (Citation2022) and Kilima and Chikuni (Citation2021) concluded that younger smallholder farmers were more likely to use mobile phones for accessing agricultural marketing information than older farmers. Thus, the study hypotheses that: -
H2:
Age is not significantly correlated with the use of mobile phones for accessing agricultural marketing information.
2.3. Marital status and the use of mobile phones for accessing agricultural marketing information
Married smallholder farmers often have higher chances of using mobile phones to access agricultural marketing information. It was reported by Spencer et al. (Citation2022) and Rissing et al. (Citation2021) that married farmers shared usage experiences as they sought to accomplish common goals hence similar intentions on mobile phone use for accessing agricultural marketing information. Sennuga et al. (Citation2020) and Otene et al. (Citation2018) observed that married farmers were willing to adopt and use mobile phones for accessing agricultural marketing information. Ahmed and Anang (Citation2019) and Issahaku et al. (Citation2018) also highlighted that married farmers had a better chance of using mobile phones to access agricultural marketing information. The study further hypothesized that:
H3:
There is no significant relationship between marital status and mobile phone use for accessing agricultural marketing information.
2.4. Education level and use of mobile phones for accessing agricultural marketing information
Education level is one of the most important socio-demographic factors influencing mobile phone use of smallholder farmers. Khan et al. (Citation2020) and Mwalupaso et al. (Citation2019) asserted that education level is positively correlated with the use of mobile phones for accessing agricultural marketing information. Ujakpa et al. (Citation2021) and Rahman et al. (Citation2020) affirmed that illiterate farmers were less likely to use mobile phones for accessing agricultural marketing information. Sousa et al. (Citation2019) claimed that educated farmers had an advantageous position in the use of mobile phones for accessing agricultural marketing information. Krell et al. (Citation2021) and Csibi et al. (Citation2021) proved that there is a significant relationship between education level and mobile phone use for accessing agricultural marketing. Thus, the following hypothesis is proposed: -
H4:
Education level does not influence the use of mobile phones for accessing agricultural marketing information.
2.5. Household size and use of mobile phones for accessing agricultural marketing information
Differences in household size contribute significantly to the use of mobile phones for accessing agricultural marketing information. Siaw et al. (Citation2020) and Quandt et al. (Citation2020) argue that higher household sizes leave farmers with less time to use mobile phones for accessing agricultural marketing information. Ling et al. (Citation2021) and Ali (Citation2021) observed that larger household size encouraged a high rate of mobile phone use among smallholder farmers. Related findings were reported by Ngenoh et al. (Citation2019) and Donkoh et al. (Citation2019), indicating that larger household sizes reduced the chance of mobile phone use in accessing agricultural marketing information. Thus, it is worth hypothesizing that: -
H5:
Household size does not influence the use of mobile phones for accessing agricultural marketing information.
2.6. Farming experience and use of mobile phones for accessing agricultural marketing information
Farming experience is also associated with mobile phone use for accessing agricultural marketing information. Abegunde et al. (Citation2019) and Michels et al. (Citation2019) elucidated that farming experience is positively associated with the adoption and use of new mobile phone technologies. Aldosari et al. (Citation2019) and Gebre et al. (Citation2019) highlighted that farmers with more years of farming experience are less likely to use mobile phones for accessing agricultural marketing information. On the same note, Michels et al. (Citation2020) revealed that farmers with more farming experience are reluctant to try new mobile phone technologies. Hence, the study hypotheses that: -
H6:
Farming experience does not affect the use of mobile phones for accessing agricultural marketing information.
2.7. Farm size and use of mobile phones for accessing agricultural marketing information
Studies have demonstrated that farm size is a common variable influencing the use of mobile phones by smallholder farmers. Ado et al. (Citation2019) and Drewry et al. (Citation2019) reported that small and large farm sizes owners differ significantly in the speed of adoption and use of mobile phones. Studies (Dagar et al., Citation2021; Ruzzante et al., Citation2021) showed that land ownership is significantly related to technological use, where farmers with more land are driven by status to use mobile phone technology to seek agricultural marketing information. Agussabti et al. (Citation2022) and Donkoh et al. (Citation2019) asserted that farmers with larger farm sizes had a higher propensity to seek agricultural marketing information through mobile phones compared to farmers with smaller farm sizes. Ma et al. (Citation2020) and Alam et al. (Citation2019) found that farmers with large farms had a high rate of mobile phone adoption and use for accessing agricultural marketing information. Thus, the following hypotheses is proposed: -
H7:
Farm size does not affect the use of mobile phones for accessing agricultural marketing information.
2.8. Income and use of mobile phones for accessing agricultural marketing information
Income appears to be an important factor influencing mobile phone use among smallholder farmers in most of the developing countries. Krell et al. (Citation2021) and Hoang (Citation2020) found that smallholder farmers with higher income had a greater likelihood of using mobile phones for accessing agricultural marketing information. Similarly, Donkor et al. (Citation2021) and Haile et al. (Citation2019) revealed that smallholder farmers with less income had a limited chance of using mobile phones for accessing agricultural marketing information. Quandt et al. (Citation2020) and Mwalupaso et al. (Citation2019) affirmed that there is no significant relationship between farmers’ level of income and mobile phone use. Furthermore, Thar et al. (Citation2021) and Alam et al. (Citation2019) observed that income is not an important factor for mobile phone adoption and use among smallholder farmers.
H8:
Income does not influence the use of mobile phones for accessing agricultural marketing information.
3. Theoretical framework
The study was guided by the Unified Theory of Acceptance and Use of Technology (UTAUT). The model is built on the assumption that performance expectancy, effort expectancy, and social influence indirectly influence technological use, whereas facilitating conditions and intentions directly influence technological usage behaviour (Alaba et al., Citation2022; Tang et al., Citation2021). Moderators like age, experience, sex, marital status, and household size are considered to exert a direct and indirect moderating influence on technological usage behaviour. The model further hypotheses that the increased intention for technology use is dependent upon the perceived performance of the existing technology (Khan & Mahmood, Citation2022; Yuliana & Aprianingsih, Citation2022). This suggests that interest in the use of new technology is associated with its ability to improve users’ activities. However, performance expectancy and intention are moderated by socio-demographic factors like sex, age, and marital status, such that performance expectancy can directly influence technological usage intentions (Dirsehan & Van Zoonen, Citation2022; Kitsiou et al., Citation2022). Again, the model highlights that the perceived easy of use increases the possibility of the intention to use the technology (Ninaus et al., Citation2021; Puriwat & Tripopsakul, Citation2021). This implies that the lesser the complexity of the technology the greater the intention of use. Similarly, socio-demographic factors are expected to significantly moderate effort expectancy for technological usage behaviour (Adjei et al., Citation2021; Anthony et al., Citation2021). If the new technology draws the intention of peers, it motivates users to increase their technological usage intentions. The model recognizes gender, age, volunteerism and experience as key moderator constructs for technology usage (Park et al., Citation2021; Yang et al., Citation2021). Li et al. (Citation2021) and Singh and Kaur (Citation2021) agreed that facilitating conditions play a significant role in moderating the influence of socio-demographic factors on technological usage intentions. The use of this model is justified based on its ability to provide a holistic understanding of the moderating power to drive the direct and indirect influence on the use of mobile phone technology.
4. Methodology
4.1. Description of the Study Area
The study was carried out in Dodoma region, Tanzania. Dodoma is one of the administrative regions of Tanzania’s mainland, located in the central part of the country. The region is heavily dependent on agriculture as a predominant sector, and grape farming is a symbol crop and a significant driver for socio-economic development for the majority of the smallholder farmers. Being the case, the region qualified as a study area because it is the only region producing grapes in the country, and grape smallholder farmers are challenged with access to agricultural marketing information, so improving it through the use of mobile phones would become an important initiative.
4.2. Research design
The study made use of a descriptive cross-sectional research design to examine the relationship between the explanatory variables and a response variable at a single point in time. To gain a richer understanding of the factors influencing the use of mobile phones for accessing agricultural marketing information, a mixed method was employed. The quantitative method intended to test hypotheses on socio-demographic factors influencing mobile phone use, while the qualitative method complemented the quantitative method (Rosalia, Citation2022). The use of a mixed method is justifiable in this study based on the fact that it allowed the cancellation of the method effect of relying on a single approach (Alharahsheh & Pius, Citation2020).
4.3. Sampling strategies
The target population comprised 2914 grape smallholder farmers, from whom a sample size of 400 grape smallholder farmers was derived. The sample size was calculated by a Raosoft online-based sample size calculator and considered to have a 0.9686 confidence level, a 0.05 margin of error, and a 0.5 skewness level. Moraes et al. (Citation2022) and Serdar et al. (Citation2021) assert that the sample size determination must carefully consider the confidence interval, margin of error and skewness level. The sample size calculator was ideally chosen because of its flexibility in yielding a representative sample for both finite and infinite populations (Alenazi et al., Citation2022; Qazi et al., Citation2022). The sample size was considered adequate for the study as it falls within the range of 200 to 500 units for carrying out rigorous statistical analysis (Niessl et al., Citation2020). To select a valid sample, a purposive sampling technique was applied in selecting Dodoma region and the three villages of Hombolo Bwawani, Mpunguzi, and Mbabala. The villages were chosen because they comprise 1063 of all the grape smallholder farmers within the region. The selection of the study respondents was done through the use of a systematic sampling technique in which the first respondent was randomly chosen followed by three interval estimates from the household list provided by the village Executive office (VEOs). The use of a systematic sampling technique was justifiable in this study because of its ability to enhance validity with reasonable cost (Rahman et al., Citation2022).
4.4. Data collection methods
Quantitative data were collected through a researcher’s administered structured questionnaire (Appendix I) and piloted with 30 respondents in Mvumi Mission Village in Chamwino District. The piloting was done in this village because it shares similar respondents’ characteristics with where the actual data collection was made. The piloting was meant to check the degree of accuracy of the data collection tools. Yusoff et al. (Citation2021) recommended that a sample size pretesting can ideally be done to 30 units. The use of a questionnaire was preferred because it is perfectly suitable when results are to be presented quantitatively (Alharahsheh & Pius, Citation2020). Two Focus Group Discussions (FGDs) (Appendix II), each with seven participants were conducted to collect primary data. The participants were purposefully selected based on marketing experience, knowledge, and gender consideration in order to reveal deep and rich opinions from the natural settings. Claessens et al. (Citation2022) argue that the purposive sampling technique is ideally suitable for the selection of six to twelve participants.
4.5. Data analysis
4.5.1. Analytical model
Quantitative data were coded on the IBM Statistical Package for Social Sciences (SPSS) version 27 and analysed to produce descriptive statistics (frequency, percentage, and mean). The level of agricultural marketing information access was measured as (5= very high access, 2=high access, 3=moderate access, 2=low access, and 1=no access). The response values were added to get a mean cut-off point of (1 + 2 + 3 + 4 + 5) = 15, then divided by 5 to obtain a mean value of 3. and the agricultural marketing information access level was recategorised as high access (mean value of 3 and above), and low access (mean value below 3). Aregaw et al. (Citation2023) used this method of calculating the cut-off points to ascertain factors affecting the competence level of agricultural extension agents.
The binary logistic regression model was used to analyse the influence of socio-demographic factors on the use of mobile phones for accessing agricultural marketing information. The model was chosen because the dependent variable is dichotomous, that is, a value of one was assigned for a farmer who used mobile phones for accessing agricultural marketing information and zero for otherwise. According to Srimaneekarn et al. (Citation2022) and Fatah and Alkaki (Citation2021), binary logistic regression model can appropriately be applied to describe and test hypotheses on the relationship between categorical dependent variables and a set of independent categorical or continuous variables.
The binary logit regression was specified as: -
Where, Logit (pi) =Y; is odds on the probability of using mobile phones for accessing agricultural marketing information (ie. mobile phone use = 1, otherwise = 0), β1 –βp= Regression coefficients α = Intercept X1, 1 –Xp, 1= predictor variables ε = Error term.
The binary logistic regression involved fitting an equation of the following form to the data:
Qualitative data were subjected to thematic analysis by first transcribing, arranging responses in codes reflective of the questions, noting common themes, theme reviewing, redefining and naming of themes, and reporting respondents’ views on mobile phone use.
4.5.2. Variable measurements
Various predictor variables were selected to estimate the predicted values of the outcome variable. The predictor variables were both continuous and discrete. The choice of the variables was based on the studies (Krell et al., Citation2021, Spencer et al., Citation2022, Dagar et al., Citation2021, Zheng and Ma (Citation2023, Citation2021, Citation2021) that reviewed factors influencing the use of mobile phones. The measurement levels of the variables included in the binary logistic regression model are presented in Table as follows:
Table 1. Variable measurements
5. Results and Discussions
5.1. Socio-demographic characteristics of respondents
The study ascertained socio-demographic characteristics in order to validate the composition of respondents. Respondents had different socio-demographic characteristics, dominated by mature, married male respondents with a primary level of education owning, less than one acre, of land and considerable years of farming experience.
Table shows that of 400 respondents 304(75.9 percent) were males while 96(24.1 percent) were females. This suggests the commercial nature of grape farming because most male respondents are sensitive to well-paying activities. Of these, nearly more than half, 212(52.9 percent) were aged between 29 and 49 years. This implies that respondents were mature and experienced enough to provide the labour force required for grape farming. Additionally, more than three-quarters, 308 (77.1%) of the respondents were married. This suggests that respondents’ participation in grape farming provided income to take care of the family. Moreover, of all 400 respondents, 260(65 percent) had attained a primary level of education indicating that the majority of the respondents did not go for higher-level education. Concerning household size 182 (45.5 percent) of the respondents admitted that they had an average of three to five members in the family. On the other hand, of 400 respondents, 312(78 percent) mentioned that they owned less than two acres of land showing that respondents are still farming on small and fragmented pieces of land. It was further reported that 160(40.1 percent) of respondents had farming experience between one and five years. This indicates that respondents had enough experience in grape farming. Finally, more than half, 235(58,8 percent) of the respondents earned an annual income ranging between Tsh5,000,000–10,000,0000 suggesting that respondents had an average income to meet their daily requirements.
Table 2. Socio-demographic characteristics of respondents(n = 400)
5.2. Mobile phone ownership status
The study intended to describe mobile phone ownership, usage experience, and associated costs to provide a basis for accessing agricultural marketing information. The findings indicate that most respondents owned cheap simple mobile phones with more than one chip and a vast usage experience.
Table indicates that more than three-quarters 312(78.1 percent) of all the respondents did not own smartphones. This suggests that respondents are more knowledgeable about the use of less complex mobile phone technologies reflecting their education level. Furthermore, half of the respondents 203(50.7 percent) reported that they owned more than one mobile phone chip. This implies that respondents intended to take advantage of the short-term incentives provided by telecommunications companies. Again, of all the respondents 118(29.5 percent) mentioned that they had used mobile phone for four to six years. This validates the reality that mobile phones have been a relevant tool of communication among farming communities. Moreover, more than half of the respondents 270(67.5 percent) afforded mobile phones sold for less than Tsh 50,000 implicating poor quality of the mobile phones owned by grape smallholder farmers. The findings were similar to Ndemdou and Fongang (Citation2021) who established that most smallholder farmers in Cameroon and Ghana were largely simple mobile phones with more than one chip and vast usage experience.
Table 3. Mobile phone ownership status (n = 400)
5.3. Mobile phones use in accessing agricultural marketing information
Mobile phone ownership can mean full access, but as such, this does not necessarily mean usability. Mobile phone usage partly determines the nature of agricultural marketing information searched for and accessed by respondents. The study examined the level of agricultural marketing information accessed through mobile phones. This was necessary to gain insights on mobile phone use in relation to grape farming. The findings in Table indicate that buyers and price information were the most commonly accessed agricultural marketing information.
Table 4. Agricultural marketing information accessed through mobile phones (n = 400)
Table shows that most of the respondents accessed buyers’ information with a mean score of 3.6 and ranked it first followed by price which scored a mean value of 3.3, and quality, which came in third position with a mean score of 2.2. Quantity and selling time scored mean values of 1.9 and 1.5 respectively. This has been possible because mobile phones are equipped with user-friendly applications like voice calls, and mobile money services. The findings imply that the use of mobile phones has helped in the search of current agricultural marketing information, which can amplify negotiations for better prices with buyers. Timely access to buyers and price information is crucial for decision-making regarding where and to whom grapes should be sold. It was also highlighted in the FGDs, where participants claimed that the sharing of agricultural marketing information has been aided by the use of mobile phones and that it is now easy to cross-check prices with other farmers from neighbouring villages. They further confirmed that if traders fall short of money, they trust them to take grapes and send the money through the available mobile money services. This is a clear indicator that the use of mobile phones has revolutionised communication among grape smallholder farmers. Bruns et al. (Citation2022) and Kaddu et al. (Citation2020) revealed that price, buyers, and quality information were the most frequently accessed agricultural marketing information. The findings partly suggest that access to agricultural marketing information cements the mutual relationship with buyers and helps grape farmers make informed decisions.
5.4. Socio-demographic factors influencing the use of mobile phone for accessing agricultural marketing information
Table indicates the selected socio-demographic factors influencing the use of mobile phones for accessing agricultural marketing information. The binary logistic regression model was used to estimate the influence of such factors. In running the model, the Omnibus test of the model coefficient produced statistically significant results at p = 0.00 and a chi-square value of 176.18. This implies that there is an adequate fit of the data to the model and that the covariates are significantly related to the outcome variable. Moreover, the Hosmer-Lemeshow test results yielded a chi-square value of 6.787 and a p-value of 0.668 indicating that the model’s estimate fit the data at an acceptable level. The independent variables captured 59% of the variance in the dependent variable as shown in the Cox and Snell R2 of 0.531 and Nagelkerke R2 of 0.598. The study findings highlight that sex, age, marital status, and education were the most significant factors influencing the use of mobile phones for accessing agricultural marketing information.
Table 5. Socio-demographic factors influencing the use of mobile phone(n = 400)
The findings in Table show that sex had a negative statistically significant relationship with the use of mobile phone for accessing agricultural marketing information at p < 0.05, Wald = 2,770, and Exp (β) = 2.022. The findings demonstrate that being a female farmer reduces the chance of using mobile phone for accessing agricultural marketing information by 2.022, with an associated odd ratio of 0.704, implying that female farmers were less likely to use mobile phones for accessing agricultural marketing information. The finding supports the non-acceptance of the hypothesis that sex is not related to the use of mobile phones for accessing agricultural marketing information. During FGDs, women participants had opinions that their mobile phone usage is sometimes limited due to domestic activities and the nature of the husbands they live with. The findings might be attributed to the fact that men are the decision makers for resource ownership and use, including mobile phones, in most of the socio-cultural environment in Tanzania. The finding supports the UTAUT model that sex plays a specific moderating role for mobile phone use in accessing agricultural marketing information. The findings concur with Quaye et al. (Citation2022) and Ayisi et al. (Citation2022), who reported that male farmers had higher possibilities of using mobile phones for accessing agricultural marketing information compared to their female counterparts. Sex differences should be considered when designing and developing agricultural information systems to give female farmers an equal chance with mobile phone technologies.
Age had a significant but negative effect on the use of mobile phones for accessing agricultural marketing information at p < 0.05, Wald = 4.658, and Exp (β) = 0.549. The findings imply that when a farmer’s age increases by 0.549 years the odds ratio is −0.600 suggesting that older farmers were more reluctant to adopt and use mobile phones for accessing agricultural marketing information. The findings confirm the rejection of the hypothesis that age is not significantly correlated with the use of mobile phones for accessing agricultural marketing information. It was further reported by older participants in the FGDs that they rarely use mobile phones because they are not conversant with multiple applications as compared to the younger participants. The findings could be due to the fact that as age increases, it reduces the knowledge and skills of the smallholder farmers for using modern technologies. The findings confirm the UTAUT model that mobile phone usage is moderated by age to access agricultural marketing information. Hoang (Citation2020) and Khan et al. (Citation2020) revealed that younger farmers had a better chance of using mobile phones for accessing agricultural marketing information. Therefore, mobile phone usage initiates should take into account the age differences of farmers such that older farmers, should be educated on the use of ICTs, including mobile phones to equip them with knowledge and skills.
The findings show a positive influence of marital status on the use of mobile phones for accessing agricultural marketing information at p < 0.05, Wald = 3.127, and Exp (β) = 1.581. The findings mean that married farmers were more likely to use mobile phones by 1.581 with an associated odd ratio of 0.458. The hypothesis that there is no significant relationship between marital status and mobile phone use for accessing agricultural marketing information was not accepted. Married farmers were under pressure to produce more to earn income to meet family obligations. The desire for high production could result in seeking agricultural marketing information through modern communications means. The findings add to the UTAUT model by showing that new constructs such as marital status can contribute to moderating the main constructs of the model. The findings are consistent with those of Alant and Bakare (Citation2021) and Abbas et al. (Citation2020), who showed that marital status played a significant role in determining the use of mobile phones for accessing agricultural marketing information. Thus, the delivery of agricultural marketing information should take into account the marital status of smallholder farmers in various farming contexts.
There was a significant effect between education and mobile phone use for accessing agricultural marketing information at p < 0.05, Wald = 1.753, and Exp (β) = 1.099. The findings show that when education increases by 1.099 with an odd ratio of 0.094, it increases farmers’ ability to seek and use agricultural marketing information. Educated farmers were more likely to make better and more informed decisions about accessing agricultural marketing information. This resulted in the non-acceptance of the hypothesis that education level does not influence the use of mobile phones for accessing agricultural marketing information. It was also highlighted in the FGDs, where participants claimed that educated younger farmers were in a better position to derive the benefits accrued from mobile phones due to their education compared to illiterates farmers. This is because if individual farmers got the knowledge they would extend their scope of experience through modern sources of information. The findings enhance the UTAUT model by postulating that new constructs such as education can also contribute to moderating the relationship among the main variables. This confirms the studies by Asravor et al. (2022) and Hoang and Drysdale (Citation2021) who reported that farmers with higher education had a higher chance of using mobile phones for accessing agricultural marketing information. This implies that information service providers who may need to design a mobile phone information delivery model should take into consideration the education level of farmers.
6. Conclusion and recommendations
Mobile phones significantly contributed to accessing agricultural marketing information by grape smallholder farmers. Access to information helped grape smallholder farmers make more informed decisions and increased interaction with other actors within the grape marketing system. The study further unveiled socio-demographic factors such as sex, age, marital status, and educational attainment significantly influenced the use of mobile phones for accessing agricultural marketing information. Male grape smallholder farmers were more likely to use mobile phones for accessing agricultural marketing information. Older grape smallholder farmers had a limited chance of using mobile phones in accessing agricultural marketing information. Besides, married grape smallholder farmers had a greater chance of using mobile phones to access agricultural marketing information. Moreover, farmers with higher education were more likely to use mobile phones for accessing agricultural marketing information. Finally, the study concludes that socio-demographic factors significantly influence the use of mobile phones for accessing agricultural marketing information. Based on the findings, the study recommends that agricultural information dissemination centers be established to provide formal information through mobile phones. Appropriate training should be provided, especially to adult farmers, to increase mobile phone usage awareness.
7. Implications, limitations, and future studies
7.1. Theoretical implications
The study contributes to an enhanced understanding of socio-demographic factors driving the use of mobile phones for accessing agricultural marketing information. The study uniquely provides useful insight on the use of UTAUT to explain the moderating role of socio-demographic factors on the use of mobile phones for accessing agricultural marketing information. The study further extends the discourse that previous studies paid little attention to socio-demographic factors and focused heavily on performance expectancy, effort expectancy, and social influence. However, the moderating contribution of socio-demographic factors to the use of mobile phones for accessing agricultural marketing information was ignored.Thus, the study made a contribution by filling out this gap by incorporating the significant contribution of moderating factors on mobile phone use for accessing agricultural marketing information.
7.2. Practical implications
From a practical perspective, the study findings will help ICT system designers and developers understand the differences in mobile phone use across socio-demographic factors. This will consequently provide valuable information for designing suitable mobile phone applications that will constructively consider socio-demographic factors influencing mobile phone use for accessing agricultural marketing information. This indicates that an information delivery system for smallholder farmers using mobile phones should establish socio-demographic factors to enhance full utilization of the information resources.
7.3. Policy implications
The current study provides policy makers with information needed to encourage developers and designers to design mobile phone-related applications that will improve digitalization among smallholder farmers and improve access to agricultural marketing information. In addition to this, the policies need to be redesigned for smallholder farmers to receive regular mobile phone-related training to improve their knowledge of how better to use mobile phone technologies for access agricultural marketing information.
7.4. Limitation and future studies
In the first instance, the study was limited by its overdependence on the primary data sources. Overreliance on these data sources suggests that the study’s construction depended on farmers’ perceptions. The study also had a shortcoming in the choice of respondents and focused on those who used mobile phones and came from villages with large production scales of grapes. This could limit the generalisation potential for similar studies in other socio-cultural contexts. Secondly, the study was cross-sectional in nature, and therefore, the variables could not be analysed for a long time. The study proposes comparative studies with other countries or regions of similar nature to validate the most influential factors influencing the use of mobile phones for accessing agricultural marketing information.
Acknowledgments
We sincerely thank all grape smallholder farmers who voluntarily and actively participated in this study in the central region of Dodoma.
Disclosure statement
No potential conflict of interest was reported by the authors.
Additional information
Notes on contributors
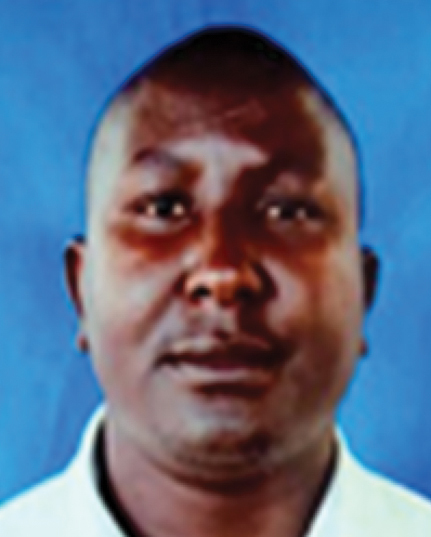
Alex I. Nyagango
Alex I. Nyagango worked as an Assistant Lecturer and a Researcher in the Discipline of Marketing under the Department of Marketing and Enterprise Management at Moshi Co-operative University(MoCU). He currently assumes a similar position under the Department of Management at Tanzania Institute of Accountancy (TIA). He holds a Master Degree in Business Management (MBM) from Moshi Co-operative University(MoCU). He has a Bachelor Degree in Business Administration (Marketing) from St. John’s University of Tanzania (SJUT). He has published several papers at reputable journals. He is recently mentoring undergraduate students on research projects. His areas of main research interests marketing communication, industrial marketing, consumer marketing, service marketing, social marketing, and marketing logistics.
References
- Abbas, K., Li, S., Xu, D., Baz, K., & Rakhmetova, A. (2020). Do socioeconomic factors determine household multidimensional energy poverty? Empirical evidence from South Asia. Energy Policy, 146, 111754. https://doi.org/10.1016/j.enpol.2020.111754
- Abdul‐Rahaman, A., & Abdulai, A. (2022). Mobile money adoption, input use, and farm output among smallholder rice farmers in Ghana. Agribusiness, 38(1), 236–21. https://doi.org/10.1002/agr.21721
- Abegunde, V. O., Sibanda, M., & Obi, A. (2019). Determinants of the adoption of climate-smart agricultural practices by small-scale farming households in King Cetshwayo District Municipality, South Africa. Sustainability, 12(1), 195. https://doi.org/10.3390/su12010195
- Adjei, T. K., Mohammed, A., Acheampong, P. R., Acquah-Gyan, E., Sylverken, A., Twumasi-Ankrah, S., Owusu-Dabo, E., & Owusu-Dabo, M. (2021). Determinants of a mobile phone-based interactive voice response (mIVR) system for monitoring childhood illnesses in a rural district of Ghana: Empirical evidence from the UTAUT model. Plos One, 16(3), e0248363. https://doi.org/10.1371/journal.pone.0248363
- Ado, A. M., Leshan, J., Savadogo, P., Bo, L., & Shah, A. A. (2019). Farmers’ awareness and perception of climate change impacts: Case study of Aguie district in Niger. Environment Development and Sustainability, 21(6), 2963–2977. https://doi.org/10.1007/s10668-018-0173-4
- Agussabti, A., Rahmaddiansyah, R., Hamid, A. H., Zakaria, Z., Munawar, A. A., & Abu Bakar, B. (2022). Farmers’ perspectives on the adoption of smart farming technology to support food farming in Aceh Province, Indonesia. Open Agriculture, 7(1), 857–870. https://doi.org/10.1515/opag-2022-0145
- Ahmed, H., & Anang, B. T. (2019). Does farmer group membership enhance technology adoption? empirical evidence from Tolon District of Ghana. Review of Agricultural and Applied Economics (RAAE), 22(1340–2019–3700), 26–32. https://doi.org/10.15414/raae.2019.22.02.26-32
- Alaba, O., Abass, O., & Igwe, E. (2022). Mobile learning via mobile devices in Nigeria higher education: Usage analysis based on UTAUT model. Journal of the Southern Association for Information Systems, 9(1), 64. https://doi.org/10.17705/3JSIS.00022
- Alam, G. M., Alam, K., Mushtaq, S., Khatun, M. N., & Mamun, M. A. K. (2019). Influence of socio-demographic factors on mobile phone adoption in rural Bangladesh: Policy implications. Information Development, 35(5), 739–748. https://doi.org/10.1177/0266666918792040
- Alant, B. P., & Bakare, O. O. (2021). A case study of the relationship between smallholder farmers’ ICT literacy levels and demographic data wrt their use and adoption of ICT for weather forecasting. Heliyon, 7(3). https://doi.org/10.1016/j.heliyon.2021.e06403
- Aldosari, F., Al Shunaifi, M. S., Ullah, M. A., Muddassir, M., & Noor, M. A. (2019). Farmers’ perceptions regarding the use of information and communication technology (ICT) in Khyber Pakhtunkhwa, Northern Pakistan. Journal of the Saudi Society of Agricultural Sciences, 18(2), 211–217. https://doi.org/10.1016/j.jssas.2017.05.004
- Alenazi, A. A., Alqahtani, A. S., Sultan, A. E., Alhazmi, H. O., & Alduhfeeri, T. M. (2022). Impact of covid related anosmia on patients quality of life at hail region. European Journal of Molecular & Clinical Medicine, 9(3), 721–729. https://ejmcm.com/article_17261_520.html
- Alharahsheh, H. H., & Pius, A. (2020). A review of key paradigms: Positivism VS interpretivism. Global Academic Journal of Humanities and Social Sciences, 2(3), 39–43.
- Ali, E. (2021). Farm households’ adoption of climate-smart practices in subsistence agriculture: Evidence from Northern Togo. Environmental Management, 67(5), 949–962. https://doi.org/10.1007/s00267-021-01436-3
- Anthony, B., Kamaludin, A., & Romli, A. (2021). Predicting academic staffs behaviour intention and actual use of blended learning in higher education: Model development and validation. Technology, Knowledge & Learning, 28(3), 1–47. https://doi.org/10.1007/s10758-021-09579-2
- Aregaw, Y. G., Endris, E. A., Bojago, E., & Wang, S. (2023). Factors affecting the competence level of agricultural Extension agents: A comprehensive analysis of core competencies in Northwestern Ethiopia. Education Research International, 2023, 1–21. https://doi.org/10.1155/2023/7928467
- Ayisi, D. N., Kozári, J., & Krisztina, T. (2022). Do smallholder farmers belong to the same adopter category? An assessment of smallholder farmers innovation adopter categories in Ghana. Heliyon, 8(8), e10421. https://doi.org/10.1016/j.heliyon.2022.e10421
- Balogun, A. L., Adebisi, N., Abubakar, I. R., Dano, U. L., & Tella, A. (2022). Digitalization for transformative urbanization, climate change adaptation, and sustainable farming in Africa: Trend, opportunities, and challenges. Journal of Integrative Environmental Sciences, 19(1), 17–37. https://doi.org/10.1080/1943815X.2022.2033791
- Benard, R., Dulle, F., & Lamtane, H. (2020). Challenges associated with the use of information and communication technologies in information sharing by fish farmers in the Southern highlands of Tanzania. Journal of Information, Communication and Ethics in Society, 18(1), 44–61. https://doi.org/10.1108/JICES-11-2018-0085
- Birner, R., Daum, T., & Pray, C. (2021). Who drives the digital revolution in agriculture? A review of supply‐side trends, players and challenges. Applied Economic Perspectives and Policy, 43(4), 1260–1285. https://doi.org/10.1002/aepp.13145
- Bruns, S., Mußhoff, O., & Ströhlein, P. (2022). Information needs and delivery channels: Experimental evidence from Cambodian smallholders. IFLA Journal, 48(4), 661–678. https://doi.org/10.1177/03400352211057148
- Chelanga, N. C., Kariuki, I. M., & Obare, G. A. (2021). Determinants of vertical coordination option choices among smallholder French beans producers in Kenya. Review of Agricultural and Applied Economics (RAAE), 24(2), 78–89. https://doi.org/10.15414/raae.2021.24.02.78-89
- Claessens, F., Castro, E. M., Jans, A., Jacobs, L., Seys, D., Van Wilder, A., Brouwers, J., Van der Auwera, C., De Ridder, D., & Vanhaecht, K. (2022). Patients’ and kin’s perspective on healthcare quality compared to Lachman’s multidimensional quality model: Focus group interviews. Patient Education and Counseling, 105(10), 3151–3159. https://doi.org/10.1016/j.pec.2022.07.007
- Csibi, S., Griffiths, M. D., Demetrovics, Z., & Szabo, A. (2021). Analysis of problematic smartphone use across different age groups within the ‘components model of addiction’. International Journal of Mental Health and Addiction, 19(3), 616–631. https://doi.org/10.1007/s11469-019-00095-0
- Dagar, V., Khan, M. K., Alvarado, R., Usman, M., Zakari, A., Rehman, A. … Tillaguango, B. (2021). Variations in technical efficiency of farmers with distinct land size across agro-climatic zones: Evidence from India. Journal of Cleaner Production, 315, 128109. https://doi.org/10.1016/j.jclepro.2021.128109
- Dirsehan, T., & Van Zoonen, L. (2022). Smart city technologies from the perspective of technology acceptance. IET Smart Cities, 4(3), 197–210. https://doi.org/10.1049/smc2.12040
- Donkoh, S. A., Azumah, S. B., & Awuni, J. A. (2019). Adoption of improved agricultural technologies among rice farmers in Ghana: A multivariate probit approach. Ghana Journal of Development Studies, 16(1), 46–67. https://doi.org/10.4314/gjds.v16i1.3
- Donkor, E. A., Garnevska, E., Siddique, M. I., & Donkor, E. (2021). Determinants of rice farmer participation in the direct marketing channel in Ghana. Sustainability, 13(9), 5047. https://doi.org/10.3390/su13095047
- Drewry, J. L., Shutske, J. M., Trechter, D., Luck, B. D., & Pitman, L. (2019). Assessment of digital technology adoption and access barriers among crop, dairy and livestock producers in Wisconsin. Computers and Electronics in Agriculture, 165, 104960. https://doi.org/10.1016/j.compag.2019.104960
- Fatah, K. S., & Alkaki, Z. R. A. (2021). Application of binary logistic regression model to cancer patients: A case study of data from Erbil in Kurdistan region of Iraq. Zanco Journal of Pure and Applied Sciences, 33(4), 117–128. https://doi.org/10.21271/ZJPAS.33.4.12
- Gebre, G. G., Isoda, H., Nomura, Y., & Amekawa, H., Nomura, H. (2019, September). Gender differences in the adoption of agricultural technology: The case of improved maize varieties in southern Ethiopia. Women’s Studies International Forum, 76, 102264. https://doi.org/10.1016/j.wsif.2019.102264
- Haile, M. G., Wossen, T., & Kalkuhl, M. (2019). Access to information, price expectations and welfare: The role of mobile phone adoption in Ethiopia. Technological Forecasting and Social Change, 145, 82–92. https://doi.org/10.1016/j.techfore.2019.04.017
- Hoang, H. G. (2020). Determinants of the adoption of mobile phones for fruit marketing by Vietnamese farmers. World Development Perspectives, 17, 100178. https://doi.org/10.1016/j.wdp.2020.100178
- Hoang, H. G., & Drysdale, D. (2021). Factors affecting smallholder farmers’ adoption of mobile phones for livestock and poultry marketing in Vietnam: Implications for extension strategies. Rural Extension and Innovation Systems Journal, 17(1), 21–30. https://doi.org/10.3316/informit.685246169618015
- Islam, M. S., Sujan, M. S. H., Tasnim, R., Mohona, R. A., Ferdous, M. Z., Kamruzzaman, S. … Pontes, H. M. (2021). Problematic smartphone and social media use among Bangladeshi college and university students amid COVID-19: The role of psychological well-being and pandemic related factors. Frontiers in Psychiatry, 12, 647386. https://doi.org/10.3389/fpsyt.2021.647386
- Issahaku, H., Abu, B. M., & Nkegbe, P. K. (2018). Does the use of mobile phones by smallholder maize farmers affect productivity in Ghana? Journal of African Business, 19(3), 302–322. https://doi.org/10.1080/15228916.2017.1416215
- Ivanova, A., & Kim, J. Y. (2022). Acceptance and use of mobile banking in central Asia: Evidence from modified UTAUT model. The Journal of Asian Finance, Economics & Business, 9(2), 217–227. https://doi.org/10.13106/jafeb.2022.vol9.no2.0217
- Kabirigi, M., Sekabira, H., Sun, Z., & Hermans, F. (2022). The use of mobile phones and the heterogeneity of banana farmers in Rwanda. Environment Development and Sustainability, 25(6), 1–21. https://doi.org/10.1007/s10668-022-02268-9
- Kaddu, S., Nanyonga, D., & Haumba, E. N. (2020). Role of small-scale farmers in making agricultural market information systems relevant and sustainable in Bugiri district, Uganda. University of Dar Es Salaam Library Journal, 15(2), 69–83.
- Kalimangasi, N. N., Isinika, A. C., & Mwembezi, G. P. (2021). Input demand response analysis of wine grape farmers in Dodoma city and Chamwino District in Dodoma region, Tanzania: Application of the profit function. African Journal of Agricultural Economics and Rural Development, 9(2), 1–9.
- Kansiime, M. K., Alawy, A., Allen, C., Subharwal, M., Jadhav, A., & Parr, M. (2019). Effectiveness of mobile agri-advisory service extension model: Evidence from Direct2Farm program in India. World Development Perspectives, 13, 25–33. https://doi.org/10.1016/j.wdp.2019.02.007
- Kassem, H. S., Alotaibi, B. A., Ghoneim, Y. A., & Diab, A. M. (2021). Mobile-based advisory services for sustainable agriculture: Assessing farmers’ information behavior. Information Development, 37(3), 483–495. https://doi.org/10.1177/0266666920967979
- Khan, M. M., & Mahmood, W. (2022). Technology adoption in Pakistani banking industry using UTAUT. International Journal of Information Technology and Computer Science, 14(1), 32–42. https://doi.org/10.5815/ijitcs.2022.01.04
- Khan, N. A., Qijie, G., Ali, S., Shahbaz, B., & Shah, A. A. (2019). Farmers’ use of mobile phone for accessing agricultural information in Pakistan:. Ciência Rural, 49(10). https://doi.org/10.1590/0103-8478cr20181016
- Khan, N., Ray, R. L., Kassem, H. S., & Zhang, S. (2022). Mobile internet technology adoption for sustainable agriculture: Evidence from wheat farmers. Applied Sciences, 12(10), 4902. https://doi.org/10.3390/app12104902
- Khan, N., Siddiqui, B. N., Khan, N., Khan, F., Ullah, N., Ihtisham, M., Ullah, R., Ismail, S., & Muhammad, S. (2020). Analyzing mobile phone usage in agricultural modernization and rural development. International Journal of Agricultural Extension, 8(2), 139–147. https://doi.org/10.33687/ijae.008.02.3255
- Kilima, F. T., & Chikuni, T. (2021). Factors underlying farmers’ choice of market information system in Lilongwe, Malawi. International Journal of Agricultural Science, Research and Technology in Extension and Education Systems (IJASRT in EES), 11(2), 115–131.
- Kitsiou, A., Despotidi, C., Kalloniatis, C., & Gritzalis, S. (2022). The role of users’ demographic and social attributes for accepting biometric Systems: A Greek case study. Future Internet, 14(11), 328. https://doi.org/10.3390/fi14110328
- Krell, N. T., Giroux, S. A., Guido, Z., Hannah, C., Lopus, S. E., Caylor, K. K., & Evans, T. P. (2021). Smallholder farmers’ use of mobile phone services in central Kenya. Climate and Development, 13(3), 215–227. https://doi.org/10.1080/17565529.2020.1748847
- Kulwijila, M., Makindara, J., & Laswai, H. (2018). Grape value chain mapping in Dodoma region, Tanzania. Journal of Economics & Sustainable Development, 9(2), 171–182. available at. https://core.ac.uk/download/pdf/234648145.pdf
- Kulwijila, M., Makindara, J., & Laswai, H. (2021). Grape value chain mapping in Dodoma region, Tanzania. Journal of Economics and Sustainable Development, 9(2), 171–182. https://core.ac.uk/download/pdf/234648145.pdf
- Ling, Z., Cherry, C. R., & Wen, Y. (2021). Determining the factors that influence electric vehicle adoption: A stated preference survey study in Beijing, China. Sustainability, 13(21), 11719. https://doi.org/10.3390/su132111719
- Li, W., Yuan, K., Yue, M., Zhang, L., & Huang, F. (2021). Climate change risk perceptions, facilitating conditions and health risk management intentions: Evidence from farmers in rural China. Climate Risk Management, 32, 100283. https://doi.org/10.1016/j.crm.2021.100283
- Ma, W., Grafton, R. Q., & Renwick, A. (2020). Smartphone use and income growth in rural China: Empirical results and policy implications. Electronic Commerce Research, 20(4), 713–736. https://doi.org/10.1007/s10660-018-9323-x
- Michels, M., Bonke, V., & Musshoff, O. (2019). Understanding the adoption of smartphone apps in dairy herd management. Journal of Dairy Science, 102(10), 9422–9434. https://doi.org/10.3168/jds.2019-16489
- Michels, M., Fecke, W., Feil, J. H., Musshoff, O., Pigisch, J., & Krone, S. (2020). Smartphone adoption and use in agriculture: Empirical evidence from Germany. Precision Agriculture, 21(2), 403–425. https://doi.org/10.1007/s11119-019-09675-5
- Mlay, F. F. (2021). Understanding marketing challenges along grapes value chain in central areas of Tanzania. The Accountancy and Business Review, 13(1), 1982–2546. https://journals.iaa.ac.tz/index.php/abr/article/view/5
- Moraes, W. B., Madden, L. V., & Paul, P. A. (2022). Characterizing heterogeneity and determining sample sizes for accurately estimating wheat fusarium head blight index in Research plots. Phytopathology®, 112(2), 315–334. https://doi.org/10.1094/PHYTO-04-21-0157-R
- Moshe, I., Terhorst, Y., Opoku Asare, K., Sander, L. B., Ferreira, D., Baumeister, H., Mohr, D. C., & Pulkki-Råback, L. (2021). Predicting symptoms of depression and anxiety using smartphone and wearable data. Frontiers in Psychiatry, 12, 625247. https://doi.org/10.3389/fpsyt.2021.625247
- Mushi, G. E., DiMarzo Serugendo, G., & Burgi, P. Y. (2022). Digital technology and services for sustainable agriculture in Tanzania: A literature review. Sustainability, 14(4), 2415. https://doi.org/10.3390/su14042415
- Mwalupaso, G. E., Wang, S., Rahman, S., Alavo, E. J. P., & Tian, X. (2019). Agricultural informatization and technical efficiency in maize production in Zambia. Sustainability, 11(8), 2451. https://doi.org/10.3390/su11082451
- Ndemdou, B., & Fongang, H. G. F. (2021). Use of information and communication technologies by farmers in Menoua division of the West region of Cameroon. American Journal of Agriculture, 3(1), 55–66. https://doi.org/10.47672/aja.879
- Ndimbo, G. K., Yu, L., & Ndi Buma, A. A. (2023). Icts, smallholder agriculture and farmers’ livelihood improvement in developing countries: Evidence from Tanzania. Information Development, 026666692311652. https://doi.org/10.1177/02666669231165272
- Ngenoh, E., Kurgat, B. K., Bett, H. K., Kebede, S. W., & Bokelmann, W. (2019). Determinants of the competitiveness of smallholder African indigenous vegetable farmers in high-value agro-food chains in Kenya: A multivariate probit regression analysis. Agricultural and Food Economics, 7(1), 1–17. https://doi.org/10.1186/s40100-019-0122-z
- Niessl, A., Allignol, A., & Beyersmann, J. (2020). Estimating state occupation and transition probabilities in non-markov multi-state models subject to both random left-truncation and right-censoring. arXiv preprint arXiv:2004.06514.
- Ninaus, K., Diehl, S., & Terlutter, R. (2021). Employee perceptions of information and communication technologies in work life, perceived burnout, job satisfaction and the role of work-family balance. Journal of Business Research, 136, 652–666. https://doi.org/10.1016/j.jbusres.2021.08.007
- Nwali, P. N., Lai-Solarin, W. I., & Dyaji, L. (2022). Evaluation of small-scale women farmers’ utilization of ICT in accessing agricultural information in Gwagwalada area council, Abuja, Nigeria. Science and Technology, 3(7), 8–15. https://doi.org/10.36686/Ariviyal.GR.2022.03.07.036
- Otene, V. A., Ezihe, J. A., & Torgenga, F. S. (2018). Assessment of mobile phone usage among farmers in Keana local government area of Nasarawa state, Nigeria. Journal of Agricultural & Food Information, 19(2), 141–148. https://doi.org/10.1080/10496505.2017.1361829
- Park, J., Hong, E., & Le, H. T. (2021). Adopting autonomous vehicles: The moderating effects of demographic variables. Journal of Retailing and Consumer Services, 63, 102687. https://doi.org/10.1016/j.jretconser.2021.102687
- Peng, Z. (2022). An empirical study of mobile teaching: Applying the UTAUT model to study University teachers’ mobile teaching behavior. Curriculum and Teaching Methodology, 5(5), 55–70. https://doi.org/10.23977/curtm.2022.050510
- Puriwat, W., & Tripopsakul, S. (2021). Explaining an adoption and continuance intention to use contactless payment technologies: During the COVID-19 pandemic. Emerging Science Journal, 5(1), 85–95. https://doi.org/10.28991/esj-2021-01260
- Qazi, W. A., Razzaq, M., Bokhari, N. A., Jahan, S., Shahzadi, J., & Begum, R. (2022). Availability of physical therapists for women after their cesarean section. Rawal Medical Journal, 47(1), 241–241. http://www.rmj.org.pk/fulltext/27-1579333666.pdf
- Quandt, A., Salerno, J. D., Neff, J. C., Baird, T. D., Herrick, J. E., McCabe, J. T., Hartter, J., & Xu, E. (2020). Mobile phone use is associated with higher smallholder agricultural productivity in Tanzania, East Africa. PLoS One, 15(8), e0237337. https://doi.org/10.1371/journal.pone.0237337
- Quaye, W., Onumah, J. A., Boimah, M., & Mohammed, A. (2022). Gender dimension of technology adoption: The case of technologies transferred in Ghana. Development in Practice, 32(4), 434–447. https://doi.org/10.1080/09614524.2021.2000588
- Quy, V. K., Hau, N. V., Anh, D. V., Quy, N. M., Ban, N. T., Lanza, S., Randazzo, G., & Muzirafuti, A. (2022). IoT-enabled smart agriculture: Architecture, applications, and challenges. Applied Sciences, 12(7), 3396. https://doi.org/10.3390/app12073396
- Rahman, M. S., Haque, M. E., & Afrad, M. S. I. (2020). Utility of mobile phone usage in agricultural information dissemination in bangladesh. East African Scholars Journal of Agriculture and Life Sciences, 3(6), 154–170. https://doi.org/10.36349/EASJALS.2020.v03i06.001
- Rahman, M. M., Tabash, M. I., Salamzadeh, A., Abduli, S., & Rahaman, M. S. (2022). Sampling techniques (probability) for quantitative social science researchers: A conceptual guidelines with examples. Seeu Review, 17(1), 42–51. https://doi.org/10.2478/seeur-2022-0023
- Rahmiati, R., Susanto, P., Hasan, A., & Pujani, V. (2022). Understanding use behavior in mobile banking: An extended of UTAUT perspective. AFEBI Management and Business Review, 7(1), 39–46. https://doi.org/10.47312/ambr.v7i01.555
- Rissing, A., Inwood, S., & Stengel, E. (2021). The invisible labor and multidimensional impacts of negotiating childcare on farms. Agriculture and Human Values, 38(2), 431–447. https://doi.org/10.1007/s10460-020-10162-1
- Rode, J. B., Dent, A. L., Benedict, C. N., Brosnahan, D. B., Martinez, R. L., & Ditto, P. H. (2021). Influencing climate change attitudes in the United States: A systematic review and meta-analysis. Journal of Environmental Psychology, 76, 101623. https://doi.org/10.1016/j.jenvp.2021.101623
- Rosalia, F. (2022). Government administration and Policy implementations: A cross-sectional Research on citizens satisfaction. Social Space, 22(2), 418–444.
- Ruzzante, S., Labarta, R., & Bilton, A. (2021). Adoption of agricultural technology in the developing world: A meta-analysis of the empirical literature. World Development, 146, 105599. https://doi.org/10.1016/j.worlddev.2021.105599
- Sennuga, S. O., Fadiji, T. O., & Thaddeus, H. (2020). Factors influencing adoption of improved agricultural technologies (IATs) among smallholder farmers in Kaduna state, Nigeria. International Journal of Agricultural Education and Extension, 6(2), 382–391.
- Serdar, C. C., Cihan, M., Yücel, D., & Serdar, M. A. (2021). Sample size, power and effect size revisited: Simplified and practical approaches in pre-clinical, clinical and laboratory studies. Biochemia Medica, 31(1), 27–53. https://doi.org/10.11613/BM.2021.010502
- Siaw, A., Jiang, Y., Twumasi, M. A., & Agbenyo, W. (2020). The impact of internet use on income: The case of rural Ghana. Sustainability, 12(8), 3255. https://doi.org/10.3390/su12083255
- Singh, J., & Kaur, R. (2021). Influencing the intention to adopt anti-littering behavior: An approach with modified TPB model. Social Marketing Quarterly, 27(2), 117–132. https://doi.org/10.1177/15245004211013333
- Sousa, F., Nicolay, G., & Home, R. (2019). Video on mobile phones as an effective way to promote sustainable practices by facilitating innovation uptake in Mali. International Journal of Sustainable Development Research, 5(1), 1–8. https://doi.org/10.11648/j.ijsdr.20190501.11
- Spencer, S., Samateh, T., Wabnitz, K., Mayhew, S., Allen, H., & Bonell, A. (2022). The challenges of working in the heat whilst pregnant: Insights from gambian women farmers in the face of climate change. Frontiers in Public Health, 10, 152. https://doi.org/10.3389/fpubh.2022.785254
- Srimaneekarn, N., Hayter, A., Liu, W., Tantipoj, C., & Khurshid, Z. (2022). Binary response analysis using logistic regression in dentistry. International Journal of Dentistry, 2022, 1–7. https://doi.org/10.1155/2022/5358602
- Tamar, M., Wirawan, H., Arfah, T., & Putri, R. P. S. (2021). Predicting pro-environmental behaviours: The role of environmental values, attitudes and knowledge. Management of Environmental Quality: An International Journal, 32(2), 328–343. https://doi.org/10.1108/MEQ-12-2019-0264
- Tamirat, N., & Zeleke, T. (2021). Analysis of onion market value chain among smallholder farmers in rural Ethiopia: A case study of south bench woreda in bench maji zone, Ethiopia. Journal of Smart Economic Growth, 6(1), 49–74.
- Tang, K. L., Aik, N. C., & Choong, W. L. (2021). A modified UTAUT in the context of m-payment usage intention in Malaysia. Journal of Applied Structural Equation Modeling, 5(1), 40–59. https://doi.org/10.47263/JASEM.5(1)05
- Thar, S. P., Ramilan, T., Farquharson, R. J., Pang, A., & Chen, D. (2021). An empirical analysis of the use of agricultural mobile applications among smallholder farmers in Myanmar. Electronic Journal of Information Systems in Developing Countries, 87(2), e12159. https://doi.org/10.1002/isd2.12159
- Ujakpa, M. M., Kristof, A., Domingos, A., Hashiyana, V., Suresh, N., Osakwe, J. O., & Iyawa, G. (2021, May). Farmers’ use of mobile devices in developing countries. Proceedings of the 2021 IST-Africa Conference (IST-Africa) (pp. 1–7). IEEE.
- Yang, M., Mamun, A. A., Mohiuddin, M., Nawi, N. C., & Zainol, N. R. (2021). Cashless transactions: A study on intention and adoption of e-wallets. Sustainability, 13(2), 831. https://doi.org/10.3390/su13020831
- Yao, B. H., Shanoyan, A., Schwab, B., & Amanor-Boadu, V. (2022). Mobile money, transaction costs, and market participation: Evidence from Côte d’Ivoire and Tanzania. Food Policy, 112, 102370. https://doi.org/10.1016/j.foodpol.2022.102370
- Yaseen, M., Ahmad, M. M., Soni, P., Kuwornu, J. K., & Saqib, S. E. (2021). Factors influencing farmers’ utilisation of marketing information sources: Some empirical evidence from pakistan. Development in Practice, 33(1), 1–13. https://doi.org/10.1080/09614524.2021.1911941
- Yuliana, P. D., & Aprianingsih, A. (2022). Factors involved in adopting mobile banking for Sharia banking sector using UTAUT 2. Jurnal Keuangan dan Perbankan, 26(1), 184–207. https://doi.org/10.26905/jkdp.v26i1.6858
- Yusoff, M. S. B., Arifin, W. N., & Hadie, S. N. H. (2021). ABC of questionnaire Development and validation for survey Research. Education in Medicine Journal, 13(1), 97–108. https://doi.org/10.21315/eimj2021.13.1.10
- Zheng, H., & Ma, W. (2023). Smartphone-based information acquisition and wheat farm performance: Insights from a doubly robust IPWRA estimator. Electronic Commerce Research, 23(2), 633–658. https://doi.org/10.1007/s10660-021-09481-0
Appendix I:
Questionnaire
The purpose of this study is to examine the influence of socio-demographic factors on the use of mobile phones for accessing agricultural marketing information by grape smallholder farmers in Dodoma, Tanzania. Successful accomplishment of the study will provide knowledge on how such factors enhance access to agricultural marketing information. The information collected through this questionnaire will be used for the intended research purposes.
SECTION A: GENERAL INFORMATION
SECTION B: RESPONDENTS SOCIO-DEMOGRAPHIC DATA
Sex of respondent 0=Male ()1=Female ()
Age in years
Marital status 0=Single ()1=Married ()
What is your highest level of education? 0=No formal education () 1=primary education () 2=secondary education 3=college/university () 4=Others, specify
How many members are you in your family (household size)
For how long have you been farming (farming experience)
What is your total farm size (acres)
What is your average annual income (Tshs)
SECTION C: MOBILE PHONE OWNERSHIP
Which of the following mobile phone (s) do you own 1=Simple () 2=Smartphone () 3=Both ()
Do you share your mobile phone (s) 1=Yes () 0=No ()
With whom do you share your mobile phone with? 1= Friends () 2=Relatives () 3=Neighbours () 4=Others, specify
Which company provides you with mobile phone service? 1=Vodacom () 2=Tigo () 3= Airtel () 4=Halotel () 5=TTCL (),6= Others, specify
How many SIM cards do you use for your mobile phone
Why do you use more than one SIM card? 1=Benefiting from different promotion () 2=Saving cost by making on-set calls () 3=Alternating when there is no network coverage () 4=Others, specify
Which application does your mobile phone have 1= Voice calls () 2=SMS () 3=FM radio () 4=Camera () 5=Others, specify
For how long have you been using mobile phone (ownership timeframe)
How much on average do you spend on mobile phone weekly(Tshs)
SECTION D: MOBILE PHONE USE IN ACCESSING AGRICULTURAL MARKETING INFORMATION
Do you need agricultural marketing information? 1= Yes () 0=No ()
If Yes, how do you rate the availability of agricultural marketing information?
(3) Have you tried to find agricultural marketing information through mobile phones 1=Yes () 0=No ()
(4) Please rate the accessibility of agricultural marketing information through mobile phones?
(5) Is the agricultural marketing information accessed through mobile phone relevant?
Thank you for your cooperation
Appendix II:
Focus Group Discussion Guide
The purpose of this study is to examine the influence of socio-demographic factors on the use of mobile phones for accessing agricultural marketing information by grape smallholder farmers in Dodoma, Tanzania. Successful accomplishment of the study will provide knowledge on how such factors enhance access to agricultural marketing information. The information collected through this focus group discussion guide will be used for the intended research purposes.
SECTION A: GENERAL INFORMATION
SECTION B: DISCUSSION QUESTIONS
Which type (s) of mobile phone do you own?
If you don’t own mobile phone from whom do you access such mobile phones?
How many mobile phones do you own and why?
Do you need agricultural marketing information in your grape farming activities?
If Yes which agricultural marketing information, do you normally require and why?
How often do you use mobile phone for accessing agricultural marketing information?
How far do you think mobile phone have helped you to access agricultural marketing information?
How do you evaluate the cost of owning and using mobile phone to access agricultural marketing information?
In your opinion how do you evaluate mobile phone usage behaviour among different groups of farmers according to their sex, age, marital status, education, farming experience, household size, and income?
Thank you for your cooperation