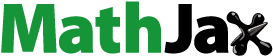
Abstract
This paper examines the post-pandemic performance of micro, small, and medium-sized firms using Self-Organizing Maps (SOMs), a type of Artificial Neural Network that groups patterns based on their similarities. The goal is to identify the key characteristics that enable firms to face market changes and overcome the effects of the global COVID-19 pandemic crisis. Considering business failure theory, a set of critical factors (including internal production processes, firm age, number of employees, resilience, financial resources, commercial strategies, management, and the impact of external factors) is used to assess the performance of Argentinian firms. The study categorizes these firms into four clusters based on their patterns. The results reveal a trade-off between a firm’s age and its number of employees, confirming that younger firms with fewer employees, limited financial resources, relatively weaker management, internal production process issues, and lower resilience tend to perform poorly, despite facing fewer impact of external factors. Consequently, the findings emphasize the significance of internal fundamentals and resilience in achieving success or avoiding failure. This highlights the effectiveness of SOM as a tool to visualize the characteristics that lead to successful paths and the survival of firms.
1. Introduction
The pandemic and the post-pandemic period are recognized as one of the most important shocks that affected small and medium-sized enterprises (SMEs) and ventures. This situation has generated the study of business failure and key factors relevant to business performance. Particularly, this period has been acknowledged as the major exogenous shock that has altered the landscape of small and large firms (Wenzel et al., Citation2020; Meahjohn & Persad, Citation2020; Brown et al., Citation2020; Liguori & Pittz, Citation2020; among others).
In response, researchers have explored the concepts of resilience and innovation as crucial factors for post-pandemic success. For example, Portuguez Castro and Gómez Zermeño (Citation2021) conducted a systematic literature review, emphasizing the emergence of resilience as a vital entrepreneurial skill that enables firms to adapt and thrive. They identified various factors contributing to resilience. Additionally, Caballero-Morales (Citation2021) underscored the significance of innovation and cost optimization for business recovery, especially among SMEs. Finally, Kantis and Angelelli (Citation2020) shed light on the pandemic’s impact on the entrepreneurial ecosystem in Latin America and the Caribbean. Their survey-based study revealed that the crisis hit most enterprises hard, particularly younger ones and those with shorter histories. However, it also highlighted the resilience demonstrated by technology sector firms and dynamic start-up.
Some studies agree that SMEs and ventures were greatly affected by the pandemic and that their performance is linked to multiple causes that interact to conclude in different trajectories of business success or failure. A variety of parametric and non-parametric techniques have been applied to explain business failure process since the pioneers Beaver’s univariate model (Beaver, Citation1966) and Altman’s multivariate model (Altman, Citation1968). Due to the nature of the problem, affected by diverse variables and expert analyst opinions, it is suitable to apply tools taken from artificial intelligence and big data analysis.
Several authors such as Tseng and Hu (Citation2010) and Alaka et al. (Citation2018) concluded that the artificial neural networks are a growing technique field for bankruptcy prediction, given that their architecture is straightforward and yields promising results. Many studies used different types of neural networks for bankruptcy prediction despite its many undesirable features such as computational intensity, absence of formal theory, and sometimes illogical behaviour. A typical neural network consists of nodes interconnected by layers to forecast results. Many architectures, algorithms, and training methods can be used to identify trajectories and predict failure.
In this work, we examined micro, small and medium-sized enterprises (MSMEs) in Argentina using a methodology based on Artificial Neural Networks (ANNs), the Self-Organizing Maps (SOMs), to analyse if firms’ performance can be clustered into different trajectories or groups according to their characteristics and key variables. The SOMs learning process is unsupervised, so the network uses the internal properties of the input data to organize the information depending on their similarities to group them into clusters. This is a type of artificial neural network algorithms, which belongs to the unsupervised learning category in order to reduce ideological bias and is applied to visualize and interpret large, high-dimensional data sets. Therefore, the ANNs approach based on SOMs can provide a clustering and displays on how the key factors identified affect firms’ performance. Therefore, the key elements for better performance can be reproduced by other MSMEs to reduce fail probabilities.
This technique offers a spatial distribution of the firms on a two-dimensional map and shows the relationship between the variables introduced and those identified as relevant for business performance. This analysis is useful for evidencing the characteristics of firms based on expert opinions in order to design specific policies to overcome the firms’ problems and contribute with the expert assessment in the diagnosis of small sample. To our knowledge, there are no similar works that study the key factors for success and cluster the firms through SOMs in the post-pandemic scenario and in this specific region in Argentina, given that the data sample is obtained through a local survey of our own, in the southwest of Buenos Aires province, Argentina. Moreover, this methodology is a novel application to Argentinian enterprises. SOMs have been globally used in numerous studies of business failure and firms’ performance to cluster and classify firms (Del Brio & Serrano-Cinca, Citation1993; Serrano-Cinca, Citation1996; Alam et al., Citation2000; Länsiluoto et al., Citation2004; Lee et al., Citation2005; Du Jardin, Citation2018; Chaves-Maza & Martel, Citation2020, among others).
The results obtained reveal four distinct performance patterns during the post-pandemic period, underlining the critical significance of resilience, access to financing, having a strong and experienced management team, an innovative internal production process, and the ability to introduce new strategies to overcome the situation for achieving success. On the other hand, younger firms with fewer employees, limited financial resources, relatively weaker management, issues in the internal production process, and lower resilience exhibit the poorest performance, despite the minimal impact of external factors. These findings emphasize the importance of internal factors and resilience in either achieving success or averting failure. This suggests that SOMs can serve as a valuable tool for visualizing the characteristics of successful pathways and the survival of firms.
The paper is organized in seven sections. After the introduction, Section 2 presents the theoretical background. Sections 3 and 4 describe the data and methodology used in the study. Section 5 establishes the application to the case of study. In Section 6, the results are discussed. Finally, Section 7 provides the main conclusions of the study.
2. Literature review
In the context of this research, the variables are selected taking as reference the literature on the prediction of business failure and insolvency situations. This study receives special attention and growing interest in periods of instability and overall crisis, such as the COVID-19 pandemic, in order to prevent bankruptcies. Business failure has been an important research area since the 1960s with Beaver’s (Citation1966) univariate model and the multivariate one of Altman (Citation1968). These failure models compare and classify firms according to quantitative indicators to predict or distinguish between healthy and unhealthy businesses. Moreover, a wide variety of models and techniques have been applied to predict distress situations and improve estimation results (Balcaen & Ooghe, Citation2006; Kumar & Ravi, Citation2007; Bahrammirzaee, Citation2010; Kumar & Ravi, Citation2007; Sun et al., Citation2014; Alaka et al., Citation2018; Scherger et al., Citation2019; Shi & Li, Citation2019; Kim et al., Citation2020; among others). In addition, some of the studies have focused on detecting the factors or causes that generate firms problems (Argenti, Citation1976, Citation1983; Gabás Trigo, Citation1997; Scherger et al., Citation2014; Citation2015; Terceño et al., Citation2018, among others) that are invisible to the expert eyes. The literature also has differentiated between the impact of endogenous causes, which can be controlled by the management, and the impact of exogenous causes, originated from external factors.
In summary, considering the literature review, we proposed some relevant variables to analyse firms’ performance after the pandemic, in terms of internal production process, firm age, number of employees, resilience, financing availability, commercial strategies implemented, management characteristics, and impact of external factors. The selected variables according to the literature review are detailed below.
- Internal production process is one of the aspects mentioned in the literature that have important impact on firm’s performance and can trigger insolvency situations. For example, technical efficiency is considered in Gabás Trigo (Citation1997) when the inefficient production system is mentioned as a general cause of bankruptcy. Moreover, Madrid and García (Citation2006) observed that management can influence the internal context of firms by modifying the weak technological position, the scarce innovation activities and the improvements in the product and/or service quality. Lastly, in their study of 72 causes, Scherger et al. (Citation2015) included the areas of innovation and technology, cost optimization, technical efficiency and quality, and prices as relevant factors for the firms’ diagnosis.
The CB Insights (Citation2021) provides yearly top reasons of start-up failure based on post-mortem analysis of 111 start-ups. For the year 2021, the main causes linked to this area are: i) got outcompeted, ii) pricing/cost issues, iii) product mistimed, and iv) poor product. Moreover, Caballero-Morales (Citation2021) identified innovation and cost optimization as key aspects of business recovery in the ongoing and post-COVID-19 pandemic period, and Sanhokwe (Citation2022) studied SMEs survival probabilities incorporating new and improved processes or products and services to reduce the likelihood of failure.
Therefore, taking this background into account, we selected this variable related to the internal production process, which includes the assessment of the characteristics and technology of the product or service, its quality and price, and cost of optimization.
- Firm age is also considered in many studies that point out the importance of business age for the analysis (Grunert et al., Citation2005; Kücher et al., Citation2020). For example, Gabás Trigo (Citation1997) highlighted the high mortality of start-ups and young firms. In the same line, Kantis and Angelelli (Citation2020) identified that the firms most affected by the pandemic are those with the shortest trajectory and younger. However, they noted that firms in the technology sector and some young, more dynamic ones had less pronounced impacts. In these terms, through conceptual maps, Ooghe and De Prijcker (Citation2008) showed the relationship between causes and effects, establishing four failure trajectories: unsuccessful start-up, firms with ambitious growth, those with little growth, and apathetic growth of an established and old firm. Hence, there is no agreement on age influence on firm performance, and this is even more uncertain after the pandemic shock.
- Number of employees. Some studies (Altman et al., Citation2022; Rhaiem & Halilem, Citation2023; Scarlat & Delcea, Citation2011; Scherger et al, Citation2015) include the impact of employees in the prediction of business failure. In this case, the models generally find that higher employment is in line with better firm performance, but the pandemic may have changed this correlation because of the high labour costs faced by firms in this context.
- Resilience. Argenti (Citation1976) regarded this factor as the flexibility to respond to changes. Since then, Gabás Trigo (Citation1997), Scarlat and Delcea (Citation2011) and Scherger et al. (Citation2015) among others added this element in the analysis. For instance, in the pandemic scenario, Portuguez Castro and Gómez Zermeño (Citation2021) highlighted that resilience is a key capacity that allows firms to adapt and strengthen themselves. In addition, many empirical works note the importance of firm flexibility and resilience to overcome the pandemic crisis (CAME, Citation2021a, b; Kantis & Angelelli, Citation2020).
- Financial availability. Financial resources are one of the most recognized obstacles to business development. On the one hand, Argenti (Citation1983) focused on the weak role of the financial director, the absence of middle managers, the lack of budgetary control, the absence of financial planning, the lack of accounting, and the response to change as determinants of bad performance. On the other, Lussier (Citation1995) associated this factor with the adequacy of start-up capital and financial control. In addition, Gabás Trigo (Citation1997) linked it to high indebtedness, bankruptcy problems, delinquency, and payment issues as financial determinants of failure.
Furthermore, the CB Insights (Citation2021) mentioned that the causes of running out of cash and failing to raise new capital are relevant for the study. In addition, Sanhokwe (Citation2022) found that fresh capital injection improves enterprise probabilities of survival. All studies conclude that better financing possibilities imply better firm performance.
- Commercial strategies. Many investigations emphasize the importance of commercial strategies for business development. For example, Lussier (Citation1995), Theng and Lim (Citation1996), Halabí and Lussier (Citation2014) and Eschker et al. (Citation2017) suggested that an inappropriate marketing strategy could end business. On the other hand, Ghosh et al. (Citation2001) and CitationScherger et al. (Citation2015) highlighted the importance of purchasing policy, logistics, delivery systems, and the ability to identify and focus on a market niche to improve firm performance. Moreover, during the pandemic, some of the empirical studies (CAME, Citation2021a, b) show that firms had to transform and adopt e-commerce, new sales platforms and ways to connect with customers and suppliers.
- Management characteristics. Some authors (Argenti, Citation1976, Citation1983; Gabás Trigo, Citation1997; Ghosh et al., Citation2001; Kücher et al., Citation2020; Lussier, Citation1995; Ooghe & De Prijcker, Citation2008; Scherger et al., Citation2015) considered aspects such as management experience, lack of budgetary control, absence of planning, centralization of decision-making, availability of professional advice, owner education, quality of staff, good network system, and business learning. Furthermore, the CB Insights (Citation2021) mentioned some characteristics linked to a flawed business model, not having the right team, disharmony among the team and investors, pivot gone bad, burn out, and lack of passion. Lastly, Portuguez Castro and Gómez Zermeño (Citation2021) stressed the role of strategic business management and decisions to face the pandemic. Considering the background, this work suggests management experience, owner training, having a clear business plan, business working hours, networking, and having external advice for the analysis.
- External factors. These exogenous changes are regarded by a set of authors who studied the causes of business failure (Argenti, Citation1976, Citation1983; Gabás Trigo, Citation1997; Khelil, Citation2016; Scherger et al., Citation2015). For example, the most common causes lie within the general environment, which includes economic, technological, political, social, and foreign country performance changes. In this line, McGahan and Porter (Citation1997) and Rumelt (Citation1997) mentioned that external factors are those that best explain business failure, rather than internal causes, and they are characterized by technological uncertainty and changes in demand, in consumer preference and in competition between firms. On the other hand, Kücher et al. (Citation2020) suggested that internal factors are the root, dismissing the influence of external ones as the primary determinants of business failure or success. Given the importance of external factors, despite the disagreement, we propose to consider the impacts of the macroeconomic situation, the COVID-19 pandemic, and public policy programs on firm performance.
3. Data
SMEs and ventures are a great driver of economic activity and job creation around the world. They constitute about 90% of the economic fabric of most countries (Kuzmisin & Kuzmisinova, Citation2017). The role of SMEs in economic development is even more imperative in developing countries, where they help encourage innovation and certain structural changes in the market (Singh & Kaur, Citation2019).
We used data from an own survey conducted online, during the months of July and August in 2021, to small firms of the southwest of the Buenos Aires province, Argentina. The survey has been designed to detect the relevant variables for the study applying the snowball methodology. The latter is a type of non-probability sampling applied when potential participants are difficult to find or when the sample is limited to a very small subset of the population. Through an anonymous Google form, and guaranteeing that the information collected would only be used for research purposes, 168 firms responded to the survey. In the dataset, we have identified 154 active firms and 14 that closed their activity or went bankrupt during the pandemic. These 154 firms come from 12 different cities and are selected to evaluate their post-pandemic trajectories by applying SOMs. The selected variables are presented and described in Table .
Table 1. Variables’ description
Each of these variables comes from quantifying the labels given to the experts to value the importance of each factor for firms’ performance. This method has been applied following Zimmermann (Citation1991), who argues that higher values correspond to the most impactful causes or factors. Therefore, a set of linguistic labels is made available to the experts to evaluate the presence of each cause. Then, the linguistic labels are translated into a quantitative scale [0,1] that displays the incidence of each cause, calculated through cumulative frequency for the defined scale in each case.
After training the network, the firm situation, identifying through three linguistic labels, is used to explain the results of the clustering. We identify three different labels of firms according to their post-pandemic performance: Good (G), Regular (R) and Bad (B). These labels are determined based on the entrepreneur’s perspective regarding the firms’ situation.
Based on the models that investigate the causes of business survival and failure and the descriptive statistics of the survey, we present eight hypotheses that reflect the expected influence of the selected variables over the post-pandemic situation on firm development:
H1:
The importance of aspects related to the internal production process positively influences post-pandemic firm performance. This implies that the firm is seeking to revalue the product and service in the market.
H2:
The impact of firm age on post-pandemic performance is not defined, but more established and experienced firms can be expected to have less pronounced impacts.
H3:
The number of employees can positively or negatively influence post-pandemic firm performance.
H4:
Resilience and flexibility to adapt to market conditions and changes have positive effects on post-pandemic firm performance.
H5:
The availability of financing sources to face commitments, investments, and business opportunities improves firm performance.
H6:
Adopting commercial strategies linked to e-commerce, promotions, and new platforms improves the situation of firms in the post-pandemic scenario.
H7:
Having an experienced, orderly, and solid management increases post-pandemic firm performance.
H8:
The external factors greatly impact post-pandemic firm performance.
4. Methodology: Self-organizing Maps
ANNs are a powerful tool in data analysis due to their ability to identifying complex functional relationships. In these networks, there are two kinds of learning process: supervised or unsupervised. In the former, an external agent compares the output of the network with the desired output and modifies the weights in order to obtain a robust result. In the latter, the network itself fits the output using distance functions that measure the similarity between the input patterns of the system. SOMs are a type of ANNs that have an unsupervised learning process, which determines the order of items considering their similarities and differences. They are especially suitable for clustering tasks, since this kind of networks gathers elements according to their homogeneity regarding all the attributes or variables defined in the investigation. There has been an increase in their use in many fields for data mining, such as market segmentation, ecology, or bioinformatics analysis (Lanzarini et al., Citation2017; Park et al., Citation2003), as well as for the study of a great variety of relationships of economic and financial variables since the pioneers’ works of Del Brio and Serrano-Cinca (Citation1993) and Serrano-Cinca (Citation1996).
The unsupervised SOMs are designed to convert high-dimensional data and greater number of samples into a simpler relationship of similarity graphs. In this article, the variables selected are considered to identify different groups or trajectories of firms in the post-pandemic scenario. In this sense, similar samples are closer on the map than the most dissimilar ones. For this reason, SOMs turned to be appropriate in this complex task of visualization and exploration of non-linear relationships and high-dimensional datasets.
The process by which SOMs obtain the feature map is as follows. First, the input data are described in terms of n-component vectors. In this way, a set of patterns (P) is obtained as with p = 1, 2, …n, where
represents the value of the i-th component for the pattern p. All the patterns are introduced into the system on a similar scale. At each training step, the patterns are chosen linearly and compared with the weight vectors that form the rows in the matrix
, where
is the weight associated with the connection between the input neuron i and the output neuron k. The winning neuron, called the Best-Matching Unit (BMU), is the one whose weight vector has the minimum distance from the pattern. Although different definitions of distance can be used, the most common is the Euclidean one. Therefore, the winning unit k* satisfies:
Once the winning neuron has been found, the weights associated with it and its neighbourhood area are updated. This is why the learning process is both competitive and cooperative. It is competitive, because only one neuron wins due to the previous rule, and cooperative, because not only the BMU is modified but also its topological neighbours.
Two variants of the SOMs training algorithm have been designed: the traditional sequential training and the batch training. This work used the second one, since weights are calculated more efficiently with Matlab (Vesanto, Citation2000).
The new weights are obtained as a result of the following expression:
where denotes the learning rate, which has a value between 0 and 1 and decreases monotonically with the number of iterations. The neighbourhood area of the winning unit is formed by adjacent units in a rectangular or hexagonal area, and whose radius decreases with the number of iterations as well.
The m number of neurons in the output layer is a key issue in the SOMs application and depends on the amount of data being analysed and the number of dimensions included. Although there is no deterministic theoretical principle to define the optimum map size, there is a trade-off in this regard: if the map size is too small, it might not explain some important differences within the data input. Conversely, if it increases, the tool loses explanatory power, since it is more difficult to identify similar patterns. For the present data set, the best map to represent the distribution of enterprises turns out to be a relatively small one of 8 × 8 neurons (64 cells in total).
The maps were generated using the SOM 2.0 Toolbox, which is a free access Matlab library containing the implementation of Kohonen Self-Organizing Maps algorithms.Footnote1 It offers various visualization options and the calculation of k-means clusters for the final map. In the initial step, the map is created using the som_data_struct script. Then, the variables are normalized using som_normalize (range method), and finally, the network is trained using som_make with default settings: linear initialization, Batch method, a square network (equal number of neurons vertically and horizontally), and a hexagonal grid. There are no strict rules to determine these hyperparameters. Other configurations are tested and the result remained consistent.
5. Application of Self-organizing Maps
Once the network is implemented, we have obtained a map that represents the distribution of firms, which comprises 64 neurons (8 rows and 8 columns). The corresponding patterns (firms) have been labelled as “G” for good or very good post-pandemic performance, “R” for average post-pandemic performance, and “B” for bad or very bad post-pandemic performance, all by mid-2021.
Similar firms are placed in the same area of the map. Through SOMs (Figure ), the feature map (Figure ), and the groups of firms (Table ), we have detected similarities between the firms included in the four clusters.
Table 2. Analysis of the groups of firms
Group 1 comprises 24 firms, which present heterogeneous characteristics. Nonetheless, these firms show the best post-pandemic performance. Moreover, they have an average age of 16 years (Var_2), more than 10 employees (Var_3), and are the least affected by external factors (Var_8). In comparative terms, this group evidences less resilience (Var_4), limited access to financing (Var_5), and the worst average in aspects related to the internal production process (Var_1) and management (Var_7), in spite of implementing fewer commercial strategies (Var_6). According to the theory, this group does not respond to the expected guidelines.
Group 2 includes 22 firms. It presents a relatively bad management (Var_7) and comprises more mature firms, with an average age of 32 years (Var_2) and more than 25 employees (Var_3). Despite the level of resilience (Var_4), the availability of financing (Var_5) and the strengths in the internal production process (Var_1), they evidence the worst performance although they have implemented fewer commercial strategies (Var_6) and not have the worst management (Var_7). This group has been affected moderately by external factors (Var_8). This can be the situation for older and established firms, which are less flexible and have high fixed costs in more mature sectors. In terms of Ooghe and De Prijcker (Citation2008), these can be associated with trajectories for apathetic growth of an established firm.
Group 3 is formed by 46 firms. It shows the second best post-pandemic performance. These are middle-aged firms of 11 years old on average (Var_2) and have a small number of employees (Var_3), which possibly implies a lower fixed cost in the pandemic. They present a relatively good internal production process (Var_1), the highest level of resilience (Var_4), availability to financing (Var_5), a strong and experienced management (Var_7) to face the problems and have introduced commercial strategies (Var_6) to overcome the situation. In addition, they are strongly affected by external factors (Var_8), but internal strengths mitigate threats. Kantis and Angelelli (Citation2020) indicated that technology sector firms and some young, more dynamic firms had registered less pronounced impacts and greater resilience, confirming the characteristics of this group.
Group 4 is the largest one, comprising 62 firms that present the worst post-pandemic performance. They are the youngest firms with an average age of 6 years (Var_2) and the lowest number of employees (Var_3). Besides the efforts in implementing new commercial strategies (Var_6), they do not offset the weaknesses in financial availability (Var_5), in the relatively poor management (Var_7), in the internal production process (Var_1) and in resilience (Var_4), despite the low impact of external factors (Var_8). According to the theory, this group may include young, less-structured firms with short trajectory, which are the most affected by the pandemic highlighting the importance of the internal fundamentals to success or avoid failure.
6. Discussion
We found four groups with different levels of performance based on the opinion of their managers. According to the hypotheses presented above, firm performance has a positive relationship with firm age and employees. Young and small firms are more vulnerable in economic crisis like the pandemic, except for Group 2, which shows relatively bad performance. This could be explained by the lack of flexibility and the high costs to adapt to crisis periods. This group is the largest in terms of employees, comprises older firms, and has more access to financing. Nonetheless, these characteristics do not necessarily imply good performance, contrary to the hypothesis presented.
With respect to the characteristics of the product or service and the new ways of commercialization, it can be seen that firms with lower performance have to revalue and adapt their main sales objective, since their performance has been decreasing. Firms belonging to Group 1 have experienced success in the post-pandemic scenario. However, in order to sustain this performance, they need to enhance their internal production processes, improve management and decision-making, and bolster their resilience. These internal fundamentals are essential for long-term survival.
Similar behaviours related to resilience, improve management, innovative and competitive internal production process are fundamental to achieve better performances and avoid failure in the case of Groups 2, 3 and 4.
These results have an important implication for understanding the influence of different aspects or characteristics of firms in times of crisis. Those who are comfortable with their performance have not to revalue the main aspects of their firms, while others showed that making efforts to improve their firms has a positive effect on their performance over the post-pandemic crisis. A policy recommendation can be inferred, suggesting that more vulnerability firms, such as small, newly established firms with limited financial resources, require assistance from public policies to survive in extreme crisis periods. Additionally, large companies operating in labour-intensive mature sectors may also benefit from public support to face such external shocks.
7. Conclusions
It is known that small firms and new enterprises are a great driver of economic growth and job creation. In times of crisis such as the pandemic, a rapid and risky change of business plans is required in order to survive. In this work, an exploratory analysis is carried out to detect some common patterns or characteristics of firms, decisive in the post-pandemic trajectory. We used data from an own survey conducted online during the months of July and August 2021, to small firms in the southwest of Buenos Aires province, Argentina.
To attain the stated goal, an unsupervised SOMs methodology is employed to simplify the linkage of numerous pertinent variables to the firms’ trajectories, resulting in the identification of four distinct clusters.
The COVID-19 pandemic has brought unprecedented challenges to the global economy, with profound implications for entrepreneurs and businesses. This study has successfully demonstrated the existence of certain patterns governing the vulnerability of MSMEs to the crisis caused by the pandemic in 2020. The results obtained show four patterns of performance in the period under analysis, strengthening the relevance of the resilience, the access to financing, to have an strong and experienced management, an innovative internal production process and the capacity to introduce new strategies to overcome challenges for achieving success. Conversely, youngest firms, with less employees, weaknesses in financial availability, relatively poor management, problems in the internal production process and less resilience exhibit the poorest performance, despite the minimal impact of external factors. This outcome highlights the importance of internal factors and resilience in either attaining success or avoiding failure. It also suggests that Self-Organizing Maps can serve as a valuable tool for visualizing the characteristics of successful pathways, providing early warnings for firms, aiding experts in diagnosing their performance, and for identifying more vulnerable groups for the development of public assistance programs.
While it is widely known that the effects of the pandemic were initially negative in general terms, those cases where firms’ management has experience and resilience to market changes have succeeded in mitigating them to some extent. Clearly, having a management team with experience, training, and a certain level of resilience go hand in hand with results obtain by more established firms with strengths in innovation and quality of the product or services offered, ensuring their sustainability over time.
Limitations are acknowledged in this study, which will be considered in future lines of research based on the existence of more extensive databases, the incorporation of objective measures regarding MSME performance through financial and economic indicators to reduce the potential bias of evaluating the post-pandemic situation through expert opinion.
Disclosure statement
No potential conflict of interest was reported by the authors.
Notes
1. Copyright (C) 1999 by Esa Alhoniemi, Johan Himberg, Jukka Parviainen and Juha Vesanto. Available at: http://www.cis.hut.fi/projects/somtoolbox/
References
- Alaka, H. A., Oyedele, L. O., Owolabi, H. A., Kumar, V., Ajayi, S. O., Akinade, O. O., & Bilal, M. (2018). Systematic review of bankruptcy prediction models: Towards a framework for tool selection. Expert Systems with Applications, 94, 164–13. https://doi.org/10.1016/j.eswa.2017.10.040
- Alam, P., Booth, D., Lee, K., & Thordarson, T. (2000). The use of fuzzy clustering algorithm and self-organizing neural networks for identifying potentially failing banks: An experimental study. Expert Systems with Applications, 18(3), 185–199. https://doi.org/10.1016/s0957-4174(99)00061-5
- Altman, E. I. (1968). Financial ratios, discriminant analysis and the prediction of corporate bankruptcy. The Journal of Finance, 23(4), 589–609. https://doi.org/10.2307/2978933
- Altman, E. I., Balzano, M., Giannozzi, A., & Srhoj, S. (2022). Revisiting SME default predictors: The omega score. Journal of Small Business Management, 61(6), 1–35. https://doi.org/10.1080/00472778.2022.2135718
- Argenti, J. (1976). Corporate collapse: The causes and symptoms. John Wiley and Sons.
- Argenti, J. (1983). Predicting corporate Failure. Accountants digest. Institute of Chartered accountants in England and Wales. Sumer.
- Bahrammirzaee, A. (2010). A comparative survey of artificial intelligence applications in finance: Artificial neural networks, expert systems and hybrid intelligent systems. Neural Computing and Applications, 19(8), 1165–1195. https://doi.org/10.1007/s00521-010-0362-z
- Balcaen, S., & Ooghe, H. (2006). 35 years of studies on business failure: An overview of the classic statistical methodologies and their related problems. The British Accounting Review, 38(1), 63–93. https://doi.org/10.1016/j.bar.2005.09.001
- Beaver, W. H. (1966). Financial ratios as predictors of failure. Journal of Accounting Research, 4, 71–111. https://doi.org/10.2307/2490171
- Brown, R., Rocha, A., & Cowling, M. (2020). Financing entrepreneurship in times of crisis: Exploring the impact of COVID-19 on the market for entrepreneurial finance in the United Kingdom. International Small Business Journal, 38(5), 380–390. https://doi.org/10.1177/0266242620937464
- Caballero-Morales, S. O. (2021). Innovation as recovery strategy for SMEs in emerging economies during the COVID-19 pandemic. Research in International Business and Finance, 57, 101396. https://doi.org/10.1016/j.ribaf.2021.101396
- CAME. (2021a). Informe IMAF- Emprendedores. Retrieved from https://www.redcame.org.ar/advf/documentos/2021/04/608af1c1495e7.pdf
- CB Insights. (2021). Start-up Failure Post-Mortems, Database. www.Cbinsights.com.
- Chaves-Maza, M., & Martel, E. M. F. (2020). Entrepreneurship support ways after the COVID-19 crisis. Entrepreneurship & Sustainability Issues, 8(2), 662–681. https://doi.org/10.9770/jesi.2020.8.2(40)
- Del Brio, B. M., & Serrano-Cinca, C. (1993). Self-organizing neural networks for the analysis and representation of data: Some financial cases. Neural Computing & Applications, 1(3), 193–206. https://doi.org/10.1007/bf01414948
- Du Jardin, P. (2018). Failure pattern-based ensembles applied to bankruptcy forecasting. Decision Support Systems, 107, 64–77. https://doi.org/10.1016/j.dss.2018.01.003
- Eschker, E., Gold, G., & Lane, M. D. (2017). Rural entrepreneurs: What are the best indicators of their success? Journal of Small Business and Enterprise Development, 24(2), 278–296. https://doi.org/10.1108/JSBED-07-2016-0112
- Gabás Trigo, F. (1997). Predicción de la insolvencia empresarial. En: Predicción de la Insolvencia Empresarial, Antonio Calvo-Flores Segura and Domingo García Pérez. In de Lema (Ed.), Asociación Española de Contabilidad y Administración de Empresas, AECA (pp. 11–32).
- Ghosh, B. C., Tan, W. L., Tan, T. M., & Chan, B. (2001). The key success factors, distinctive capabilities and strategic thrusts of top SMEs in Singapore. Journal of Business Research, 51(3), 209–221. https://doi.org/10.1016/s0148-2963(99)00047-8
- Grunert, J., Norden, L., & Weber, M. (2005). The role of non-financial factors in internal credit ratings. Journal of Banking and Finance, 29(2), 509–531. https://doi.org/10.1016/j.jbankfin.2004.05.017
- Halabí, C. E., & Lussier, R. N. (2014). A model for predicting small firm performance: Increasing the probability of entrepreneurial success in Chile. Journal of Small Business and Enterprise Development, 21(1), 4–25. https://doi.org/10.1108/JSBED-10-2013-0141
- Kantis, H., & Angelelli, P. (2020). Los ecosistemas de emprendimiento de América Latina y el Caribe frente al Covid 19. Impacto, necesidades y recomendaciones, BID. Retrieved from https://publications.iadb.org/publications/spanish/document/Los-ecosistemas-de-emprendimiento-de-America-Latina-y-el-Caribe-frente-al-COVID-19-Impactos-necesidades-y-recomendaciones.pdf
- Khelil, N. (2016). The many faces of entrepreneurial failure: Insights from an empirical taxonomy. Journal of Business Venturing, 31(1), 72–94. https://doi.org/10.1016/j.jbusvent.2015.08.001
- Kim, H., Cho, H., & Ryu, D. (2020). Corporate default predictions using machine learning: Literature review. Sustainability, 12(16), 6325. https://doi.org/10.3390/su12166325
- Kücher, A., Mayr, S., Mitter, C., Duller, C., & Feldbauer-Durstmüller, B. (2020). Firm age dynamics and causes of corporate bankruptcy: Age dependent explanations for business failure. Review of Managerial Science, 14(3), 633–661. https://doi.org/10.1007/s11846-018-0303-2
- Kumar, P. R., & Ravi, V. (2007). Bankruptcy prediction in banks and firms via statistical and intelligent techniques: A review. European Journal of Operational Research, 180(1), 1–28. https://doi.org/10.1016/j.ejor.2006.08.043
- Kuzmisin, P., & Kuzmisinova, V. (2017). Small and medium-sized enterprises in global value chains. Economic Annals-XXI, 162(11–12), 22–27. https://doi.org/10.21003/ea.V162-05
- Länsiluoto, A., Eklund, T., Back, B., Vanharanta, H., & Visa, A. (2004). Industry‐specific cycles and companies’ financial performance comparison using self‐organizing maps. Benchmarking: An International Journal, 11(3), 267–286. https://doi.org/10.1108/14635770410538754
- Lanzarini, L. C., Monte, A. V., Bariviera, A. F., & Santana, P. J. (2017). Simplifying credit scoring rules using LVQ+ PSO. Kybernetes, 46(1), 8–16. https://doi.org/10.1108/K-06-2016-0158
- Lee, K., Booth, D., & Alam, P. (2005). A comparison of supervised and unsupervised neural networks in predicting bankruptcy of Korean firms. Expert Systems with Applications, 29(1), 1–16. https://doi.org/10.1016/j.eswa.2005.01.004
- Liguori, E. W., & Pittz, T. G. (2020). Strategies for small business: Surviving and thriving in the era of COVID-19. Journal of the International Council for Small Business, 1(2), 106–110. https://doi.org/10.1080/26437015.2020.1779538
- Lussier, R. N. (1995). A non-financial business success versus failure prediction model for young firms. Journal of Small Business Management, 33(1), 8–20.
- Madrid, A., & García, D. (2006). Factores que explican el fracaso empresarial en la PyME. Gestión: Revista de Economía, 36, 5–9. Retrieved from.https://dialnet.unirioja.es/servlet/articulo?codigo=2055435
- McGahan, A., & Porter, M. (1997). How much does industry matter, really? Strategic Management Journal, 18, 15–30. https://www.jstor.org/stable/3088208
- Meahjohn, I., & Persad, P. (2020). The impact of COVID-19 on entrepreneurship globally. Journal of Economics and Business, 3(3), 1165–1173. https://doi.org/10.31014/aior.1992.03.03.272
- Ooghe, H., & De Prijcker, S. (2008). Failure processes and causes of company bankruptcy: A typology. Management Decision, 46(2), 223–242. https://doi.org/10.1108/00251740810854131
- Park, Y. S., Céréghino, R., Compin, A., & Lek, S. (2003). Applications of artificial neural networks for patterning and predicting aquatic insect species richness in running waters. Ecological Modelling, 160(3), 265–280. https://doi.org/10.1016/S0304-3800(02)00258-2
- Portuguez Castro, M., & Gómez Zermeño, M. G. (2021). Being an entrepreneur post-COVID-19 – resilience in times of crisis: A systematic literature review. Journal of Entrepreneurship in Emerging Economies, 13(4), 721–746. https://doi.org/10.1108/JEEE-07-2020-0246
- Rhaiem, K., & Halilem, N. (2023). The worst is not to fail, but to fail to learn from failure: A multi-method empirical validation of learning from innovation failure. Technological Forecasting & Social Change, 190, 122427. https://doi.org/10.1016/j.techfore.2023.122427
- Rumelt, R. (1997). How much does industry matter? Strategic Management Journal, 12(3), 167–185. https://doi.org/10.1002/smj.4250120302
- Sanhokwe, H. (2022). Reimagining the SME failure phenomenon: Incorporating regenerative effects and predicting enterprise regenerative capacity. Journal of Entrepreneurship in Emerging Economies. https://doi.org/10.1108/JEEE-01-2022-0010
- Scarlat, E., & Delcea, C. (2011). Complete analysis of bankruptcy syndrome using grey systems theory. Grey Systems: Theory and Application, 1(1), 19–32. https://doi.org/10.1108/20439371111106704
- Scherger, V., Terceño, A., & Vigier, H. (2019). A systematic overview of the prediction of business failure. International Journal of Technology, Policy and Management, 19(2), 196–211. https://doi.org/10.1504/IJTPM.2019.100601
- Scherger, V., Terceño, A., Vigier, H., & Barberá-Mariné, M. G. (2015). Detection and assessment of causes in business diagnosis. Economic Computation and Economic Cybernetics Studies and Research, 49(4), 211–229. http://www.ecocyb.ase.ro/nr20154/13%20-%20Scherger%20Valeria.pdf
- Scherger, V., Vigier, H., & Barberá-Mariné, G. (2014). Finding business failure reasons through a fuzzy model of diagnosis. Fuzzy Economic Review, 19(1), 45–62. https://EconPapers.repec.org/RePEc:fzy:fuzeco:v:xix:y:2014
- Serrano-Cinca, C. (1996). Self-organizing neural networks for financial diagnosis. Decision Support Systems, 17(3), 227–238. https://doi.org/10.1016/0167-9236(95)00033-x
- Shi, Y., & Li, X. (2019). An overview of bankruptcy prediction models for corporate firms: A systematic literature review. Intangible Capital, 15(2), 114–127. https://doi.org/10.3926/ic.1354
- Singh, P., & Kaur, C. (2019). Factors determining financial constraint of SMEs: A study of unorganized manufacturing enterprises in India. Journal of Small Business & Entrepreneurship, 33(3), 269–287. https://doi.org/10.1080/08276331.2019.1641662
- Sun, J., Li, H., Huang, Q. H., & He, K. Y. (2014). Predicting financial distress and corporate failure: A review from the state of the art definitions, modeling, sampling, and featuring approaches. Knowledge-Based Systems, 57, 41–56. https://doi.org/10.1016/j.knosys.2013.12.006
- Terceño, A., Vigier, H., & Scherger, V. (2018). Prediction of business failure with fuzzy models. International Journal of Uncertainty, Fuzziness & Knowledge-Based Systems, 26(1), 21–38. https://doi.org/10.1142/S0218488518400020
- Theng, L. G., & Lim, J. W. B. (1996). An exploratory study of factors affecting the failure of local small and medium enterprises. Asia Pacific Journal of Management, 13(2), 47–61. https://doi.org/10.1007/BF01733816
- Tseng, F. M., & Hu, Y. C. (2010). Comparing four bankruptcy prediction models: Logit, quadratic interval logit, neural and fuzzy neural networks. Expert Systems with Applications, 37(3), 1846–1853. https://doi.org/10.1016/j.eswa.2009.07.081
- Vesanto, J. (2000). Neural network tool for data mining: SOM toolbox. In Proceedings of symposium on tool environments and development methods for intelligent systems, Oulu, Finland (pp.184–196).
- Wenzel, M., Stanske, S., & Lieberman, M. B. (2020). Strategic responses to crisis. Strategic Management Journal, 41, 7–18. https://doi.org/10.1002/smj.3161
- Zimmermann, H. (1991). Fuzzy set theory and its Applications. Kluwer Academic Publishers. https://doi.org/10.1007/978-94-015-7949-0