Abstract
This study aims to fill the gap by identifying factors and their effects on job seekers’ satisfaction with existing e-recruitment systems. A longitudinal cohort research design was employed for the study. Semi-structured interviews and a survey questionnaire were used as research instruments. Two hundred sixty-five job seekers (265) and seven human resource staff were purposively sampled for the study. The human resource staff answered the interview questions while the job seekers responded to the survey questionnaire. The finding indicates that factors affecting job seekers’ satisfaction with the e-recruitment systems are expectation, performance, perceived e-recruitment fraud, and e-recruitment brand quality. More so, the finding reveals that expectation, perceived performance, and confirmation are positively associated with the satisfaction of e-recruitment systems. On the contrary, perceived e-recruitment fraud is negatively associated with confirmation and consequently affects job seekers’ e-recruitment satisfaction. Surprisingly, another finding shows that jobseekers are dissatisfied with the existing e-recruitment because their expectations and perceived e-recruitment fraud are higher than the perceived performance of the existing e-recruitment systems. These findings have several implications for policy and recommendations for various levels of stakeholders focusing on e-recruitment systems. Detailed results of theoretical contribution and policy implications are discussed.
1. Introduction
One of the human resource managerial responsibilities is to recruit suitable job seekers to fill vacant or newly created jobs. Recruitment is reported in the literature as the process of analysing the job requirements, pooling together a network of qualified candidates and hiring the most suitable person for a specific role (Abbasi et al., Citation2022; El Maohub et al., Citation2022, Citation2022; Lepistö & Ihantola, Citation2018; Vetráková et al., Citation2018). Thus, the recruitment process is used to search for the services of capable job seekers with the required knowledge and expertise. Among other HRM practices, recruitment is an imperative part of their functions to draw human assets into the organisation. No matter the organisation, using the proper recruitment process to hire job seekers for various positions is necessary for competitive advantage in today’s emerging markets. Attracting the best job seekers is crucial for guaranteeing the practical function of an organisation (Sarpong, Citation2019). Thus, the wrong recruitment process will likely negatively affect the search for suitable job seekers.
E-recruitment practices significantly influence job seekers’ attraction and contribute to recruitment outcomes (Baum & Kabst, Citation2014; Kucherov & Tsybova, Citation2021). Some studies have indicated that e-recruitment systems are more effective when compared with the traditional recruitment process as the system provides organisations with sizeable geographic coverage and saves effort, time, and cost of searching for potential jobseekers (Javed et al., Citation2017; Sills, Citation2014; Singh et al., Citation2010). However, job seekers continue to apply for jobs using the traditional recruitment process, where paper-based applications and resumes are physically sent to the organisation (Masarova & Gullerova, Citation2020). This may be possible if the intended jobseekers are not satisfied with the performance of the e-recruitment systems.
Most recent e-recruitment studies have been conducted from the management or employers’ perspective (Abia & Brown, Citation2020; Kucherov & Tsybova, Citation2021; Mishra & Kumar, Citation2019; Rahman et al., Citation2020). Although the employers’ views are highly significant, the viewpoints of the job seekers who use e-recruitment frequently are missed in the literature. A study from the perspective of job seekers could provide firm empirical evidence on factors related to the e-recruitment context in an organisation and the effects of these factors on e-recruitment satisfaction. Up to now, studies from the perspective of job seekers to establish the satisfaction of the current e-recruitment systems are missing in the literature. Thus, there are limited studies on jobseekers’ perceptions of e-recruitment (Baum & Kabst, Citation2014, Carpentier et al., Citation2017; Monica, Citation2020). This study aims to fill the gap by identifying the factors associated with existing e-recruitment satisfaction and examining the effect of these factors on existing e-recruitment satisfaction. The objective of the study is to:
Identify factors that affect job seeker’s satisfaction with the existing e-recruitment systems.
Investigate the effect of the identified factors in research question 1 on e-recruitment satisfaction among job seekers.
Examine whether job seekers are satisfied or dissatisfied with the e-recruitment system, considering the effect of expectation and perceived performance.
Examine whether job seekers are satisfied or dissatisfied with the existing e-recruitment system, considering the effect of perceived e-recruitment fraud activities and perceived performance.
The findings of these twofold studies have significant implications for policymakers and designers of e-recruiters of organisations. First, the study benefits human resource management with guidelines and planning for implementing prudent policies to improve job seekers’ satisfaction with e-recruitment systems. Second, the study provides findings that enable the policymakers for e-recruitment systems to rethink the measures and features to put in place to make the system more accessible, user-friendly and secure from fraudulent activities to encourage job seekers to use it for job applications.
The rest of the study is organised into four sections. The first section presents the theoretical framework supporting the study, the development of the hypotheses and the proposed conceptual framework. The second section provides a comprehensive account of the research methodology used in carrying out the research. The third section involves the results and discussion related to the objectives. Finally, the last part of the study comprises the conclusion, theoretical contribution and policy implication.
2. Literature Review
2.1. Theoretical framework: expectancy confirmation theory
The Expectancy Confirmation Theory (ECT), also known as the Expectancy Disconfirmation Theory (EDT), was introduced first by Oliver to study customer satisfaction (Jin et al., Citation2013). The theory has been accepted as a paradigm for explaining and determining users’ satisfaction or dissatisfaction (Cai & Chi, Citation2021; Lee et al., Citation2022a, Citation2022b; Pizam & Milman, Citation1993; Zhang et al., Citation2022). The entire process by which clients form satisfaction or dissatisfaction in an ECT framework is presented in Figure . The theory posits that client satisfaction or dissatisfaction results from comparing performance (of a product or service) with pre-established standards of expectation. From the theory, the pre-established standards are the client’s projective expectations, with three likely results from the comparison. The first result is positive confirmation. This arises if performance is perceived to be higher than the pre-established expectations, then the result indicates clients’ satisfaction with the products or services. The second result, which is zero confirmation, occurs if the pre-established expectation is precisely equal to the perceived performance, then the clients will likely be satisfied. Finally, the third aspect, negative confirmation, happens when the pre-established expectation is higher than the perceived performance, and then the clients are dissatisfied with the services or products. Therefore, positive confirmation leads to a satisfied or happy customer, while negative confirmation results in dissatisfied or unhappy clients.
Figure 1. Expectancy disconfirmation/confirmation theory (Oliver, Citation1980).
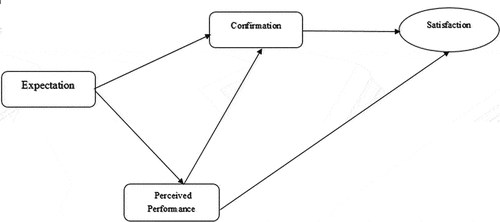
2.2. Job recruitment satisfaction and dissatisfaction
The level of job recruitment satisfaction can be defined as the level of contentment or fulfilment of jobseekers and employers’ experience during the recruitment process (Muduli & Trivedi, Citation2020). The recruitment process is measured according to how well it meets both parties’ expectations and needs. Organisations’ ability to attract and retain talent can significantly affect their satisfaction with the recruitment process (Acikgoz, Citation2019). Jobseekers who are satisfied with their jobs are more likely to accept offers and stay with the company, while satisfied employers are more likely to find suitable candidates (Carballo‐Penela et al., Citation2023) The recruitment process can be improved by focusing on effective communication, transparency, and mutual respect between candidates and employers (Asanov & Mavlikeeva, Citation2023). However, jobseekers may experience dissatisfaction in the recruitment process if representatives are rude, communication is unprofessional, or interview logistics are disorganized (Lashkari & Cheng, Citation2023). Again, dissatisfaction can result if job seekers feel they are subjected to bias or discrimination during recruitment.
2.3. Electronic recruitment system
The Electronic Recruitment System (ERS), or the Applicant Tracking System (ATS), is an application platform that facilitates and streamlines a company’s recruitment and hiring processes (Gusain et al., Citation2023). By managing and automating the process, employers can use it to find, attract, assess, and hire candidates. An e-recruitment system provides employers with valuable data and analytics that can be used to make data-driven decisions (Van Esch et al., Citation2019). E-recruitment methods track recruiting metrics, assess sourcing channels, and identify improvements (Koechling et al., Citation2023). To optimise recruitment processes, organisations must use e-recruitment systems (Black & van Esch, Citation2020). Both employers and job seekers can benefit from them in various ways, including efficiency, cost-effectiveness, improvement in communication, and improved decision-making based on data. Despite many advantages, electronic recruitment systems can also have disadvantages and drawbacks. This may include a dehumanised hiring process, making it difficult for organisations to examine jobseekers’ soft skills, personalities, and culture (Okolie & Irabor, Citation2017).
2.4. Jobseekers
Jobseekers are people enthusiastically looking for employment for a new job or a career change. They search for employment opportunities, prepare application materials (such as resumes and cover letters), and attend interviews or job fairs to find a job (Carballo‐Penela et al., Citation2023). The job seekers may be recent graduates, experienced experts looking for a career change, or people currently unemployed and seeking work and engaging in several activities (Choi et al., Citation2023). Two distinct jobseekers, passive and active, always look for employment. Individuals actively searching for new employment opportunities are considered active job seekers (Subbarao et al., Citation2022). There may be a current unemployment problem or they may be dissatisfied with their current job and actively searching for a new position. There are various job search strategies that active job seekers use, such as applying for job openings, networking, attending job fairs, and using job boards and career sites online. In most cases, they invest significant time and effort into finding a new job and are more motivated and committed (Mowbray & Hall, Citation2020). A passive job seeker is not actively seeking a new job but is open to considering new opportunities if they arise. While they might be employed and content with their current position, recruiters can connect them with potential jobs or offer them a chance to explore opportunities (Subbarao et al., Citation2022). Jobseekers who are passive may not actively look for jobs or apply for them, but they may regularly update their LinkedIn profiles and occasionally respond to recruiter inquiries. Their qualifications and experience often make them attractive to recruiters in the job market.
2.5. Hypothesis development
2.5.1. The association between confirmation, perceived E-recruitment fraud and E-recruitment brand quality, and satisfaction
From the perspective of this study, perceived e-recruitment fraud refers to jobseekers’ perception or belief that falsified activities or unscrupulous practices occur during the online recruitment process. In recent years, job seekers have encountered fake job ads featuring high wages, flexible hours, telework, and career growth opportunities (Vidros et al., Citation2016). These fraud activities are presented to job seekers through job advertising, spam conversations, or text, which attempt to collect innocent jobseekers’ information messages (Mahbub et al., Citation2022). Jobseekers who have experienced perceived e-recruitment fraud would likely spread their experience through the online platform or word-of-mouth to tarnish the organisation’s e-recruitment brand. When job seekers e-recruitment fraud (potentially deceptive or fraudulent practices in online recruitment), it negatively impacts their perception of the organisation’s e-recruitment branding efforts (Niharika Reddy et al., Citation2018). More so, jobseekers will likely not confirm the e-recruitment system if they experience fraudulent activities. However, e-recruitment branding quality may play a crucial role in attracting and promoting job seekers (Wijaya et al., Citation2023). Moreover, online recruitment branding will likely affect the user’s satisfaction (Hsieh et al., Citation2022). Hence, the following hypotheses were derived:
H1:
Perceived e-recruitment fraud is negatively associated with e-recruitment branding quality
H2:
Perceived e-recruitment fraud is negatively associated with jobseeker confirmation
H3:
E-recruitment branding quality is positively associated with jobseeker e-recruitment satisfaction.
2.5.2. The relationship between expectation, perceived performance, confirmation and E-recruitment satisfaction
The ECT postulates that there are associations between expectation, performance, confirmation and satisfaction. The associations have been verifiable and supported in previous empirical studies from different perspectives, such as Wearable health information systems (Shen et al., Citation2018), policy and online repurchase intention (Wang et al., Citation2019), Predicting visitors’ satisfaction with their destination (Pizam & Milman, Citation1993) and citizen satisfaction (Lee et al., Citation2022a). In consequence, it is expected that there are relationships between expectation, perceived performance, confirmation and e-recruitment satisfaction among job seekers. This leads to the following hypotheses.
H4:
Expectation is positively associated with job seekers’ confirmation
H5:
Expectation is positively associated with e-recruitment perceived performance
H6:
perceived performance is positively associated with job seekers’ confirmation
H7:
perceived performance is positively associated with job seekers’ e-recruitment satisfaction
H8:
Confirmation is positively associated with jobseeker e-recruitment satisfaction
2.5.3. Determination of E-recruitment satisfaction based on expectation and perceived performance from ECT
From ECT, satisfaction or dissatisfaction is determined by the gap between expectation and perceived performance. In the same scenario, this presented study suggests that job seekers’ satisfaction or dissatisfaction will be predicted by the difference between their expectations and perceived performance of e-recruitment. Hence, the following null and alternative hypotheses were proposed:
HO: Comparing the effect of expectation and perceived performance on the confirmation, job seekers are satisfied with e-recruitment systems
Ha: Comparing the impact of expectation and perceived performance on the confirmation, job seekers are dissatisfied with e-recruitment systems.
2.5.4. Determination of E-recruitment satisfaction based on perceived performance and perceived e-recruitment fraud
Fraud activities can negatively affect job seekers’ satisfaction with e-recruitment systems. The performance of the e-recruitment system and fraud activities are likely to affect confirmation and consequently generate satisfaction or dissatisfaction with the system. Therefore, this current study theoretically suggests that when perceived e-recruitment fraud is superior or higher than the system’s perceived performance, job seekers are dissatisfied with e-recruitment systems. On the other hand, if perceived e-recruitment fraud is inferior or lesser than the perceived performance, job seekers are satisfied with the e-recruitment systems. However, the study anticipates job seekers are unsatisfied due to perceived e-recruitment fraud activities. Per this conclusion, the following null and alternative hypotheses were proposed:
HO: Comparing the effects of expectation and perceived e-recruitment fraud on the confirmation, jobseekers are satisfied with e-recruitment systems.
Ha: Comparing the effects of expectation and perceived e-recruitment fraud on the confirmation, jobseekers are dissatisfied with e-recruitment systems.
2.6. Proposed research conceptual framework
The ECT indicates how individual expectations and experiences relate to their overall satisfaction (Rathjens et al., Citation2023; Tan et al., Citation2023; Wen et al., Citation2023; Ye et al., Citation2023). The theory focuses on the central idea that individuals have pre-existing expectations about certain events or experiences (Lee, Hung, & Chen, Citation2022a). When these expectations are not fulfilled, they react in specific ways (Abdelkarim, Citation2022). From the ECT, it is conceptualised that jobseekers evaluate their e-recruitment experience compared to their initial expectations. The study proposed that if the e-recruitment process meets or exceeds their expectations, it leads to confirmation, which results in satisfaction. If the job seeker encounters a complicated application process that falls short of their expectations, it results in disconfirmation, which leads to dissatisfaction. Perceived e-recruitment fraud could naturally lead to dissatisfaction and negative emotional responses involving anger, disappointment, and betrayal, resulting in negative disconfirmation. More so, the study extended ECT by establishing that perceived e-recruitment fraud and e-recruitment brand quality may influence jobseekers’ satisfaction, as presented in Figure . The augment is that fraud can wear down trust and credibility in online activities (Bera et al., Citation2023), while system brand quality can enhance reputation and trustworthiness, which may lead to trust, confidence and satisfaction (Mtotywa & Kekana, Citation2023). Table reports the operational definition of constructs or latent variables used in the study.
Table 1. Summary explanation of research model constructs
3. Research methodology
A longitudinal cohort research design with both quantitative and qualitative data (mixed method) collection approaches was used in the study. Research designs involving longitudinal cohorts follow groups of individuals (cohorts) over extended periods, usually with multiple data points or assessments (Askelund et al., Citation2023; Menard, Citation2002). This study was conducted over time, with data collected at multiple points in time and various measurement assessments (semi-structured interviews and survey questionnaires). The current study purposively selected Jobseekers and human resources personnel in Greater Accra and the Ashanti Region of Ghana because of the area’s highest unemployment (jobseekers) rate. More so, jobseekers were selected for this study because they could offer feedback on the features and functionalities of the e-recruitment system, for example, the application process, resume submission, and communication with employers. Also, human resources personnel were selected because they are responsible for recruiting and selecting jobseekers and use the recruitment systems in the organisation. In justification of sample size, Cattell (Citation1978) reported that using a minimum sample size of 250 in research involving factor analysis is suitable. In another view, Guilford et al. (Citation1954) suggested that 200 participants of sample size are acceptable. Also, Alreck and Settle (Citation1985) indicated that between 250 and 1,000 participants is the recommended sample size to use in a population of 10,000 and above. In addition, Gorsuch (Citation1983) and Kline (Citation1994) supported at least 100 participants as a sample size. Therefore, the study employed 265 Job seekers and seven (7) human resource personnel.
3.1. Development of research instrument
Semi-structured interviews and survey questionnaires were employed to collect data from the participants. The Semi-structured Interview questions and survey questionnaires were adopted from literature studies. The detail of the field notes was done using recording devices and was transcribed to enhance the reliability of semi-structured interviews. Also, for trustworthiness, there was a long engagement in the field. More so, the survey questionnaire involves two parts to investigate the formulated research objectives with its associated research hypothesis. The first part of the survey questionnaire focuses on the participants’ demographics, including gender, age, educational background and years of unemployment. The other part involves six (6) latent variables and twenty-five (25) observed variables, which were adopted from previous empirical studies (Bhattacherjee, Citation2001; Jin et al., Citation2013; Shiau et al., Citation2020, Román, 2010; García et al., Citation2018). The study used a five-Likert scale with a rating range from Strongly Disagree (SD = 1), Disagree (D = 2), Undecided (U = 3), Agree (A = 4) and Strongly Agree (SA = 5).
A pilot study was conducted to test the reliability of the survey questionnaire using Cronbach’s alpha. This was done to find out not only the internal consistency of the survey questionnaire but also to check errors in the statement of observed variables, such as spelling mistakes and omission of letters or words. The present study piloted the instrument with thirty-five job seekers of different participants. The Cronbach Alpha was 0.796, which indicates good internal consistency. For good internal consistency, Henseler et al. (Citation2009) suggested Cronbach’s Alpha of 0.80, Taber (Citation2018) recommended 0.70 or more and Hair et al. (Citation2014) and Nunnally (Citation1978) reported 0.90 or above being the excellent value. Therefore, from Table , the value of Cronbach’s Alpha ranges from 0.860 to 0.941, suggesting good and excellent internal consistency of the collected data from the participants.
Table 2. Result of reliability analysis of survey questionnaire
3.2. 3.2. Validity of the research survey questionnaire
3.2.1. Convergent validity
Table presents the summary of the exploratory factor analysis (EFA), the average variance extracted (AVEs) ranged from 0.629 to 0.752. However, the recommended value for Factor loading should be greater than 0.6 (Bagozzi, Citation1981), the AVEs should be more than 0.5 (Chin, Citation1998; Hair et al., Citation2014) and composite reliability should exceed 0.7 (Henseler et al., Citation2009). Therefore, similar questionnaire items produce the same results suggested by Fornell and Larcker (Citation1981).
Table 3. Summary of exploratory factor analysis (EFA)
3.2.2. Discriminant validity
In finding discriminant validity, Fornell and Larcker (Citation1981) suggested that the square root of AVEs of the latent variables should be more than the correlated value of other latent variables for acceptance of discriminant validity. As reported in Table , the value of the square root of AVEs is more than its corresponding correlated values of other latent variables. Therefore, the latent variables that theoretically should not be related to each other are indeed not related.
Table 4. Discriminant analysis of factors
More so, Common method bias was checked using Variance Inflation Factor (VIF) and tolerance. VIF ranged from 1.009 to 1.178 and tolerance ranged from 0.849 to 0.992, which falls within the expected value threshold (Kock, Citation2015; O’brien, Citation2007).
3.3. Normality testing of the data
Skewness, Kurtosis, Bartlett’s test of sphericity and Kaiser—Meyer–Olkin (KMO) were used to examine the normality of the data collected from the participants of the study. Lei and Lomax (Citation2005) recommended that both the skewness and Kurtosis values should be less than |2.3|, while Brown (Citation2006) suggested that the acceptable values of skewness should be |3| and |10| for kurtosis when applying studies involving structural equation modelling (SEM). As reported in Table , the value of skewness ranges from −1.705 to −1.004 and kurtosis ranges from 0.534 to 2.517, which falls in the threshold. More so, simple adequacy was investigated. The result suggested significant statistics of χ2 (496) = 5910.409 (p = 0.00 < 0.05) and the KMO measure = 0.824 > 0.500. This means that the collected data from the participants are normally distributed for confirmation factor analysis to continue.
3.4. Data collection procedure
The survey questionnaire was purposively distributed to the participants (jobseekers) in person. The participants were asked to use any specific time of their choice to complete the questionnaire. Three hundred (300) were distributed to the participants. Out of that, two hundred and seventy-six (276) representing 92% were returned. Only 265 (88%) were fully completed. More so, the seven (7) human resources personnel working with e-recruitment systems were purposively selected to answer semi-structured interviews. The data of the participants were collected from 11 June 2022 to 4 December 2022. The duration of the data collection was determined based on the research objectives and the nature of the study.
3.4.1. Ethical consideration
The aim and objectives of the study were explained to each of the participants. The participants were asked to participate freely or could opt out or choose not to participate in the study. Moreover, to ensure anonymity in the research, the collection of data from the participants was free from identifying information of the individual participants such as name, e-mail address, and postal address. The participants were given assurance of confidentiality of data collected from them.
3.5. Data analysis of the instrument
During the semi-structured interview, a recording device was used to record the conversation of the interview. The recorded data was transcribed according to the individual responses. NVivo software was employed to analyse the transcripts into codes and consequently into themes. For the questionnaire, the study used two significant statistical stools, SPSS and LISREL.
3.6. Model goodness fit measurement
In the examination of the proposed model fit, the fit index (p-value = 0.005, chi-square = 59.35, df = 28, GFI = 0.960, AGFI = 0.921, IFI = 0.905, RMSEA = 0.065, CFI = 0.900, RMR = 0.634 and standardised RMR = 0.0626) fall within the threshold (Hu & Bentler, Citation1999; Kline, Citation2005; Lomax, Citation1982; Steiger, Citation2007; Tabachnick & Fidell, Citation2007) as presented in Table . Therefore, the proposed model has a good model fit
Table 5. Model indices of goodness of fit
4. Results and discussion
4.1. Demography of the participants
4.1.1. Demography of the job seekers
Out of 265 who were involved in this study, 55.1% (n = 146) were females and 44.9% (n = 119) were males as reported in Table . Also, out of 265 participants, 16.6% (n = 44), 50.9% (n = 135), 18.9% (n = 50) and 13.6% (n = 36) are below 24 years, between 24–34 years, 35–45 years and above 45 years respectively. Moreover, the results indicate that 4.5% (n = 12), 22.6% (n = 60), 62.3% (n = 165), 9.1% (n = 24) and 1.5% (n = 4) of the participants have completed senior high school or below, Diploma, first degree, second degree and Doctoral degree respectively. Also, 48.3% (n = 128), 36.7% (n = 97), 10.6% (n = 28), 3.7% (n = 10) and 0.7% (n = 2) of the participants reported that they have been searching for a job below 2 years, between 2–4 years, 5–7 years, 8–10 years and above 10 years accordingly.
Table 6. Demographic profile of the respondents
4.1.2. Demography of human resource personnel participants
As reported in Table , out of 7 participants for the semi-structured interview, 57.1% (n = 4) were females and 42.9% (n = 3). Further, 14.3% (n = 1) each were between the ages of 35–45 and below 24. Also, 42.8% (n = 3) of them are between 24–34 years. Again, 28.6% (n = 3) were above 45 years. The participants were asked about their educational background. None have a High school certificate or below. More so, 28.6% (n = 2) and 71.4% (n = 5) have Professional certificates and First/second/third degrees respectively. Out of the 7, 14.3% (n = 1), 28.6% (n = 2) and 57.1% (n = 5) have worked below 2 years between 2–4 years and above 5 years respectively
Table 7. Human resource personnel participant Demography
4.2. Results and discussions
For research objective 1, the results from the semi-structured interview suggested the following themes were generated as the factors that influence job seekers’ satisfaction with e-recruitment systems for their job applications.
4.2.1. Expectations of the E-recruitment system
Human resource professional working with e-recruitment (HRPEC) 1 who has worked for the past three years indicated that: in fact, I have several expectations about the use of e-recruitment system for job application., however, the most important one is the content and the instruction displayed by the system. The e-recruitment system should have clear, readable and understandable content and directions to complete the job application (24 June 2022). This is supported by Yoon Kin Tong (Citation2009) who has high expectations for e-recruitment. HRPEC2 who is the manager of the e-recruitment system also reported that: She is expected the e-recruiter should have clear, direct, straightforward and free communication with the job seekers. Most importantly, not having feedback from the system will produce undesirable feelings and a possible loss of interest in the job opportunity (25 June 2022). In support, Yoon Kin Tong (Citation2009) indicated that it is expected that the e‐recruitment system and services will improve to attract these “passive” talented groups of candidates for employment.
4.2.2. Performance of E-recruitment system
HRPEC 7 who is the monitoring and evaluation officer of the e-recruitment system, indicated that: the e-recruitment system saves time and processes the application process faster. I also believe that the e-recruitment system leads to a reduction in human efforts (26 August 2022). In agreement, Kucherov and Tsybova (Citation2021) reported that the performance of the e-recruitment system is extremely significant in the search for job seekers. HRPEC 2 reported that the e-recruitment system is now fashionable among recruiters and job seekers because of its performance in minimising the costs of buying a newspaper in search of a job, travelling expenses for interviews, and posting relevant documents to the organisation (25 June 2022). More so, HRPEC 3, who holds an HRM master’s degree reported that: the use of an e-recruitment system in performing recruitment activities is essential for job seekers. HRPEC 5 continues to say that using e-recruitment for job applications is just a click away. HRPEC 4 also indicated that several Job portals and e-recruitment systems assist in finding and processing your document for vacant positions (10 July 2022).
4.2.3. E-recruitment brand quality
HRPEC 6, who just completed his Doctorate in business administration and is now in charge implementation of a human resource information system in his organisation said: I am very particular about the promotion of the e-recruitment system brand quality which may encourage qualified job seekers to apply for a job vacancy. HRPEC 4 is a first-degree holder in business management information systems and working in the human resources department reported that her preference for using an e-recruitment system is based on the branding of e-recruitment by the company requesting applicants for a job. Without this, I will not trust the system and will find it difficult to submit my documents for that job (10 July 2022). HRPEC 1 also stated that the company’s e-recruitment system should be branded in a way that the jobseekers will be able to search for information and preview the advert of the job, submit curriculum vitae and fill out application forms (24 June 2022). This finding is inconsistent with the study conducted by,Lievens et al. (Citation2002), where it was revealed that the branding of the organisational page for recruitment could support candidates in searching for a job, submitting curriculum vitae and filling out application forms.
4.2.4. Perceived e-recruitment fraud
HRPEC 3 points out that job seekers should be very careful when using the e-recruitment system for job applications because of the increase in perceived e-recruitment fraud. I have experienced one when a fraudster offered me fake job opportunities by listing jobs that do not exist, expecting to obtain my money and personal data. HRPEC 6 in response to the interview questions said that perceived e-recruitment fraud sometimes affected his decision about the system. For instance, there were several reports from unemployed friends in their search for a job, they encountered fake recruitment e-mails, SMS texts, fake websites, fraudulent job posts and e-recruitment services appealing to be from renowned and organised organisations. HRPEC 7 also testified that there are several instances where I received viruses or malware on the computer I used for e-application through attachments or links in the job posting. Those e-recruitment job descriptions are not clear and the systems do not ask for specific skills, qualifications or previous experience. In accordance, Niharika Reddy et al. (Citation2018) revealed that fraud affects the e-recruitment system and therefore, requires attention.
In conclusion, the responses from the semi-structured interview indicated that factors that influence e-recruitment satisfaction are (a) the expectation of the e-recruitment, (b) perceived performance of the system, (c) perceived e-recruitment fraud and (e) e-recruitment brand quality.
4.2.4.1. Research objective 2
In Figure and Table , the results from structural equation modelling of path coefficients analysis report that H1 is unsupported. The perceived e-recruitment fraud (β = 0.028, t = −0.535, p = 0.559) is not negatively associated with the e-recruitment brand quality. On the contrary, some studies (Apte et al., Citation2019; Fracarolli Nunes & Lee Park, Citation2021; Soon et al., Citation2019; Viosca et al., Citation2004) indicated that fraud activity could affect the brand reputation of an organisation. Surprisingly, H2 is supported. Thus, the perceived e-recruitment fraud activity (β = 0.189, t = 3.795, p = 0.000) is negatively associated with job seekers’ confirmation. In support of the findings, Vidros et al. (Citation2016) reported that e-recruitment systems fraud endangers job seekers’ confidentiality and damages the reputations of organisations. Also, H4 and H5 are supported. This means that jobseekers’ confirmation (β = 0.553, t = 10.698, p = 0.000) and perceived performance (β = 0.314, t = 5.309, p = 0.000) are positively associated with jobseekers’ expectations. This finding supports the ECT, which revealed that there are relationships among expectation, perceived performance and confirmation (Oliver, Citation1980). Moreover, H6 and H7 are supported. Thus, perceived performance is positively associated with job seekers’ confirmation (β = 0.177, t = 2.955, p = 0.003) and their e-recruitment satisfaction (β = 0.162, t = 2.862, p = 0.005) is influenced by perceived performance. Interestingly, H3 and H8 are supported. Thus, job seekers’ confirmation (β = 0.150, t = 2.652, p = 0.008) and e-recruitment brand quality (β = 0.533, t = 9.264, p = 0.000) are positively associated with e-recruitment satisfaction.
Table 8. Summary of the effect of coefficient path
4.2.4.2. For research objective 3
results from H4, the jobseekers’ expectation (β = 0.553) on confirmation is more than the H6, job seekers’ perceived performance (β = 0.177) on confirmation. Meaning that the expectations of job seekers exceeded the perceived performance of the existing e-recruitment system, suggesting negative confirmation. Therefore, job seekers are dissatisfied with the e-recruitment system for job applications. In other words, the perceived performance of the e-recruitment system could not attract job seekers’ satisfaction. This finding is unique and original in the literature since it seems no related studies are found in the literature.
4.2.4.3. For research objective 4
surprisingly, the results from H2, the jobseekers’ perceived e-recruitment fraud activity (β = 0.189) on confirmation exceed H5, perceived performance (β = 0.177) on confirmation. Implying that the jobseekers’ perceived e-recruitment fraud activities surpassed the perceived performance of the e-recruitment systems, suggesting negative confirmation. As a result, job seekers are dissatisfied with the e-recruitment systems because of fraudulent activities. To the best of the researcher’s knowledge, these findings are unique and novel in the literature.
5. Conclusion, theoretical contribution and policy implications
The present study presents study was based on the Expectancy Confirmation Theory (ECT). This is the first time a study has examined job seekers’ satisfaction or dissatisfaction based on ECT, particularly in the area of e-recruitment systems. More so, this present study contributes theoretically by extending ECT by adding perceived e-recruitment fraud and e-recruitment brand quality to investigate e-recruitment satisfaction. The study theoretically proposed and tested a conceptual framework that suggested job seekers are not satisfied with the e-recruitment system, considering the effect of perceived fraud activities and the performance of the system. Lastly, ECT is mostly applied to explain satisfaction with public services. Little is known about the satisfaction of technology users, particularly users of e-recruitment systems.
The findings of this research have several implications for policy and recommendations for various levels of stakeholders in the human resource department focusing on e-recruitment systems. The finding suggested that e-recruitment fraud is negatively associated with confirmation and consequently affects job seekers’ e-recruitment satisfaction. Therefore, the implementation of a fraud policy is a critical tool for the human resource department to deal with fraud-related activities towards the e-recruitment systems. This should be done by explicitly defining actions that constitute fraud. This fraud policy promotes employers’ and job seekers’ awareness to minimise the damage caused by other fraudulent occurrences. The finding of the study indicates that e-recruitment brand quality is positively associated with job seeker e-recruitment satisfaction. Hence, policymakers and regulators must increase the brand quality of their e-recruitment system to find the best possible candidates that suit their organisational needs and objectives. To keep the brand quality of e-recruitment, the policymakers and all other stakeholders should (a) be consistent with the brand quality of e-recruitment systems across all platforms, (b) make sure e-recruitment systems features look professional and meet international standards and (c) be unique and specific with high-quality to showcase the e-recruitment brand to attract potential and talented job seekers. Surprisingly, the findings indicate that job seekers are not satisfied with the existing e-recruitment systems. Therefore, there is a need for better e-recruitment systems and practices which should include consistent updates of interview schedules, organisational expectations, and timely e-recruitment link videos. Further, the stakeholders should create an in-depth profile of what the organisation needs including expert knowledge, the right competence, and the attitude required for the job. More so, the management of the e-recruitment system should ensure that the e-recruitment systems are accessible for the best or most talented job seekers to apply.
5.1. Limitations and recommendations for further studies
Despite the significant findings and empirical approach of this study, 265 job seekers and 7 human resource personnel were selected as the sample size which may limit the generalisation of the findings. However, the study employed a trend longitudinal survey which makes the findings more reliable. Therefore, further studies should consider employing other areas with a large sample size to expand the generalisation of the findings. More so, it is significant for further studies to trace the changes in job seekers’ satisfaction perceptions with the e-recruitment systems over time to the environmental changes. Finally, further studies should test how e-recruitment fraud and performance influence the expectations of job seekers.
Disclosure statement
No potential conflict of interest was reported by the author.
References
- Abbasi, S. G., Tahir, M. S., Abbas, M., & Shabbir, M. S. (2022). Examining the relationship between recruitment & selection practices and business growth: An exploratory study. Journal of Public Affairs, 22(2), e2438. https://doi.org/10.1002/pa.2486
- Abdelkarim, S. (2022). The role of economic conditions in shaping citizens’ satisfaction with government in Tunisia’s fragile democracy: An expectancy-disconfirmation analysis. International Journal of Public Administration, 1–18. https://doi.org/10.1080/01900692.2022.2069117
- Abia, M., & Brown, I. (2020). Conceptualisations of E-recruitment: A literature Review and analysis. In M. Hattingh, M. Matthee, H. Smuts, I. Pappas, Y. K. Dwivedi, & M. Mäntymäki (Eds.), Responsible design, implementation and use of information and communication technology. Springer. https://doi.org/10.1007/978-3-030-45002-1_32
- Acikgoz, Y. (2019). Employee recruitment and job search: Towards a multi-level integration. Human Resource Management Review, 29(1), 1–13. https://doi.org/10.1016/j.hrmr.2018.02.009
- Alreck, P. L., & Settle, R. B. (1985). The survey research handbook.
- Apte, M., Palshikar, G. K., & Baskaran, S. (2019). Frauds in Online Social Networks: A Review. In T. Özyer, S. Bakshi, & R. Alhajj (Eds.), Social Networks and Surveillance for Society. Lecture Notes in Social Networks. Springer. https://doi.org/10.1007/978-3-319-78256-0_1
- Asanov, I., & Mavlikeeva, M. (2023). Can group identity explain the gender gap in the recruitment process?. Industrial Relations Journal, 54(1), 95–113. https://doi.org/10.1111/irj.12392
- Askelund, A. D., Ask, H., Ystrom, E., Havdahl, A., & Hannigan, L. J. (2023). Exploring the differentiation of behavioural and emotional problems across childhood: A prospective longitudinal cohort study. Journal of Child Psychology and Psychiatry Advances, e12176. https://doi.org/10.1002/jcv2.12176
- Bagozzi, R. P. (1981). Attitudes, intentions, and behaviour: A test of some key hypotheses. Journal of Personality & Social Psychology, 41(4), 607. https://doi.org/10.1037/0022-3514.41.4.607
- Baum, M., & Kabst, R. (2014). The effectiveness of recruitment advertisements and recruitment websites: Indirect and interactive effects on applicant attraction. Human Resource Management, 53(3), 353–378. https://doi.org/10.1002/hrm.21571
- Bera, D., Ogbanufe, O., & Kim, D. J. (2023). Towards a thematic dimensional framework of online fraud: An exploration of fraudulent e-mail attack tactics and intentions. Decision Support Systems, 171, 113977. https://doi.org/10.1016/j.dss.2023.113977
- Bhattacherjee, A. (2001). Understanding information systems continuance: An expectation-confirmation model. MIS Quarterly, 25(3), 351–370. https://doi.org/10.2307/3250921
- Black, J. S., & van Esch, P. (2020). AI-enabled recruiting: What is it and how should a manager use it? Business Horizons, 63(2), 215–226. https://doi.org/10.1016/j.bushor.2019.12.001
- Brown, M. (2006). Duality of thermal regimes is the distinctive characteristic of plate tectonics since the Neoarchean. Geology, 34(11), 961–964. https://doi.org/10.1130/G22853A.1
- Cai, R., & Chi, C. G. Q. (2021). Pictures vs. reality: Roles of disconfirmation magnitude, disconfirmation sensitivity, and branding. International Journal of Hospitality Management, 98, 103040. https://doi.org/10.1016/j.ijhm.2021.103040
- Carballo‐Penela, A., Ruzo‐Sanmartín, E., & Sousa, C. M. (2023). Does business commitment to sustainability increase job seekers’ perceptions of organisational attractiveness? The role of organisational prestige and cultural masculinity. Business Strategy and the Environment, 1–15. https://doi.org/10.1002/bse.3434
- Carpentier, M., Van Hoye, G., Stockman, S., Schollaert, E., Van Theemsche, B., & Jacobs, G. (2017). Recruiting nurses through social media: Effects on employer brand and attractiveness. Journal of Advanced Nursing, 73(11), 2696–2708. https://doi.org/10.1111/jan.13336
- Cattell, R. B. (1978). Fixing the number of factors: The most practicable psychometric procedures. In The scientific use of factor analysis in behavioral and life sciences (pp. 72–91). Springer. https://doi.org/10.1007/978-1-4684-2262-7_5
- Chin, W. W. (1998). Commentary: Issues and opinion on structural equation modeling. MIS Quarterly, 22(1), 7–16. https://www.jstor.org/stable/249674
- Choi, J. H., Pacelli, J., Rennekamp, K. M., & Tomar, S. (2023). Do jobseekers value diversity information? Evidence from a field experiment and human capital disclosures. Journal of Accounting Research, 61(3), 695–735. https://doi.org/10.1111/1475-679X.12474
- El Maohub, Y., Rangelov, N., & Suggs, L. S. (2022). The best person for the job is the most qualified, right? An experimental study about islamophobia in hiring practices. Journal of Social Marketing, 13(1), 63–81. (ahead-of-print) https://doi.org/10.1108/JSOCM-07-2021-0164
- Fornell, C., & Larcker, D. F. (1981). Evaluating structural equation models with unobservable variables and measurement error. Journal of Marketing Research, 18(1), 39–50. https://doi.org/10.1177/002224378101800104
- Fracarolli Nunes, M., & Lee Park, C. (2021). Your fraud, our country: Impacts on National brands and reputations. In Business ethics and Environmental Fraud. Palgrave Macmillan, Cham. https://doi.org/10.1007/978-3-030-73800-6_9
- García, J. A. C., Galindo, A. D. V., & Suárez, R. M. (2018). The effect of online and offline experiential marketing on brand equity in the hotel sector. Spanish Journal of Marketing-ESIC, 22(1), 22–41. https://doi.org/10.1108/SJME-03-2018-003
- Gorsuch, R. L. (1983). Factor analysis. Lawrence Erlbaum Associates.
- Guilford, J. P., Christensen, P. R., Bond, N. A., Jr., & Sutton, M. A. (1954). A factor analysis study of human interests. Psychological Monographs: General & Applied, 68(4), 1. https://doi.org/10.1037/h0093666
- Gusain, A., Singh, T., Pandey, S., Pachourui, V., Singh, R., & Kumar, A. (2023, March). E-Recruitment using Artificial Intelligence as Preventive Measures. Proceedings of the 2023 International Conference on Sustainable Computing and Data Communication Systems (ICSCDS), Chicago, IL, USA (pp. 516–522). IEEE.
- Hair, J. F., Jr., Sarstedt, M., Hopkins, L., & Kuppelwieser, V. G. (2014). Partial least squares structural equation modeling (PLS-SEM): An emerging tool in business research. European Business Review. https://doi.org/10.1108/EBR-10-2013-0128
- Henseler, J., Ringle, C. M., & Sinkovics, R. R. (2009). The use of partial least squares path modeling in international marketing. In New challenges to international marketing. Emerald Group Publishing Limited. https://doi.org/10.1108/S1474-7979(2009)0000020014
- Hsieh, S. H., Lee, C. T., & Tseng, T. H. (2022). Psychological empowerment and user satisfaction: Investigating the influences of online brand community participation. Information & Management, 59(1), 103570. doi:10.1016/j.im.2021.103570
- Hu, L. T., & Bentler, P. M. (1999). Cutoff criteria for fit indexes in covariance structure analysis: Conventional criteria versus new alternatives. Structural Equation Modeling: A Multidisciplinary Journal, 6(1), 1–55. https://doi.org/10.1080/10705519909540118
- Javed, F., Hoang, P., Mahoney, T., & McNair, M. (2017, February). Large-scale occupational skills normalisation for online recruitment. Proceedings of the AAAI Conference on Artificial Intelligence, San Francisco California USA, 31, 4627–4634
- Jin, X. L., Zhou, Z., Lee, M. K., & Cheung, C. M. (2013). Why users keep answering questions in online question answering communities: A theoretical and empirical investigation. International Journal of Information Management, 33(1), 93–104. https://doi.org/10.1016/j.ijinfomgt.2012.07.007
- Kline, P. (1994). An easy guide to factor analysis. Routledge.
- Kline, R. B. (2005). Principles and practice of structural equation modeling (2nd Edition ed.). The Guilford Press.
- Kock, N. (2015). Common method bias in PLS-SEM: A full collinearity assessment approach. International Journal of E-Collaboration (IJeC), 11(4), 1–10. doi:10.4018/ijec.2015100101
- Koechling, A., Wehner, M. C., & Warkocz, J. (2023). Can I show my skills? Affective responses to artificial intelligence in the recruitment process. Review of Managerial Science, 17(6), 2109–2138. doi:10.1007/s11846-021-00514-4
- Kucherov, D., & Tsybova, V. (2021). The contribution of e-recruitment practices to e-recruitment outcomes in Russian companies. Measuring Business Excellence, 26(3), 366–377. doi:https://doi.org/10.1108/MBE-02-2021-0017
- Lashkari, M., & Cheng, J. (2023, April). “Finding the magic sauce”: Exploring perspectives of recruiters and job seekers on recruitment bias and automated tools. Proceedings of the 2023 CHI Conference on Human Factors in Computing Systems, Hamburg Germany (pp. 1–16).
- Lee, C. P., Hung, M. J., & Chen, D. Y. (2022a). Factors affecting citizen satisfaction: Examining from the perspective of the expectancy disconfirmation theory and individual differences. Asian Journal of Political Science, 30(1), 1–26. doi:10.1080/02185377.2022.2047081
- Lee, C. P., Hung, M. J., & Chen, D. Y. (2022b). Factors affecting citizen satisfaction: Examining from the perspective of the expectancy disconfirmation theory and individual differences. Asian Journal of Political Science, 30(1), 35–60. https://doi.org/10.1080/02185377.2022.2047081
- Lei, M., & Lomax, R. G. (2005). The effect of varying degrees of nonnormality in structural equation modeling. Structural Equation Modeling, 12(1), 1–27. doi:10.1207/s15328007sem1201_1
- Lepistö, L., & Ihantola, E. M. (2018). Understanding the recruitment and selection processes of management accountants: An explorative study. Qualitative Research in Accounting and Management, 15(1), 104–122. doi:https://doi.org/10.1108/QRAM-11-2016-0080
- Lievens, F., Van Dam, K., & Anderson, N. (2002). Recent trends and challenges in personnel selection. Personnel Review, 31(5), 580–601. https://doi.org/10.1108/00483480210438771
- Lomax, R. G. (1982). A guide to LISREL-type structural equation modeling. Behavior Research Methods & Instrumentation, 14(1), 1–8. https://doi.org/10.3758/BF03202105
- Mahbub, S., Pardede, E., & Kayes, A. S. M. (2022). Online Recruitment Fraud Detection: A Study on Contextual Features in Australian Job Industries. Institute of Electrical and Electronics Engineers Access, 10, 82776–82787. doi:10.1109/ACCESS.2022.3197225
- Masarova, T., & Gullerova, M. (2020). Digitisation in recruitment. Economic and Social Development: Book of Proceedings, Lisbon, Portugal (pp. 37–44).
- Menard, S. W. (2002). Longitudinal research (Vol. 76). Sage.
- Mishra, S., & Kumar, S. P. (2019). E-recruitment and training comprehensiveness: Untapped antecedents of employer branding. Industrial and Commercial Training, 51(2), 125–136. doi:10.1108/ICT-12-2017-0106
- Monica, D. B. (2020). Perception of job seekers towards innovative e-recruitment. Journal of Contemporary Issues in Business and Government, 26(2), 447–452.
- Mowbray, J., & Hall, H. (2020). Networking as an information behaviour during job search: A study of active jobseekers in the Scottish youth labour market. Journal of Documentation, 76(2), 424–439. https://doi.org/10.1108/JD-05-2019-0086
- Mtotywa, M. M., & Kekana, C. (2023). Post COVID-19 online shopping in South Africa: A mediation analysis of customer satisfaction on e-service quality and purchase intention. African Journal of Science, Technology, Innovation & Development, 15(5), 1–14. doi:10.1080/20421338.2022.2153982
- Muduli, A., & Trivedi, J. J. (2020). Recruitment methods, recruitment outcomes and information credibility and sufficiency. Benchmarking: An International Journal, 27(4), 1615–1631. doi:10.1108/BIJ-07-2019-0312
- Niharika Reddy, M., Mamatha, T., & Balaram, A. (2018, January). Analysis of e-recruitment systems and detecting e-recruitment fraud. In International Conference on Communications and Cyber Physical Engineering 2018 (pp. 411–417). Springer, Singapore.
- Nunnally, J. C. (1978). An overview of psychological measurement. Clinical Diagnosis of Mental Disorders, 97–146. https://doi.org/10.1007/978-1-4684-2490-4_4
- O’brien, R. M. (2007). A caution regarding rules of thumb for variance inflation factors. Quality & Quantity, 41(5), 673–690. https://doi.org/10.1007/s11135-006-9018-6
- Okolie, U. C., & Irabor, I. E. (2017). E-recruitment: practices, opportunities and challenges. European Journal of Business and Management, 9(11), 116–122.
- Oliver, R. L. (1980). A cognitive model of the antecedents and consequences of satisfaction decisions. Journal of Marketing Research, 17(4), 460–469. https://doi.org/10.1177/002224378001700405
- Pizam, A., & Milman, A. (1993). Predicting satisfaction among first time visitors to a destination by using the expectancy disconfirmation theory. International Journal of Hospitality Management, 12(2), 197–209. https://doi.org/10.1016/0278-4319(93)90010-7
- Rahman, M., Aydin, E., Haffar, M., & Nwagbara, U. (2020). The role of social media in e-recruitment process: Empirical evidence from developing countries in social network theory. Journal of Enterprise Information Management, 35(6), 1697–1718. doi:10.1108/JEIM-12-2019-0382
- Rathjens, B., Van Der Heide, B., Pham, D., Earle, K., Ulusoy, E., Mason, A. J., & and Bredland, A. (2023). Negative online reviews and manager response: Applying expectancy disconfirmation theory in a CMC context. Communication Reports, 36(2), 110–122. https://doi.org/10.1080/08934215.2022.2154815
- Sarpong, S. (2019). The challenges of job hunting and recruitment in Ghana: Opportunity for improvement. Global Business and Organizational Excellence, 38(3), 35–42. doi:10.1002/joe.21921
- Shen, X. L., Li, Y. J., & Sun, Y. (2018). Wearable health information systems intermittent discontinuance: A revised expectation-disconfirmation model. Industrial Management & Data, 118(3), 506–519. doi:10.1108/IMDS-05-2017-0222
- Shiau, W. L., Yuan, Y., Pu, X., Ray, S., & Chen, C. C. (2020). Understanding fintech continuance: Perspectives from self-efficacy and ECT-IS theories. Industrial Management & Data Systems, 120(9), 1659–1689. https://doi.org/10.1108/IMDS-02-2020-0069
- Sills, M. (2014). E-recruitment: A comparison with traditional recruitment and the influences of social media: A qualitative and quantitative review [Published Thesis]. Metropolia University of Applied Sciences. https://www.theseus.fi/handle/10024/80428
- Singh, A., Rose, C., Visweswariah, K., Chenthamarakshan, V., & Kambhatla, N. (2010, October). PROSPECT: A system for screening candidates for recruitment. Proceedings of the 19th ACM international conference on Information and knowledge management, Toronto ON Canada (pp. 659–668). ACM.
- Soon, J. M., Krzyzaniak, S. C., Shuttlewood, Z., Smith, M., & Jack, L. (2019). Food fraud vulnerability assessment tools used in food industry. Food Control, 101, 225–232. https://doi.org/10.1016/j.foodcont.2019.03.002
- Steiger, J. H. (2007). Understanding the limitations of global fit assessment in structural equation modeling. Personality and Individual Differences, 42(5), 893–898. https://doi.org/10.1016/j.paid.2006.09.017
- Subbarao, N. V., Chhabra, B., & Mishra, M. (2022). A multigroup analysis of active and passive job seekers using social media. Global Business Review, 09721509221124167. doi:10.1177/09721509221124167
- Tabachnick, B. G., & Fidell, L. S. (2007). Using multivariate statistics (5th ed.). Allyn and Bacon.
- Taber, K. S. (2018). The use of Cronbach’s alpha when developing and reporting research instruments in science education. Research in Science Education, 48(6), 1273–1296. https://doi.org/10.1007/s11165-016-9602-2
- Tan, F., Liu, J., Alvi, A., Luqman, A., Shahzad, F., & Sajjad, A. (2023). Unpacking the relationship between technological conflicts, dissatisfaction, and social media discontinuance intention: An integrated theoretical perspective. Acta Psychologica, 238, 103965. https://doi.org/10.1016/j.actpsy.2023.103965
- Van Esch, P., Black, J. S., & Ferolie, J. (2019). Marketing A.I. recruitment: The next phase in job application and selection. Computers in Human Behavior, 90, 215–222. doi:10.1016/j.chb.2018.09.009
- Vetráková, M., Hitka, M., Potkány, M., Lorincová, S., & Smerek, L. (2018). Corporate sustainability in the process of employee recruitment through social networks in conditions of Slovak small and medium enterprises. Sustainability, 10(5), 1670. doi:10.3390/su10051670
- Vidros, S., Kolias, C., & Kambourakis, G. (2016). Online recruitment services: Another playground for fraudsters. Computer Fraud & Security, 2016(3), 8–13. https://doi.org/10.1016/S1361-37231630025-2
- Viosca, R. C., Bergiel, B. J., & Balsmeier, P. (2004). Effects of the electronic Nigerian money fraud on the brand equity of Nigeria and Africa. Management Research, 27(6), 1–20. doi:https://doi.org/10.1108/01409170410784167
- Wang, Y., Anderson, J., Joo, S. J., & Huscroft, J. R. (2019). The leniency of return policy and consumers’ repurchase intention in online retailing. Industrial Management & Data Systems, 120(1), 22–38. doi:10.1108/IMDS-01-2019-0016
- Wen, C., Wang, N., Fang, J., & Huang, M. (2023). An integrated model of continued M-Commerce applications usage. Journal of Computer Information Systems, 63(3), 632–647. https://doi.org/10.1080/08874417.2022.2091682
- Wijaya, C. N., Mustika, M. D., Bulut, S., & Bukhori, B. (2023). The power of e-recruitment and employer branding on Indonesian millennials’ intention to apply for a job. Frontiers in Psychology, 13, 1062525. https://doi.org/10.3389/fpsyg.2022.1062525
- Ye, J. H., Lee, Y. S., Wang, C. L., Nong, W., Ye, J. N., & Sun, Y. (2023). The continuous use intention for the online learning of Chinese vocational students in the post-epidemic era: The extended technology acceptance model and expectation confirmation theory. Sustainability, 15(3), 1819. doi:10.3390/su15031819
- Yoon Kin Tong, D. (2009). A study of e‐recruitment technology adoption in Malaysia. Industrial Management & Data Systems, 109(2), 281–300. https://doi.org/10.1108/02635570910930145
- Zhang, J., Chen, W., Petrovsky, N., & Walker, R. M. (2022). The expectancy‐disconfirmation model and citizen satisfaction with public services: A meta‐analysis and an agenda for best practice. Public Administration Review, 82(1), 147–159. https://doi.org/10.1111/puar.13368