Abstract
Issues relating to Monitoring and Evaluation (M&E) have been established as a key and fundamental tool for the successful implementation of projects regardless of the industry. The study therefore sought to address the following questions: what effect do monitoring and evaluation practices have on tech start-ups project outcomes, as well as the role that business environment play in the relationship between M&E and project outcomes. The study followed a positivist mind-set, relying only on quantitative methods and an explanatory research design. Primary data via structured questionnaire was obtained from 317 respondents in managerial positions in the tech industry and analysed using inferential and descriptive tools. The study found that monitoring practices had a positive significant effect on project outcome. Evaluation practices also had a positive significant effect on project outcome. Business environment was found to have a dampening significant moderating effect in the relationship between evaluation practices and project outcome. However, business environment did not have any significant effect in the relationship between monitoring practice and project outcome. These findings will enable project practitioners understand the dynamics of monitoring and evaluation and the business environment when it comes to project execution. It will further enable project managers, personnel, and donors recognize how significant M&E tools are when creating policies and managing performance. Moreover, tech start-ups should create policies that recognize the integration of M&E in their operations and business functions.
1. Introduction
The immense contribution of a successful project to the development and growth of many countries across the world cannot be emphasized enough (Kahn, Citation2019). Laursen et al. (Citation2018) indicated that projects are essential for value creation and economic development, it is through projects that process and products are developed for the use of people and society. Similarly, Tyulin and Chursin (Citation2020) stressed that projects are a structured manner of bringing about change, such as developing a new product, discovering a cancer treatment or constructing a bridge across a river. Businesses and our entire way of life would stagnate without projects if we merely maintained the status quo. It is indicated that over 570 construction projects worth US$450 billion were undertaken, energy sector project worth US$370 billion and the transport sector including roads, airport and railways worth US$280 billion, evidencing the relevance of projects to the African economy. Start-Ups firms being it small, medium or large has been widely recognized as an industry that initiates and undertake numerous projects (Piccarozzi, Citation2017). There is no widely accepted definition for start-ups, according to Blank (Citation2013), and they have been categorized over time based on a variety of factors, including the age of operation, revenue, employee size, growth and development, profitability, stability, culture, and the mindset of people within the organization.
Ripsas and Tröger (Citation2014) looked at start-ups as a young business that is less than ten years in operation, uses innovative technology or has an innovative business model, and/or has a rapid increase in the size of employees or turnover. Technology start-up companies are described in a variety of ways, but they always revolve around the study of technology as well as the use of technology in the productions of goods and services (Choi et al., Citation2020). However, regardless of direct technological development, having technology or using technology to create value is included (Candi & Saemundsson, Citation2011). A technological start-up is seen as a source of employment because it typically produces new products and services, resulting in increased demand and highly skilled personnel, which necessitates the creation of new positions. According to Amedofu et al. (Citation2019), these start-ups account for the bulk of enterprises in the private sector worldwide, particularly in emerging nations. These businesses have aided in the creation of jobs and the reduction of poverty on the African continent (Abisuga-Oyekunle et al., Citation2020). For instance, it is estimated that about 450 of the tech firms in South Africa employs about 40,000 people (Mureithi, Citation2021).
Aside it’s numerous positive impacts on individuals, firm and the economy, these tech start-ups have helped to attract more investment and funds into the continent (Liu et al., Citation2023; Olaoye, Citation2023). For instance, the 2018 venture investment report indicated that start-ups in Africa raised a record of US$725.6 million across 458 contracts in 2018. Similarly, the 2020 African Tech Start-up funding report indicated that, the year 2020 was a record breaking one for African Tech firms with 397 start-ups securing US$701.5 million worth of investment. In the case of Ghana, Sasu (Citation2022) opined that 18 tech start-ups received funding in the year 2022. According to the OECD (Citation2004), there has been an increasing push for the creation of entrepreneurial start-ups in developing nations, fueled by both government and private sector initiatives. High levels of graduate unemployment, as well as the retrenchment of government workers, are pushing the burgeoning entrepreneurial trend in developing countries (Owualah, Citation1999).
The expansion of start-up businesses in developing nations like Ghana has been facilitated by the increased use of information technology like inter-organizational systems and enterprise resource planning systems, as well as the rise of global sourcing, outsourcing and offshoring (Agbenyo et al., Citation2018; Agyei-Owusu et al., Citation2018; Asamoah et al., Citation2015). Modern technology is now more accessible and inexpensive for start-ups in Ghana and Africa because of the growth of start-up eco-systems, co-working spaces, and technology hubs during the past 10 years, enabling the emergence of numerous tech start-ups (Ndabeni, Citation2008). These start-up ecosystems often give start-ups with access to financial sources, information technology, networking, co-working spaces, training, and other services that aids them flourish. The activities of these start-up ecosystems provide a platform for African and other developing-world start-ups to compete with their developed-world counterparts (Luo & Bai, Citation2021).
However, despite efforts by the government and other private entities to provide Start-ups with access to information technology and sources of funding, among other things, Start-ups in Ghana continue to face problems of sustainability and growth (Kodjokuma, Citation2018), with the majority of them failing soon after launching (Blank, Citation2013; Ries, Citation2011). A start-up assessment report by Mesa Community College on a global level showed that only 80% of start-ups established in 2014 reached the second year, 70% made it to the third year, 62% reached year fourth and only 56% survived up to the fifth year (Mansfield, Citation2019). In the African continent, statistics from the best Africa report shows that every five out of ten start-ups fail in Africa (Jha, Citation2020). In the case of Ghana, Mensah et al. (Citation2019) added to Kodjokuma (Citation2018) claim indicating that a large number of tech start-ups in the country remain the same way with no prospect of growth. The implication is that they may not be able to contribute significantly to economic development as expected.
Even though evidence from the African Development Bank (Citation2006) identified factors such as political, socio-economic and technological among others as the main actors that play a major role in the cause of start-up project failure, Polishchuk et al. (Citation2019) however indicated that a critical aspect of the business strategy and management is the issue of implementing monitoring and evaluating programs in start-up projects. Kusek and Rist (Citation2004) opined that, Monitoring and Evaluation (M&E) is one of the critical elements and most relevant tool for influencing project success and completion. This assertion was later supported by Damoah et al. (Citation2015) who deemed M&E-related issues to be the most important key determinant of project success, hence establishing the relevance of M&E in ensuring project success. M&E is defined by Pullin and Knight (Citation2003) as an activity that supports decision-making based on evidence to attain project objectives.
Shapiro (Citation2007) characterized M&E as the methodical collection and analysis of data and the procedures for determining whether or not targets and milestones are being reached, as well as analyzing any differences. Ngeru and Ngugi (Citation2019) further stressed that M&E constantly tries to improve project efficiency and effectiveness. According to Kissi et al. (Citation2019), Project management organizations and agencies can satisfy the needs of donors and financiers by implementing M&E systems. This is so that it provides evidence of the project’s success. Chebet (Citation2021) asserts that continuous project monitoring guarantees that the project’s implementing team oversees the project’s activities, reviews and updates the project plan and budget as necessary, and examines timetables and deliverables to help clarify any changes that depart from the original project plan. As a result, early warning signs are provided to management by M&E in terms of delays and cost of variations, as well as evidence.
Notwithstanding, the successful implementation of M&E depends mostly on the actors in the environment in which the firm operates. As indicated by the Institutional Theory, organizational decision-making, operations, and practices are constrained by a variety of external pressures. The environment is said to interact with one another to affect how well a firm conducts its internal operations and performance. In a complex and dynamic society like Ghana, where organizations in the business world don’t function independently, understanding the business environment is essential for effective management (Hanaysha, Citation2016). For tech start-ups to remain relevant and expand, they must adapt to these changes. The goal of this study is to examine the effects of project monitoring and evaluation on project outcomes, as well as the function of the business environment in influencing the relationship between these factors. The business environment plays a major role by influencing the effective implementation of monitoring and evaluation practices (Eruemegbe et al., Citation2015). Threat from the environment can hinder the successful implementation of M&E systems, availability of infrastructures and other business development services would help tech start-ups to run an effective monitoring and evaluation practices as the presence of these factors provides a smooth mechanism for the execution of M&E practices.
Previous studies however have focused on issues of resource constraints to start up growth and development emphasizing on access to funding and the absent of business strategy. For instance, studies such as Ayalew and Xianzhi (Citation2020) analyzed how financial constraints affect innovation development in eleven African countries. Similarly, Tullock (Citation2010) assessed the capital constraint to agribusiness sector as an emerging start-up in South Africa. Johnson (Citation2018) found that many of the issues of these tech start-ups in Ghana are caused by institutional elements like rules and administrative procedures. It is evident from these studies that, much attention has not been paid to the critical role M&E play in tech start-up success within the Ghanaian context. It is therefore relevant to assess the role M&E plays when it comes to SMEs specifically tech-start-ups project success and outcomes in the Ghanaian society.
Furthermore, existing studies on M&E have looked at these two concepts as a composite variable. For instance, Kamau and Mohamed (Citation2015) focused on M&E role in achieving project success in the Kenyan society. Kissi et al. (Citation2019) and Tengan and Aigbavboa (Citation2017) looked at M&E in the construction industry of Ghana. Fransisko (Citation2016) focused on M&E effectiveness in ensuring project success in Indonesia. A similar study by Arbolino et al. (Citation2018) looked at the role of M&E in industrial sustainability in the Italian region. It is evident from these studies that, these two concept have been looked at as composite word, however, it is worth noting that monitoring and evaluation are two different concepts that are related but not the same, it is therefore necessary to assess their individual effect on project outcomes in order to draw an objective conclusion on their individual impact on project success.
Moreover, these studies again provide evidence of both geographical and contextual gap in existing literature. This is because a number of these studies on M&E have focused on other sectors rather than the tech industry. Others also concentrated in different countries thereby making it inappropriate to adopt their recommendations for the tech industry in Ghana as it may be misleading. Also, none of these studies have also looked at how the business environment influences the implementation of internal policies and operations within the tech start-up sector. The study is motivated by the need to analyze how M&E impact on tech start-ups growth and sustainability, impacting on their business operations. Thus, leading to their contribution to economic development. It further investigates how the factors of the business environment influence the effective implementation of M&E and how it subsequently affect the outcome of tech start-ups projects.
The study demonstrates how monitoring and evaluation can be a powerful tool for helping stakeholders and organisations achieve more accountability and transparency, which will aid policymakers in developing effective monitoring and evaluation systems. Also, the conclusions reached would offer a great value to the body of knowledge for project management researchers, particularly in the application of monitoring and evaluation practices. The paper is organized in four sections. The first section focuses on the introduction highlighting the background, statement of the problem as well as the research gap. The second section discusses literature on M&E by providing theoretical foundations, discussing the different concepts in the study as well as reviewing pertinent literature which served as the foundation for establishing the gap in this study. The third section outlined the methods employed in the study whereas the last section focused on the results, discussion and conclusion of the analysis.
2. Literature Review
The Program Theory, Evaluation Theory and the Institutional Theory guided the study. The study used the first two theories due to their applicability in explaining the relevance of M&E in ensuring project success. And the Institutional Theory was used to explain the role of the business environment in influencing the impact of internal operation on firm performance. The programs theory have been used over the years to explain the relevance of undertaking M&E activities as an intervention to achieve desired results. The theory clarifies the way an intervention (project, program, policy, or a strategy) adds to a chain of outcomes that create the desired or actual outcomes. Depending on how the intervention is implemented, it might have both positive (useful) and negative (harmful) consequences (Thomson et al., Citation2019). It provides a rationale for why the activities you provide will result in the outcomes or benefits you seek, making it the foundation of the success of any program or initiative. The current study analyzes how M&E as an intervention predicts project outcome of tech Start-ups in line with the tenets of the programme theory. As an intervention M&E procedures are fundamental inputs that, when properly applied, result in input processing and, ultimately, quantifiable output. In this perspective, program theory examines the impact of changing input and processes to increase output and produce quality results. Similar to the programmes theory, the fundamental principle underlying the evaluation theory is that in order to ensure the successful achievements of a project objectives and determines its relevance and sustainability, there is the need to have in place a designed mechanism to help compares the project impact to what was planned in the project plan.
The institutional theory on the other hand discussed how symbolic actions and outside influences rather than functional considerations were more likely to be the driving forces behind organizational founding and change. The institutional theory’s underlying assumption is that organizational decision-making, operations, and practices are constrained by a variety of external pressures. Organizations are therefore concerned with putting the right policies in place as well as gaining the trust and support of external stakeholders. Several researchers have stressed on the level of power the forces in the environment have over firms’ activities and performance. This theory asserts organizations operations are likely to be successful in a stable environment than a constant changing and dynamic environment. Hence indicating that if firms are unable to establish appropriate mechanism to respond to these constraints from the environment, they are likely to face challenges with regards to implementing internal operations and practices such as monitoring and evaluation practices.
2.1. Empirical review
Globally, monitoring and evaluation have received it fair share in literature with a number of studies looking at its impacts on project success. For instance, Kissi et al. (Citation2019) investigated the influence of project M&E practises on construction project success criteria and discovered a positive statistically significant association between M&E practises and construction project success criteria. A similar study by Fransisko (Citation2016) shows that in order to enhance the effectiveness of project execution in CINTA, management commitment and the availability of procedure implementation monitoring and evaluation are recommended. Kamau and Mohamed (Citation2015) conduct a literature assessment on the effectiveness of monitoring and evaluation in ensuring project success in Kenya. Strength of the M&E team, monitoring technique used, political influence, and project lifecycle stage have all been recognised as factors that contribute to project success. The study also highlighted managerial support as a mediating element between M&E and project success. A good M&E without managerial support is unlikely to succeed. Arbolino et al. (Citation2018) examined the monitoring and evaluation of industrial sustainability in Italian regions and offered a novel technique based on a reinterpretation of the traditional SWOT analysis to assess industrial sustainability at the regional level. The following section reviewed pertinent articles in relation to the specific objectives of the study with the aim of comparing and contrasting their findings.
2.2. Monitoring practices and project outcome
At each stage of a project’s life cycle monitoring practices are required to assure the efficacy of the given project (Herman-Mercer et al., Citation2018). For a project to be finished on schedule and on budget, and within the project’s scope, monitoring and control are crucial. Procedures for monitoring tracks deviations from the project plan and help managers to make sure it is timely, effective, and efficient (Yousefi et al., Citation2019). Several studies have explored the relevance of monitoring as a tool in ensuring project success. For instance, Muchelule et al. (Citation2017) revealed that monitoring techniques have a substantial influence on project output and outcome within the Kenyan state corporation. In a similar vein, Nega (Citation2020) found that project monitoring and control practices have a significant impact on project success.
Belout and Gauvreau (Citation2004) conducted yet another investigation on the relationship between project success and monitoring. They discovered that the adoption of formal monitoring practices, such as progress reports and performance indicators, was particularly advantageous and that project monitoring was positively related with project success. The study also discovered that when project monitoring was included into the broader project management process, it performed at its best. Another study was conducted by Turner and Müller (Citation2005) to investigate the relationship between construction project performance and project monitoring. They discovered that efficient monitoring procedures, including regular progress meetings and performance monitoring, were positively related to project success. Additionally, the study discovered that monitoring practices worked best when they were included into the project management cycle and when they were in line with the objectives of the project.
Huang et al. (Citation2011) study looked at how monitoring practices affect software project outcomes. The study discovered that the success of a project was favorably correlated with the adoption of efficient monitoring techniques, such as project status reporting and progress tracking. Effective monitoring techniques were also shown to be crucial in the study’s sophisticated software development initiatives. Karim et al. (Citation2015) looked at the relationship between project success in the public sector and monitoring practices. The study revealed that efficient monitoring techniques, performance indicators and progress reports, were strongly related to project success. Additionally, the study discovered that efficient monitoring procedures were crucial for public sector initiatives, which frequently include intricate stakeholder interactions and conflicting demands.
Abebe (Citation2018) also revealed that project Monitoring and Controlling process groups had a substantial influence on the outcome of the project, suggesting that high levels of project monitoring and control are more likely to provide greater project success. Young et al. (Citation2019) further revealed five project governance techniques namely “monitoring, change, vision, sponsor and KPI” to have a substantial correlation with project performance and to be effective at various points of the project lifecycle. Monitoring according to Kabonga (Citation2018) provides information on how an intervention is doing in relation to its objectives. Monitoring provides signs that models may be veering off course or not functioning as planned, In light of this, the study aims to determine the effect of monitoring practices on project outcome within the tech start-up sector in Ghana. The study therefore hypothesis that:
H1:
Monitoring practice has a significant effect on project outcome in tech Start-ups in Ghana
2.3. Evaluation practices and project outcome
Evaluation have been considered as the key measure of project efficacy (Otieno, Citation2000; Sherman & Ford, Citation2014). Over the years project managers have focused on evaluation as a tool to measure and assess whether their project output was able to meet its objectives and also determines the relevance and sustainability of the project. On the other hand a number of managers fall on this tool when they are confronted with several project options to choose from. Thus in the case of mutually exclusive project, evaluation tools are employed to assessed the profitability, risk and impact of each project to aid project managers make an informed decision (Habibi et al., Citation2018). This demonstrates how imperative evaluation tools are in the project-based industry.
Studies have shown that effective evaluation practices may enhance project planning, monitoring, and control procedures, which can lead to better project outcomes. For instance, a study by Zhang and Yang (Citation2018) showed that incorporating evaluation practices into the project management process increased project success rates. Blackwood et al. (Citation2018) study looked into how evaluation practices affected project outcomes in the nonprofit sector. The findings revealed a positive association between evaluation and project outcomes. Olejniczak, Kupiec and Newcomer (Citation2017) also indicated that learning from evaluation results considerably increased the efficacy and efficiency of project performance. They argued that evaluation practices may aid organizations in learning from past mistakes and enhancing the success of upcoming projects. This is due to the fact that evaluation offers project managers feedback that helps them to recognize strengths and flaws and make the required modifications for next projects. Oliveros-Romero and Aibinu (Citation2019), also find out from interviewing experts that ex-post evaluations are relevant to PPP projects. A study by Uzunkaya (Citation2017) also revealed that theory-based evaluation is a potentially useful evaluation technique that could be tailored to the complexity of PPP projects and programs and would broaden the toolkits available to evaluators.
Kabonga (Citation2018) asserted that if objectives are not met, evaluation reveals the cause behind it. Causality is said to be a function of evaluation. Thus evaluation then reveals the truth behind, bringing the larger project to the forefront environment. This evidences the relevance of evaluation with regards to ensuring project success and outcome. On this note, it is relevant to have an empirical evidence showing the impact of evaluation on project success in the tech start-ups within the Ghanaian context. The study therefore argues that evaluation practices influence project outcome. Given this we hypothesis that:
H2:
Evaluation practice has a significant effect on project outcome in tech Start-ups in Ghana
2.4. Business environment, monitoring practices and project outcome
The relevance of monitoring has been documented in extant literature established by several researchers as demonstrated in the sections above. Monitoring practices are critical procedures at any stage of a project since it’s allow for continuous observing of the project’s efficacy (Ahuja & Thiruvengadam, Citation2004; Kissi et al., Citation2019). Monitoring according to Kihuha (Citation2018) serves as an intervention to aid improve success of a project output. Arce et al. (Citation2020) established that these interventions depends on certain circumstance to execute it role effectively. Thus any activity depends greatly on certain circumstances which could affect it positively or negatively. These circumstance is termed as the environment in the management world and they play a crucial role in firm activities and performance.
This assertion is evident in the work of Eruemegbe et al. (Citation2015) who indicated that, given how organizations and the environment interact, an organization’s performance depends on how it reacts to, understands, and influences particular environmental changes. Hence for the achievement of optimal organizational performance, resources must be used carefully to prevent waste (Eruemegbe et al., Citation2015). Both the internal and external environment influences each decision made in the organization especially factors that plays in the external environment are beyond the firms’ control and requires managers to keep up to date information about these factors playing in the external environment. This indicates that if management does not handle these threats effectively, all initiatives and decisions that the organization makes is likely to be affected negatively. For instance, a large tax system would reduce firms profit affecting their ability to establish and implement monitoring practices as they may lack funding to do so. Also lack of management support in M&E implementation can invariably hamper its effectiveness thereby affecting the organizational goals and project outcomes. Due to this negativity associated with the business environment, Eruemegbe et al. (Citation2015) stated that management must create appropriate adjustments and measures to control and handle these environmental issues.
In addition to this, numerous studies have examined the moderating effect of the business environment on the relationship between monitoring practices and project outcome. For instance, Krogstie et al. (Citation2017) looked at how the business environment affected how well project monitoring practices worked in the construction sector. They discovered that the degree of collaboration among project stakeholders as well as the project’s complexity and level of business environment unpredictability all had an impact on how successful monitoring practices were. Similar to this, Joslin and Müller (Citation2016) looked at how the business environment affected the relationship between project success and monitoring practices in the information technology sector. They discovered that elements including the degree of industry competitiveness, the state of technical development, and the regulatory environment had an impact on the efficacy of monitoring practices.
Another research by Eroglu and Karaarslan (Citation2018) looked at the business environment’s moderating impact on the relationship between project performance and monitoring practices in the manufacturing sector. They discovered that the degree of market volatility, the amount of industry innovation, and the degree of competitiveness all had an impact on the efficacy of monitoring practices. These studies suggest that a number of business environment variables, such as project complexity, level of uncertainty, degree of collaboration, degree of competition, degree of technological advancement, regulatory environment, degree of market turbulence, and degree of innovation, can have an impact on the effectiveness of implementing monitoring practices. In light of this, the study argues that if the business environment is favorable, it is likely to improve on firms monitoring activities therefore leading to a positive outcome. Based on this the study hypothesized that:
H3:
Business environment plays a significant moderating role in the relationship between monitoring practice and project outcome in tech Start-ups in Ghana.
2.5. Business environment, evaluation practices and project outcome
Evaluation have been established as a tool to assess the relevance, efficiency, impact, effectiveness and sustainability of a developmental intervention. Without evaluation management would not be able to determine whether resources have been used effectively and whether the project outcome meets specification and ultimately addresses its intended purpose. However, it is worth noting that the establishment and implementation of these evaluation practices depend heavily on the state of the environment in which the business is operating. Eruemegbe et al. (Citation2015) asserted that every business entity must exist to some extent; no organization can operate in isolation. Each organization has objectives and duties in relation to people in its surroundings. And due to the uncertainty nature of the environment, actions taken internally within the organization can be influenced.
Numerous studies have examined the moderating effect of the business environment in the relationship between evaluation practices and project outcome. A study by Lee and Kim (Citation2018) revealed that the relationship between project performance and evaluation practices is moderated by the business environment. Belout and Gauvreau (Citation2004) discovered in another study that the association between project success and evaluation practices is moderated by the business environment. They discovered that whereas evaluation practices had no discernible impact on project performance in a dynamic and unpredictable business environment, they are favorably correlated with project success in a stable and predictable business environment.
Similarly in a recent study, Shehu and Shehu (Citation2015) also discovered that the association between project success and evaluation practices is moderated by the business environment. They discovered that whereas evaluation practices have no discernible impact on project performance in an adversarial and unstable business environment, they are favorably associated to it in a stable and supportive company environment. Evidence from these literature indicate that the business environment is a major key that can make and unmake organizational policies, thus in a highly competitive and stable business environment, evaluation practices can enhance project performance and success, However, in a less competitive and dynamic business environment, evaluation practices may have limited impact on project performance and success hence the need for management to handle these factors effectively to ensure that it does not hinder the successful implementation of their evaluation policies and systems. This study thereby argues that the business environment if managed effectively could enhance evaluation practices and subsequently improve on project performance and outcome. Based on this, the study hypothesized that:
H4:
Business environment plays a significant moderating role in the relationship between evaluation practice and project outcome in tech Start-ups in Ghana.
2.6. Conceptual Model
Based on the results obtained from the reviewed literature, we develop a research model to demonstrate the relationship between monitoring practices, evaluation practices, business environment and project outcome. The framework is depicted in Figure .
3. Research methodology
The study followed a positivist research philosophy, relying only on quantitative methods and an explanatory research design. Data from prior research on monitoring practices, evaluation practices, the business environment, and project outcomes were used to create a structured questionnaire. The study’s population comprised tech start-ups in Ghana. Specifically, the study targeted tech start-ups within some selected regions of Ghana including Greater Accra, Ashanti, Central, Eastern, Northern, Savannah, Volta and Western. The selection of these regions was due to the highly concentrated number of tech start-ups in these areas. The study specifically targeted managers occupying various positions in the tech industry to acquire information about monitoring and evaluation and project outcome in the industry. The simple random sample procedure was utilized to collect data from 317 respondents in managerial positions. The items measuring monitoring practice were adapted from Kissi et al. (Citation2019); evaluation practices were adopted from Gomes (Citation2020) and Thaddee, Prudence and Valens (Citation2020); business environment was adopted from Cherunilam (Citation2021) and Dvorský et al. (Citation2021) whereas project outcome was adopted from Cruz Villazón et al. (Citation2020). It was measured on a 5-point likert-like scale ranging from 1=least agreement to 5=highest agreement. The data was processed using IBM SPSS Statistics (version 23) and SmartPLS (version 3). Data was analyzed using both descriptive and inferential statistics. The respondents’ socio-demographic data was analyzed using frequencies and percentages. Following previous studies, we used structural equation modelling method to test the hypotheses (Aditjandra et al., Citation2012; Elahi et al., Citation2022). The significance test assumed here was that the t-statistics should be greater than 1.96 and the p-value should be less than 0.05.
4. Data analysis and discussions
4.1. Respondents’ demographic features`
Majority of the respondents (189) were males, represented 59.6% of the total 317 respondents whiles females were 122 representing 38.5%. However, the remaining 6 respondents representing 1.9% did not indicate their sex. This suggest that the tech sector has more males managing their project activities as compare with females. In terms of respondents’ position in the firm, 164 representing 51.7% were owners/managers, 82 were project managers representing 25.9%, M&E manager were 34 representing 10.7% of the respondents and the remaining 37 representing 11.7% occupies various different positions in their respective firms. This result showed that in most of these tech start-ups, the owner’s doubles as managers of their project activities. Respondents’ were also ask to indicate the specific region that their firm is located. 122 representing 38.5% indicated that their firms’ are located in the Greater Accra region. This was followed by the Ashanti region which recorded a total of 82 firms representing 25.9%. Western region had a total of 52 representing 16.4%, Eastern region had 18 representing 5.7%, followed by Central region which had a total of 10 representing 3.2%. Northern region, Savannah region and Volta region had a total of 13, 11 and 9 representing 4.1%, 3.5% and 2.8% respectively. This showed that most of these tech start-ups are located in the capital city.
Additionally, respondent were asked to specify the sector of their firm. 81 representing 25.6% were into product, services and process development. 72 (22.7%) were into marketing and 70 representing 22.1% were into Transport activities. A total of 42 (13.2%) firms were in the financial sector, e-commerce sector had a total of 35 representing 11.0%. 14 representing 4.4% are into agriculture and 3 of the firms representing 0.9% are into areas other than those specified above. This shows that most of these tech start-ups are more into products, service, process, marketing and transport operations. The number of employees in each of these firms were also inquired, it was observed that 252 of the firms representing 79.5% had between 1 to 10 employees. Those with 11 to 20 employees were 36 representing 11.4%, 10 of the firms representing 3.2%, had 21 to 30 employees. 8 representing 2.5% had 31 to 40 employees, 4 representing 1.3% had 41 to 50 employees. Also those with 51 to 60 employees were 3 representing 0.9%. On the other hand, those with 61 to 70 employees were 2 representing 0.6%. Likewise those with 71 to 80 employees were also 2 representing 0.6% as well. This shows how the sector is contributing to economic development through job creation. Finally the study sought to ascertain whether these firms have an M&E expert in their respective firms that oversees the monitoring and evaluation activities during their project execution. 161 represented 50.8% indicated that their firm has an M&E expert whiles 156 representing 49.2% indicated that their firm does not have an M&E expert.
4.2. Assessment of PLS-SEM
Prior to the actual hypotheses testing, the qualities of the PLS-SEM were first assessed using indicator reliability (IR), convergent validity (CV), construct reliability (CR) and discriminant validity (i.e., HTMT). Hair et al. (Citation2019) and Henseler (Citation2017) stressed that the model qualities are assessed and reported to make meaning of the regression model results. They also ensure that the model meets the expected criteria and thus, its findings could be relied upon to influence policies and practices in any organizational setting.
4.2.1. Indicator reliability
The first step in assessing the quality of the model was to determine whether the measurement items for the construct in the outer model are reliable. The indicator values for the constructs were then evaluated in order to assess the quality of the scale items. The evaluation was carried out to make sure that each indicator offers an accurate reflection of the allocated construct. The rule of thumb for acceptable item reliability is that, each indicator’s loading should be greater than 0.70 to indicate that it is a good predictor of its construct (Hair et al., Citation2021; Henseler et al., Citation2015). Therefore, items considered to be subpar measures of their assigned constructs, were eliminated since their items loadings were less than 0.70 (Hair et al., Citation2017, Citation2019; Memon et al., Citation2021; Wong et al., Citation2019).
Item loadings that are deleted from the model, according to Hair et al. (Citation2019), do not offer accurate measurements of the assigned constructs. Because of this, leaving them could affect the model’s output. As a result, all item loadings below 0.7 in the initial model that affected AVE and composite reliability were appropriately deleted, showing that not all of the items collected from prior studies were accurate measurements of the constructs they were allocated in the context of this study. Following the removal of all indicator loadings below 0.70 as recommended by Hair et al. (Citation2017) and Henseler et al. (Citation2009) the final model structure is showed in Figure .
4.2.2. Internal consistency reliability and validity
To assess the internal consistency reliability of the model, Cronbach and Meehl’s (Citation1955) Cronbach Alpha, Jöreskog’s (1971) composite reliability and Dijkstra and Henseler’s (Citation2015) rho_A were used to assess the internal consistency reliability of the model.. This study however relied on the composite reliability to determine the internal consistency reliability other than the others. Composite reliability, unlike Cronbach’s Alpha, does not demand that the population’s indicator loadings be uniform across the board. This follows the working principle of the PLS-SEM algorithm, which prioritizes the indicators in the model estimation on the basis of their reliability.
Internal consistency reliability seems to be undervalued by Cronbach Alpha, which frequently depends on how many elements are on the scale. Thus, scores between 0.60 and 0.70 are deemed appropriate when employing composite reliability, whereas values between 0.70 and 0.90 are preferred in a more advanced research phases (Nunnally & Bernstein, Citation1994). From Table , composite reliability for the outer model varied from 0.70 to 0.90, indicating that the constructions’ internal consistency was confirmed.
Table 1. Assessment of measurement model
This section also evaluated and discussed the convergent validity (CV) thus the outer model validity of the regression model and the results are shown in Table . The average variance extracted (AVE) values are used to describe the CV (Hair et al., Citation2014; Citation2017). The AVE values show the degree to which an indicator’s variance is captured by the latent construct with respect to the sum of variance and its resulting measurement error. The study complied with the rule that all AVE values should be > 0.50 for CV to occur (Bagozzi & Yi, Citation1988). The table showed that all of the AVE scores were greater than 0.50, with the lowest value (BE) being 0.501 and the highest being 0.556 (PO). Simply put, the model met the quality criterion hence its validity was convergent.
The model’s discriminant validity (DV), as proposed by Henseler et al. (Citation2015) was also tested as part of the study to evaluate the model’s quality. In a model, DV looks for potential collinearity problems (Hair et al., Citation2017). According to Hair et al. (Citation2017), DVs with significant degrees of discriminant validity typically don’t have collinearity. Three main methods for examining DV in a PLS-SEM model have been presented in earlier research (Fornell & Larcker, Citation1981; Hair et al., Citation2019; Henseler et al., Citation2015). These approaches included Fornell and Larcker (Citation1981), cross loadings and Heterotrait-Monotrait (HTMT) ratio. However, this study employed the Fornell and Larcker and HTMT approach (i.e., in Table ).
Table 2. Fornell-Larcker criterion
Table 3. Heterotrait-monotrait ratio (HTMT)
The Fornell and Larcker method reveals that constructs differ from their indications more than any other construct. Thus constructs have more variance with their indicators than any other construct. Each construct’s AVE must be greater than the greatest square correlation of any other construct in order to meet that requirement. The impacts of the Fornell-Larcker Criterion are presented in Table and the AVE of each construction exceeds the square.
Nevertheless, current research suggests that the Fornell-Larcker criteria are insufficient for establishing the validity of discriminants. According to Henseler et al. (Citation2015), the Fornell-Larcker criterion does not work well when the stressors on a construct indicator vary widely. Voorhees et al. (Citation2016) suggested a heterotrait-monotrait (HTMT) link as a consequence. The (geometric) average correlations of the objects that measure the same construct divided by the average value of the item correlations across all constructs is known as the HTMT. More precisely, HTMT has the strength of easily detecting absence of DV in basic research unlike the others. Values for the construct’s HTMT is displayed in Table below.
The rule of thumb for assessing HTMT is that the correlation values between the constructs should be < 0.90 (Wetzels et al., Citation2009). Simply put, discriminant validity is achieved if the HTMT scores are < 0.90. It could, therefore, be deduced from Table that all the HTMT values for the constructs are < 0.90 with the highest value of 0.835 in the relationship between MP and PO. This result suggests that the constructs are clearly different from each other.
4.2.3. Structural model assessment
After assessing the measurement model for quality purposes, Hair et al. (Citation2019) indicates that there is the need to assess the structural model. Assessing the structural modelling includes assessing the multi-collinearity (VIF), co-efficient of determination (R2), Effect size (f2) and predictive relevance (Q2). This is proceeded with an assessment of the significance and size of the path co-efficient for the hypothesized relationships.
The inner VIF scores are presented in Table in order to test for potential multicollinearity. Additionally, it aids in minimizing frequent method bias in the research. According to Hair et al. (Citation2021) multicollinearity is evaluated to determine whether the path coefficients are bias-free. Additionally, it makes sure that any substantial areas of potential collinearity between the exogenous variables are drastically reduced. To check for multicollinearity, all inner VIF values must be less than 10 (Pallant & Manual, Citation2007). Pallant and Manual (Citation2007) claim that multicollinearity exists when the VIF scores are greater than 10, and that this could have an impact on the model’s accuracy or quality. All of the VIF values were well below 10, which indicated the absence of multicollinearity. To be more exact, there was no evidence of multicollinearity among the constructs as the VIF values ranged between 1.041 and 2.090.
Table 4. Vif
4.2.4. Explanation of target endogenous variable variance
With the absence of multicollinearity, this section describes the model’s predictive accuracy by reporting the coefficient of determination (R2) score. It also reported other key estimations such as, “predictive relevance (Q2) based on the Stone-Giesser’s test and effect size (f2)” (Hair et al., Citation2019). Table presents the results obtained for the co-efficient of determination (R2), the cross validated blinding redundancy measure (Q2), and the effect size (f2).
Table 5. Explanatory power of exogenous variables
These components were examined to see if the constructs were reliable measurements of the model’s quality and if so, whether the model’s output could be trusted to produce factual results. First reported was the predictive relevance score using the R2 value. According to Hair et al. (Citation2017), the R2 represents the sum of the predictors’ (MP, EP) contributions to the dependent construct (PO). Simply put, R2 suggests the change in PO that is linearly accounted for by combining the two independent variables (MP, EP). According to Henseler et al. (Citation2009), R2 values 0.25, 0.50 and 0.75 represent respectively weak, moderate and strong contributions of the predictor constructs to the endogenous construct.
From Table , the R2 value was 0.562; meaning that when the two independent variables (MP, EP) are combined, they linearly account for about 56.2 percent of change in the project outcome (PO). Simply put, for any change in tech start-up project outcome, MP and EP combine to linearly account for about 56.2 percent of such change. However, because R2 values increase with the number of predictors, adjusted R2 is recommended since it accounts for model complexity and helps compare models. Table presents R2 adj. values of 0.555 for project outcome. Thus, monitoring practices and evaluation practices explained 55.5% of consumers buying behavior variances.
The effect size of each independent constant was assessed by adopting Cohen (Citation1988) impact criterion and the results are presented in Table V. According to Cohen (Citation1988), values of 0.02 denote a small effect size, 0.15 a medium effect size, and 0.35 a big effect size. From the table, BE had the lowest f2 value of 0.006; followed by EP with 0.117. However, MP had the highest f2 value of 0.216. Based on Cohen (Citation1988) criteria, BE is said to have a weak effect size whiles EP and MP had a medium effect size (f2). These results suggest that when the two independent variables (MP and EP) are individually implemented, MP would have the largest effect on the project outcome of these tech firms followed by EP respectively.
Finally, the model’s predictive relevance based on Stone-Geisser’s (Q2) test (Hair et al., Citation2014) was reported. Q2 is analyzed by removing a portion of the data matrix, analyze the model and predict the removed part based on the estimations (Roldán & Sánchez-Franco, Citation2012). Chin (Citation2010) suggested that Q2 is achieved if it is > 0 for the construct. Henseler et al. (Citation2009) proposed that, 0.02 ≤ Q2 <0.15 shows weak effect, 0.15 ≤ Q2 <0.35 indicates moderate effect and Q2 >0.35 signifies strong effect. One can therefore conclude that all the Q2 values were > 0 indicating that the predictors can relevantly predict the endogenous variable in the model. However, BE had the highest Q2 of 0.298; followed by EP (0.268), and MP (0.248) respectively. This means that although BE has a small and weak effect size, it is a better predictor of project outcome when compared with EP and MP which had high effect size.
4.2.5. Significance of path coefficients
Following a quality assessment of the PLS-SEM, the study examined the hypotheses to see whether significant effects exist among the associations. This was accomplished by analyzing the data with 5000 bootstraps, as suggested by Hair et al. (Citation2017). Table presented the results with five columns representing structural paths, path coefficients (β), t-stats, p-values and decision rule of each hypothesis.
Table 6. Structural equation model output and decision rule
The hypotheses were tested in this study by reporting the t-stats values indicated by Hair et al. (Citation2021), Ringle et al. (Citation2012), and Roldán and Sánchez-Franco (Citation2012). The criterion indicates that the t-stat should be greater than 1.96 (i.e., p < 0.05) to demonstrate that the hypothesized relationship is significant (Hair et al., Citation2014; Citation2014). Simply put, a t-stat >1.96 is equivalent to a p value of less than 0.05, implying that the directional hypotheses (as shown in Table ) are supported, however one of the moderating relationship was not significant at 0.05. The results of the hypotheses were given and discussed in the sections below.
4.2.6. Direct relationships
The PLS-SEM technique was employed to examine the relationship between monitoring practices and project outcome, it further analyze the effect of evaluation practices on project outcome. By interpreting the bootstrapping results, the significant effects at a 95% confidence level on causal paths as presented in Table reported a significant effect of monitoring practice on project outcome (t = 7.119, p= 0.000). Given this result, the t-test of 7.119 was greater than 1.96 threshold and a 0.000 p-value is also lesser than 0.05, hence meeting the criteria by (Hair et al., Citation2014, Citation2014). With a β value of 0.442 (as showed in Table ), indicates that the relationship between monitoring practices and project outcome is positively significant. Thus MP can directly predict a change in PO. This also implies that any unit change in monitoring practices would lead to a significant positive unit change in project outcome by 44.2 percent. Also, per the β value, it can be deduced that the effect of monitoring practices on project outcome is moderate. In a similar vein, the result in Table revealed that evaluation practices positively influenced project outcome (β = 0.328; t = 4.879; p = 0.000). This result implies that the model’s t-test of 4.879 was more than 1.96 and the p-value of 0.000 was less than the 0.05. This indicates that any unit improvement in evaluation practices would result in a 32.8 percent increment in the outcome of tech project. This result based on the β = 0.328, shows a moderate effect.
4.2.7. Moderation relationships
To assess potential moderation effects we employed the simple slope analysis to first establish possible interaction among the variables under consideration. From Figure , it was revealed that the slopes had a dis-ordinal interaction with each other indicating that there is an interaction between business environment, monitoring practices and project outcome, however by interpreting the bootstrapping results, the significant effects at the 95% confidence level showed that the interaction was not significant. The bootstrapping results reported a [t = 1.820, p < 0.069] indicating that even though an interaction exists between these variables, such interaction was not significant, hence the hypothesis was not supported.
On the other hand, business environment base on the slope in Figure showed an interaction between business environment, evaluation practices and project outcome. This interaction was however significant with a t = 2.334, p < 0.020 based on the bootstrapping results. However, evidence from Table using the β values showed that the introduction of the business environment weakens the direct relationship. Thus it drop from (β = 0.442 to β = 0.164).
4.3. Discussion of results
We examined the effect of monitoring practices on project outcome of tech start-up in Ghana. In view of this, the study hypothesized that Monitoring Practices (MP) had a significant effect on project outcome (PO). The PLS-SEM result supported our hypothesis that monitoring practices have a significant effect on project outcome. This could be justify that project objectives and outcomes are easily achieved when monitoring practices are put in place by these firms. Regular progress meeting, process and performance monitoring are all essential activities to ensure project success. Evidence from previous studies confirms that monitoring practices have a positive significant effect on project outcome. For instance, Muchelule et al. (Citation2017), revealed that monitoring techniques have a substantial influence on project output and outcome within the Kenyan state corporation. Nega (Citation2020), found that project monitoring and control practices have a significant impact on project success. Turner and Müller (Citation2005), discovered that the adoption of formal monitoring practices, such as progress reports and performance indicators, was particularly advantageous and that project monitoring was positively related with project success. Similarly, Belout and Gauvreau (Citation2004) revealed that the adoption of formal monitoring practices, such as progress reports and performance indicators, was particularly advantageous and that project monitoring was positively related with project success. Also, Huang et al. (Citation2011) and Karim et al. (Citation2015) among others all revealed that monitoring practices have a significant positive effect on project outcome and success.
Also, the Weiss (Citation1972) programs theory, which describes how an intervention contributes to a chain of results that creates an intended or actual outcome, has been used to corroborate the study’s findings. Depending on how it is carried out, the aforementioned intervention could have a beneficial or negative outcome (Thomson et al., Citation2019). The current result indicates monitoring as an intervention, predicts project outcome of tech Start-ups in line with the tenets of the programme theory. Thus monitoring practices are in the form of basic inputs that, when used correctly, translate to input processing and, finally, measurable output. Hence if these intervention are executed effectively, it would affect the outcome of tech firms’ projects.
Moreover, this study revealed a positive significant relationship between evaluation practice and project outcome within the tech Start-ups sector in Ghana. The outcome course of the PLS-SEM result supported our theoretical prediction that evaluation practice has a significant effect on project outcome. The positive effect of evaluation practices on project outcome could be explained that it is through evaluation that project output and performances can be measured against planned. Thus it is through evaluation that one can determine whether a development intervention is effective, successful, relevant, efficient, impactful and sustainable. Thus, evaluation helps to assess the efficacy of a particular program (Rossi et al., Citation2018).
The importance and significance of undertaking evaluation practices can further be justified based on empirical, theoretical and practical evidence. The result is been supported by the evaluation theory which according to McCoy (Citation2005), compares the project impact (outcome) to what was planned in the project plan. Evaluation theory evaluates the efficacy of a project in meeting its objectives and determining the relevance and sustainability of a continuing project. Implying that the best way to assessed whether a particular project has met its intended purpose is through evaluation, hence the need for managers to ensure that evaluation activities are done at the right time with the appropriate tools to prevent the project activities from deviating from it intended purpose. The findings are also consistent with the findings of Zhang and Yang (Citation2018), who discovered that incorporating evaluation practices into the project management process increased project success rates, Blackwood et al. (Citation2018), also revealed a positive association between evaluation and project outcomes, likewise, Olejniczak, Kupiec and Newcomer (Citation2017) who indicated that learning from evaluation results considerably increased the efficacy and efficiency of project performance
Also, the study simple slope analysis reveal an interaction between business environment, monitoring practices and project outcome, however, it was not statistically significant. This could be justified by the fact that irrespective of the actors in the business environment, tech start-ups continue to practice their monitoring activities. The findings are also inconsistent with the findings of Krogstie et al. (Citation2017) who discovered that the degree of collaboration among project stakeholders as well as the project’s complexity and level of business environment unpredictability all had an impact on how successful monitoring practices were. Also, Joslin and Müller (Citation2016) discovered that elements including the degree of industry competitiveness, the state of technical development, and the regulatory environment had an impact on the efficacy of monitoring practices. Furthermore, Eroglu and Karaarslan (Citation2018), found that that the degree of market volatility, the amount of industry innovation, and the degree of competitiveness all had an impact on the efficacy of monitoring practices.
Finally, using the PLS-SEM and the simple slope analysis, the empirical results support our theoretical predictions that business environment moderate the relationship between evaluation practices and project outcome, however, the relationship was a dampening relationship. These results can be justified base on the fact that the dynamic nature of the environment plays a crucial role when it comes to tech start-ups executing their evaluation activities. Moreover the dynamic nature of the environment surrounding the implementation of evaluation practices was not favourable causing the weakening of the relationship. This assertion is supported by Eruemegbe et al. (Citation2015) who revealed that the dynamic nature of variables in the business environment is complex, as are their controls over the outcomes of events initiated inside an organization. Previous studies have also confirmed this findings, for instance, a study by Shehu and Shehu (Citation2015), also discovered that the association between project success and evaluation practices is moderated by the business environment. They discovered that whereas evaluation practices have no discernible impact on project performance in an adversarial and unstable business environment, they are favorably associated to it in a stable and supportive environment. Lee and Kim (Citation2018), further revealed that the relationship between project performance and evaluation practices is moderated by the business environment. Also, Belout and Gauvreau (Citation2004), discovered in another study that the association between project success and evaluation practices is moderated by the business environment.
4.4. Conclusion and recommendation
The study sought to provide answers to the effect that monitoring and evaluation practice have on tech start-ups project outcomes, and also assess the role that business environment play in the relationship between M&E and project outcomes. The study followed a positivist mind-set, relying only on quantitative methods and an explanatory research design. Primary data via structured questionnaire was obtained from 317 respondents in managerial positions in the tech industry and analysed using inferential and descriptive tools. It was found that M&E have a positive effect on tech firms’ project outcome, also the industry perceived that their M&E practices is not done within a favourable environmental factors. These empirical findings have validated existing theories from which the study’s hypothesis were derived. The findings also demonstrates\ the relevance of M&E practices and the need to take appropriate actions to improve it. It further informs management that the environment should be observed closely by keeping pace with relevant current information of the actors in the environment to reduce its negative impacts on the industry internal operations. Thus all environmental factors likely to impede the successful implementation of M&E systems should be closely monitored. The findings demonstrate that, tech start-ups should create policies that recognize the integration of M&E in their operations and business functions. The study was limited by the fact that there is no specific consideration of differences in geographical and industry in which tech start-ups belong to, thereby limiting the generalisation of findings to a particular geographical context and industry. It is therefore recommended that future studies should focus on start-ups in other sectors such as mining, health and construction among others.
Disclosure statement
No potential conflict of interest was reported by the authors.
Additional information
Notes on contributors
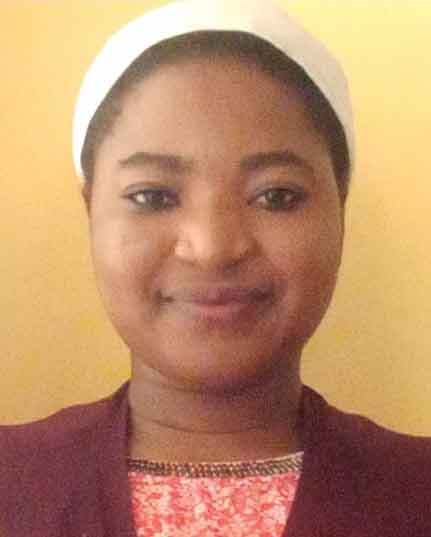
Ramatu Issifu
Ramatu Issifu is a Research Assistant at Foundation to Support Education, Networking, Skills Development, and Enterprise Start-up in Cape Coast, Ghana since 2021. She is a researcher with three articles to her credit. She holds an MPhil (Project Management) and MSc (Monitoring and Evaluation) from the University of Cape Coast. She is a Student Member of the Chartered Institute of Accountants (Ghana). She is a graduate of the prestigious BMGA Fellowship Programme for empowering young African Women. She has been involved in the following projects; Building Expertise and Training for Growth in the Consumer Goods and Food Processing Industry in Ghana, GIZ ComCashew, Switch Africa Green E-MAGIN, Building Bridges Across Continents, Applied Research and Teaching for Sustainable Development in Africa.
Daniel Agyapong
Daniel Agyapong, a Professor of Finance and Entrepreneurship, has worked at the University of Cape Coast (Ghana) since 2004. He is an SME trainer and researcher with 60+ articles on SME financing and sustainable business development. He holds a PhD and is an Associate Member of the Chartered Institute of Marketing (UK) and Institute of Professional Managers Association, UK. He has been involved in the following projects; African Institute of Transformational Entrepreneurship, Building Expertise and Training for Growth in the Consumer Goods and Food Processing Industry in Ghana, GIZ ComCashew, Switch Africa Green, E-MAGIN, Graduate Enterprise Development Initiative, Participatory Appraisals of Competitive Advantage, Building Bridges Across Continents, Partnership for Applied Sciences, Applied Research and Teaching for Sustainable Development in Africa and German-African University Partnership Platform for the Development of Entrepreneurs. He is a co-founder of the Foundation to Support Education, Networking, Skills Development, and Enterprise Start-up.
References
- Abebe, H. (2018). Assessing the effect of project monitoring and controlling practice on project success: In The Case of Ethiopian Airlines Digital Project Management Office. Available at: http://213.55.95.56/handle/123456789/17709
- Abisuga-Oyekunle, O. A., Patra, S. K., & Muchie, M. (2020). Smes in sustainable development: Their role in poverty reduction and employment generation in sub-saharan Africa. African Journal of Science, Technology, Innovation & Development, 12(4), 405–22. https://doi.org/10.1080/20421338.2019.1656428
- Aditjandra, P. T., Cao, X. J., & Mulley, C. (2012). Understanding neighbourhood design impact on travel behaviour: An application of structural equations model to a British metropolitan data. Transportation Research Part A: Policy and Practice, 46(1), 22–32. https://doi.org/10.1016/j.tra.2011.09.001
- African Development Bank. (2006). African development report 2006: Aid, debt relief and development in Africa. Oxford University Press.
- Agbenyo, L., Asamoah, D., & Agyei-Owusu, B. (2018). Drivers and effects of Inter-Organizational Systems (IOS) use in a sub-Saharan African country. Twenty-fourth Americas Conference on Information Systems, New Orleans.
- Agyei-Owusu, B., Asamoah, D., & Agbenyo, L. (2018). Examining the effects of information technology outsourcing on competitive advantage. Twenty-fourth Americas Conference on Information Systems, New Orleans.
- Ahuja, V., & Thiruvengadam, V. (2004). Project scheduling and monitoring: Current research status. Construction Innovation, 4(1), 19–31. https://doi.org/10.1108/14714170410814980
- Amedofu, M., Asamoah, D., & Agyei-Owusu, B. (2019). Effect of supply chain management practices on customer development and start-up performance. Benchmarking: An International Journal, 26(7), 2267–2285. https://doi.org/10.1108/BIJ-08-2018-0230
- Arbolino, R., Boffardi, R., Lanuzza, F., & Ioppolo, G. (2018). Monitoring and evaluation of regional industrial sustainability: Evidence from Italian regions. Land Use Policy, 75, 420–428. https://doi.org/10.1016/j.landusepol.2018.04.007
- Arce, R., Arias, E., Novo, M., & Fariña, F. (2020). Are interventions with batterers effective? A meta-analytical review. Psychosocial Intervention, 29(3), 153–164. https://doi.org/10.5093/pi2020a11
- Asamoah, D., Andoh-Baidoo, F. K., & Agyei-Owusu, B. (2015). Impact of ERP implementation on business process outcomes: A replication of a United States study in a sub-Saharan African Nation. AIS Transactions on Replication Research, 1(1), 4. https://doi.org/10.17705/1atrr.00004
- Ayalew, M. M., & Xianzhi, Z. (2020). The effect of financial constraints on innovation in developing countries: Evidence from 11 African countries. Asian Review of Accounting, 28(3), 273–308. https://doi.org/10.1108/ARA-02-2019-0036
- Bagozzi, R. P., & Yi, Y. (1988). On the evaluation of structural equation models. Journal of the Academy of Marketing Science, 16(1), 74–94. https://doi.org/10.1007/BF02723327
- Belout, A., & Gauvreau, C. (2004). Factors influencing project success: The impact of human resource management. International Journal of Project Management, 22(1), 1–11. https://doi.org/10.1016/S0263-7863(03)00003-6
- Blackwood, D. L., Pettit, K. L., Hager, M. A., Flanagan, E., & Scott, J. (2018). The impact of evaluation practices on project outcomes in nonprofit organizations. Nonprofit Management and Leadership, 28(3), 327–341.
- Blank, S. (2013). Why the lean start-up changes everything? Harvard Business Review, 91(5), 63–72.
- Candi, M., & Saemundsson, R. J. (2011). Exploring the relationship between aesthetic design as an element of new service development and performance. Journal of Product Innovation Management, 28(4), 536–557. https://doi.org/10.1111/j.1540-5885.2011.00827.x
- Chebet, W. K. (2021). Role of monitoring and evaluation in development of school infrastructure in Marakwet West Sub-County, Kenya (Doctoral dissertation, Moi University).
- Cherunilam, F. (2021). Business environment. Himalaya Publishing House Pvt. Ltd.
- Chin, W. W. (2010). How to write up and report PLS analyses. In Vincenzo, E., Vinz Wynne, W. C., Jörg, H., & Huiwen, W. (Eds), Handbook of partial least squares (pp. 655–690). Springer.
- Choi, D. S., Sung, C. S., & Park, J. Y. (2020). How does technology startups increase innovative performance? The study of technology startups on innovation focusing on employment change in Korea. Sustainability, 12(2), 551. https://doi.org/10.3390/su12020551
- Cohen, J. (1988). Statistical power analysis for the behavioural sciences. Laurence Erlbaum Associates.
- Cronbach, L. J., & Meehl, P. E. (1955). Construct validity in psychological tests. Psychological Bulletin, 52(4), 281. https://doi.org/10.1037/h0040957
- Cruz Villazón, C., Sastoque Pinilla, L., Otegi Olaso, J. R., Toledo Gandarias, N., & López de Lacalle, N. (2020). Identification of key performance indicators in project-based organisations through the lean approach. Sustainability, 12(15), 5977.
- Damoah, I., Akwei, C., & Mouzughi, Y. (2015). Causes of government project failure in developing countries. Proceedings of the Focus on Ghana British Academy of Management (BAM) Conference, Portsmouth University, Portsmouth, United Kingdom
- Dijkstra, T. K., & Henseler, J. (2015). Consistent partial least squares path modeling. MIS Quarterly, 39(2), 297–316. https://doi.org/10.25300/MISQ/2015/39.2.02
- Dvorský, J., Petráková, Z., Mezuláník, J., & Caha, Z. (2021). Impact of social environment indicators on students´ propensity to do business: Case study from central European countries. Journal of International Studies, 14(3), 205–219. https://doi.org/10.14254/2071-8330.2021/14-3/13
- Elahi, E., Khalid, Z., & Zhang, Z. (2022). Understanding farmers’ intention and willingness to install renewable energy technology: A solution to reduce the environmental emissions of agriculture. Applied Energy, 309, 118459. https://doi.org/10.1016/j.apenergy.2021.118459
- Eroglu, H., & Karaarslan, E. (2018). The moderating role of the business environment on the relationship between project monitoring and performance. International Journal of Project Management, 36(3), 449–463.
- Eruemegbe, G. O., Wanzek, J., & Yovanoff, P. (2015). Response to intervention. European Scientific Journal, 2015(Suppl 0), 260–264. https://doi.org/10.1177/0014402914532234
- Fornell, C., & Larcker, D. F. (1981). Evaluating structural equation models with unobservable variables and measurement error. Journal of Marketing Research, 18(1), 39–50. https://doi.org/10.1177/002224378101800104
- Fransisko, F. (2016). Implementation of project monitoring and evaluation to improve project effectiveness and efficiency. International Journal of Business and Commerce, 5(7), 18–34.
- Gomes, P. J. D. C. V. (2020). Project Success Evaluation of Xperts Council. [ Doctoral dissertation]. Universidade Catolica Portugesa (Portugal).
- Habibi, F., Birgani, O., Koppelaar, H., & Radenović, S. (2018). Using fuzzy logic to improve the project time and cost estimation based on project evaluation and Review technique (PERT). Journal of Project Management, 3(4), 183–196. https://doi.org/10.5267/j.jpm.2018.4.002
- Hair, J. F., Astrachan, C. B., Moisescu, O. I., Radomir, L., Sarstedt, M., Vaithilingam, S., & Ringle, C. M. (2021). Executing and interpreting applications of PLS-SEM: Updates for family business researchers. Journal of Family Business Strategy, 12(3), 100392. https://doi.org/10.1016/j.jfbs.2020.100392
- Hair, J. F., Henseler, J., Dijkstra, T. K., & Sarstedt, M. (2014). Common beliefs and reality about partial least squares: Comments on rönkkö and Evermann. Comments on Rönkkö and Evermann” Faculty Publications, 3666. https://digitalcommons.kennesaw.edu/facpubs/3666
- Hair, J. F., Risher, J. J., Sarstedt, M., & Ringle, C. M. (2019). When to use and how to report the results of PLS-SEM. European Business Review, 31(1), 2–24. https://doi.org/10.1108/EBR-11-2018-0203
- Hair, J. F., Jr., Sarstedt, M., Hopkins, L., Kuppelwieser, V. G., & Hair, J. F. (2014). Partial least squares structural equation modeling (PLS-SEM): An emerging tool in business research. European Business Review, 5(1), 105–115. https://doi.org/10.1016/j.jfbs.2014.01.002
- Hair, J. F., Jr., Sarstedt, M., Ringle, C. M., & Gudergan, S. P. (2017). Advanced issues in partial least squares structural equation modeling. SAGE publications.
- Hanaysha, J. (2016). Testing the effects of employee engagement, work environment, and organizational learning on organizational commitment. Procedia-Social and Behavioral Sciences, 229, 289–297. https://doi.org/10.1016/j.sbspro.2016.07.139
- Henseler, J. (2017). Partial least squares path modeling. In Advanced methods for modeling markets (pp. 361–381). Springer.
- Henseler, J., Ringle, C. M., & Sarstedt, M. (2015). A new criterion for assessing discriminant validity in variance-based structural equation modeling. Journal of the Academy of Marketing Science, 43(1), 115–135. https://doi.org/10.1007/s11747-014-0403-8
- Henseler, J., Ringle, C. M., & Sinkovics, R. R. (2009). The use of partial least squares path modelling in international marketing. In R. R. Sinkovics & P. N. Ghauri(Eds.), New Challenges to International Marketing (Advances in International Marketing (Vol. 20, pp. 277–319). Emerald Group Publishing Limited. https://doi.org/10.1108/S1474-7979(2009)0000020014
- Herman-Mercer, N., Antweiler, R., Wilson, N., Mutter, E., Toohey, R., & Schuster, P. (2018). Data quality from a community-based, water-quality monitoring project in the Yukon River basin. Citizen Science: Theory and Practice, 3(2), 3(2. https://doi.org/10.5334/cstp.123
- Huang, C. Y., Yang, C. L., & Wu, C. C. (2011). The impact of monitoring on software project outcome. Journal of Systems and Software, 84(10), 1710–1721.
- Jha, N. (2020). The better Africa report: Five out of every ten start-ups failed in Africa in the last ten years. Available at: How many African start-ups failed in the last Ten Years?. https://weetracker.com/2020/03/12/the-better-africa-report-startups-failed-in-africa/
- Johnson, N. B. (2018). Facilitating innovation in technology start-ups in Ghana – a multiple case study of the technology entrepreneurship ecosystem in Ghana. School of Social Sciences, Södertörn University.
- Joslin, R., & Müller, R. (2016). The impact of project methodologies on project success in different project environments. International Journal of Managing Projects in Business, 9(2), 364–388.
- Kabonga, I. (2018). Principles and practice of monitoring and evaluation: A paraphernalia for effective development. Africanus: Journal of Development Studies, 48(2), 1–21. https://doi.org/10.25159/0304-615X/3086
- Kahn, H. (2019). World economic development: 1979 and beyond. Routledge.
- Kamau, C. G., & Mohamed, H. B. (2015). Efficacy of monitoring and evaluation function in achieving project success in Kenya: A conceptual framework. Science Journal of Business and Management, 3(3), 82–94. https://doi.org/10.11648/j.sjbm.20150303.14
- Karim, A., Marnewick, C., & Kroeze, J. (2015). Project monitoring practices and project success: Evidence from the South African public sector. South African Journal of Business Management, 46(4), 25–36.
- Kihuha, P. (2018). Monitoring and Evaluation Practices and Performance of Global Environment Facility Projects in Kenya: A Case of United Nations Environment Programme. (Master’s dissertation, Kenyatta University).
- Kissi, E., Agyekum, K., Baiden, B. K., Tannor, R. A., Asamoah, G. E., & Andam, E. T. (2019). Impact of project monitoring and evaluation practices on construction project success criteria in Ghana. Built Environment Project and Asset Management, 9(3), 364–382. https://doi.org/10.1108/BEPAM-11-2018-0135
- Kodjokuma, R. (2018). Entrepreneurship And Development In Africa: The Role of Tech Start-Ups on Ghana’s Socioeconomic Development (Doctoral dissertation, University of Ghana).
- Krogstie, J. G., Jorgensen, G., & van Marrewijk, A. (2017). Business environment and project monitoring in construction projects. International Journal of Project Management, 35(8), 1642–1655. https://doi.org/10.1016/j.ijproman.2017.09.010
- Kusek, J. Z., & Rist, R. C. (2004). Ten Steps to a results-based monitoring and evaluation system: A handbook for development practitioners. World Bank Publications.
- Laursen, A. S. D., Dahm, C. C., Johnsen, S. P., Tjønneland, A., Overvad, K., & Jakobsen, M. U. (2018). Substitutions of dairy product intake and risk of stroke: A Danish cohort study. European Journal of Epidemiology, 33(2), 201–212. https://doi.org/10.1007/s10654-017-0271-x
- Lee, S., & Kim, S. (2018). The moderating effect of business environment on the relationship between evaluation practice and project performance. Sustainability, 10(10), 3437.
- Liu, K., Sun, Y., & Yang, D. (2023). The administrative center or economic center: Which dominates the regional Green development pattern? A case study of Shandong Peninsula Urban Agglomeration, China. Green and Low-Carbon Economy. https://doi.org/10.47852/bonviewGLCE3202955
- Luo, Y., & Bai, Y. (2021). Business model innovation of technical start-ups in emerging markets. Journal of Industrial Integration and Management, 6(3), 319–332. https://doi.org/10.1142/S2424862221500202
- Mansfield, M. (2019, March). Startup statistics – the numbers you need to know. Available at: https://smallbiztrends.com/2019/03/startup-statistics-small-business.html
- McCoy, M. (2005, March). Evaluating public relations’ effects: Implications from mass communication theory and research. In Chartered Institute of Public Relations Academic Conference. Lincoln, UK.
- Memon, M. A., Ramayah, T., Cheah, J. H., Ting, H., Chuah, F., & Cham, T. H. (2021). PLS-SEM statistical programs: A review. Journal of Applied Structural Equation Modeling, 5(1), 1–14. https://doi.org/10.47263/JASEM.5(1)06
- Mensah, A. O., Fobih, N., & Adom, Y. A. (2019). Entrepreneurship development and new business start-ups: Challenges and prospects for Ghanaian entrepreneurs. African Research Review, 13(3), 27–41. https://doi.org/10.4314/afrrev.v13i3.3
- Muchelule, Y., Iravo, M., Odhiambo, R., & Shalle, N. (2017). Effect of monitoring techniques on project performance of Kenyan state Corporations. European Scientific Journal, 13(19), 19. https://doi.org/10.19044/esj.2017.v13n19p264
- Mureithi, C. (2021). The biggest job-creating opportunity in South Africa, according to the World Bank. Available at: World Bank: South Africa’s digital sector could solve job crisis — Quartz Africa (qz.com).
- Ndabeni, L. L. (2008). The contribution of business incubators and technology stations to small enterprise development in South Africa. Development Southern Africa, 25(3), 259–268. https://doi.org/10.1080/03768350802212022
- Nega, S. (2020). The effect of project monitoring and controlling practice on project success: A case study of projects in Information Network Security Agency (INSA). Available at: http://213.55.95.56/handle/123456789/24366
- Ngeru, B. K., & Ngugi, P. K. (2019). Determinants of effective implementation of monitoring and evaluation systems in county governments in Kenya. International Academic Journal of Information Sciences and Project Management, 3(5), 12–37.
- Nunnally, J. C., & Bernstein, I. H. (1994). Psychometric theory 3E. Tata McGraw-hill education.
- OECD, O. (2004). The OECD principles of corporate governance. Contaduría y administración, (216), 216. https://doi.org/10.22201/fca.24488410e.2005.562
- Olaoye, O. (2023). Environmental quality, energy consumption and economic growth: Evidence from selected African countries. Green and Low-Carbon Economy. https://doi.org/10.47852/bonviewGLCE3202802
- Olejniczak, K., Kupiec, T., & Newcomer, K. (2017). Learning from evaluation–the knowledge users’ perspective”. Evaluation Theory and Practice, 5(2), 49–74.
- Oliveros-Romero, J., & Aibinu, A. A. (2019). Ex post impact evaluation of PPP projects: An exploratory research. Built Environment Project and Asset Management, 9(2), 315–330. https://doi.org/10.1108/BEPAM-01-2018-0036
- Otieno, F. A. O. (2000, November). The roles of monitoring and evaluation in projects. Proceedings of the 2nd International Conference on Construction in Developing Countries: Challenges facing the construction industry in developing countries, Gaborone, Botswana (pp. 15–17).
- Owualah, S. L. (1999). Tackling youth unemployment through entrepreneurship. International Small Business Journal, 17(3), 49–59. https://doi.org/10.1177/0266242699173003
- Pallant, J., & Manual, S. S. (2007). A step by step guide to data analysis using SPSS for windows. SPSS Survival Manual, 14(4), 20–30.
- Piccarozzi, M. (2017). Does social innovation contribute to sustainability? The case of Italian innovative start-ups. Sustainability, 9(12), 2376. https://doi.org/10.3390/su9122376
- Polishchuk, V., Kelemen, M., Gavurová, B., Varotsos, C., Andoga, R., Gera, M., Christodoulakis, J., Soušek, R., Kozuba, J., Blišťan, P., & Szabo, S. (2019). A fuzzy model of risk assessment for environmental start-up projects in the air transport sector. International Journal of Environmental Research and Public Health, 16(19), 3573. https://doi.org/10.3390/ijerph16193573
- Pullin, A. S., & Knight, T. M. (2003). Support for decision making in conservation practice: An evidence-based approach. Journal for Nature Conservation, 11(2), 83–90. https://doi.org/10.1078/1617-1381-00040
- Ries, E. (2011). The lean startup: How today’s entrepreneurs use continuous innovation to create radically successful businesses. Currency.
- Ringle, C. M., Sarstedt, M., & Straub, D. W. (2012). Editor’s comments: A critical look at the use of PLS-SEM in“MIS Quarterly”. MIS Quarterly, 36(1), iii–xiv. https://doi.org/10.2307/41410402
- Ripsas, S., & Tröger, S. (2014). Deutscher Startup Monitor 2014. KPMG, Berlin.
- Roldán, J. L., & Sánchez-Franco, M. J. (2012). Variance-based structural equation modeling: Guidelines for using partial least squares in information systems research. In M., Mora, O., Gelman, A., Steenkamp, & M., Raisinghani (Eds.), Research methodologies, innovations and philosophies in software systems engineering and information systems (pp. 193–221). IGI global.
- Rossi, P. H., Lipsey, M. W., & Henry, G. T. (2018). Evaluation: A systematic approach. Sage publications.
- Sasu, D. D. (2022, March). Number of funded tech startups in Ghana from 2019 to 2021. Available at: https://www.statista.com/statistics/1299370/number-of-funded-tech-startups-in-ghana/
- Shapiro, J. (2007). Monitoring and evaluation. World Alliance for Citizen Participation.
- Shehu, A. M., & Shehu, R. (2015). The moderating role of business environment in the relationship between entrepreneurial orientation and business performance among Nigerian SMEs. Jurnal Pengurusan, 43(2015), 119–128.
- Sherman, M. H., & Ford, J. (2014). Stakeholder engagement in adaptation interventions: An evaluation of projects in developing nations. Climate Policy, 14(3), 417–441. https://doi.org/10.1080/14693062.2014.859501
- Tengan, C., & Aigbavboa, C. (2017). Level of stakeholder engagement and participation in monitoring and evaluation of construction projects in Ghana. Procedia Engineering, 196, 630–637. https://doi.org/10.1016/j.proeng.2017.08.051
- Thaddee, B., Prudence, N., & Valens, S. (2020). Influence of project management practices on project success in Rwanda-the case of Girinka project in Runda sector, Kamonyi district, Rwanda. European Journal of Management and Marketing Studies, 5(3).
- Thomson, D., Brooks, S., Nuspl, M., & Hartling, L. (2019). Programme theory development and formative evaluation of a provincial knowledge translation unit. Health Research Policy and Systems, 17(1), 1–9. https://doi.org/10.1186/s12961-019-0437-y
- Tullock, Z. (2010). Capital constraint to entrepreneurial start-ups in South Africa’s emerging agribusiness industry (Doctoral dissertation, University of Pretoria).
- Turner, J. R., & Müller, R. (2005). The project manager’s leadership style as a success factor on projects: A literature review. Project Management Journal, 36(2), 49–61. https://doi.org/10.1177/875697280503600206
- Tyulin, A., & Chursin, A. (2020). The New economy of the product life cycle. Springer Books.
- Uzunkaya, M. (2017). Theory-based evaluation of Public–Private Partnership projects and grogrammes. In J. Leitão, E. de Morais Sarmento, & J. Aleluia(Eds.), The Emerald Handbook of Public–Private Partnerships in Developing and Emerging Economies (pp. 579–604). Emerald Publishing Limited. https://doi.org/10.1108/978-1-78714-493-420171022
- Voorhees, C. M., Brady, M. K., Calantone, R., & Ramirez, E. (2016). Discriminant validity testing in marketing: An analysis, causes for concern, and proposed remedies. Journal of the Academy of Marketing Science, 44(1), 119–134. https://doi.org/10.1007/s11747-015-0455-4
- Weiss, C. H. (1972). Evaluation research: Methods for assessing program effectiveness. Prentice-Hall.
- Wetzels, M., Odekerken-Schröder, G., & Van Oppen, C. (2009). Using PLS path modeling for assessing hierarchical construct models: Guidelines and empirical illustration. MIS Quarterly, 33(1), 177–195. https://doi.org/10.2307/20650284
- Wong, S. C., Lim, J. Y., Lim, C. S., & Hong, K. T. (2019). An empirical study on career choices among undergraduates: A PLS-SEM hierarchical component model (HCM) approach. International Journal of Human Resource Studies, 9(2), 276–298. https://doi.org/10.5296/ijhrs.v9i2.14841
- Young, R., Chen, W., Quazi, A., Parry, W., Wong, A., & Poon, S. K. (2019). The relationship between project governance mechanisms and project success: An international data set. International Journal of Managing Projects in Business, 13(7), 1496–1521. https://doi.org/10.1108/IJMPB-10-2018-0212
- Yousefi, N., Sobhani, A., Naeni, L. M., & Currie, K. R. (2019). Using statistical control charts to monitor duration-based performance of project. Journal of Modern Project Management, 6(3), 1–26.
- Zhang, Y., & Yang, R. (2018). Evaluation-driven project management: A model for project success. International Journal of Project Management, 36(1), 1–12.