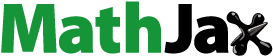
Abstract
Despite the general increase in remittance inflows in Kenya over the last four decades, it remains unknown as to whether part of these remittances forms an integral portion of productive investment in the aggregate economy. We, therefore, examined the impact of foreign remittances on Gross Fixed Capital Formation (GFCF) in Kenya using the ARDL model and time-series data for the period 1980–2020. We also sought to establish the direction of causality between the two variables and whether structural breaks mattered in modeling the remittances-GFCF relationship. First, we found that while remittances significantly reduced GFCF in the short run, their impact was insignificant in the long run. Secondly, the Granger Causality test showed evidence of a uni-directional causality running from remittances to GFCF. Thirdly, structural breaks significantly and positively moderated the remittances-GFCF nexus in Kenya; highlighting the importance of testing for structural breaks when analyzing a remittance-GFCF model. To promote remittance inflows in Kenya, there is a need to develop a robust financial system to facilitate the optimal harnessing of investment benefits accruing from such remittances. Further, and owing to the negative impact of remittances on investment in the short run; remittance-receiving households need to be sensitized on the productive use of remittances to ensure that they are forward-looking and, thus, use their remittances on productive investments as envisaged by pure self-interest and tempered altruistic remittance motives.
1. Introduction
International remittances are those goods and/or money that are sent to left-behind households by migrants working abroad (Adams & Cuecuecha, Citation2010). Globally, remittances have been on a rising trend; increasing from about USD 3 billion in 1975 to about USD 689 billion in 2018 (World Bank, Citation2018). These remittance inflows to the developing economies now constitute one of the largest prime sources of external finance surpassing other external finance sources i.e., Official Development Assistance (ODA) and Foreign Direct Investment (FDI). For instance, remittance inflows amongst Low-Income Countries (LICs) represented 6.06% of the Gross Domestic Product (GDP) in 2017 while FDI accounted for 3.2% of GDP over the same period. Similarly, among the Low Middle-Income Countries (LMICs), remittance inflows accounted for 4.09% of GDP against 1.9% of the GDP contributed by FDI over the same period. This reveals the increasing trend of remittances and highlights their significance as a prime foreign source of financial flows for many LICs globally.
Further, the World Bank (Citation2018) observed that remittance inflows to developing countries amounted to about USD 529 billion in 2018, with countries in East Africa receiving a total of USD 17.38 billion in remittances between 2013 and 2018. More importantly, Kenya tops the region as the largest beneficiary with a receipt of 60% of all the remittances in the East Africa region & about 10% of all the remittances received by the sub-Saharan African (SSA) countries (World Bank, Citation2019). Similar statistics by the World Bank (Citation2019) also ranked Kenya in the 3rd position amongst the top ten remittance-receiving countries in the SSA region with its remittances amounting to about 2.72 billion USD in 2018. This figure represented about 3.1% of its Gross Domestic Product (GDP) over the same period. According to statistics from the World Bank (Citation2020), remittance inflows to Kenya have, thus, tremendously increased from approximately USD 934.14 million recorded in 2011 to about USD 2.84 billion in 2019. This has been occasioned by increased migration which saw a steady increase of remittances at a rate of 15.8% annually over the last decade.
The upsurge in remittance inflows has been associated with positive effects such as poverty reduction (Ahmed et al., Citation2010), economic growth (Cooray, Citation2012; Fayissa & Nsiah, Citation2010), development of financial systems (Chowdhury, Citation2016; Misati et al., Citation2019), and human capital development (Hines & Simpson, Citation2019). Nevertheless, literature remains silent on whether part of these remittances forms an integral portion of productive investment in the overall economy. This study, therefore, contributes to the literature by first exploring the impact of foreign remittances on Gross Fixed Capital Formation (GFCF) in Kenya using the ARDL model approach and time series data for the period 1980–2020. Secondly, the prevalence of sharp swings in the trend of GFCF and remittance inflows for Kenya over the study period (see Figures ) motivates us to investigate whether structural breaks played a significant role in moderating the remittances-GFCF nexus. Thirdly, and vital for policy direction, we also determine the direction of causality between the two variables in Kenya.
Theoretically, Lucas and Stark (Citation1985) point out three major motivations behind the decision by migrant workers to remit to their left-behind households or family members. These include pure altruism, pure self-interest & tempered altruism. More implicitly, the pure self-interest motivation posits that migrants target their remittances towards investment in fixed capital intending to return home at some later point in time to claim ownership over those investments.
Despite the presence of a vast array of literature on remittances and their impact on poverty decrease, economic growth, and financial and human capital development; a significant gap exists as far as exploring the time series linkage between foreign remittances and investment or Gross Fixed Capital Formation (GFCF) in the Kenyan context is concerned. Most studies have analyzed expenditure patterns following the receipt of remittances at household levels (Adams & Cuecuecha, Citation2013; Ahamada & Coulibaly, Citation2013; Ajefu & Ogebe, Citation2020). As a result, investment decisions have been modeled as binary at micro-levels. It is, therefore, uncertain as to whether the increase in remittance inflows has a long-run relationship with investment in Kenya. Further, with the majority of empirical studies associating remittances with household consumption (see Adams & Cuecuecha, Citation2010; Edwards & Ureta, Citation2003; Yang, Citation2011), it remains unknown as to whether part of these remittances forms an integral portion of productive investment in the overall economy. This implies that the foreign remittances’ influence on domestic investment in Kenya at the macro-level remains relatively unknown and inconclusive.
Conversely, at a macro level, the impact of foreign remittances on investment has only been analyzed at panel levels by generally using the Generalized Method of Moments (GMM) and/or the panel fixed effects estimator with the Instrumental Variable (IV) approach being employed to control for the endogeneity issues. This study, therefore, adds novelty to the literature by first exploring the impact of foreign remittances on GFCF in Kenya using the ARDL model approach for the period 1980–2020. Secondly, the prevalence of sharp swings in the trend of GFCF and remittance inflows for Kenya over the study period (see Figures ) drives us to investigate the role of structural breaks in informing remittances-GFCF nexus for Kenya. Thirdly, we also sought to determine the direction of causality between remittances and GFCF in Kenya.
At the African continental level and more particularly in the SSA region, there has been an upward trend in remittance inflows to the region since 2016. This has largely been explained by the robust economic conditions in the high-income countries where many SSA migrants earn their income (Migration and Development, Citation2019). According to the World Bank (Citation2019) estimates, remittances to the SSA region grew by 9.6% from USD 42 billion in 2017 to USD 46 billion in 2018 with Nigeria being the largest remittance-recipient country with a receipt of about USD 24.3 billion in 2018. Kenya is ranked 3rd in the top ten countries in SSA with remittance inflows of about 2.7 billion USD over the same period (see Figure ).
Figure 1. Top 10 remittance-recipient countries in the SSA region in 2018 (in billion USD).
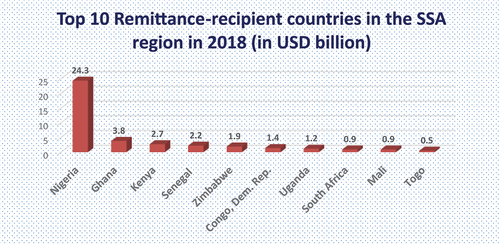
The general trend in Kenya also reveals an increase in remittance inflows since 1980. This is notwithstanding the sharp inflow swings recorded between the periods 1996–2002 (see Figure ).
Figure 2. Kenya’s personal remittance inflows as a ratio of GDP: 1980–2020.
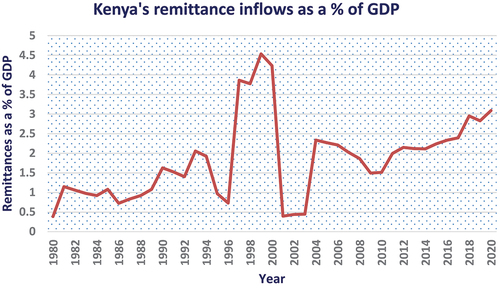
The remittances-inflow trend in Figure also seems to suggest that structural breaks might have indeed played a significant moderating role in influencing gross fixed capital formation in Kenya.
We equally present the trend in GFCF as a proportion of GDP in Kenya for the period (1980–2020) in an attempt to unravel the link between the two variables (see Figure ).
Figure 3. Kenya’s Gross fixed capital Formation as a ratio of GDP: 1980–2020).
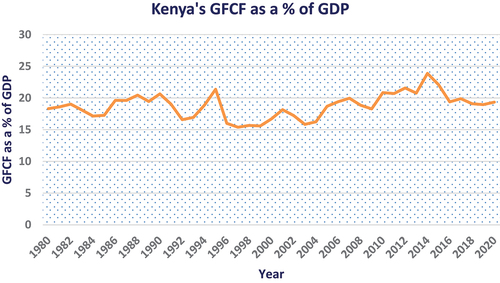
Figure also depicts an increasing trend in GFCF in Kenya over the last four decades. While the statistics presented in Figures illustrate the rising trend in remittance inflows in Kenya, it is still unclear as to whether these remittances impact GFCF in Kenya at the macro level. This study, therefore, contributes to the literature by examining whether foreign remittances are only instrumental for self-consumption at the household level or equally form part of a significant contribution to domestic investment at an aggregate level.
The ARDL model results revealed that while remittances significantly reduced GFCF in the short run, their impact was positive but insignificant in the long run. These results tend to insinuate that remittances may not provide the much-needed panacea to optimizing aggregate investment in the economy. Further, it seemingly suggests that remittances may be largely instrumental in only offsetting expenditures at the household consumption level with little or none of it being devoted to productive investment in the economy. Secondly, we found that structural breaks indeed played a significant role in moderating the impact of remittances on GFCF. Finally, the Granger Causality test showed evidence of a uni-directional causality between the two variables. This causality was found to run from remittances to GFCF.
The rest of the paper is structured as follows: Section two reviews the existing literature while section three discusses the methodology, data & variables used. Section four discusses the empirical findings while section five concludes the paper and provides policy implications based on the research findings.
2. Literature review
Various theoretical explanations have been provided on what drives or motivates foreign remittances. The theoretical justifications tend to be synonymous and/or intertwined at both the micro and macro levels. According to Lucas and Stark (Citation1985), there are three major motivations behind the decision by migrant workers to remit to their left-behind households or family members. These include pure altruism, pure self-interest & tempered altruism. Docquier and Rapoport (Citation2012) consider migrant workers as altruistic if the increase in remittances is accompanied by a rise in wages but with a wane in household income in their home country prior to receiving any remittances.
Concerning pure self-interest motivation, migrants target their remittances towards investment in fixed capital intending to return home at some later point in time with a view of claiming ownership over the same investments. Under this motive, foreign remittances may also be targeted by the migrant towards acquiring public assets to augment their political influence or prestige (Lucas & Stark, Citation1985). In contrast, Lucas and Stark (Citation1985) explain tempered altruism as a modality of risk-sharing or as a valuable investment meant to access or generate higher earnings streams. As a result, these remittances are termed as a self-enforcing contractual agreement between the migrant and the households left behind in the home country.
Generally, notable empirical studies lend credence to the three hypotheses as remittances motivating factors (Agarwal & Horowitz, Citation2002; Arun & Alku, Citation2010; Yang & Choi, Citation2007). Conversely, some studies derive the migrant’s motivation to remit from either one or a combination of the three motives (Brown & Poirine, Citation2005; Gubert, Citation2002; Osili, Citation2007). Further to these motivations, Hines and Simpson (Citation2019) allude that migration poses contending effects on household resources, that is, while households lose income when a wage earner migrates; this effect is counteracted when the migrant worker remits to their left-behind households. This study seeks to evaluate the investment decisions from the receipt of such remittances.
According to Adams and Cuecuecha (Citation2010, Citation2013), there is a positive link between the receipt of remittance and investment in health, education and housing in Ghana and Guatemala. Yang (Citation2011) observed that the decision on whether to use remittances for consumption or investment depended on a household’s income level. Whereas poor households are more likely to spend on food, health and durable goods, more affluent households can afford to invest in productive physical or human capital goods. The study by Jena (Citation2018) found a significant and positive impact of remittances on physical capital investment in Kenya. Using the 2009 Kenya Migration Household Survey data, Hines and Simpson (Citation2019) explored the relationship between remittances & human capital investment using both the Ordinary Least Squares (OLS) & the IV estimation techniques. The study findings revealed a significant & positive association between remittances and education spending in Kenya.
Remittances-receiving households have a high probability of investing in productive investments, i.e., housing, land, education, and other entrepreneurial income-generating activities (Edwards & Ureta, Citation2003; Osili, Citation2004; Woodruff & Zenteno, Citation2007; Yang, Citation2008). Conversely, Lopez-Cordova (Citation2005) found mixed results when analyzing the effects of foreign remittances on education and health in Mexico.
Several studies posit that the impact of remittances on investment is conditional on a given home country’s level of financial and institutional advancement. For instance, Aggarwal et al. (Citation2011) investigated the effect of remittances on financial development using the GMM approach. The study covered 99 developing countries and was conducted for the period 1975–2003. The findings showed that an enhanced institutional framework increased deposits & bank credits in the native or home banking system. Similarly, using data from 79 developing economies over the period 1995 to 2005, Dzansi (Citation2013) found complementarity evidence amongst the two variables in enabling investment advancement.
According to Gani (Citation2016), remittances first increase domestic savings which in turn increases domestic investment in the home country. Therefore, remittances are an integral household income source for many LICs (Edwards & Ureta, Citation2003). Subsequently, higher household income level is customarily linked with rising saving rates in less developed economies (Sahoo & Dash, Citation2013). Remittances also provide a vital foreign exchange that can be employed in the import of essential intermediate & capital commodities. This plays a pivotal role in fostering domestic investment in less developed economies (Chami et al., Citation2008). However, Ahamada and Coulibaly (Citation2013) reiterate that remittances can lessen domestic investment if they are extensively employed for consumption purposes rather than funding physical and human capital investments.
Dash (Citation2020) used the GMM approach to examine the influence of remittances on domestic investment in South Asia for the period 1991 to 2017. The findings revealed that remittances increased domestic investment both in the short run and in the long run. This implied that remittances were used for both consumption and investment activities i.e., human & physical capital advancement. Moreover, the panel causality results suggested the existence of uni-directional causality running from remittances to investments.
In investigating the impact of foreign remittances on expenditure patterns of left-behind households in the SSA region, Ajefu and Ogebe (Citation2020) analyzed both the aggregate and distributional effects using the IV-quantile regression model. The international remittances variable was instrumented by the economic conditions in migrants’ home countries. The results revealed that remittances increased expenditures on food, durables, education, and health. Similarly, the estimates from the IV-quantile regression model showed that the impact of international remittances on household expenditure on the aforementioned indicators increased across the different expenditure quantiles.
From the reviewed literature, it is apparent that the majority of the previous studies have analyzed the relationship between international remittances and household expenditure decisions. While many of these studies have explored the investment decisions by the remittance-receiving households, the analysis has rather been cross-sectional or panel and evaluated at the micro-level. Further, most of them have employed the OLS, panel fixed effects, the GMM, or the IV estimation techniques (see Adams & Cuecuecha, Citation2013; Ajefu & Ogebe, Citation2020; Hines & Simpson, Citation2019; Jena, Citation2018).
Further, studies at the macro-level tend to pay much focus on the remittances-economic growth nexus (see Rehman et al., Citation2021 for a sample of Western Balkan countries; Zardoub & Sboui, Citation2021 for selected developing countries). The other strand of literature analyzes the impact of remittances on financial system development (Chowdhury, Citation2016; Misati et al., Citation2019), human capital development (Hines & Simpson, Citation2019), and poverty reduction (Ahmed et al., Citation2010). It, thus, remains unknown as to whether remittances impact investment in Kenya at an aggregate level. This study, therefore, departs from the rest in three ways. First, we seek to explore the impact of international remittances on Gross Fixed Capital Formation (GFCF) in Kenya. Unlike the investment decisions analyzed at household levels in previous studies, GFCF is a broader investment concept and will be analyzed at the macro level using time series data. Secondly, and considering the prevalence of sharp swings in the trend of GFCF and remittance inflows for Kenya over the study period, we are also interested in establishing whether structural breaks play a significant role in moderating the remittances-GFCF nexus. Moreover, the present study unravels the direction of causality between remittances and GFCF, a feat that also remains relatively unexplored.
3. Methodology
3.1. Theoretical framework
Following Lucas (Citation1967) and Fry (Citation1993), we employ the flexible accelerator theory in tracing out the theoretical background on GFCF/investment determination. The flexible accelerator model provides for the desired or preferred level of capital stock that is proportional to real output
as follows:
We can express Equationequation (1)(1)
(1) in terms of the preferred ratio of investment to output
as follows:
Where and
represent GDP and the gross domestic fixed investment in current prices respectively. On the other hand,
denotes the growth rate of real GDP. This adjustment mechanism permits the partial adjustment of the actual investment rate in any one given period to the difference between the preferred investment rate and the previous period’s rate of investment. This is illustrated in Equationequation (3)
(3)
(3) .
We can rearrange Equationequation (3)(3)
(3) and rewrite it as follows:
Where is the adjustment coefficient and is influenced by various economic factors as provided for by the flexible accelerator model. For simplicity,
can be expressed as follows:
Where denotes the explanatory variables that influence investment and includes the intercept term for a constant rate of depreciation.
are the estimable parameters. We consider the investment as a partial adjustment process (that is, we adjust investment between the current & the desired level of capital stock) from the perspective of both liquidity & time adjustment constraints. Given that the rate of investment is a structural constituent of any given economy, it is expected that the lagged coefficient depicts a high persistence that corresponds to that of an autoregressive behavior.
3.2. Empirical Model specification
This paper sought to examine the impact of foreign remittances on Gross Fixed Capital Formation (GFCF) in Kenya for the period 1980–2020. Therefore, the remittance inflow (REM) in Kenya as a ratio to GDP is our main explanatory variable. The other control variables employed in this study include GDP per capita (GDP), Exchange rate (ER) against the USD, Inflation rate (INF), lending rate (LR), trade as a % of GDP (TRD), the Net Official Development Assistance as a % of GDP (ODA), and Financial Development (FD). We, thus, specified the following model:
Where is the error term, t denotes the time trend &
is the natural logarithm. This study employed the ARDL model where the dependent variable (GFCF) is expressed as a function of its lagged values and the current and lagged values of the exogenous variables. The ARDL model assumes no autocorrelation of the error terms, no heteroskedasticity, normal distribution of data and stationarity of variables at I (0) or I (1), or a combination of both. A generalized ARDL model takes the following form:
Whereas ,
are the short-run dynamic coefficients of the model’s Adjustment long-run equilibrium while p and q are the optimal lag orders for the dependent & exogenous variables respectively. The
are the long-run coefficients. In Equationequation (7)
(7)
(7) , the short-run equations have the differenced operator (
).
In modeling the ARDL Bounds testing procedure as developed by Pesaran and Shin (Citation1999) & Pesaran et al. (Citation2001), the null hypothesis postulates that there is No Cointegration. If the F statistic is found to be lower than the I (1) upper bound critical values, we fail to reject the null hypothesis of No Cointegration. In the absence of Cointegration, the short-run (ARDL) model is specified and estimated (see equation 8).
In contrast, if the F statistic is found to be greater than the I (1) upper bound critical values; we reject the null hypothesis of No Cointegration implying that Cointegration does exist. We, thus, model the long-run relationship and consequently, estimate the Error Correction Model (ECM). Further, we generate the structural break dates using the Zivot and Andrews (Citation1992) unit root test and consequently incorporate the structural break dummy variable in our model (see simplified equation 9).
Where X and Z are vectors of the short-run and long-run coefficients respectively. SBD is the generated structural break dummy variable. is the speed of adjustment parameter with a negative sign & denotes model convergence in the long run while
is the Error Correction Term.
This study also sought to ascertain the direction of causality between foreign remittances (REM) and Gross Fixed Capital Formation (GFCF). We employ the Engle and Granger (Citation1987) causality test to establish the causality direction between REM & GFCF in Kenya. This can be illustrated as follows:
The direction of causality may take two forms: Unidirectional (one-way) or bidirectional (two-way) causality.
The ARDL model specification enables us to determine whether the international remittance inflows impact investment in the short-run or the long-run or both. As such, the most salient policies would either be geared towards strengthening the financial system or household sensitization on the use of remittances. On the other hand, the Granger causality test is indeed imperative in policy direction as far as effective utilization of remittances is concerned.
3.3. Variable description, measurement, and hypothesized relationships
3.3.1. Gross fixed capital formation as a ratio of GDP GFCF
GFCF (formerly referred to as gross domestic fixed investment) broadly encompasses land improvements, plant, machinery and equipment purchases; and the construction of roads, railways, and the like, including schools, offices, hospitals, private residential dwellings, and commercial and industrial buildings. It equally includes net acquisitions of valuables. It is our dependent variable and is expressed as a proportion of the GDP.
3.3.2. Remittances as a ratio of GDP (REM)
The ratio to GDP of all those goods and/or money that is sent to the left-behind households by migrants working abroad. Remittances are expected to positively influence domestic investment as they help relax household expenditures and, thus, consequently stimulate investment in the economy (Aggarwal et al., Citation2011; Dash, Citation2020; Dzansi, Citation2013).
3.3.3. GDP per capital (GDP)
The total economic output per individual, obtained by dividing Kenya’s annual GDP by its population. It’s used as a proxy for the GDP growth rate. Theoretically, a higher GDP per capita increases private investment activity. However, this is conditioned on the relationship between the level of real output and the desired capital stock being relatively fixed (Greene & Villanueva, Citation1991). Further, Ali et al. (Citation2013) found a significant and positive impact of GDP per capita on investment. In our study, we measured GDP per capita in United States Dollars (USD) million but expressed it in a natural log in our model.
3.3.4. Exchange rate (ER)
The rate at which one currency exchanges for another. The Kenya Shilling is expressed in terms of the USD. It is logarithmically transformed in our model. The variable is expected to be inversely related to investment since an exchange rate decrease (appreciation) stimulates investment through higher capital inflows (Agrawal, Citation2000; Chami et al., Citation2008; Dash, Citation2020).
3.3.5. Inflation rate (INF)
The persistent increase in the general price levels of commodities in the economy. It’s expressed in percentage form. High inflation uncertainty lowers investment prospects since firms tend to employ more working capital than fixed assets (Fischer, Citation2013). However, some studies find a significant & positive impact of the inflation rate on investment (see. Pindyck & Solimano, Citation1993).
3.3.6. Lending rate (LR)
The average rate at which commercial banks lend out money to their credit-worthy clients. It acts as a proxy for the cost of capital. The higher the rate, the lower the amount of money that individuals or firms can borrow and this stifles their investment prospects (Mody & Murshid, Citation2005).
3.3.7. Trade as a % of GDP (TRD)
Higher trade activities increase investment. This is realized through increased human & physical capital which generates more savings & technological transfer enhancement. This is as envisioned by Nowak-Lehmann (Citation2003).
3.3.8. Net official development assistance (ODA)
Refers to the net funding provided by public entities in the most developed countries to the developing countries and is aimed at promoting the economic development & welfare of citizens in those recipient countries. It excludes humanitarian aid, non-concessional loans & aid provided by Non-Governmental Organizations (NGOs). Its hypothesized relationship with investment is indeterminate.
3.3.9. Financial development (FD)
Relates to the increase in efficiency and stability of financial markets, instruments, and intermediaries and is commonly realized through reduced information asymmetry. In this study, financial development is measured by domestic credit to the private sector as a ratio to the GDP. Higher levels of financial development are expected to spur investment in an economy (see Balcilar et al., Citation2016; Hassan, Citation2015).
3.4. Data
This study employed time series data for the period 1980–2020 in examining the impact of foreign remittances on GFCF in Kenya. We chose this study period since it captures both periods of pre-development and post-development financial systems in Kenya. Equally, and given the structural changes in the Kenyan economy over the period; we are able to integrate structural breaks in our analysis. Data on GFCF, remittance inflows, GDP per capita, trade as a % of GDP, Net ODA, financial development, and the lending rate were obtained from World Bank Development Indicators. Data on the inflation rate and exchange rate were obtained from the Central Bank of Kenya (CBK) Statistics database.
3.5. Summary statistics
These are shown in Table .
Table 1. Summary statistics (N = 41, T = 1980–2020)
The Gross Fixed Capital Formation (GFCF) as a proportion of the Kenyan GDP averaged 18.78%. GFCF also termed as investment, therefore, considerably contributes to the Kenyan GDP with the highest fraction reported to be 23.88% and the lowest at 15.39% over the study period. Remittance inflows as a ratio of Kenya’s GDP averaged 1.83% with the minimum and maximum ratios recorded at 0.38% and 4.54% respectively. The small ratios tend to suggest that while remittances may yield a substantive effect on household consumption; the same may not necessarily hold for aggregate investment in the economy. The mean GDP per capita for Kenya is about USD 741.04. The variable had a standard deviation of 446.62 and varied within the intervals of USD 226.52 and USD 1970.11 around the mean value. GDP per capita is an indicator of the living standards of a country. A higher GDP per capita implies improved living standards and this is expected to be reflected through increased investment capacity in the economy. Over the study period, the Kenya shilling (KES) averagely exchanged for the USD at KES. 59.19. The highest rate was reported at 106.45. A strong domestic currency (currency appreciation) triggers high capital inflows into the country, hence providing ample ground for domestic investment. The rate of inflation in Kenya over the study period averaged 11.65% and varied within the intervals of 1.55% and 45.98% around the mean value. The mean lending rate in Kenya is about 17.97%, with the lowest rate charged by commercial banks for their loans being 10.58% and the highest rate being 36.24%. A higher lending rate implies constrained credit to the borrowers and this has a potential negative impact on investment. Trade as a ratio of GDP averaged 52.84%. This considerable rate suggests high trade flows in Kenya. This has the potential impact of stimulating investment through technological transfer and human & physical capital inflows. The Net Official Development Assistance (ODA) to Kenya as a proportion of GDP averaged 0.21%. ODA supplements remittances and is, thus, expected to positively influence investment. In this study, we proxied financial development by domestic credit to the private sector as a ratio of the GDP. With vast literature suggesting that remittance inflows are conditioned by the level of financial development in the recipient country; financial development becomes imperative in moderating the effect of remittances on investment. The domestic credit to private sector ratio averaged 24.57% and varied within the intervals of 18.42% and 36.70%. These rates suggest that Kenya’s financial system is moderately developed. A developed financial system is vital as it provides ample ground for the receipt of remittances and the subsequent channeling of the same to productive investments in the economy.
4. Empirical findings
4.1. Pre-estimation tests
4.1.1. Unit root test
We test for the order of integration of each variable in the model to avoid potential spurious and/or inconsistent regression. In this study, we use the Zivot and Andrews (Citation1992) unit root test. We choose this test over other tests such as the Augmented Dickey-Fuller (ADF) and Phillips-Perron (PP) test since it effectively captures the structural breaks. The latter tests have been identified as being asymptotically equivalent with less predictive power (Pesaran, Citation2015; Zivot & Andrews, Citation1992), Further, they do fail to reject the invalid null hypothesis in cases where the coefficient in AR (1) process is close to one.
We predict the presence of structural break(s) in our model given the sharp upward and downward trends in both the GFCF and the remittances variables across various points over the study period. Structural break(s) usually generate an unexpected or abrupt change in the time-series variables hence causing a shift in the mean or other parameters in the series. This, as observed by Ndirangu et al. (Citation2014), may yield incorrect forecasts. We used the Zivot-Andrews unit root test which yielded the following results (see Table ).
Table 2. Zivot-Andrews unit root test results
The unit root test results (shown in Table ) revealed that the variables were integrated with a mixture of order 0 and 1; hence, the ARDL model became applicable (Pesaran & Shin, Citation1999; Pesaran et al., Citation2001). We, thus, employed the ARDL model to analyze the impact of international remittances on GFCF in Kenya. The model is advantageous since it does not require all variables to be integrated in the same order. It is also efficient in cases where small & finite sample data sizes are involved. Moreover, according to Harris and Sollis (Citation2003), the model yields unbiased long-run estimates.
4.1.2. ARDL Bounds Test
Since the results in Table revealed that the variables were integrated of a mixture of order 1 & 0, we employed the ARDL bounds testing procedure to test for the existence of cointegration among the variables (Pesaran & Shin, Citation1999; Pesaran et al., Citation2001) (see Table for the results).
Table 3. ARDL Bounds test results
The results in Table showed that the F statistic was greater than the I (1) upper bound critical values; hence, we rejected the null hypothesis of No Cointegration. This meant that Cointegration was present. We, thus, fitted an Error Correction Model (ECM) within the ARDL framework to capture both the short-run and long-run relationships.
4.2. Empirical findings
4.2.1. Econometric estimates
We also conducted various diagnostic tests to ascertain the validity and accuracy of our econometric estimates. The heteroskedasticity test was done using the Breusch and Pagan (Citation1979) test and the results indicated that the model was homoscedastic (0.5397 > 0.05). Schwarz’s Bayesian Information Criterion (SBIC) for optimal lag selection was adopted and it yielded the following order: (1,1,0,0,0,2,0,0,1,0). Further, we used the Breusch (Citation1978) and Godfrey (Citation1978) LM autocorrelation test to check whether the residuals were serially correlated. The findings showed that the error terms were not serially correlated (0.8668 > 0.05). We employed the Jarque and Bera (Citation1987) test in determining the normality of the residuals and the results (0.8659 > 0.05) also revealed that the residuals followed a normal distribution. We also tested for model stability using the Cusum squared test. From this graphical test result, our model was found to lie within the accepted significance levels hence suggesting model stability (see Appendix Figure ). The model was subsequently estimated with the results presented in Table .
Table 4. ARDL model results
The empirical findings in Table revealed that the ECT coefficient was negative and significant implying model convergence in the long run. This suggested the presence of a long-run relationship between GFCF and its determinants (remittances inclusive) in Kenya. It implied that any deviations from the long-run equilibrium were corrected at an adjustment speed of 75%.
Our main regressor (remittance as a ratio of GDP) was found to significantly reduce GFCF in Kenya in the short run. This impact was found to be positive but insignificant in the long run. These findings suggested that at first glance of remittances receipt, households generally used them to offset basic household expenditures such as food, health, and education. This meant that none or very little of the remittances were set aside for major investments at an aggregate level. The general notion would be that once these expenditures have been offset, households become forward-looking and, thus, dedicate future remittance receipts toward productive investment. However, the insignificant nexus in the long run seems to offer a divergent outcome; implying that remittances do not substantially impact GFCF in the long run and that their primary utilization may simply be pegged at the household level. These results disapprove the common conception that remittances have a positive and significant effect on aggregate investment both in the short run and in the long run, thus, providing a new insight on remittance utilization. In literature, our results contradict those of Dash (Citation2020) who found a positive and significant link between remittance inflows and domestic investment (both in the short run and the long run) on a panel of South Asian countries. Our findings also deviate from those of Aggarwal et al. (Citation2011) and Dzansi (Citation2013).
Consistent with our expectations, the structural break dummy was found to significantly moderate the impact of remittances on GFCF in Kenya. This impact was positive in the long run and highlighted the importance of testing for structural breaks when analyzing a remittance-GFCF model, something that has been largely ignored in previous studies. Resultantly, we posit that a conducive investment climate provides an antidote for long-run investment and is effective in controlling for the shocks generated by the structural changes in the economy. Incorporating the structural breaks yields a more accurate forecast as far as the remittances-GFCF nexus is concerned.
We also incorporated a number of control variables in our model. First, we found a positive and significant impact of GDP per capita on GFCF (at 5%) in the long run. Higher GDP per capita implies higher economic growth coupled with improved living standards & higher capital requirements. As a result, GDP per capita is expected to significantly increase an economy’s investment rate. This is theoretically and empirically supported (see Ali et al., Citation2013; Greene & Villanueva, Citation1991). Secondly, the exchange rate variable insignificantly determined GFCF. Thirdly, while the lending rate significantly increased GFCF in the short run, its impact was insignificant in the long run. These findings are contrary to our expectations. This is considering that the lending rate acts as a proxy for the cost of capital. The higher the rate, the lower the amount of money that individuals or firms can borrow and this stifles the investment prospects in an economy (Mody & Murshid, Citation2005).
Fourth, trade as a % of GDP significantly increased investment (at a 1% significance level). Higher trade activities as reiterated by Nowak-Lehmann (Citation2003), increase human and physical capital which generates more savings & technological transfer enhancement. Fifth, we found a considerable impact of net ODA on GFCF in the long run. We argue that ODA supplements remittances and are, therefore, expected to positively and significantly influence investment. This impact is significantly larger considering that ODA inflows, unlike remittances, are directly channeled toward investment activities in an economy. Sixth, while financial development (measured by domestic credit to private sector as a ratio of the GDP) significantly increased GFCF in the short run; the impact was rather insignificant in the long run. Vast literature argues that domestic investment thrives on a well-developed financial system due to reduced information asymmetries. As such, higher levels of financial development are expected to spur investment in the economy (Balcilar et al., Citation2016; Hassan, Citation2015). Finally, the regressors in our model explained 67.90% of the variations in the gross capital formation when adjusted for the degrees of freedom.
4.2.2. Causality test between remittances and gross fixed capital formation
To design policies geared towards the effective utilization of remittances, we performed the Granger Causality test to ascertain the causality direction between Remittances (REM) and Gross Fixed Capital Formation (GFCF) in Kenya. Considering that GFCF is integrated of order 1 and REM is integrated of order 0, we performed the Granger-Causality test using the first difference of GFCF. The Wald test results were presented in Table .
Table 5. Granger causality wald test results
Given that the probability value of chi-square was found to be 0.001 in our first case (which is less than the 5% significance level), we rejected the null hypothesis that REM does not cause GFCF. This implied that REM indeed caused GFCF. In the second case, the probability value was found to be greater than the level of significance (0.119 > 0.005), hence we failed to reject the null hypothesis that GFCF does not cause REM. The results in Table , thus, revealed evidence of a uni-directional Granger Causality that runs from remittances to gross fixed capital formation. Empirically, our findings are consistent with those obtained by Dash (Citation2020). Further, the remittance to GFCF causality direction is theoretically supported in the literature. The ‘remittance-led growth hypothesis posits that the inflow of foreign remittances triggers investment in the remittance-recipient economies by raising domestic savings, developing human & physical capital, and developing the financial sector (see Mundaca, Citation2009; Rao & Hassan, Citation2012).
5. Conclusions and policy implications
This study found evidence of a long-run relationship between international remittances and gross fixed capital formation (investment) in Kenya. First, while these remittances significantly reduced domestic investment in Kenya in the short run, their impact was rather insignificant in the long run. Secondly, we found a positive and significant moderating impact of the structural break dummy on the remittances-GFCF nexus in Kenya, highlighting the importance of testing for structural breaks when analyzing a remittance-GFCF model; something that has been largely ignored in previous studies. Thirdly, the causality test showed evidence of a uni-directional causality between the two variables running from remittances to GFCF, hence leading to the conclusion that remittances drove investment in the country of origin and not the other way around.
From our findings, we first conclude on the need for government sensitization on the productive use of remittances at household levels. This will ensure that the remittance-receiving households or individuals are forward-looking and hence use their remittances on productive investments as opposed to just offsetting the household consumption expenditures. Secondly, governments need to put in place measures that will necessitate the smooth and efficient inflow of foreign remittances. This can be realized through the development of a robust banking financial system as it will create more formal channels for remittance flows. This is considering the studies’ established complementary role of financial system development on aggregate investment. It re-affirms the fact that higher levels of financial development are critical in spurring investment in the economy. Thirdly, a uni-directional causality between the two variables running from remittances to GFCF leads to the conclusion that remittances drive investment in the country of origin hence focus by governments and private sector players should be placed on the development of the financial system as well the provision of a conducive investment climate for the optimal harnessing of remittance-driven investments. Furthermore, a conducive investment climate would be vital for long-run investment and effective in controlling for the shocks generated by the structural changes in the economy.
Future studies should attempt to analyze remittance inflows in Kenya in the wake of global shocks and more notably the COVID-19 pandemic. This will enable the design of policies that can sustain remittance inflows within the shock settings.
Acknowledgments
The author expresses sincere gratitude to the 4 anonymous reviewers and the Editor for their valuable comments on this paper. The usual disclaimer applies.
Disclosure statement
No potential conflict of interest was reported by the author.
Data availability statement
The data used for this study and the supporting Stata do-files can be made available upon reasonable request from the author. The data is readily accessible and can be downloaded free of charge from the World Bank’s World Development Indicators (WDI) database.
Additional information
Funding
References
- Adams, R. H., & Cuecuecha, A. (2010). Remittances, household expenditure and investment in Guatemala. World Development, 38(11), 1626–19. https://doi.org/10.1016/j.worlddev.2010.03.003
- Adams, R. H., & Cuecuecha, A. (2013). Remittances, household expenditure and investment in Ghana. World Development, 50(C), 24–40. https://doi.org/10.1016/j.worlddev.2013.04.009
- Agarwal, R., & Horowitz, A. (2002). Are International remittances altruism or insurance: Evidence from Guyana using multiple-migrant households. World Development, 30(11), 2033–2044. https://doi.org/10.1016/S0305-750X(02)00118-3
- Aggarwal, R., Kunt, A. D., & Peria, M. S. M. (2011). Do remittances promote financial Development? Journal of Development Economics, 96(2), 255–264. https://doi.org/10.1016/j.jdeveco.2010.10.005
- Agrawal, P. (2000). Economic impact of foreign direct investment in South Asia. Indira Gandhi Institute of Development Research.
- Ahamada, I., & Coulibaly, D. (2013). Remittances and growth in Sub-Saharan African countries: Evidence from a panel causality test. Journal of International Development, 25(3), 310–324. https://doi.org/10.1002/jid.2830
- Ahmed, V., Sugiyarto, G., & Jha, S. (2010). Remittances and household welfare: A case study of Pakistan. ADB Economics Working Paper Series, Asian Development Bank.
- Ajefu, J. B., & Ogebe, J. O. (2020). The effects of international remittances on expenditure patterns of the left-behind households in Sub-Saharan Africa. Review of Development Economics, 25(1), 405–429. https://doi.org/10.1111/rode.12721
- Ali, L., Shamsi, A. F., Panhawar, I. A., & Bashir, R. (2013). An Empirical Investigation of relationship between financial sector Development and domestic private investment in Pakistan. Global Management Journal for Academic & Corporate Studies; Karachi, 3(1), 176–185. https://www.proquest.com/docview/1517636388
- Arun, T., & Alku, H. (2010). Determinants of remittances: The case of the South Asian community in Manchester. Journal of Development Studies, 47(6), 894–912. https://doi.org/10.1080/00220388.2010.527951
- Balcilar, M., Ciftcioglu, S., & Gungor, H. (2016). The effects of financial development on investment in Turkey. The Singapore Economic Review, 61(4), 1–36. https://doi.org/10.1142/S0217590816500028
- Breusch, T. S. (1978). Testing for autocorrelation in dynamic linear models. Australian Economic Papers, 17(31), 334–355. https://doi.org/10.1111/j.1467-8454.1978.tb00635.x
- Breusch, T., & Pagan, A. (1979). A simple test for heteroskedasticity and random coefficient variation. Econometrica, 47(5), 1287–1294. https://doi.org/10.2307/1911963
- Brown, R., & Poirine, B. (2005). A model of migrants’ remittances with human capital investment and intrafamilial transfers. International Migration Review, 39(2), 407–438. https://doi.org/10.1111/j.1747-7379.2005.tb00272.x
- Chami, R., Barajas, A., Cosimano, T., Fullenkamp, C., Gapen, M., & Montiel, P. (2008). Macroeconomic consequences of remittances. IMF Occasional Paper No. 259. IMF.
- Chowdhury, M. (2016). Financial Development, remittances and economic growth: Evidence using a dynamic panel estimation. Margin: The Journal of Applied Economic Research, 10(1), 35–54. https://doi.org/10.1177/0973801015612666
- Cooray, A. (2012). Migrant remittances, financial sector development and the government ownership of banks: Evidence from a group of non-OECD economies. Journal of International Financial Markets, Institutions and Money, 22(4), 936–957. https://doi.org/10.1016/j.intfin.2012.05.006
- Dash, R. K. (2020). Impact of remittances on domestic investment: A panel study of six South Asian countries. South Asia Economic Journal, 21(1), 7–30. https://doi.org/10.1177/1391561420903199
- Docquier, F., & Rapoport, H. (2012). Globalization, brain drain, and Development. Journal of Economic Literature, 50(3), 681–730. https://doi.org/10.1257/jel.50.3.681
- Dzansi, J. (2013). Do remittance inflow promote manufacturing growth? The Annals of Regional Science, 51(1), 89–111. https://doi.org/10.1007/s00168-012-0529-x
- Edwards, C., & Ureta, M. (2003). International migration, remittances and schooling: Evidence from El-Salvador. Journal of Development Economics, 72(2), 429–461. https://doi.org/10.1016/S0304-3878(03)00115-9
- Engle, R. F., & Granger, C. W. J. (1987). Co-integration and error Correction: Representation, estimation and testing. Econometrica, 55(2), 251–276. https://doi.org/10.2307/1913236
- Fayissa, B., & Nsiah, C. (2010). The impact of remittances on economic growth and development in Africa. The American Economist, 55(2), 92–103. https://doi.org/10.1177/056943451005500210
- Fischer, G. (2013). Investment choice and inflation uncertainty. LSE Research online documents on Economics 54259. The London School of Economics and Political Science, LSE Library.
- Fry, M. J. (1993). Foreign direct investment in a macroeconomic framework: Finance, efficiency, incentives, and distortions. policy Research working paper series 1141. Debt and International Finance Division, International Economics Department, World Bank.
- Gani, A. (2016). Remittances and savings in Asia: Some empirical evidence based on the life-cycle model. Journal of Finance and Economics, 4(1), 24–38. https://doi.org/10.12735/jfe.v4i1p24
- Godfrey, L. G. (1978). Testing for higher order Serial Correlation in regression Equations when the regressors include lagged dependent variables. Econometrica, 46(6), 1303–1310. https://doi.org/10.2307/1913830
- Greene, J., & Villanueva, D. (1991). Private investment in developing countries: An empirical analysis. IMF Staff Papers, Palgrave Macmillan Journals, 38(1), 33–58. https://doi.org/10.2307/3867034
- Gubert, F. (2002). Do migrants insure those who stay behind? Evidence from the Kayes Area (Western Mali). Oxford Development Studies, 30(3), 267–287. https://doi.org/10.1080/1360081022000012699
- Harris, R. I., & Sollis, R. (2003). Applied time series modelling and forecasting. John Wiley and Sons.
- Hassan, O. M. (2015). The impact of monetary policy on private capital Formation in Nigeria. Journal of Empirical Economics, 4(3), 138–153.
- Hines, A. L., & Simpson, N. B. (2019). Migration, remittances and human capital investment in Kenya. Economic Notes, 48, e12142. 1–18. https://doi.org/10.1111/ecno.12142
- Jarque, C. M., & Bera, A. K. (1987). A test for normality of observations and regression residuals. International Statistical Review / Revue Internationale de Statistique, 55(2), 163–172. https://doi.org/10.2307/1403192
- Jena, F. (2018). Migrant remittances and physical investment purchases: Evidence from Kenya households. Journal of Development Studies, 54(2), 312–326. https://doi.org/10.1080/00220388.2017.1288219
- Lopez-Cordova, E. (2005). Globalization, Migration, and Development: The role of Mexican migrant remittances. Economia, 6(1), 217–256. https://doi.org/10.1353/eco.2006.0010
- Lucas, R. (1967). Optimal investment policy and the flexible accelerator. International Economic Review, 8(1), 78–85. https://doi.org/10.2307/2525383
- Lucas, R., & Stark, O. (1985). Motivations to remit: Evidence from Botswana. Journal of Political Economy, 93(5), 901–918. https://doi.org/10.1086/261341
- Migration and Development. (2019). Migration and remittances: Recent developments and Outlook. Migration and remittances brief 31. World Bank Group-KNOMAD.
- Misati, R. N., Kamau, A., & Nassir, H. (2019). Do migrant remittances matter for financial development in Kenya? Financial Innovation, 5(31), 1–25. https://doi.org/10.1186/s40854-019-0142-4
- Mody, A., & Murshid, A. P. (2005). Growing up with capital flows. Journal of International Economics, 65(1), 249–266. https://doi.org/10.1016/j.jinteco.2004.02.003
- Mundaca, D. (2009). Remittances, financial markets development and economic growth: The case of Latin America and the Caribbean. Review of Development Economics, 13(2), 288–303. https://doi.org/10.1111/j.1467-9361.2008.00487.x
- Ndirangu, L., Garcia, C., & Gitau, C. (2014). Evidence of structural breaks in Kenya macroeconomic Variables. A paper prepared for the 2014 CSAE conference held on 23rd to 25th March 2014, University of Oxford: CSAE.
- Nowak-Lehmann, F. (2003). Trade policy and its impact on economic growth: The Chilean experience in the period of 1960 to 1998. Applied Econometrics and International Development, 3(2), 25–53.
- Osili, U. O. (2004). Migrants and housing investments: Theory and evidence from Nigeria. Economic Development and Cultural Change, 52(4), 821–849. https://doi.org/10.1086/420903
- Osili, U. O. (2007). Remittances and savings from international migration: Theory and evidence using a matched sample. Journal of Development Economics, 83(2), 446–465. https://doi.org/10.1016/j.jdeveco.2006.06.003
- Pesaran, M. H. (2015). Time series and panel data econometrics. Oxford University Press.
- Pesaran, M. H. & Shin, Y. (1999). An autoregressive distributed-lag modeling approach to cointegration analysis. In S. Strom (Ed.), Econometrics and economic theory in the 20th century. The Ragnar Frisch centennial symposium (pp. 371–413). Cambridge University Press. https://doi.org/10.1017/CCOL521633230.011
- Pesaran, M. H., Shin, Y., & Smith, R. J. (2001). Bounds testing approaches to the analysis of level relationships. Journal of Applied Econometrics, 16(3), 289–326. https://doi.org/10.1002/jae.616
- Pindyck, R. S., & Solimano, A. (1993). Economic instability and aggregate investment. In O. Blanchard & S. Fischer (Eds.), NBER macroeconomics annual (Vol. 8, pp. 259–318). NBER, MIT Press.
- Rao, B. B., & Hassan, G. M. (2012). Are the direct and indirect growth effects of remittances significant? The World Economy, 35(3), 351–372. https://doi.org/10.1111/j.1467-9701.2011.01399.x
- Rehman, N. U., Hysa, E., & Poon, W. C. (2021). The effect of financial development and remittances on economic growth. Cogent Economics & Finance, 9(1), 1932060. https://doi.org/10.1080/23322039.2021.1932060
- Sahoo, P., & Dash, R. K. (2013). Financial sector development and domestic savings in South Asia. Economic Modelling, 33(C), 388–397. https://doi.org/10.1016/j.econmod.2013.04.018
- Woodruff, C., & Zenteno, R. (2007). Migration networks and microenterprises in Mexico. Journal of Development Economics, 82(2), 509–528. https://doi.org/10.1016/j.jdeveco.2006.03.006
- World Bank. (2018). World Development Indicators Online Database. Retrieved from http://data.worldbank.org/indicator.
- World Bank. (2019). World development indicators online database.
- World Bank. (2020). Personal remittances, received (current US$)-Kenya. Retrieved from: https://data.worldbank.org/indicator/BX.TRF.PWKR.CD.DT?locations=KE.
- Yang, D. (2008). International Migration, remittances and household investment. Evidence from Philippine migrants’ exchange rate shocks. The Economic Journal, 118(528), 591–630. https://doi.org/10.1111/j.1468-0297.2008.02134.x
- Yang, D. (2011). Migrant Remittances. Journal of Economic Perspectives, 25(3), 129–151. https://doi.org/10.1257/jep.25.3.129
- Yang, D., & Choi, H. (2007). Are remittances insurance? Evidence from rainfall shocks in the Philippines. The World Bank Economic Review, 21(2), 219–248. https://doi.org/10.1093/wber/lhm003
- Zardoub, A., & Sboui, F. (2021). Impact of foreign direct investment, remittances and official development assistance on economic growth: panel data approach. PSU Research Review, 7(2), 73–89. https://doi.org/10.1108/PRR-04-2020-0012
- Zivot, E., & Andrews, D. (1992). Further evidence on the great crash, the oil-price shock, and the unit-root hypothesis. Journal of Business and Economic Statistics, 10(3), 251–270. https://doi.org/10.2307/1391541