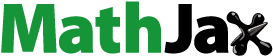
Abstract
Intrinsic dynamics of proteins are known to play important roles in their function. In particular, collective dynamics of a protein, which are defined by the protein’s overall architecture, are important in promoting the active site conformation that favors substrate binding and effective catalysis. The primary sequence of a protein, which determines its three-dimensional structure, encodes unique dynamics. The intrinsic dynamics of a protein actually link protein structure to its function. In the present study, coarse-grained normal mode analysis was performed to examine the intrinsic dynamic patterns of 24 different enzymes involved in primary metabolic pathways. We observed that each metabolic enzyme exhibits unique patterns of motions, which are conserved across multiple species and functionally relevant. Dynamic cross-correlation matrices (DCCMs) are visibly identical for a given enzyme family but significantly different from DCCMs of other protein families, reinforcing that proteins with similar function exhibit a similar pattern of motions. The present work also reasserted that correct identification of unknown proteins is possible based on their intrinsic mobility patterns.
Public Interest Statement
In the present work, the intrinsic dynamics of enzymes from 24 different families, which are involved in key metabolic pathways, were analyzed. The goal of this study is to better understand the connection between intrinsic dynamics and enzymatic function. As each enzyme exhibits a unique pattern of motions, these intrinsic dynamic patterns can be used to characterize the function of unknown proteins. This work demonstrates that (i) dynamic-based functional characterization is a highly reliable method and (ii) computationally less expensive coarse-grained normal mode analysis (NMA) is extremely useful to explore intrinsic dynamics of a vast number of proteins in relatively short duration of time. Overall, this work reasserted the importance of dynamics in protein function and suggested that coarse-grained NMA could be used for protein identification and classification. These findings can be applied by researchers working in the fields of protein chemistry and enzymology.
Competing Interests
The authors declare no competing interest.
1. Introduction
Proteins play crucial roles in nearly all biological processes. Each protein has a primary amino acid sequence that dictates the tertiary structure of the protein, and ultimately determines the protein’s overall function. In other words, the amino acid sequence → structure → function relationship exists for proteins and enzymes. Although the static structure of proteins is commonly correlated with their function, proteins are intrinsically dynamic in nature, and their internal motions, over a wide-range of timescales, assist their function. In fact, it has been suggested that the protein’s dynamics is the fundamental link between its structure and function (Hensen et al., Citation2012). However, the local dynamical character of an enzyme active site does not alone produce the functionally/catalytically competent state necessary to perform the protein’s biological function. In fact, the whole protein’s dynamism is essential for its overall function. Several studies revealed the influence of protein dynamics on enzyme catalysis, substrate binding, allosteric signal propagation, and protein–protein interactions (Agarwal, Citation2005; Agarwal, Billeter, Rajagopalan, Benkovic, & Hammes-Schiffer, Citation2002; Bhabha et al., Citation2011; Chennubhotla & Bahar, Citation2007; Chennubhotla, Yang, & Bahar, Citation2008; Eisenmesser et al., Citation2005; Hammes-Schiffer & Benkovic, Citation2006; Henzler-Wildman & Kern, Citation2007; Henzler-Wildman et al., Citation2007; Klinman & Kohen, Citation2013; Lisi & Patrick Loria, Citation2016; Liu et al., Citation2016; Singh, Francis, & Kohen, Citation2015; Vöhringer-Martinez & Dörner, Citation2016; Zen, Micheletti, Keskin, & Nussinov,, Citation2010). In addition, it has been observed that the dynamics of residues that are not directly involved in catalysis are also important for enzyme function (Roston, Kohen, Doron, & Major, Citation2014; Tousignant & Pelletier, Citation2004).
For a majority of known protein sequences and structures, functional information is lacking (Erdin, Lisewski, & Lichtarge, Citation2011). To date, the functional classification of proteins has been largely dominated by sequence- or structure-based methods. However, both methods have strict limitations. Sequence-based methods are limited due to evolutionary divergence, as a protein structure is more likely to remain conserved than its sequence (Chothia & Lesk, Citation1986), and effective sequence homology requires a minimum of 40% identity over significant portions of the sequence (Devos & Valencia, Citation2000; Hensen et al., Citation2012; Park, Teichmann, Hubbard, & Chothia, Citation1997; Whisstock & Lesk, Citation2003). Structure-based methods have revealed more direct functional relationships when coupled with sequence data. Unfortunately, structure-based prediction methods are limited due to diverse structures possessing similar functions or similar structures carrying out a diverse range of functions. Structure-based methods alone do not produce reliable functional predictions (reliability ≤ 30%) (Hensen et al., Citation2012). Hensen et al. (Citation2012) found that the intrinsic dynamics of a protein provide a more accurate depiction of protein function due to the large correlation between protein dynamics and function. They observed that functionally similar proteins tend to exhibit similar dynamic patterns and thereby, protein functional annotations could be accomplished based on dynamics (Hensen et al., Citation2012). Their work demonstrated that the functional classification of proteins based solely upon protein dynamics has a success rate of 46% within the broad functional classes, which is much higher than the structure-based prediction rate of 32%. Other researchers have also explored protein dynamics-function relationships by performing dynamics-based comparisons of proteins (Micheletti, Citation2013; Munz, Lyngsø, Hein, & Biggin, Citation2010; Pandini, Mauri, Bordogna, & Bonati, Citation2007; Tobi, Citation2013). Here, we have explored the dynamics of enzymes involved in some important metabolic pathways in an attempt to further our understanding of the relationships between protein structure, dynamics, and function.
Earlier, Hensen et al. have performed long timescale (100 ns) atomistic molecular dynamic (MD) simulations and derived 34 dynamic descriptors to characterize dynamics of proteins (Hensen et al., Citation2012). Picosecond to nanosecond timescale atomic fluctuations can be simulated using all-atom MD simulations, and important information about functional dynamics could be obtained. Unfortunately, it is computationally expensive to investigate functionally significant displacements occurring in microsecond to millisecond timescale using MD simulations. Alternatively, normal mode analysis (NMA) can be utilized to examine protein dynamics at a slower timescale (Bahar & Rader, Citation2005; Hinsen, Citation1998; Hinsen, Thomas, & Field, Citation1999). Both low-frequency vibrations that represent the protein’s collective motions as well as the higher frequency vibrations, which represent local deformations, can be studied by NMA. Earlier studies have shown that low-frequency vibrational modes or normal modes (large fluctuations) of proteins are functionally relevant (Brooks & Karplus, Citation1983; Go, Noguti, & Nishikawa, Citation1983; Marques & Sanejouand, Citation1995). Despite a few limitations such as local fluctuations upon substrate binding being poorly captured by the coarse-grained NMA simulations (Strom, Fehling, Bhattacharyya, & Hati, Citation2014), NMA is not limited by the system size and can be used to analyze functionally relevant conformational dynamics from protein structure, making it an incredibly powerful method of analysis. Additionally, the NMA method that employs course-grained elastic network model (ENM) offers a dependable yet computationally inexpensive way to evaluate large displacements (conformational changes) of proteins comprised of thousands of residues (Frank & Agrawal, Citation2000; Strom et al., Citation2014; Tama & Brooks, Citation2005; Weimer, Shane, Brunetto, Bhattacharyya, & Hati, Citation2009).
In the present study, ENM-based coarse-grained NMA was employed to characterize intrinsic dynamics of metabolically relevant enzymes and formulate a protein functional characterization method emphasizing dynamical importance. The dynamics of 24 enzymes involved in primary metabolic pathways were studied and sequence, structure, and dynamic similarities were calculated across six species to determine the degree of conservation of sequence, structure, and dynamics within a protein group. We examined the relationship among these three properties, as well as developed a catalog of dynamic cross-correlation matrices (DCCMs). We then attempted to match a set of proteins to the catalog of DCCMs for their functional identification. The present study demonstrated that each metabolic enzyme exhibits unique patterns of motions, which are conserved across multiple species; proteins with similar function exhibit a similar pattern of motions. Moreover, this work also demonstrated that the coarse-grained NMA is a faster method to develop protein-specific DCCMs, which could be used for functional characterization. Also, it is established from our study that dynamic-based functional characterization is highly predictable. This study could be extended to include all known protein families and generate a database of DCCMs of proteins for functional annotation.
2. Methods
2.1. Protein sequences and structures
Twenty-four proteins from common metabolic pathways, as listed in Table , were selected from the Protein Data Bank (PDB) (Berman et al., Citation2000) based upon structural data availability. We attempted to select proteins from six different species for which crystal structures are known. Also, proteins were screened for similar amino acid chain length. A total of 141 proteins were considered in this study because only 5 crystal structures were available for 3 of the 24 protein families. The symmetry and stoichiometry analyses revealed that all but one of the selected protein families are homopolymers. Therefore, a single polypeptide chain (chain A) was chosen to compare the sequence and structure, as well as intrinsic dynamics between proteins (from different species) within a given family. For the heterodimer protein, succinyl-CoA synthetase, both chains were used. In the present study, structures of proteins were visualized using the Visual Molecular Dynamics (VMD) program (Humphrey, Dalke, & Schulten, Citation1996).
Table 1. Comprehensive list of the 24 protein groups and the six selected proteins for each group with the organism name, PDB ID, and number of amino acid residues (sequence length) in chain A of the protein
2.2. Sequence comparison
The sequence identity of proteins in each group was examined. These pairwise sequence alignments were carried out with the Basic Local Alignment Search Tool (BLAST) (Altschul et al., Citation1997), which aligns two sequences and provides the percentage of identical residues. The sequence identity is the percentage of identical residues between protein sequences being compared. A mean and standard deviation was calculated for each protein group.
2.3. Structure comparison
The pairwise DaliLite server (Holm & Rosenstrom, Citation2010) was used to determine the degree of structural similarity between two proteins. Pairwise structural alignments were performed between all proteins of a given group. Root-mean-square deviation (RMSD) was used to address structural (protein backbone) comparison, which represents the variation of a particular locus between two superimposed structures. The mean and standard deviation of the sample were calculated for each protein group.
2.4. Single and comparative NMA
In the coarse-grained ENM, developed by Hinsen, Petrescu, Dellerue, Bellissent-Funel, and Kneller (Citation2000), the protein is simplified to a string of beads, where each bead represents a Cα atom. The interactions between Cα atoms are expressed as a harmonic pair potential as follows:(1)
(1)
where ri and rj are the positions of residues i and j in the current conformation of the protein, and the superscript 0 denotes the equilibrium conformation; kij is the force constant for the spring connecting residues i and j. The force constant kij is distance dependent and expressed as(2)
(2)
where a, b, c, and d are determined by fitting the equation to an all-atom model as discussed by Hinsen et al. (Citation2000) and Tiwari et al. (Citation2014). The overall potential of the system, which is the sum of the pair potential over all pairs, is expressed as follows:(3)
(3)
Eigenvectors and eigenvalues of the mass weighted matrix of the second derivative of U(r) correspond to normal modes and the squares of the frequencies for each normal mode, respectively (Tiwari et al., Citation2014). In the present study, normal mode calculations were carried out using the WEBnm@ server (Fuglebakk, Tiwari, & Reuter, Citation2014; Hollup, Salensminde, & Reuter, Citation2005; Tiwari et al., Citation2014). WEBnm@ uses a molecular modeling toolkit and employs the coarse-grained ENM to calculate the low frequency normal modes. Individual PDB files were submitted to the online WEBnm@ server (Hollup, Fuglebakk, Taylor, & Reuter, Citation2011; Hollup et al., Citation2005; Skjaerven, Hollup, & Reuter, Citation2009; Tiwari et al., Citation2014) for single NMA analysis. The correlated/anticorrelated motions between residues (Cα atoms) were identified from the DCCMs. The DCCM was calculated from the normal modes using the method described by Ichiye and Karplus (Ichiye & Karplus, Citation1991). Each element in the DCCM quantifies the coupling between two Cα atoms i and j as:(4)
(4)
In Equation (3), Xm and Ym represent eigenvectors and eigenvalues of the mth normal mode, respectively. In the present study, the DCCMs were obtained using the default setting of WEBnm@ i.e. the combined 200 modes were used to generate each DCCM. The DCCMs of the 24 protein families were compared and a catalog of all DCCMs was assembled for identification of unknown (test) proteins. The single WEBnm@ analysis also provides atomic (Cα atoms) fluctuation profiles (vide infra) and vector coordinates files, which allow for the visualization of the protein’s dynamics in different modes. In WEBnm@, modes 1–6 correspond to the rotational and translational motions and are ignored. Therefore, mode 7 actually represents the lowest frequency mode of a protein system.
The comparative NMA was performed for each enzyme to examine the conservation of dynamics across species (Fuglebakk, Echave, & Reuter, Citation2012). In the present study, dynamics of a given enzyme from five to six different species were compared. Aligned FASTA sequence (See Supplementary Table S1) and corresponding PDB coordinate files of each protein group were used for the comparative NMA (Sievers et al., Citation2011). From the comparative analysis, the dynamic similarity, in terms of Bhattacharyya coefficient (BC) (Bhattacharyya, Citation1943), as well as the heat map showing the theoretical evolutionary relatedness between proteins solely based upon their intrinsic dynamics were obtained.
2.5. Bhattacharyya coefficient
The BC measures the dynamical similarity between proteins by comparing their covariance matrices, which are obtained from the normal modes of the conserved parts of proteins under consideration (Tiwari et al., Citation2014). The BC calculations were performed following the method described by Tiwari et al. (Citation2014). The BC ranges from 0 to 1, and represents the amount of overlap between the collective dynamics of the aligned proteins; BC of 1 represents maximum overlap or dynamical similarity between proteins. For a given protein group, the average BC with the sample standard deviation is calculated for comparative purposes.
2.6. The Cα atom fluctuations
The normalized squared Cα atom fluctuations for each protein were calculated as the sum of the displacement of each Cα atom along the lowest modes (Tiwari et al., Citation2014). The fluctuations are the sum of the atomic (Cα atoms only) displacements in each mode weighted by the inverse of their corresponding eigenvalues. In the present study, the default setting of WEBnm@, which includes the first 200 modes, was used. The x-axis of a fluctuation profile represents residue index in the protein sequence, while the y-axis represents the normalized displacement corresponding to each amino acid. Peaks in the fluctuation profile correspond to flexible regions of proteins.
2.7. Root-mean-squared inner product
For quantitative comparison of atomic (Cα atoms) fluctuations between proteins, the RMSIP was computed for the lowest normal modes using the following equation as described in Amadei, Ceruso, and Di Nola (Citation1999), Carnevale, Pontiggia, and Micheletti (Citation2007), Fuglebakk et al. (Citation2014), Tiwari et al. (Citation2014) and Yang, Song, Carriquiry, and Jernigan (Citation2008):(5)
(5)
where Xi and Yj represent the eigenvectors of a pair of proteins being compared and i and j represent the mode numbers. In the present study, the default setting of WEBnm@, which includes the first 10 lowest energy nontrivial modes (Amadei et al., Citation1999; Tiwari et al., Citation2014), was used. The RMSIP values range from 0 to 1; RMSIP of 1 represents maximum similarity in Cα atom fluctuations between proteins being compared.
2.8. Identification of test proteins
PDB coordinate files of 12 separate proteins, which either belong to one of the 24 protein groups included in the present study or were randomly chosen proteins, were selected from the PDB. The coordinate files of these proteins were modified to remove any identifying evidence, leaving only the Cartesian coordinates of each atom in the PDB file to run a single NMA analysis. The DCCMs of these test proteins were then compared to the catalog of DCCMs (obtained from the NMA of 24 protein groups) in an attempt to correctly match a test protein to a known protein group for functional identification. Wherever possible, quantitative analyses were performed using the comparative NMA analysis option of WEBnm@. The comparative analysis provides the extent of dynamic similarities between proteins in terms of BC and RMSIP values.
3. Results and discussion
3.1. Protein sequences and structures
A total of 141 protein sequences and structures were collected from the PDB files. The proteins are from metabolic pathways including glycolysis, gluconeogenesis, fermentation, the citric acid cycle, and the urea cycle (Table ). These 24 protein groups along with the name of organisms, PDB codes, and the number of amino acids in polypeptide chain A are listed in Table . Each protein group has six different organisms associated with it, with three exceptions; arginase, phosphoglycerate kinase, and ornithine transcarbamoylase, which only have five structures. Each protein group possessed a similar number of residues per chain.
3.2. Sequence comparison
The sequence identities between proteins of a given family are computed and listed in Table . The sequence identities range from about 24 to 61% with an average of 46% among all protein groups. Nine of the protein families had sequence identities greater than 50%, which means that the individual protein structures have at least 90% of their residues within the common cores (Chothia & Lesk, Citation1986). For the other 15 protein families with sequence identities between 20 and 50% are found to have 42–98% of individual protein residues within the common cores (Chothia & Lesk, Citation1986).
Table 2. The number of species studied (N), the sequence identity, structural similarity, and dynamic similarity (in terms of BC) of the 24 protein groups studied
3.3. Structure comparison
The RMSD values that represent structural similarities between proteins within an enzyme family are also shown in Table . The RMSD represents the deviation between two superimposed structures. The observed RMSD values ranged from 1.0 to 3.1 Å, with the lower numbers representing more similar structures. The RMSD is usually small (< 3 Å) for homologous proteins (Chothia & Lesk, Citation1986; Kosloff & Kolodny, Citation2008). The RMSD between homologous proteins within an enzyme family studied in this work were observed to be ≤3 Å, which suggested that these homologous proteins possess considerable extent of structural similarities. For higher sequence identities, we expected to see a low RMSD for the protein group, which was satisfied (Table ).
3.4. The Cα atom fluctuations
The fluctuation profiles for Cα atoms of all 24 groups of proteins were obtained from the NMA study. The calculated normalized squared fluctuations of Cα atoms matched satisfactorily with the experimental results for these enzymes. For example, the structure of the Escherichia coli methionine adenosyltransferase (MAT) (Figure ) revealed a dynamic loop region shown in red (Fu, Hu, Markham, & Takusagawa, Citation1996). Both the loop region (residues 100 to 112 for E. coli MAT) and the adjacent residues displayed high flexibility as observed in the normalized fluctuation (Cα atoms) profile (Figure ). This observation confirmed that the experimentally observed flexible regions of MAT correlate well with peaks in the fluctuation profile. Also, high flexibility was noticed from the active site motions, which can be seen around residues 232 to 254.
Figure 1. The structure of E. coli methionine adenosyltransferase homodimer (1FUG) with the flexible gating loops shown in red and the active site residues in orange. The structure visualization was achieved with VMD.
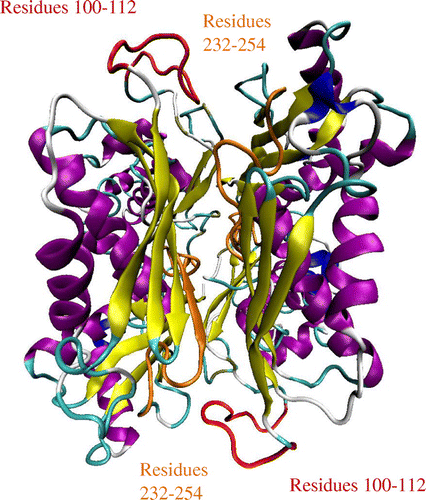
Figure 2. The Cα atom fluctuations of the E. coli methionine adenosyltransferase. The fluctuations describe the flexibility of Cα atoms of the enzyme.
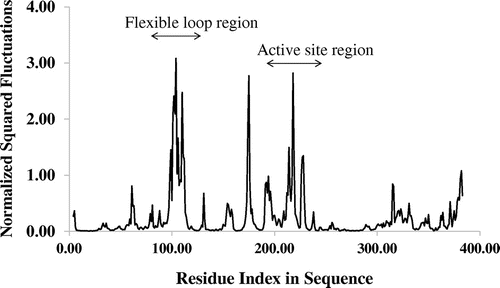
3.5. Correlated and anticorrelated motions
The single NMA for each protein within a particular group revealed strikingly similar correlation matrices. Slight differences were seen with respect to the extent of correlated and anticorrelated motions; however the overall patterns remained consistent. As an example, Figure shows the six DCCM files for the MAT group. All six protein matrices appear identical, as was the case with all other protein groups. Therefore, only one representative DCCM was chosen for each enzyme group and listed in Table . Because DCCMs of homomultimeric proteins revealed that each polypeptide chain displays identical patterns of motions (Figure S1), only one polypeptide chain of multimeric proteins was used to represent the intrinsic dynamic patterns (DCCMs) of all protein families, except for succinyl-CoA synthetase. For succinyl-CoA synthetase, a heteromultimeric protein, both polypeptide chains were used to represent the intrinsic dynamic patterns (Table ). However, to examine the entire dynamical nature of a protein system and the functional relevance of those intrinsic dynamics, the other chains were also taken into account. For example, MAT (Figure ) functions as a homodimer and as shown in Figure , the flexible loop appears to gate the active site. The majority of the active site consists of residues 247 to 258, and the flexible loop consists of residues 101–109. Interestingly, the flexible loop shows anticorrelated motion with the active site (Figure ). This anticorrelated motion suggests that the loop would open, which will allow the substrate to have proper access to the active site. The anticorrelated motion between active site pocket and the loop region also suggests that the two subunits move apart to make room for substrate molecules to enter active sites. These motions are seen across all species, suggesting that these functional dynamics have been conserved throughout MAT evolution.
Figure 3. Dynamic cross-correlation matrices of six proteins in the methionine adenosyltransferase group with a color key depicting the correlated motions in red and anticorrelated motions in blue. The overall pattern remains consistent across all species within the group, apart from minute differences that are barely visible to the naked eye.
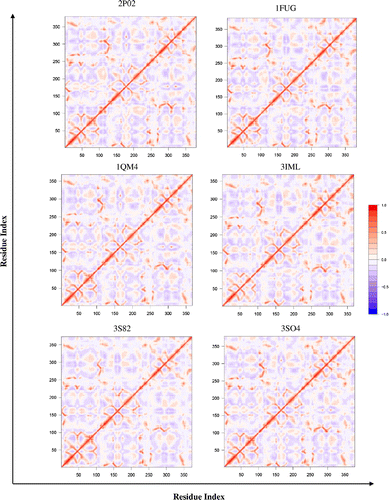
Table 3. A list of proteins with PDB codes, DCCM of the protein represented in bold, and the group BC heat map
Figure 4. A dynamic cross-correlation matrix for E. coli Methionine Adenosyltransferase (1FUG) showing the correlated (red) and anticorrelated (blue) motions between Cα atoms.
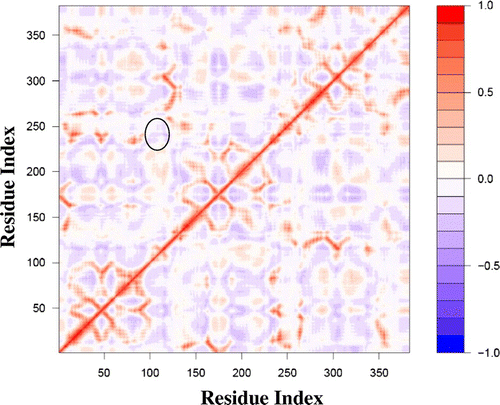
3.6. Bhattacharyya coefficients
The average BC of the 24 protein groups remained remarkably consistent (Table ), with the lowest score of 0.73 and the highest score of 0.89, where BC of 1.0 represents the maximum similarity in dynamics of proteins used for comparison. It appears that even for the lower sequence identities and similarities, the dynamic similarity of each protein group remains relatively high. Similar observations were made in the case of the cytochrome P450 family of enzymes, where these enzymes share a strong dynamic similarity (BC > 80%) despite low sequence identity (<25%) (Dorner et al., Citation2015).
In the present study, the comparison of the dynamic similarities among proteins within a group was also made. Table provides a representative heat map of the BCs for each enzyme. Heat map data describe the pairwise comparison of protein structures using the Bhattacharyya coefficients, with red shading representing identical dynamics between proteins being compared, and blue shading showing the least similar intrinsic dynamics. The heat map also groups the individual proteins into clusters, based upon the relatedness of enzyme dynamics. This provides additional information to enable the investigation of protein evolution with regard to protein dynamics. For example, the heat map for the BC of MAT is shown in Figure (the sequence alignment file used for dynamic comparison is shown in Supplementary Table S2). The cluster data is shown on the x- and y-axis, grouping the dynamics of E. coli (1FUG), B. peudomallei (3IML), and M. avium (3S82) MATs together. The dynamics of 3IML are more similar to the dynamics of 1FUG, than to the dynamics of 3S82. The dynamics of 2P02 (H. sapiens), 3SO4 (E. hystolytica), and 1QM4 (R. norvegicus) form another cluster, with 1QM4 and 3SO4 being more related to each other than to 2P02.
Figure 5. Methionine Adenosyltransferase heat map of BC showing the dynamic relatedness between proteins, with the color key at the bottom.
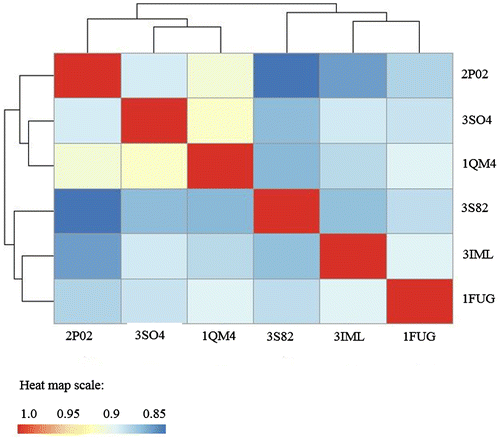
3.7. Functional relevance of the existing intrinsic dynamics
Close analysis of the intrinsic dynamics of the metabolic enzymes studied here revealed some functionally relevant motions, which are described below. For this study, depending upon the protein system, single or multiple polypeptide chains were considered to analyze the functional dynamics. In particular, an effort has been made to consider as many polypeptide chains as possible to maintain the dynamic integrity of the given protein system and show their functional relevance. Also, for simplicity, the Cα atom displacement graph of a specific mode(s) that was functionally more relevant has been considered here. The online NMA server failed to calculate and analyze the normal modes of five enzyme families when the multimeric proteins, instead of single polypeptide chains, were used. Therefore, intrinsic dynamics and their functional relevance of 19 out of 24 enzyme systems were analyzed here. Information regarding the general enzymatic properties and catalytic roles of various enzymes studied here were taken from Biochemistry 7th Edition (Berg, Tymoczko, & Stryer, Citation2012).
Citrate synthase (PDB code: 2H12; polypeptide chains A and B of the tetrameric protein were visualized for structural and dynamical analyses) is responsible for condensing acetyl-CoA and oxaloacetate to form citrate. To accomplish this, the enzyme undergoes an “open” to “closed” conformational change (Wiegand & Remington, Citation1986). First, the substrate, oxaloacetate, enters the enzyme in its “open” conformation; the coenzyme, acetyl-CoA, further interacts with nearby residues in the “closed” conformation. These residues are located too far to facilitate the reaction while in the “open” state. The enzyme accomplishes this by having residues 274 to 364, in conjunction with surrounding residues, exhibit an anticorrelated motion with greater flexibility (boxes in the fluctuations plot and DCCM, Figure ) to the active site and residues 423 to 436, as it opens and closes around the active site of citrate synthase. Residues 423 to 436 on both subunits are shown to interact with the opposing subunit, suggesting that these residues assist in conformational changes of each other, as well as facilitate substrate binding and catalysis.
Figure 6. A list of proteins with corresponding PDB code and their structural visualization, the functionally relevant Cα atom displacement graph, and DCCM.
Fumarase (PDB code: 4ADL, a tetrameric protein; in the present study chains A and B and chains C and D are visualized separately for structural and dynamical analyses) catalyzes the reversible reaction of fumarate to malate. This reaction occurs with a conformational change after the substrate enters the active site, which promotes the enzyme to transition into its “closed” conformation (Stoddard, Dean, & Koshland, Citation1993). For fumarase to carry out its function, it exists as a tetramer with two active sites that interact with its substrates. However, only three of the four chains interact with each substrate (chains A, B, C and chains A, B, D interact with each substrate). For chains A and B, interactions with the substrate occur between residues 185 to 193 and 403 to 460, along with surrounding residues (red boxes in the displacement graph; Figure ). These two groups of residues, 185 to 193 and 403 to 460, are also highly flexible and anticorrelated to each other, allowing for the greatest range of motion, so that the substrate can both easily diffuse in or out, in addition to binding tightly to the enzyme (boxes in DCCM, Figure ). Chains C and D only interact with one of the two substrates through residues 315 to 325, which are shown in the Cα atom displacement graph as having the greatest movement (blue boxes in displacement graph; Figure ).
Isocitrate dehydrogenase (PDB code: 2DHT, chains A and B was visualized for structural and dynamical analyses) is a homodimeric protein that is responsible for catalyzing the oxidative decarboxylation of isocitrate. The substrate interacts with the active site of isocitrate dehydrogenase via the three arginine residues, R115, R125 and R149 as well as five other residues within the active site (arginine residues shown in green space filled spheres, Figure ) (Fedøy, Yang, Martinez, Leiros, & Steen, Citation2007). The two active sites of isocitrate dehydrogenase are on opposite sides of each other, meaning that when one active site is in the “open” state, the other is in its “closed” state conformation (Figure ). The Cα atom displacement graph of isocitrate dehydrogenase shows general fluctuations throughout the whole enzyme, which is consistent to the overall motion. The active site for isocitrate dehydrogenase is unique in the fact that it is located between the two subunits. The mechanism for catalysis then relies on the concerted movement of both subunits. This is reflected by residues 150 to 196 on both subunits, that interact with each other, acting as a hinge site for both subunits to pivot around. Residues 1 to 100 (red box in displacement graph; Figure ) on either chain interacts with the residues 200 to 300 (blue box in displacement graph; Figure ) on the opposing chains, portraying a pincer-type mechanism around the active site by moving in an anticorrelated motion to each other (DCCM, Figure ).
Malate dehydrogenase (PDB code: 4CL3; chain A of the tetrameric protein was visualized) has the role of converting malate to oxaloacetate. To do so, the enzyme is comprised of two or four chains, forming a homodimer or homotetramer complex that moves anticorrelated to each other. Malate dehydrogenase has a highly flexible loop between residues 80 and 90 (Figure ) that opens and closes around the active site, allowing entry and exit of reactants and products as well as excludes surrounding solvents during the reaction. This flexible loop region is highly conserved within malate dehydrogenases, emphasizing its importance in catalysis (Goward & Nicholls, Citation1994). The overall observed anticorrelated movement within a chain appeared to be quite weak because the chain revolves in a circular pattern, making the cross-correlation map appear whiter (DCCM, Figure ). There is a weak anticorrelated motion between residues 1 to 75 and residues 80 to 90, since they are on opposing sides due to the enzyme’s helical movement (boxes in DCCM, Figure ).
Succinyl-CoA synthetase (PDB code: 1CQI; visualized chains A and B only for structural and dynamical analyses) is a heterotetramer made up of two identical heterodimers. The role of the enzyme is to convert succinyl-CoA to succinate. This reaction is carried out through two different binding pockets, one on each distinct subunit within a heterodimer (Wolodko et al., Citation1994). Chain A contains the succinyl-CoA binding pockets, while chain B contains the ATP/GTP binding pockets. For succinate to form, ADP needs to be present in the binding pocket of chain A. The enzyme accommodates this by having chain A and chain B rotate in an anticorrelated motion to each other (DCCM, Figure ), allowing ATP to become closer to the chain A, which is responsible for dephosphorylating ATP to ADP and the reaction to occur.
Alcohol dehydrogenase (PDB code: 6ADH; both subunits of the dimeric protein were visualized for structural and dynamical analyses) is a homodimeric protein with one active site on each identical subunit. Alcohol dehydrogenase undergoes a conformational change, not in the presence of alcohols, but rather the coenzyme NAD (Eklund et al., Citation1976). Within each subunit, there is a highly flexible loop between residues 240 and 250 that interacts with the active site of the enzyme, indicative of a lid-type mechanism (boxes in Cα atom displacement graph, Figure ). The loop and surrounding residues move in an anticorrelated motion (DCCM, Figure ). This anticorrelated motion results in a wide opening that allows substrate binding and catalysis.
Enolase (PDB code: 1IYX; both subunits of the dimeric protein were visualized for structural and dynamical analyses) is an enzyme that is part of the glycolysis pathway, converting 2-phosphoglycerate to phosphoenolpyruvate. The mechanism for this reaction is rather simple. Enolase has a very generic opening and closing motion, using flexible loops to interact with the active site (Lebioda & Stec, Citation1991). The first set of residues, residues 1 to 140, move in an anticorrelated motion with the other residues, residues 141 to 300 (boxes in DCCM, Figure ). Residues 250 to 255 have the greatest flexibility (boxes in Cα atom displacement graph, Figure ), suggesting a lid-type mechanism protecting the substrate in the active site. This simple motion is needed for fast turnover rates in the glycolysis pathway to supply the organism with energy.
Fructose 1–6 bisphosphate aldolase (PDB code: 2FJK; visualized chains A and B of the tetrameric protein) catalyzes the reversible step in glycolysis that converts fructose 1,6-bisphosphate, which is a six carbon molecule, into dihydroxyacetone phosphate and glyceraldehyde 3-phosphate, which are both three carbon molecules. Fructose 1–6 bisphosphate aldolase is a homotetramer with four identical active sites, one within each monomer. The monomers of the enzyme shift between an “open” and “closed” conformation (Hester et al., Citation1991). Within the tetramer, chains A and B work in concert and chains C and D mirror the motions of chains A and B. Chains A, B and chains C, D have similar helix-turn-helix residues (residues 258 to 289) where they overlap with each other and with the active sites on opposing sides. This allows the overlapping helix-turn helix residues to assist each other in facilitating the conformational change from “open” to “closed”. For example, when chain A opens, its helix-turn-helix moves out of the way and allows the helix-turn-helix of chain B to close, and vice versa. The overall circular motion of the enzyme takes place because residues 135 and 150 (red boxes in Cα atom displacement graph, Figure ) rotate with a greater range of motion than the rest of the enzyme, allowing them to act as a lid over the active site. The motion of this lid, which is constituted of residues 135 and 150, is important for substrate binding and catalysis.
Glyceraldehyde 3-phosphate dehydrogenase (PDB code: 4QX6) is an important enzyme necessary for glycolysis. This enzyme converts glyceraldehyde 3-phosphate into 1, 3-bisposphoglycerate. Glyceraldehyde phosphate dehydrogenase is a homotetramer made up of 4 identical subunits, each with their own active sites. Although they are identical subunits, each chain interacts differently with each other. Interactions between chains A and B are mirrored by chains C and D, while interactions between chains A and C are mirrored by chains B and D. The openings of the active site pockets on chain A and chain B overlap, so the opening and closing of both chains occur simultaneously. Additionally each chain has a loop within residues 299 to 307 that interacts with the same residues in another chain (chains A, C and chains B, D), that further facilitates this simultaneous open and closing motion required for substrate binding (Lin et al., Citation1990).
Hexokinase (PDB code: 1BDG; the monomeric protein is visualized) is the first enzyme in the glycolysis pathway and also catalyzes the rate limiting reaction for glycolysis. The enzyme is responsible for converting glucose to glucose-6-phosphate, using one molecule of ATP in the process. The enzyme is a monomer with one active site where the enzyme opens and closes with a hinge like motion around the substrate. The flexibility of the enzyme allows the residues 50 to 260 (red boxes in Cα atom displacement graph, Figure ) to move anticorrelated with the rest of the enzyme to ensure a tight “closed” conformation and the loose “open” conformation (boxes in DCCM, Figure ). This flexibility assists in the mechanism of the enzyme to tightly bind the substrate and prevent wasteful hydrolysis of ATP (Bennett & Steitz, Citation1978).
Phosphoglycerate kinase (PDB code: 1PHP) is a monomeric protein that is responsible for catalyzing the transfer of phosphate group from 1, 3-biphosphoglycerate to ADP in order to form 3-phosphoglycerate and ATP. The two substrates bind in two separate pockets of phosphoglycerate kinase. The ADP binds at the site formed by residues 181 to 384, whereas the second binding pocket is formed by residues 1 to 180, which binds 1, 3-biphosphoglycerate. After both substrates are bound, it allows the enzyme to undergo a conformational change in an anticorrelated rotating motion that brings the two substrate binding sites close enough for the catalysis to take place, as well as to exclude water molecules to prevent unwanted ATP hydrolysis (Banks et al., Citation1979).
Phosphoglycerate mutase (PDB code: 1YFK; visualized chains A and B of dimer for structural and dynamical analyses) is involved in glycolysis and is responsible for catalyzing the transfer of a phosphate group from C3 to C2 to form 2-phosphoglycerate from 3-phosphoglycerate. Phosphoglycerate mutase is a homodimer with two identical active sites within each subunit that allows phosphate and 3-phosphoglyceric acid to bind (Winn et al., Citation1981). The enzyme catalyzes the transfer of a phosphate group from a specific histidine residue, to the C2 carbon, taking the phosphate from the C3 carbon of the 3-phosphoglycerate, through a formation of a 2, 3-bisphosphoglycerate intermediate. At the active site of phosphoglycerate mutase, is a very flexible loop (residues 100 to 125) that moves in an anticorrelated motion to the rest of the active site, acting like a lid (boxes in Cα atom displacement graph, Figure ). The anticorrelated movement of the lid allows the entering of substrate and exiting of product from the active site.
Pyruvate decarboxylase (PDB code: 1QPB; chains A and B of tetramers were visualized for structural and dynamical analyses) catalyzes the last step in glycolysis where phosphoenolpyruvate is converted into pyruvate. Pyruvate decarboxylase is a homotetramer with identical active sites within each subunit (Lu, Dobritzsch, Baumann, Schneider, & König, Citation2000). For catalysis, a pair of subunits, A and B functions together as residues 1 to 194 and 351 to 560 of opposing chains anchor to each other. This allows residues 195 to 350 to have greater movement and flexibility since the rest of the enzyme is stationary. Residues 195 to 350 then move anticorrelated to the other residues allowing it to open and close around the active site of the enzyme. This suggests that residues 195 to 350 can act as a lid mechanism to the active site (boxes in the Cα atom displacements, Figure ).
Pyruvate kinase (PDB code: 3SRF; chains A and B of the tetramers were visualized for structural and dynamical analyses) catalyzes the final step in glycolysis. The enzyme has identical active sites within each subunit of its homotetramer, where it catalyzes the conversion of phosphoenolpyruvate into pyruvate. A lid-type mechanism is employed by each subunit to allow for the catalysis to occur (Mattevi, Bolognesi, & Valentini, Citation1996). Residues 117 to 217 (boxes in Cα atom displacement graph, Figure ) are highly flexible as they move from an “open” to “closed” conformation relative to the active site, as shown in the box in the Cα atom displacement graph. The DCCM (see regions in boxes) further shows that residues 117 to 217 are involved in an anticorrelated motion with respect to the rest of the enzyme to have the greatest movement during its conformational changes.
Triose phosphate isomerase (PDB code: 8TIM; both chains of the homodimer were visualized) catalyzes the reversible interconversion of different triose phosphate isomers. Triose phosphate isomerase exists as a homodimer with identical active sites within each subunit. The mechanism of catalysis is indicative of a pincer-type mechanism, with loops opening and closing over the active site (Davenport et al., Citation1991). Residues 170 to 220 of the enzyme open and close around the active site, and move in an anticorrelated motion to the rest of the enzyme (boxes in the DCCM, Figure ). This anticorrelated motion alone does not capture the full catalytic mechanism, as the displacement graph shows a general flexibility exists throughout the whole enzyme to assist in catalysis.
Phosphoenolpyruvate carboxykinase (PDB code: 1OS1; only chain A was visualized) catalyzes the reaction from oxaloacetate to phosphoenolpyruvate (PEP) and CO2. PEP carboxykinase does this by employing an induced-fit mechanism. The enzyme opens and closes around the substrates, oxaloacetate and ATP, taking on a different conformation in the presence of ATP (Dunten et al., Citation2002). In doing so, the enzyme is able to exclude water molecules that could potentially hydrolyze ATP. This mechanism also allows residues on the enzyme that could not originally reach the active site to interact with the substrates and favor catalysis. Residues 442 to 510 (box in displacement graph, Figure ) and surrounding residues move anticorrelated with the active site and the rest of the enzyme, as shown in the DCCM (Figure ).
Argininosuccinate synthetase (PDB code: 1J1Z; all four chains of the tetrameric protein were visualized for structural and dynamical analyses) catalyzes the synthesis of arginosuccinate, which is a necessary precursor for arginine and fumarate. Arginosuccinate synthetase is a homotetramer with identical active sites within each subunit. The motion of the enzyme is indicative of a lid-type mechanism with “open” and “closed” conformations, depending on substrate presence around the active site (Lemke & Howell, Citation2001). Residues 1 to 165 show a greater range of flexibility (boxes in Cα atom displacement graph, Figure ) as it moves in an anticorrelated motion to the rest of the enzyme and active site (black square boxes in DCCM, Figure ) to assist in the “open” and “closed” conformations of the enzyme.
Ornithine transcarbamylase (PDB code: 4OH7; chains A and B of the homotrimer were visualized for structural and dynamical analyses) is an enzyme necessary to catalyze the reaction between carbamoyl phosphate and ornithine to form citrulline. Visualization of the structure suggests that the two subunits have little interaction with one another. Overall, ornithine transcarbamylase is very flexible and the mechanism of catalysis is quite simplistic, where half the residues, residues 150 to 280, move in an anticorrelated motion to the rest of the enzyme (black square boxes in the DCCM, Figure ). This indicates that the enzyme has a pincer-type mechanism around the active site, which might favor substrate binding and product release (Allewell, Shi, Morizono, & Tuchman, Citation1999).
Pantothenate synthetase (PDB code: 2A86; both chain A and chain B of the dimer were visualized for structural and dynamical analyses) functions as a homodimer with identical domains and active sites where it carries out the catalysis of pantothenate from condensation of pantoate and β-alanine. Pantothenate synthetase also has a flexible active site cavity to allow substrate and product entry and exit to assist catalysis (Wang & Eisenberg, Citation2003). The dynamics of pantothenate synthetase exhibit a circular motion where all the domains rotate back and forth around the active site, which allows residues 200 to 290 to rotate with a greater extent of flexibility (boxes in displacement graph, Figure ), to dictate the opening and closing of the active site. This lid-type mechanism of residues 200 to 290 is further confirmed based on its anticorrelated motion with the rest of the enzyme and active site, which lies within residues 30 to 50 (black square boxes in DCCM, Figure ).
3.8. Identification of test proteins
A set of 12 proteins (test No. 1–12) were used to examine if dynamic features of a protein could be used for its functional identification. Six of them (test No. 1–6) belong to the protein families reported in Figure but are from different species, and six of them (test No. 7–12) were chosen randomly. Using the Cartesian coordinates of these 12 proteins, DCCMs from single NMA were obtained and compared with the catalog of DCCMs reported in Table . We were able to correctly identify six proteins (test No. 1–6) by simple comparison of DCCMs. The test proteins No. 1–6 were matched to phosphoglycerate kinase, pyruvate kinase, enolase, arginase, fumarase, and amine oxidase, respectively (Figure ). A difference in the extent of correlation/anticorrelation can be seen in some cases such as in test protein No. 4 and arginase, while the DCCMs for test protein No. 6 and amine oxidase appear nearly identical. As expected, the DCCM of the six proteins, which is chosen randomly, did not match with any of the DCCMs reported in Table , suggesting that they belong to different families of proteins. As these test proteins (No. 7–12) exhibit different patterns of motions (Table S3) compared to the 24 proteins studied here, they must also possess different function. For example, we observed that aminoacyl-tRNA synthetases (Warren et al., Citation2014) and cytochrome P450 enzymes (Dorner et al., Citation2015) display very unique patterns of motion, and their functions are distinctly different from the set of proteins considered in the present study.
Figure 7. The six test proteins’ DCCMs are shown along with their matched protein representative DCCM.
4. Conclusion
The NMA study of 24 important enzymes has demonstrated that each enzyme has signature dynamic patterns and the intrinsic dynamics of proteins are conserved across species, from simple organisms like E. coli to the complex H. sapiens. As expected, it was observed that for a given family of proteins, as protein sequences and structures become more similar, the protein dynamics become more related as well. We successfully created a catalog of DCCMs for the 24 protein groups. These patterns are unique to each protein and have the potential to act as fingerprints of identification for unknown proteins. This catalog of intrinsic dynamics assisted us in the correct identification of six test proteins. These test proteins were successfully identified solely by comparing their respective dynamical patterns. Similar attempt to compare DCCMs of proteins was made earlier for dynamic comparison of proteins (Munz et al., Citation2010). However, Munz et al. have performed MD simulations and essential dynamic analysis to develop and compare DCCM of proteins. The present approach of using coarse-grained normal mode simulation is relatively faster than MD simulation-based protein dynamic comparison and could be useful for functional identification of uncharacterized proteins. Currently, one of the main challenges in the study of the proteome is the lack of an effective and timely method of protein function identification. A majority of known protein sequences and structures have remained largely unidentified. The Protein Structure Initiative (PSI), established in 2000 by the National Institutes of General Medical Sciences, resulted in thousands of structures of proteins whose functions remain unknown. Experimental investigation of protein function is costly and time-consuming. Here we have presented a computational method for predicting protein function. Coarse-grained NMA is a fast approach and effective way to identify and characterize the function of unknown proteins. Although coarse-grained NMA has some limitations, as it provides less detailed information about the local fluctuations compared to that obtained from all-atom NMA or MD simulations (Strom et al., Citation2014), the method is very useful for establishing this unique dynamical fingerprint, and it only requires the PDB coordinates of a protein. Therefore, this work could be extended to the greater scope of identifying the function of uncharacterized proteins.
In the present study, DCCMs of various proteins were only visually compared; however the advancement of computational analytical methods opens the possibility of an online catalog of dynamics. This study could be extended to include more metabolic enzymes and proteins from various functional classes. For the future, we anticipate the use of NMA and the role of protein dynamics to play a larger part in protein identification and classification.
Abbreviations and symbols | ||
BLAST | = | Basic local alignment search tool |
BC | = | Bhattacharyya coefficient |
DCCM | = | Dynamic cross-correlation matrix |
ENM | = | Elastic network model |
MAT | = | Methionine adenosyltransferase |
NMA | = | Normal mode analysis |
PDB | = | Protein Data Bank |
RMSD | = | Root-mean-square deviation |
RMSIP | = | Root-mean-square inner product |
VMD | = | Visual molecular dynamics |
Funding
The authors received no direct funding for this research.
Supplementary material
Supplementary material for this article can be acessed here http://dx.doi.org/10.1080/23312025.2017.1291877.
Supplementary_Materials_1_31_2017.docx
Download MS Word (3.1 MB)Additional information
Notes on contributors
Sanchita Hati
Our research is focused on the simple question - how protein motions influence substrate binding and catalysis. The relationship between protein dynamics and its role in function (molecular recognition, catalysis, and allostery) is an evolving perspective in enzymology. For the past several years, we have been investigating the interplay of protein dynamics and enzymatic processes using computations and experiments. In particular, we are exploring how dynamics of different domains modulate substrate recognition, catalysis, and allosteric communication in multi-domain enzymes. Our overall goal is to advance the drug design and screening process, by taking into consideration proteins’ intrinsic flexibility.
References
- Agarwal, P. K. (2005). Role of protein dynamics in reaction rate enhancement by enzymes. Journal of the American Chemical Society, 127, 15248–15256.10.1021/ja055251s
- Agarwal, P. K., Billeter, S. R., Rajagopalan, P. R., Benkovic, S. J., & Hammes-Schiffer, S. (2002). Network of coupled promoting motions in enzyme catalysis. Proceedings of the National Academy of Sciences, 99, 2794–2799.
- Allewell, N. M., Shi, D., Morizono, H., & Tuchman, M. (1999). Molecular recognition by ornithine and aspartate transcarbamylases. Accounts of Chemical Research, 32, 885–894.10.1021/ar950262j
- Altschul, S. F., Madden, T. L., Schäffer, A. A., Zhang, J., Zhang, Z., Miller, W., & Lipman, D. J. (1997). Gapped BLAST and PSI-BLAST: A new generation of protein database search programs. Nucleic Acids Research, 25, 3389–3402.10.1093/nar/25.17.3389
- Amadei, A., Ceruso, M. A., & Di Nola, A. (1999). On the convergence of the conformational coordinates basis set obtained by the essential dynamics analysis of proteins’ molecular dynamics simulations. Proteins: Structure, Function, and Genetics, 36, 419–424.10.1002/(ISSN)1097-0134
- Bahar, I., & Rader, A. J. (2005). Coarse-grained normal mode analysis in structural biology. Current Opinion in Structural Biology, 15, 586–592.10.1016/j.sbi.2005.08.007
- Banks, R. D., Blake, C. C. F., Evans, P. R., Haser, R., Rice, D. W., Hardy, G., … Phillips, A. W. (1979). Sequence, structure and activity of phosphoglycerate kinase: A possible hinge-bending enzyme. Nature, 279, 773–777.10.1038/279773a0
- Bennett, W. S., Jr, & Steitz, T. A. (1978). Glucose-induced conformational change in yeast hexokinase. Proceedings of the National Academy of Sciences, 75, 4848–4852.10.1073/pnas.75.10.4848
- Berg, J. M., Tymoczko, J. L., & Stryer, L. (2012). Biochemistry (7th ed.). New YorK, NY: W.H. Freeman and Company.
- Berman, H. M., Westbrook, J., Feng, Z., Gilliland, G., Bhat, T. N., Weissig, H., … Bourne, P. E. (2000). The protein data bank. Nucleic Acids Research, 28, 235–242.10.1093/nar/28.1.235
- Bhabha, G., Lee, J., Ekiert, D. C., Gam, J., Wilson, I. A., Dyson, H. J., … Wright, P. E. (2011). A dynamic knockout reveals that conformational fluctuations influence the chemical step of enzyme catalysis. Science, 332, 234–238.10.1126/science.1198542
- Bhattacharyya, A. (1943). On a measure of divergence between two statistical populations defined by their probability distributions. Bulletin of Calcutta Mathematical Society, 35, 99–109.
- Brooks, B., & Karplus, M. (1983). Harmonic dynamics of proteins: Normal modes and fluctuations in bovine pancreatic trypsin inhibitor. Proceedings of the National Academy of Sciences, 80, 6571–6575.10.1073/pnas.80.21.6571
- Carnevale, V., Pontiggia, F., & Micheletti, C. (2007). Structural and dynamical alignment of enzymes with partial structural similarity. Journal of Physics: Condensed Matter, 19, 285206–285214.10.1088/0953-8984/19/28/285206
- Chennubhotla, C., & Bahar, I. (2007). Signal propagation in proteins and relation to equilibrium fluctuations. PLOS Computational Biology, 3, 1716–1726.
- Chennubhotla, C., Yang, Z., & Bahar, I. (2008). Coupling between global dynamics and signal transduction pathways: a mechanism of allostery for chaperonin GroEL. Molecular BioSystems, 4, 287–292.10.1039/b717819k
- Chothia, C., & Lesk, A. M. (1986). The relation between the divergence of sequence and structure in proteins. The EMBO Journal, 5, 823–826.
- Davenport, R. C., Bash, P. A., Seaton, B. A., Karplus, M., Petsko, G. A., & Ringe, D. (1991). Structure of the triosephosphate isomerase-phosphoglycolohydroxamate complex: an analog of the intermediate on the reaction pathway. Biochemistry, 30, 5821–5826.10.1021/bi00238a002
- Devos, D., & Valencia, A. (2000). Practical limits of function prediction. Proteins: Structure, Function, and Genetics, 41, 98–107.10.1002/(ISSN)1097-0134
- Dorner, M. E., McMunn, R. D., Bartholow, T. G., Calhoon, B. E., Conlon, M. R., Dulli, J. M., … Hati, S. (2015). Comparison of intrinsic dynamics of cytochrome P450 proteins using normal mode analysis. Protein Science, 24, 1495–1507.
- Dunten, P., Belunis, C., Crowther, R., Hollfelder, K., Kammlott, U., Levin, W., … Wertheimer, S. J. (2002). Crystal structure of human cytosolic phosphoenolpyruvate carboxykinase reveals a new GTP-binding site. Journal of Molecular Biology, 316, 257–264.10.1006/jmbi.2001.5364
- Eisenmesser, E. Z., Millet, O., Labeikovsky, W., Korzhnev, D. M., Wolf-Watz, M., Bosco, D. A., … Kern, D. (2005). Intrinsic dynamics of an enzyme underlies catalysis. Nature, 438, 117–121.10.1038/nature04105
- Eklund, H., Nordström, B.Zeppezauer, E., Söderlund, G., Ohlsson, I., Boiwe, T., … Åkeson, Å (1976). Three-dimensional structure of horse liver alcohol dehydrogenase at 2.4 Å resolution. Journal of Molecular Biology, 102, 27–59.
- Erdin, S., Lisewski, A. M., & Lichtarge, O. (2011). Protein function prediction: towards integration of similarity metrics. Current Opinion in Structural Biology, 21, 180–188.10.1016/j.sbi.2011.02.001
- Fedøy, A. E., Yang, N., Martinez, A., Leiros, H. K. S., & Steen, I. H. (2007). Structural and functional properties of isocitrate dehydrogenase from the psychrophilic bacterium desulfotalea psychrophila reveal a cold-active enzyme with an unusual high thermal stability. Journal of Molecular Biology, 372, 130–149.10.1016/j.jmb.2007.06.040
- Frank, J., & Agrawal, R. K. (2000). A ratchet-like inter-subunit reorganization of the ribosome during translocation. Nature, 406, 318–322.10.1038/35018597
- Fu, Z., Hu, Y., Markham, G. D., & Takusagawa, F. (1996). Flexible loop in the structure of s-adenosylmethionine synthetase crystallized in the tetragonal modification. Journal of Biomolecular Structure and Dynamics, 13, 727–739.10.1080/07391102.1996.10508887
- Fuglebakk, E., Echave, J., & Reuter, N. (2012). Measuring and comparing structural fluctuation patterns in large protein datasets. Bioinformatics, 28, 2431–2440.10.1093/bioinformatics/bts445
- Fuglebakk, E., Tiwari, S. P., & Reuter, N. (2014). Comparing the intrinsic dynamics of multiple protein structures using elastic network models. Biochimica et Biophysica Acta, 1850, 911–922.
- Go, N., Noguti, T., & Nishikawa, T. (1983). Dynamics of a small globular protein in terms of low-frequency vibrational modes. Proceedings of the National Academy of Sciences, 80, 3696–3700.10.1073/pnas.80.12.3696
- Goward, C. R., & Nicholls, D. J. (1994). Malate dehydrogenase: A model for structure, evolution, and catalysis. Protein Science, 3, 1883–1888.10.1002/pro.v3:10
- Hammes-Schiffer, S., & Benkovic, S. J. (2006). Relating protein motion to catalysis. Annual Review of Biochemistry, 75, 519–541.10.1146/annurev.biochem.75.103004.142800
- Hensen, U., Meyer, T., Haas, J., Rex, R., Vriend, G., & Grubmüller, H. (2012). Exploring protein dynamics space: The dynasome as the missing link between protein structure and function. PLoS ONE, 7, e33931.doi:10.1371/journal.pone.0033931
- Henzler-Wildman, K. A., Lei, M., Thai, V., Kerns, S. J., Karplus, M., & Kern, D. (2007). A hierarchy of timescales in protein dynamics is linked to enzyme catalysis. Nature, 450, 913–916.10.1038/nature06407
- Henzler-Wildman, K., & Kern, D. (2007). Dynamic personalities of proteins. Nature, 450, 964–972.10.1038/nature06522
- Hester, G., Brenner-Holzach, O., Rossi, F. A., Struck-Donatz, M., Winterhalter, K. H., Smit, J. D., & Piontek, K. (1991). The crystal structure of fructose-1,6-bisphosphate aldolase from Drosophila melanogaster at 2.5 A resolution. FEBS Lett, 292, 237–242.
- Hinsen, K. (1998). Analysis of domain motions by approximate normal mode calculations. Proteins: Structure, Function, and Genetics, 33, 417–429.10.1002/(ISSN)1097-0134
- Hinsen, K., Petrescu, A. J., Dellerue, S., Bellissent-Funel, M. C., & Kneller, G. (2000). Harmonicity in slow protein dynamics. Chemical Physics, 261 25 –37.10.1016/S0301-0104(00)00222-6
- Hinsen, K., Thomas, A., & Field, M. J. (1999). Analysis of domain motions in large proteins. Proteins: Structure, Function, and Genetics, 34, 369–382.10.1002/(ISSN)1097-0134
- Hollup, S. M., Fuglebakk, E., Taylor, W. R., & Reuter, N. (2011). Exploring the factors determining the dynamics of different protein folds. Protein Science, 20, 197–209.10.1002/pro.558
- Hollup, S. M., Salensminde, G., & Reuter, N. (2005). WEBnm@: A web application for normal mode analysis of proteins. BMC Bioinformatics, 6, 52.10.1186/1471-2105-6-52
- Holm, L., & Rosenstrom, P. (2010). Dali server: Conservation mapping in 3D. Nucleic Acids Research, 38(Web Server), p. W545–W549.10.1093/nar/gkq366
- Humphrey, W., Dalke, A., & Schulten, K. (1996). VMD: Visual molecular dynamics. Journal of Molecular Graphics, 14, 33–38.10.1016/0263-7855(96)00018-5
- Ichiye, T., & Karplus, M. (1991). Collective motions in proteins: A covariance analysis of atomic fluctuations in molecular dynamics and normal mode simulations. Proteins: Structure, Function, and Genetics, 11, 205–217.10.1002/(ISSN)1097-0134
- Klinman, J. P., & Kohen, A. (2013). Hydrogen tunneling links protein dynamics to enzyme catalysis. Annual Review of Biochemistry, 82, 471–496.10.1146/annurev-biochem-051710-133623
- Kosloff, M., & Kolodny, R. (2008). Sequence-similar, structure-dissimilar protein pairs in the PDB. Proteins: Structure, Function, and Bioinformatics, 71, 891–902.10.1002/prot.v71:2
- Lebioda, L., & Stec, B. (1991). Mechanism of enolase: the crystal structure of enolase-magnesium-2-phosphoglycerate/phosphoenolpyruvate complex at 2.2-.ANG. resolution. Biochemistry, 30, 2817–2822.10.1021/bi00225a012
- Lemke, C. T., & Howell, P. L. (2001). The 1.6 Å crystal structure of E. coli argininosuccinate synthetase suggests a conformational change during catalysis. Structure, 9, 1153–1164.10.1016/S0969-2126(01)00683-9
- Lin, Y. Z., Liang, S. J., Zhou, J. M., Tsou, C. L., Wu, P., & Zhou, Z. (1990). Comparison of inactivation and conformational changes of d-glyceraldehyde-3-phosphate dehydrogenase during thermal denaturation. Biochimica et Biophysica Acta (BBA) - Protein Structure and Molecular Enzymology, 1038, 247–252.10.1016/0167-4838(90)90212-X
- Lisi, G. P., & Patrick Loria, J. P. (2016). Using NMR spectroscopy to elucidate the role of molecular motions in enzyme function. Progress in Nuclear Magnetic Resonance Spectroscopy, 92-93, 1–17.10.1016/j.pnmrs.2015.11.001
- Liu, X., Speckhard, D. C., Shepherd, T. R., Sun, Y. J., Hengel, S. R., Yu, L., … Fuentes, E. J. (2016). Distinct roles for conformational dynamics in protein-ligand interactions. Structure, 24, 2053–2066.10.1016/j.str.2016.08.019
- Lu, G., Dobritzsch, D., Baumann, S., Schneider, G., & König, S. (2000). The structural basis of substrate activation in yeast pyruvate decarboxylase. European Journal of Biochemistry, 267, 861–868.10.1046/j.1432-1327.2000.01070.x
- Marques, O., & Sanejouand, Y. H. (1995). Hinge-bending motion in citrate synthase arising from normal mode calculations. Proteins: Structure, Function, and Genetics, 23, 557–560.10.1002/(ISSN)1097-0134
- Mattevi, A., Bolognesi, M., & Valentini, G. (1996). The allosteric regulation of pyruvate kinase. FEBS Letters, 389, 15–19.10.1016/0014-5793(96)00462-0
- Micheletti, C. (2013). Comparing proteins by their internal dynamics: Exploring structure–function relationships beyond static structural alignments. Physics of Life Reviews, 10(1), 1–26.10.1016/j.plrev.2012.10.009
- Munz, M., Lyngsø, R., Hein, J., & Biggin, P. C. (2010). Dynamics based alignment of proteins: an alternative approach to quantify dynamic similarity. BMC Bioinformatics, 11, 188.10.1186/1471-2105-11-188
- Pandini, A., Mauri, G., Bordogna, A., & Bonati, L. (2007). Detecting similarities among distant homologous proteins by comparison of domain flexibilities. Protein Engineering Design and Selection, 20, 285–299.
- Park, J., Teichmann, S. A., Hubbard, T., & Chothia, C. (1997). Intermediate sequences increase the detection of homology between sequences. Journal of Molecular Biology, 273, 349–354.10.1006/jmbi.1997.1288
- Roston, D., Kohen, A., Doron, D., & Major, D. T. (2014). Simulations of remote mutants of dihydrofolate reductase reveal the nature of a network of residues coupled to hydride transfer. Journal of Computational Chemistry, 35, 1411–1417.10.1002/jcc.v35.19
- Sievers, F., Wilm, A., Dineen, D., Gibson, T. J., Karplus, K., Li, W., … Higginsa, D. G. (2011). Fast, scalable generation of high-quality protein multiple sequence alignments using Clustal Omega. Molecular Systems Biology, 7, 539.
- Singh, P., Francis, K., & Kohen, A. (2015). Network of remote and local protein dynamics in dihydrofolate reductase catalysis. ACS Catalysis, 5, 3067–3073.10.1021/acscatal.5b00331
- Skjaerven, L., Hollup, S., & Reuter, N. (2009). Normal mode analysis for proteins. Journal of Molecular Structure: THEOCHEM, 898, 42–48.10.1016/j.theochem.2008.09.024
- Stoddard, B. L., Dean, A., & Koshland, D. E., Jr (1993). Structure of isocitrate dehydrogenase with isocitrate, nicotinamide adenine dinucleotide phosphate, and calcium at 2.5-.ANG. resolution: A pseudo-Michaelis ternary complex. Biochemistry, 32, 9310–9316.10.1021/bi00087a008
- Strom, A. M., Fehling, S. C., Bhattacharyya, S., & Hati, S. (2014). Probing the global and local dynamics of aminoacyl-tRNA synthetases using all-atom and coarse-grained simulations. Journal of Molecular Modeling, 20, 2245.10.1007/s00894-014-2245-1
- Tama, F., & Brooks, C. L. (2005). 3rd diversity and identity of mechanical properties of icosahedral viral capsids studied with elastic network normal mode analysis. Journal of Molecular Biology, 345, 299–314.10.1016/j.jmb.2004.10.054
- Tiwari, S. P., Fuglebakk, E., Hollup, S. M., Skjærven, L., Cragnolini, T., Grindhaug. S. H., … Reuter, N. (2014). WEBnm@ v2.0: Web server and services for comparing protein flexibility. BMC Bioinformatics, 15, 6597.
- Tobi, D. (2013). Large-scale analysis of the dynamics of enzymes. Proteins: Structure, Function, and Bioinformatics, 81, 1910–1918.10.1002/prot.24335
- Tousignant, A., & Pelletier, J. N. (2004). Protein motions promote catalysis. Chemistry & Biology, 11, 1037–1042.10.1016/j.chembiol.2004.06.007
- Vöhringer-Martinez, E., & Dörner, C. (2016). Conformational substrate selection contributes to the enzymatic catalytic reaction mechanism of pin1. The Journal of Physical Chemistry B, 120, 12444–12453.10.1021/acs.jpcb.6b09187
- Wang, S., & Eisenberg, D. (2003). Crystal structures of a pantothenate synthetase from M. tuberculosis and its complexes with substrates and a reaction intermediate. Protein Science, 12, 1097–1108.10.1110/(ISSN)1469-896X
- Warren, N., Strom, A., Nicolet, B., Albin, K., Albrecht, J., Bausch, B., … Gunderson, A. (2014). Comparison of the Intrinsic Dynamics of Aminoacyl-tRNA Synthetases. The Protein Journal, 33, 184–198.10.1007/s10930-014-9548-z
- Weimer, K. M., Shane, B. L., Brunetto, M., Bhattacharyya, S., & Hati, S. (2009). Evolutionary basis for the coupled-domain motions in thermus thermophilus leucyl-tRNA synthetase. Journal of Biological Chemistry, 284, 10088–10099.10.1074/jbc.M807361200
- Whisstock, J., & Lesk, A. (1999). Prediction of protein function from protein sequence and structure. Quarterly Reviews of Biophysics, 36, 307–340.10.1017/S0033583503003901
- Wiegand, G., & Remington, S. J. (1986). Citrate synthase: Structure, control, and mechanism. Annual Review of Biophysics and Biophysical Chemistry, 15, 97–117.10.1146/annurev.bb.15.060186.000525
- Winn, S. I., Watson, H. C., Harkins, R. N., & Fothergill, L. A. (1981). Structure and activity of phosphoglycerate mutase. Philosophical Transactions of the Royal Society of London B: Biological Sciences, 293, 121–130.
- Wolodko, W. T., Fraser, M. E., James, M. N., & Bridger, W. A. (1994). The crystal structure of succinyl-CoA synthetase from Escherichia coli at 2.5-A resolution. The Journal of Biological Chemistry, 269, 10883–10890.
- Yang, L., Song, G., Carriquiry, A., & Jernigan, R. L. (2008). Close correspondence between the motions from principal component analysis of multiple HIV-1 Protease structures and elastic network modes. Structure, 16, 321–330.10.1016/j.str.2007.12.011
- Zen, A., Micheletti, C., Keskin, O., & Nussinov,, R. (2010). Comparing interfacial dynamics in protein-protein complexes: an elastic network approach. BMC Structural Biology, 10, 26.10.1186/1472-6807-10-26