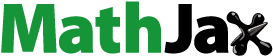
Abstract
One of the most important applications of remote sensing is presented in mining and exploration of mineral deposits and evaluation of prospective targets. This project discusses how to use remote sensing knowledge in order to make classification and separation of surface rocks in the Dir-o-Morreh mine. The main purpose of this research is to identify the areas containing high-quality basalt. In this regard, we utilize ASTER multi-spectral satellite imagery, which has relatively good spectral and spatial resolution. At the first step, in order to achieve the correct spectral composition of the basalt spectrum, the spectral signature of basalt stone, defined by Johns Hopkins University, was used. Afterward, the basalt extraction index (BEI) was defined regarding the behavior of the ASTER satellite image bands as well as the initial data provided by the owners of the intended study area. Then, the Convolution and Morphology filter was applied over the images to extract high-quality basalt using an appropriate color composition of the images. At the next step, in order to have better visualization, different maps containing different classes were created using the Maximum Likelihood algorithm. Finally, two indices were developed regarding all research data and field investigations in order to extract basalt zones. The first index discovers basalt zones in the study area, and the second one classifies high-quality basalt and altered basalt zones.
Public Interest Statement
One of the most important applications of remote sensing is presented in mining and exploration of mineral deposits, and evaluation of prospective targets. This project discusses how to use remote sensing knowledge in order to make classification and separation of surface rocks in a mining area. The main purpose of this research is to identify the areas containing high-quality basalt. In this regard, we utilize ASTER multi-spectral satellite imagery, which has relatively good spectral and spatial resolution. Then, the basalt extraction index (BEI) was defined regarding the behavior of the ASTER satellite image bands. At the next step, in order to have better visualization, different maps containing different classes were created. Finally, two indices were developed regarding all research data and field investigations. The first index discovers basalt zones in the study area, and the second one classifies high-quality basalt and altered basalt zones.
1. Introduction
Lavas are formed through the exit of magma as well as molten and semi-fluid minerals from the mantle to the Earth’s surface. Basalt as a typical example of lavas contains 50–54% of silica and less than 10% of calcium and potassium feldspars. In the case of chemical composition of this material with other materials such as clay and lime (in the early stages of rock formation), mechanical strength and other physical properties will be changed and its economic value will be reduced. Having this in mind, we can see that basalts, which have less chemical and mechanical changes, have higher quality. In other words, the target and objective of mining operations are these rocks. In our case, the objective of basalt excavation is to use it in highway and railroad constructions as well as use in building and construction materials. Consequently, high-quality basalt, which would satisfy the standards of the construction materials, was investigated. Using remote sensing knowledge is one of the methods to identify mineral hotspots. Many researches have been done in this field (Amer, El Mezayen, & Hasanein, Citation2016; Asran, Emam, & El-Fakharani, Citation2017; Pour, Hashim, Hong, & Park, Citationin press; Rajendran & Nasir, Citation2017; Tayebi, Tangestani, Vincent, & Neal, Citation2014).
For example, a research entitled “Application of remote sensing in the field of ore deposits” was done by Asgari, Alimohammadi, and Nasiri (Citation2014), which investigated the capabilities of ASTER and the Hyperion EO-1 images to detect alteration of mineral deposits. In this research, some methods such as Minimum Noise Fraction (MNF) index, color staining False-Color Composite (FCC), Spectral Angle Mapper (SAM), and Principal Components Analysis (PCA) have been used. The research determined that the use of SWIR Bandwidth of Advanced Space borne Thermal Emission and Reflection Radiometer (ASTER) could be a suitable tool for identifying mineral deposits (Asgari et al., Citation2014).
In 2011, Masoudi et al. investigated the application of remote sensing for finding Pumice in the Damavand Mountain area (Masoudi, Fereydouni, & Matkan, Citation2010). In that study, Indian Remote Sensing (IRS), ASTER, and Landsat 7 Enhanced Thematic Mapper Plus (ETM+) images were used. It was also found that the spectral range of the SWIR sensor is efficient in identifying rocks. They have used false color composite, HIS color conversion, principal component analyses, and optimal index factor (OIF) in their research (Masoudi et al., Citation2010).
In 2002, Christopher Legg introduced remote sensing as one of the most effective methods in mining exploration in the book “Remote Sensing and Geographic Information Systems” (Legg, Citation1994).
In 2013, Mitchell et al. conducted research investigating single and multi-date Landsat classifications of basalt to support soil survey efforts in western US. This study also uses color mixing techniques as well as band ratios, and NDVI. The reason for using this index is to identify the numerical values on thresholds between vegetation and non-vegetation areas during classification, and eliminate vegetation in order to improve the detection of lithology. In this study, thermal bands and comparisons with other bands are recommended in order to reduce the errors. The reason for using images with different dates was to examine the effects of the difference in the sun’s radiation angle. Afterward, the classified maps have been generated using supervised classification, and defining the training data-sets to make the classification (Mitchell, Shrestha, Moore-Ellison, & Glenn, Citation2013).
Markoski and Rolim used the ASTER (Level 1A) for the classification of igneous rocks, especially basalt. The low spatial resolution of the ASTER satellite was reported as one of the most important problems in that project. They looked for basalt matching the spectral signature defined by NASA. After finding these zones, supervised classification was performed using the Maximum Likelihood algorithm on the examined points in order to provide a distribution map. The resulting map became an indicator for finding basalts in the state of Rio Grande do Sul in Brazil (Markoski & Rolim, Citation2014).
In 2015, Ozen-Corumluoglu and his research colleagues used remote sensing techniques to focus on basalt extraction in the Kola region of Turkey (Corumluoglu, Vural, & Asri, Citation2015). In this study, they used color composites and banding techniques to achieve the most desirable result. They were able to achieve the appropriate color composites in the project to determine the basalt area and boundaries (Corumluoglu et al., Citation2015). Therefore, in this paper, we try to use the technology of remote sensing to reach the basalt zones with the highest possible economic quality.
The study area is the Dir-o-Morreh mine where a massive dike has intruded a marble bed. According to preliminary studies and field investigations, the Morreh Mountain consists of sequential basalt dikes intruding marine calcareous deposits. This intrusive mass converted the calcareous deposits to marble. Extrusive igneous rocks erupt onto the surface form basaltic dikes, where they cool quickly to form small crystals. Upon moving up basaltic dikes, the clay soil in the study area has created conditions that result in a series of low-grade basalt. The first purpose of this study is to provide a map of the study area showing the basalt zones. As a secondary purpose, high-quality and low-grade basalts will be distinguished.
In this paper, remote sensing has been used to identify the lithology on the surface to explore basalt minerals. ASTER multi-spectral satellite images have been utilized. This satellite provides appropriate spatial and spectral resolution to identify basalt outcrops (Amoosoltani & Bagheri, Citation2014). Then, two indices to extract basalt have been proposed. The first index, BEI, was introduced to find basalt area from other mineral deposits in the searching area based on the NIR data provided by ASTER. The second, EBEI, was an enhanced index, which has used the color composites, and the results that extracted from the first index to enhance the basalts zones, and classify the quality of the previous found areas.
2. Study area (Dir-o-Morreh Region)
The study area is located in Tehran Province, 52 km south of the Tehran city and 22 km to the south of Hassan Abad of Fashafooia and Shams Abad Town. The mine is located between 35° 09′ 30″ and 35° 11′ 6″ N and 51° 11′ 1″ and 51° 16′ 49″ E geographical coordinate system. Figure shows the mine location with the most important natural phenomena in the study region, along with the geographical location of the province boundary. As it is located in the desert, the region climate is hot and dry during the summer and freezing cold in the winter. There is no vegetation growing in the area. Hence, regarding the mentioned geographic location, clouds and aerosols are not a problem for remote sensing operations during different seasons of the year. In terms of geology, the area is located in the Alborz geological zone. The oldest rocks in the region belong to the Eocene period, and geological surveys show that almost all surface rocks belong to the post Eocene period, as shown by the fossils that have been discovered.
There are three main categories of rocks in the area, which include basalt, Limestone, and marble (altered limestone). The existing basalts in the region are commonly oxidized. Hence, their physical and chemical properties have changed. This process has also affected the basalt specifications in this area, and the technical quality, which is expected, has reduced. Therefore, one purpose of this study is to identify high-quality basalts, which is not altered basalt. The targeted high-quality basalt satisfies the standards of the road, railway, and building construction materials. Afterward, in the next step, the information and data used are dependant on extracted types of basalt stones. In this paper, it should be said when we discuss how to obtain basalt, the goal is to get it in high quality. Figure shows the overall view of the study area, and Figure shows an overview of the primary excavation of a basalt area. In this figure, the targeted basalts are being mined.
3. Data and method
3.1. Images
ASTER (Level 1A) satellite images are particularly applied by researchers due to their satisfactory spectral resolution and high capability to produce acceptable images, and also being free of charge. Consequently, they were used as the primary data-set in this study. ASTER satellite can create images with 14 different bands, which are 9 optical and 5 thermal bands. Six bands out of the nine optical bands are shortwave infrared (SWIR), and the remaining three bands are visible and near infrared bands (California Institute of Technology, Citation2001; Department of the Interior U.S. Geological Survey, Citation2015). In order to reduce atmospheric effects like cloudy weather, the images, which were captured in the summer (August), have been used in this study. Furthermore, thermal bands have not been used in this study due to their low spatial resolution (Safari & Manouchehri Nia, Citation2017).
3.2. Preprocessing
The electromagnetic energy of the Sun’s irradiation is reflected to the satellite sensor after earth surface’s incidence and scattering. This electromagnetic energy is also reflected from the atmosphere components during its sky path to the satellite. These frequent scatterings make some noise on the captured images (Shayan, Zare, Yamani, & Sharifi Kia, Citation2014).
It is necessary to eliminate the destructive atmospheric and radiometric effects before starting to analyze and evaluate the implementation of remote sensing studies. It was performed to minimize these effects by transforming the image digital numbers to radiance and reflectance values. Figure shows a sample spectral radiance at a sensor of the study area that contains basalt rocks before applying any corrections. As it is clear from Figure , there is no phenomenon detectable without making image corrections. Almost all bands have the same values, and no specific trend is observed.
Figure 4. Spectral radiance of the study area containing basalt rocks without applying any correction.
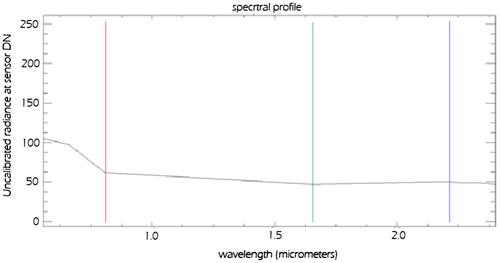
ASTER provides image data at different levels to the users and applies specific processes on its data. Regarding mentioned points, all necessary corrections were applied to the received ASTER (Level 1A) images. At the first step, atmospheric and radiometric corrections were applied to the images.
The atmospheric and radiometric corrections are applied to reduce undesirable surface and atmosphere impacts on electromagnetic radiance reflected on the satellite sensor, and generated digital numbers on images. Some examples of undesirable impacts include sensor sensibility over changing surface materials and phenomena and Sun altitude during the imaging. (Legg, Citation1994). It is necessary to say that these corrections are conducted according to the standard calibration data that are provided by the NASA metadata for ASTER images. Figure depicted the radiometric corrections, which have been applied to an image section containing basalt rocks. As it displays, due to the corrections, the wavelength range has been completely changed. It makes a significant contribution to the upcoming analysis during the next investigation steps.
In the next step, in order to increase the accuracy of investigations, and reduce the undesirable scattering impacts over the reflected waves, the Quick Atmospheric Correction was utilized over the image data-set. Quick Atmospheric Correction is an in-scene approach to atmospheric correction and determines parameters directly from the information contained within the scene using the observed pixel spectra. Quick Atmospheric Correction is based on the empirical finding that the average reflectance of diverse material spectra (excluding highly structured materials such as vegetation, shallow water, and mud) is not dependent on each scene. As the name implies, this correction is applied to the data to achieve the minimum reduction of unwanted atmospheric impacts (Darvishi Blvrany, Rashidian, Arsnjany, & Shaygan, Citation2012).
Figure shows the basalt surface spectral profile after applying atmospheric and radiometric corrections.
4. Research findings
4.1. Main processes
In order to find appropriate band combination for basalt investigation, the basalt spectral signature, which was derived from the spectral library of Johns Hopkins University, was used. Figure illustrates this spectral signature. Consider that this spectral signature has been measured in laboratory condition. Consequently, due to the focus of the present study on non-thermal bands, the obtained signature may not exactly fit the basalt spectral signature, and it may have some differences in some parts.
Figure 7. Reflected spectrum defined for basalt by Johns Hopkins University (Markoski & Rolim, Citation2014)
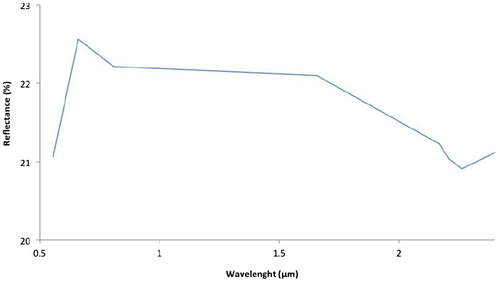
According to the basalt spectral signature, the spectral behavior of ASTER bands, and the initial ground surveyed data, the basalt extraction index (BEI) is proposed as follows:
The obtained results of applying the BEI show that the outcome image specifies the initial basalt identifying points. Hence, in this step, the ground fields that contain basalt rocks have been extracted. The reason for considering band numbers 4, 5, 6, and 8 in the proposed BEI formula was the probability of these bands to specify the clay, and lime in the study area, alongside this fact that they have the biggest spectral difference with the NIR band (B3).
Afterward, the 20% High Pass Convolution and Morphology filter with the kernel size of 11 was applied over the produced image of applied proposed index. Figure depicted the obtained binary image, which just separates basalt zones from non-basalt areas. Dark points in the image are defined as the locations containing basalt. Figure shows the enhanced image after applying the mentioned filter. Here, there are no classification results to identify the quality of basalt.
In the next step, in order to distinguish high-quality basalt from low-quality ones, an appropriate color composite was defined using the behavior of various bands of ASTER, as well as using the previous step results, which were obtained by applying the BEI to clarify the areas that contain basalt. During the study of the appropriate composition, it was observed that the maximum basalt reflection occurs in the infrared band (B3), and the clay and lime appeared in the bands 4 and 6 of RGB color composite (R = 3, G = 4, B = 6). This effect caused the basaltic area to be seen as red in the resultant image. Fortunately, the results of color composite investigation and applied filter are mutually confirmed.
According to the results provided by applying the filter (based on BEI) and proposed color composite, the classified basalt area with the same properties has been chosen and verified by field survey. In addition, the impact of basalt dust can be ignored in the study area because the operation site has been under operation for a long time, and almost all basalt out-crops were stripped. During the field operation, the extracted points of applying the proposed BEI were identified in the field, and the rock samples of extracted zone have been confirmed by XRD analysis tests. Furthermore, it was observed that the derived image of applying the BEI and the color composite image accurately confirmed the field observations. In other words, the spectral behavior of 35 points of 40 observed points has a spectral similarity to the Johns Hopkins library, applied BEI, color composite, and the field data. Next, new study zones were selected in order to help subsequent analysis and increase the accuracy of the investigations. The determination of new zones was based on the following points: the area has not been located in the shadow, not having a high slope, and was widespread in the study area (according to spatial resolution of the sensor).
Therefore, after confirming the results, the classification and map generation were performed in the next steps. It should be noted that during the field operations, a number of points were selected to determine the soil and calcareous marble in order to reduce the error as well as ensure the accuracy of the classification carried out in the next stage.
4.2. Classification
During image classification, each pixel is assigned to be a member of a class in the classified image. In general, image classification is performed in three categories of unsupervised, supervised, and object-oriented classification methods. The unsupervised classification is performed using specific software algorithms using ENVI software, which the user operator does not have many controls on the algorithm. Unlike the unsupervised algorithm, in supervised classification, the user introduces specific groups of pixels to be categorized and represents a specific type of land cover. The classification algorithm starts to search an image to find pixels with similar spectral properties. In this paper, the supervised classification method was used, and two different maps with two different classifications were created in order to have better clarification. In the first map, the study area was classified into five categories. According to the color combination and spatial signature of the images, these classes were: (a) high-quality basalt; b) low-quality basalt; (c) clay; (d) limestone; and (e) marble. A map of the five classifications is shown in Figure .
As shown in Figure , the basalt mass (including high-quality basalt in black and low-quality and weathered basalt in yellow) is surrounded by clay soils (red). According to the geological reports as well as local surveys, the calcareous/marble zones surround the massif of intrusive basalt dikes. Accordingly, the calcareous layers, beside the basalt dikes, have been baked and turned to marble layers in this area.
In the next step, in order to better represent the map and better interpret the study area, the classification of three classes was implemented. In this classification, due to less importance of soil types in this study, as well as the proximity of soil and lime layers with high-quality basalt zones, different soil classes are combined together, which means that instead of two classes of clay soils and calcareous soils, one combined class of soil was used. Similarly, in order to highlight the basalt zones, and making the ground surveys easier to perform, two basalt zones were combined, and a unique basalt class was created. The third class in the new classification was marble. An overview of the map of three new categories is shown in Figure . It should be noted that soil and marble classes were determined according to local surveys.
For preparation of classification maps (Figures and ), the Maximum Likelihood algorithm was used. These maps completely confirmed the color combination maps of previous steps, as well as the filter and index result maps. The Maximum Likelihood method is a supervised classification that uses a statistical method for classification to assign each pixel to a class. Classifiers like minimum distance and maximum likelihood use these statistical approaches, and these are generally able to handle a large number of categories simultaneously (Legg, Citation1994).
4.3. The enhanced basalt extraction index
In order to improve the accuracy, and to classify the extracted basalt areas to high- and low-quality basalt, a new enhanced index, Enhanced Basalt Extraction Index (EBEI), was proposed using the image obtained from the color combination, the proposed index in the previous steps, and the ground points taken from the field surveys:
Then, to distinguish high-quality basalt, a threshold was applied over the results that were obtained by applying the proposed EBEI for classifying the quality of extracted basalt area. The value range of the map, which was calculated by applying the EBEI, was from 0.60 to 1.25, and the applied threshold range for extracting high-quality basalt was from 1.16 to 1.25. This numerical range has been confirmed by field observation and laboratory tests for defined properties of high-quality basalt and XRD analysis tests. This step helps represent the final image of basalt zones. Figure shows the final image result by applying the proposed EBEI. As demonstrated in Figure , the red color represents the objective high-quality basalt zones.
Based on the final results of the applied index, the area of high- and low-quality basalt was calculated; the results are presented in Table . The results show that low-quality zones are four times greater in area than high-quality zones.
Table 1. The area of the high-quality and low-quality basalt
Field survey and GPS control were again performed to double check and confirm the obtained results. The classified zones were: high-quality basalt, weathered and low-quality basalt, soil (lime and clay), and marble. The results of the field operations strongly confirmed the classification.
Finally, it is important to mention that the main objective of this study is to identify high-quality basalts on the surface of the earth and it does not represent the type of the basaltic layers in depth. In addition, consider that areas containing low-quality basalts on the surface can contain high-quality basalts at depth (even at depth of less than one meter). This situation has occurred in one of the trenches, which was drilled in non-target basalt layers.
5. Conclusion and discussion
The main objective of this study is to prepare a classified map using multi-spectral images to provide high capability of specifying high-quality basalt from the low-quality and weathered basalt in the study area’s surface. The field data of this study were generated from mining excavation and field observations. Afterward, in the next step, the spectral plot of the ground points was compared with a spectral chart approved by Johns Hopkins University for basalt stones. After confirming the spectral behavior of the sample basalts, Johns Hopkins University’s spectral signature was used to provide an indicator for separating points and find the basalt zones. The proposed index was a useful aid in separating the basalt from other zones of rocks (mainly lime, marble, and weathered basalt). The next step was to rebuild the Convolution and Morphology filter in order to obtain an RGB color combination.
According to the map, which was prepared at this step, a field survey was performed on the study area, which confirmed the obtained results and prepared maps. To improve certainty and correctness, supervised classification was applied. This step was done to increase the accuracy of high-quality basalt separation from the clay, lime stone, marble rocks, and low-quality altered basalts.
In the last step, two maps were divided into three and five classes. Finally, using the proper number of points with appropriate distribution, and the maps prepared in previous steps, the optimized index was proposed. The final resultant map separates high-quality basalt zones in the surface from other mineral deposits in the study area like weathered basalt, marble, limestone, and clay.
Final results show that there is high amount of basalt on northern and northwestern parts of the studied mine. So, it would suggest to change the extraction plan of the mine to these zones. This helps extract basalt using less volume of digging and excavation operations. Furthermore, the final results highlight the northern part of study area to contain high acceptable amounts of high-quality basalt, which leads the beneficiary of the mine to focus on these zones for extraction operations.
Funding
The authors received no direct funding for this research.
Additional information
Notes on contributors
Meysam Argany
Meysam Argany received his BSc degree in surveying and geomatics engineering, and MSc degree (with the first rank among all graduate students) in remote sensing from the Faculty of Engineering, University of Tehran, Tehran, Iran, in 2004 and 2007, respectively. He received his PhD degree in Geographic Information System (GIS) from the Département des Sciences Géomatiques, Faculté de Foresterie, de Géographie et de Géomatique, Université Laval, Québec, QC, Canada in 2015. Since 2016, he has been an assistant professor with the Department of Remote Sensing and GIS, Faculty of Geography, University of Tehran, Tehran, Iran. He is also an invited researcher at the Département des Sciences Géomatiques, Faculté de Foresterie, de Géographie et de Géomatique, Université Laval, Québec, QC, Canada. His research interests are modeling, analysis, and optimization algorithms for deployment and coverage analysis of geosensor networks. He also works on satellite image processing, spectral analysis, and mineral mapping.
References
- Amer, R., El Mezayen, A., & Hasanein, M. (2016). ASTER spectral analysis for alteration minerals associated with gold mineralization. Ore Geology Reviews, 75, 239–251.10.1016/j.oregeorev.2015.12.008
- Amoosoltani, S., & Bagheri, H. (2014). Remote sensing application on identification and separation of mineralization of copper and gold alterations over the South West of Ardestan. Second National Conference of Applied Petrology, Isfahan Khourasgan IAU, Isfahan, Iran.
- Asgari, J., Alimohammadi, A., & Nasiri, Y. (2014). Application of remote sensing in mineral deposit exploration. National Conference of Advanced Models on Spatial Analysis in Land Use Planning, Yazd IAU.
- Asran, A. M., Emam, A., & El-Fakharani, A. (2017). Geology, structure, geochemistry and ASTER-based mapping of Neoproterozoic Gebel El-Delihimmi granites, Central Eastern Desert of Egypt. Lithos, 282–283, 358–372.10.1016/j.lithos.2017.03.022
- California Institute of Technology. (2001). ASTER higher-level product user guide. Japan and USA: Jet propulsion Laboratory California Institute of Technology.
- Corumluoglu, O., Vural, A., & Asri, I. (2015). Determination of Kula basalts (geosite) in Turkey using remote sensing techniques. Arabian Journal of Geosciences , 8(11), 10105–10117.10.1007/s12517-015-1914-4
- Darvishi Blvrany, A., Rashidian, A., Arsnjany, J., & Shaygan, M. (2012). Study the application of science and technology of remote sensing and GIS in the health system (Part I: Overview of external sources). Hakim, 15(2), 87–100.
- Department of the Interior U.S. Geological Survey. (2015). USGS. South Dakota: USGS EROS Data Center Sioux Falls.
- Legg, C. (1994). Remote sensing and geographic information systems: Geological mapping, mineral exploration and mining. Hoboken, NJ: Wiley. Retrieved from https://books.google.com/books/about/Remote_Sensing_and_Geographic_Informatio.html?id=iXHxAAAAMAAJ
- Markoski, P., & Rolim, S. (2014). Evaluation of ASTER images for characterization and mapping of volcanic rocks (Basalts). Remote Sensing and GIS, 3(1), 486–498. doi:10.5194/isprsannals-I-7-153-2012
- Masoudi, S., Fereydouni, F., Matkan, A. (2010). Application of remote sensing on pumice exploration near the damavand mountain. Journal of Earth Sciences, 1–8, 2010
- Mitchell, J. J., Shrestha, R., Moore-Ellison, C. A., & Glenn, N. F. (2013). Single and multi-date landsat classifications of basalt to support soil survey efforts. Remote Sensing, 5(10), 4857–4876. doi:10.3390/rs5104857
- Pour, A. B., Hashim, M., Hong, J. K., & Park, Y. (in press). Lithological and alteration mineral mapping in poorly exposed lithologies using Landsat-8 and ASTER satellite data: North-eastern Graham Land, Antarctic Peninsula. Ore Geology Reviews, doi:10.1016/j.oregeorev.2017.07.018.
- Rajendran, S., & Nasir, S. (2017). Characterization of ASTER spectral bands for mapping of alteration zones of volcanogenic massive sulphide deposits. Ore Geology Reviews, 88, 317–335.10.1016/j.oregeorev.2017.04.016
- Safari, M., & Manouchehri Nia, M. (2017). Tracking the Prospecting Mineral Area using ASTER Satellite Images, Case Study: Salaran Copper Mine. 10th National Conference of Geology, Payam Nour University, Tabriz, Iran.
- Shayan, S., Zare, G. H., Yamani, M., & Sharifi Kia, M. (2014). Boushehr mand delta change detection using remote sensing data. Journal of Geographic Information, 93, 27–40.
- Tayebi, M. H., Tangestani, M. H., Vincent, R. K., & Neal, D. (2014). Spectral properties and ASTER-based alteration mapping of Masahim volcano facies, SE Iran. Journal of Volcanology and Geothermal Research, 287, 40–50.10.1016/j.jvolgeores.2014.09.013