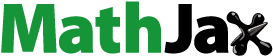
Abstract
Preeclampsia is a hypertensive disorder of pregnancy that affects 2–8% of pregnant women. The purpose of this study was to identify factors associated with hypertension measurements and time-to-onset of preeclampsia among pregnant women attending antenatal care service at Arerti Primary Hospital. Separate and joint methods of longitudinal data analysis and survival data analysis were fitted to answer the research objectives. A retrospective longitudinal study design was employed on a total of 201 pregnant women attending the antenatal clinic of Arerti Primary Hospital between September 2018 and June 2019. To analyze our data we employed descriptive method, linear mixed effect model, Cox-PH model and joint models for longitudinal and survival outcomes. Relevant demographic and clinical covariates were included in sub models. This study revealed that baseline age, visiting times, weight, diabetes, history of Preeclampsia and parity had significantly associated with mean change in the blood pressure measurements. From the Cox model result, age, weight, history of Preeclampsia and marital status were associated with a significant hazard of developing preeclampsia. The uni-variate joint models reveal that each longitudinal blood pressure measurements are significantly associated with hazard of developing preeclampsia. Form the bivariate joint model; only diastolic blood pressure is significantly associated with risk of developing Preeclampsia. As the result obtained in this study, we summarized that, age, weight, history of Preeclampsia and marital status had a significant effect on time to developing preeclampsia. Furthermore, due to significance of association between the longitudinal blood pressure measurements and time to onset of preeclampsia, joint model analysis was suggested as it incorporates all information simultaneously and provides valid and efficient inferences over separate models analysis.
PUBLIC INTEREST STATEMENT
Preeclampsia is the leading causes of maternal and perinatal morbidity and mortality worldwide and it is a hypertensive disorder which usually occurs after 20 weeks of gestation. It is a rapidly progressive condition characterized by elevated blood pressure and protein in the urine. Ethiopia is implementing Maternal, Newborn, and Child Health programs as a priority health agenda to reduce maternal and child morbidity and mortality. Identifying potential risk factors and the group of a population at risk is imperative to achieve Maternal, Newborn, and Child Health programs. Therefore, the finding from the analysis of longitudinal responses and survival time to onset of preeclampsia of pregnant women could be important for pregnant women, health programmers, antenatal care service providers and future researchers in prevention and management of PE.
Competing interests
All authors declare no competing interest.
1. Background
Preeclampsia (PE) is a hypertensive disorder of pregnancy that affects 2–8% of pregnant women worldwide and characterized by elevated blood pressure and proteinuria in the urine during second or third trimester of gestation (Ghulmiyyah & Sibai, Citation2012). It most commonly develops during the last trimester, but it can happen at any time in the second half of pregnancy, during labor, or even up to six weeks after delivery. It is a serious and poorly understood complication of pregnancy, which can progress to preeclampsia and health problems among mothers and their babies (Choudhury & Friedman, Citation2012).
The World Health Organization (Citation2011) and American College of Obstetricians and Gynecologists (Citation2013) develop guidelines for the prevention, early diagnosis, and management of PE and other
Hypertensive Disorders of pregnancy. Low-dose aspirin and calcium are recommended for prevention (Bodnar et al., Citation2007; Rumbold et al., Citation2008). Despite such recommendations, delay in identification and diagnosis of PE, the prevalence of preeclampsia occurs in 10% of pregnancies in African women, which is significantly higher than the global average of approximately 2% (Nakimuli et al., Citation2014).
According to Ethiopian Demographic Health Survey (2016), the estimate of the maternal mortality ratio is 412 deaths per 100,000 live births; that is, for every 1000 births, there are about 4 maternal deaths. The Ethiopian National Emergency Obstetric and Newborn Care (EMONC) showed that preeclampsia contributed for the complication of approximately 1% of all deliveries and 5% of all pregnancies. Moreover, 16% of direct maternal mortality was due to preeclampsia/eclampsia (Gaym et al., Citation2011). Ethiopia is one of the five countries that together account for 50% of the world’s maternal deaths and rural areas mostly contribute to maternal deaths (Gaym et al., Citation2011). A maternal mortality trend analysis showed an increasing trend of preeclampsia in Ethiopia.
Several studies have been conducted related to pregnant women to determine risk factors associated with survival time and longitudinal outcomes separately and epidemiological studies for example, (Garomssa & Dwivedi, Citation2008; Gaym et al., Citation2011; Jacobs et al., 2003; Mohammednur & Abdo, Citation2019; Mo et al., Citation2019; Musa et al., Citation2018; Tessema et al., Citation2015; Vataet al., 2015). However, the linear mixed model for longitudinal data and the Cox proportional hazards model for time-to-event data do not consider dependencies or interrelationships between these two different data types (longitudinal and time-to-event data) separately (L. Yang et al., Citation2016). Hence, appropriate statistical method (joint model) was proposed by several researchers in order to identify potential relationships between the longitudinal measures and disease risk (Faucett & Thomas, Citation1996; Rizopoulos, Citation2012; Wulfsohn & Tsiatis, Citation1997). Joint modeling of longitudinal and survival data is preferable than separate analysis as it incorporates all information simultaneously and provides valid and efficient inferences for the prediction of the time-to-onset of preeclampsia (L. Yang et al., Citation2016). Hence, the aim of this study focused on jointly modeling of hypertension measurements and time-to-onset of preeclampsia among pregnant women attending antenatal care service at Arerti Primary Hospital.
Ethiopia is implementing Maternal, Newborn, and Child Health (MNCH) programs as a priority health agenda to reduce maternal and child morbidity and mortality (Bergen et al., Citation2020). Identifying potential risk factors and the group of a population at risk is imperative to achieve MNCH programs (Skjærven et al., Citation2002). Therefore, the relevance of the finding from the analysis of longitudinal responses and survival time to onset of preeclampsia of pregnant women could be important for pregnant women, antenatal care service providers and future researchers in prevention and management of PE.
2. Methods and materials
2.1. Study area and population of interest
The study was conducted in Arerti Primary Hospital located in Arerti town in Minjar Shenkora Woreda in the North Shoa Zone at Amhara regional state of Ethiopia. The study population covered of all pregnant women attending antenatal care service at Arerti Primary Hospital between September 2018 and June 2019.
2.2. Study design
A retrospective longitudinal study design was conducted at the antenatal clinic of Arerti Primary
Hospital from September 2018 and June 2019. Both the longitudinal and survival data was extracted from the pregnant woman’s follow-up cards which contains socio-demographic characteristics, baseline clinical, laboratory measurement and other information of all pregnant women attending antenatal care service.
2.3. Study population
A total of 201 of pregnant women were included from women’s pregnant at antenatal care follow up at Arerti primary hospital. The Sample size (number of events) determination formula for studying the relationship between event time and the longitudinal process was calculated using the formula:
And the required total number of subjects (pregnant women) can be computed as:
(Chen et al., Citation2011). The parameters were estimated from pilot survey because no previous joint analyses are available to determine appropriate hypothesized values of association parameters. The assumptions include 95% Confidence level and 80% power. Thus the final sample size was 201. A systematic random sampling method was adopted for selecting a representative sample from the list of the medical charts that contain the list of pregnant women name and identification number. A total of 1220 pregnant women were at ANC follow-up during the study period. An interval (k) was calculated by dividing the total number of pregnant women at ANC follow-up in the hospital in the study period by the sample size (K = 1220/244 = 5). Every 5 pregnant women at ANC follow-up in the hospital during the study period was selected for assessment.
2.4. Operational definitions
Preeclampsia is defined as gestational hypertension [systolic blood pressure (SBP) ≥140 mmHg and/or diastolic blood pressure (DBP) ≥90 mmHg] after 20 weeks of gestation plus the presence of proteinuria. Proteinuria is assessed using urine dipstick method. Those women having a protein level of 1+ classified as having proteinuria (Belay & Wudad, Citation2019).
2.5. Data collection tools and procedures
The data was extracted by preparing check list or questionnaire from pregnant women follow up antenatal care service card which contains socio-demographic characteristics, baseline clinical, laboratory measurement including proteinuria and other information of all pregnant women attending antenatal care service at Arerti Primary Hospital from September 2018 and June 2019. Data regarding proteinuria and other clinical data were accessed from the women’s medical records. Proteinuria was assessed using urine dipstick method and was part of the routine investigation for all pregnant women. To check the validity and reliability of the data we have applied a cronbach alpha, so that the value was 0.80, the result indicated that the test to be internally more consistent. The data was collected by nurses working at Arerti Primary Hospital, who were well trained and experienced collected the data. Sampling procedure and data collection. A total of 1220 pregnant women were at ANC follow-up during the study period. All pregnant women were identified by a unique identifier, say unit number 1, unit number 2 … unit number N = 1220. Systematic sampling involves randomly selecting a population unit from the first k population units and selecting every kth population unit thereafter, such that the selected population units collectively form a systematic sample with sampling interval k, where the value of k is found by dividing the population size (N) by the sample size (n). Finally by preparing a check list or questionnaire we obtained the required information.
2.6. Response variable of the study
Three outcome variables were considered for this study; the longitudinal Systolic Blood Pressure (SBP), Diastolic Blood Pressure (DBP) in units of millimeters of mercury (mmHg) and survival outcome; time to the development of preeclampsia or delivery from ANC follow-up of pregnant women.
2.7. Data analysis methods
Separate and joint methods of longitudinal data analysis and survival data analysis were fitted to answer the research objectives. The analysis consists; exploratory data analysis, linear mixed effects model for the longitudinal data, Cox proportional hazards model for the time to event data and joint model for both longitudinal and time to event data.
3. Results
3.1. Descriptive statistics
The research data analysis was conducted using R software version 3.2.3. During the 10 months study period (September 2018 to June 2019), a total of 201 of pregnant women who attended antenatal care (ANC) are obtained in Arerti Primary Hospital. In the supporting file (Table 4.1) descriptive statistic of baseline covariate of those women was illustrated.
We observed 39 out of the 201 normotensive women included in the study developed PE and 162 of them were censored observations during the study period. The mean age and weight of the study pregnant women were 26.0 and 58.2, respectively. Out of the total sample, 23 (11.4%) had diabetes mellitus. Regarding the previous history of preeclampsia, 172 (85.6%) had no previous history of Preeclampsia. Out of the total pregnant women who examined, 177 (88.1%) of the women had no consecutive previous abortion.
Out of the total number of the current pregnancy, 172 (85.6%) were singleton pregnancy and 29 (14.4%) of them were twin; and out of singleton pregnant women 154 (95.1%) were censored, the remaining 18 (46.2%) were event occurred; and out of twin pregnant women 8 (4.9%) were censored, the remaining 21 (53.8%) were event occurred. Majority of the pregnant women, 175 (87.1%) of them were married and 26 (12.9%) were unmarried; and out of married women, 159 (98.1%) were censored, the remaining 16 (41.0%) women were event occurred; and out of unmarried women 3 (1.9%) were censored, the remaining 23 (59.0%) were event occurred.
Before fitting the uni-variate and bi-variable linear mixed-effects models, first we should checked the assumption of normality of the data. To check normality assumption of linear mixed effects model, normal Q-Q plots of the BP measurements with corresponding Shapiro-Wilk tests of normality were checked. In the supporting file Figure 4.1a and 4.1b shows the normal Q-Q plots of the SBP and DBP normality of the distributions of BP measurements. Similarly result from Shapiro Wilk test are not significant for SBP and DBP implying that the BP data set appear to follow a normal distribution (see supporting information file Table 4.2).
3.2. Summaries of longitudinal blood pressures (BP)
The bi-variable continuous longitudinal SBP and DBP measurements were measured at the 4 time points over a 10-month time period; first visit (4th–13th weeks gestation), second visit (14th—27th weeks gestation), third visit (28th–35th weeks gestation) and last visit (36th–40th weeks gestation).
A total of 201 pregnant women were observed at first visit and second visit. Then, 165(82.1%) pregnant women were observed at the third visit and 101(50.2%) pregnant women stayed at the last visit during follow-up periods. Mean with the corresponding standard deviation at each time points with respective sample sizes for both outcome variables were shown in . The numbers of participants are decreasing in successive time points. The average SBP and DBP were 116.84 and 66.99 at first visit respectively. There was a general increment in the mean value up to the last time point. When we look at the standard deviations, smaller variations were observed at first visit and higher variations were seen at second visit.
Table 1. Summary measures of the two longitudinal responses at each time points
3.3. Univariate linear mixed effect model result
The separate longitudinal analysis was started from the fixed effect modeling to select appropriate covariates that predicts SBP and DBP. To select the fixed effect components of the response variable SBP and DBP, including all covariates without considering the corresponding different random effects using a backward variable selection method. The predictor variables visit, age, weight, multiplicity, PE, gravidity, parity and diabetes were considered for linear mixed model for longitudinal BP. To identify the appropriate co-variance structure for the two longitudinal response variables; the three commonly used co-variance structures were considered. In the supporting file Table 4.4 shows that the First Order Auto-regressive (AR (1)) co-variance structure best fits for our data compared to the other co-variance structures.
In order to retain the random effects from the model, it is better to fit the linear mixed effects model with different random effects. In the supporting file () shows that random intercept and random slope model were used in linear mixed effect model that appropriately predicts the mean change of SBP and DBP over time.
Table 2. Parameter estimate of the univariate linear mixed effect models of SBP and DBP
shows the result of linear mixed effect model of SBP and DBP which found the covariates visit, age, weight, PE, multiplicity, parity and visit, age, weight, PE, multiplicity, parity, diabetes were significantly associated with mean change in the SBP and DBP at 5% level of significance respectability.
3.4. Bi-variable linear mixed-effects analysis of SBP and DBP
The parameter estimates of the final fitted bi-variable linear mixed model are presented in . The variables visit, age, weight, multiplicity and parity were found significant at 5% level of significance for the change in SBP and DBP. However, PE and diabetes were significantly associated with mean change in the DBP at 5% level of significance. Gravidity was the only variable did not significant in both responses. The intercept 65.7 and 117.79 with standard error of 4.81 and 5.87 represent estimates of the average level of DBP and SBP during the first follow up time respectively.
Table 3. Bi-variable mixed model fixed parameter estimates, standard error and P -Values for the final model
A parameter estimate for weight indicates a one-kg increase in weight was associated with a normal increase of 0.129 mmHg (Std. error = 0.044) in DBP and 0.144 mmHg (Std. error = 0.054) in SBP respectively. A parameter estimate of age indicates a one year increase in age was associated with a normal increase of 0.46 mmHg (Std. error = 0.114) in DBP and 0.57 mmHg (Std. error = 0.14) in SBP respectively. The average intercepts for multiplicity (twin) are 6.311 and 6.24, which indicates that on average pregnant women with twin pregnancy started with the higher DBP and SBP measure than singleton pregnancy at baseline.
3.5. Survival data analysis result
The pregnant women were followed up for the total of 40 weeks. The median survival time was found to be 31 weeks. At the end of the follow up, 39 (19.4%) women developed preeclampsia, the remaining 162 (80.6%) didn’t developed preeclampsia and loss to follow-up. Separate graphs of the estimates of the Kaplan-Meier survivor functions for the categorical covariates are presented in order to see the patterns of survival experience those different covariates. The survivor ship pattern of one is lying above another means the group defined by the upper curve has a better survival than the group defined by the lower curve (see the supporting file Figure 4.2).
The Log-rank test was performed to investigate the significance of the observed difference in the Kaplan-Meier estimates of the survivor functions among different categories of the factors. The result indicates that there were significant differences in survival probability of pregnant women in covariates of marital status, abortion, diabetes, PE, substance, multiplicity, gravidity and parity at 5% level of significance (see the supporting file Table 4.8).
3.6. Cox proportional hazard model
In Cox proportional regression model (see supporting file Table 4.9), the factors associated with a significant hazard of developing preeclampsia during the gestational follow-up time were age, weight, PE, multiplicity and marital status. Looking for the age of pregnant women, the risk of developing preeclampsia increased by 43.3% (HR = 1.43, Std. Error = 0.074, P-value <0.0001). The hazard of developing preeclampsia for those older age of pregnant women were 43.3 % higher risked than those are younger women. Controlling for other risk factors, the risk of developing preeclampsia, as estimated from a Cox model, was times higher per unit difference in weight. That is, holding the other covariates constant, a higher value of weight is associated with a poor survival. Regarding multiple pregnancy of women, twin pregnant had higher risk of developing preeclampsia compared to singleton pregnant (
). Women with PE had 1.07 times greater risk of developing PE compared to those with no prior history (
). The estimated risks of preeclampsia for unmarried pregnant women compared to those married women were 2.66 (
). This means the hazard rate of preeclampsia for unmarried pregnant women is 2.66 times more likely to develop preeclampsia than married pregnant women.
3.7. Results for univariate joint analysis
The joint model of the BP measurements and time to preeclampsia was analyzed by using R software, JM package. The two separate joint models showed that both SBP and DBP were significantly associated with preeclampsia risk. , revealed that most covariates found to be statistically significant on joint analysis and a strong association between the longitudinal bio-markers (BP). For longitudinal sub models, the covariates age, weight, multiplicity, parity and PE are found to be significantly associated the longitudinal change of BP measurements. A one unit increase in age was associated with 0.53 and 0.54 increased in the mean DBP and SBP, respectively. The mean changes in DBP and in SBP were 5.87 and 2.439 times higher for twin pregnant when compared to singleton. Comparing mean change in by PE, the mean change in DBP and in SBP were 6.751 and 5.01, respectively, times higher for women with PE compared to without PE.
Table 4. Results of univariate joint modeling of time to preeclampsia event and longitudinal BP
The survival sub models revealed that baseline maternal age, weight, PE and marital status were significant in both sub-models. But multiplicity was significant in survival sub models of SBP only. The association parameters () were significantly different from zero (
) indicating that there is strong association between both DBP and SBP and the risk for preeclampsia. The positive value of the association parameters (
= 0.046 and
= 0.085) indicated that true value of DBP and SBP were positively associated with the risk of preeclampsia, and with unit increase in the longitudinal DBP and SBP the risk of preeclampsia were increased by
and
respectively.
3.8. Results for Bi-variable joint analysis
In this section we fitted the bi-variable joint model of longitudinal and survival data to analyze the two longitudinal bio-markers (DBP and SBP) simultaneously through the linear mixed effect model and the cox model setup.
presents the estimation results of mean intercept, mean slope and the fixed effect in the longitudinal sub-model. According to this, we have observed a significant positive trend for DBP and SBP. The covariates visiting time, age, weight, PE and parity were appeared to be associated with the change in SBP and visiting time, age, weight, PE, parity and diabetes were significant factors for the change in DBP at 5% level of significance.
Table 5. Parameter estimates of bi-variable longitudinal sub model in joint analysis
The Parameter Estimates of Survival Sub-Model is presented in . The association parameter for SBP and
for DBP depicts the association between the two longitudinal responses and the event.
Table 6. Parameter estimates of survival sub-model in Bi-variable joint analysis
shows observed from those association parameters; a unit increase of DBP is associated with increases in women’s risk of developing PE assuming that the baseline covariates and SBP remain the same. But SBP was not significantly associated with hazard of PE at 5% level of significance (
) once DBP was adjusted in the model.
The fitted model also identified several other risk factors for PE, i.e. women’s age, weight; marital status and PE appeared to be risk of PE. This indicates that the hazard ratio of the baseline age of the women was means that as the age increases the hazard of PE increases.
Regarding baseline weight of women, a one unit increase in weight of a woman leads to increase the hazard of PE by . Looking at the marital status of the women, the hazard of those women who were unmarried were 47% higher than (
, p–value = 0.0041) those who were married.
A comparison between the separate cox model with uni-variable joint model and bi-variable joint model reveals some interesting features. Most importantly, we found that multiplicity of pregnancy is found to be significant in separate analysis of the survival outcome, but in bi-variable joint analysis and uni-varaite joint analysis (Longitudinal DBP and time to PE) was not significant.
4. Discussion
This study attempted to assess predictors that are associated with hypertension measurements and survival time/time to preeclampsia at Arerti Primary Hospital. So as to address mentioned objectives four different analyses were explored; the bi-variable linear mixed-effects model, Cox proportional hazards model, univariable joint modeling for each longitudinal outcome and event data and bi-variable joint model of longitudinal BP and time to preeclampsia with the sets of both continuous and categorical covariates. The longitudinal process was characterized by linear mixed (Laird & Ware, Citation1982) sub models, and the survival process was characterized by Cox Proportional (Cox, 1972) sub model. The Maximum likelihood via EM algorithm was used to estimate unknown parameters of the joint model (Rizopoulos, Citation2012; Wulfsohn & Tsiatis, Citation1997). In the separate analysis of the longitudinal data, the assumption of normality was checked using normal Q-Q plots with corresponding Shapiro-Wilk tests. Both plots and test of the data indicate that there are no a deviation from normality assumption. This data was analyzed using different plots (exploratory data analysis) followed by model based outputs. From individual profile plots, we observed the existence of variability in BP measurements within and between individuals but, the exploratory analysis result for the mean structure also suggested that on average, BP measurements increases in a linear pattern over time.
From the final model of LMM, we have found that visiting time, weight, parity, multiplicity of pregnancy, gestational diabetes and previous history of PE were found to be significant at 5% significance level similar to the study by (Rebeloet al, 2014). Our finding revealed that baseline age is an important socio-demographic predictor of BP, BP increases with increasing in baseline age; so age at baseline was a significant predictor for BP of pregnant women which is supported by study like (Mo et al., Citation2019). Results from this study demonstrate that the correlation between the two responses (DBP and SBP) estimated to be 0.96. The finding provides direct evidence that SBP and DBP measure were dropped at the beginning of pregnancy and increased throughout pregnancy and the result was supported by studies like (Mo et al., Citation2019).
Women with previous history of preeclampsia and baseline weight of gestation had a significantly higher risk and hazard of developing preeclampsia during the gestational follow up time (Musa et al., Citation2018). Our study also supports this findings in addition to other risk factors age of pregnant women, marital status and previous history of PE of the women. In this study, the association of maternal age and risk of preeclampsia was found to be significant (). This is congruent with the study conducted by (Belay & Wudad, Citation2019; Tessema et al., Citation2015). This study showed marital status had statistically significant association with the development of preeclampsia in both separate and joint model. The finding was in line with the study conducted in Dessie (Tessema et al., Citation2015).
On the other hand, we observed the significance of the association parameters () between two outcomes in uni-variate joint modeling and the estimated association parameters are
= 0.046 and
=0.085, p–value < 0.0001 indicating that the true value of DBP and SBP were positively associated with the risk of preeclampsia in a 40 weeks follow-up period and similar with the study conducted by Baschat et al. (Citation2018) who showed that average first-trimester DBP and second-trimester SBP measurements are independent risk factors for preeclampsia.
The results were able to show that the development of preeclampsia is positively related to the high blood-pressure values during the pregnancy. So women’s be going to monitor their blood pressures and be check at every visit during pregnancy which is supported by study (Mo et al., Citation2019). Health professionals shall counsel women about risk factors associated PE to prevent PE. The relevance of frequent and timely ANC visits shall be advocated to screen women who are at high risk to PE. Counseling women about healthy diet and appropriate body weight in every ANC visit shall be strengthened which is supported by study (Bookari et al., Citation2017).
5. Conclusion
In this study separate, joint analysis of a single longitudinal outcome and bi-variable longitudinal outcomes and time to event data were conducted to establish the relationship between longitudinal bio-marker measurements and the duration to preeclampsia. The finding of our study suggests that there are different risk factors for PE and the change of blood pressure measurements. Blood pressure measurements are influenced considerably by visit (follow-up time), age, baseline weight and multiplicity of pregnancy. The covariates age, weight, multiplicity of pregnancy, previous history of PE and marital status were found to be statistically significant effect for time to preeclampsia.
The pregnant women at ANC that their SBP and DBP measured repeatedly over time are associated with the risk for developing PE during their pregnancy, implying that women with higher baseline SBP and DBP have more likely to the event occurred; women with smaller baseline SBP and DBP have less likely to occur an event than women with higher SBP and DBP.
Generally, the estimated association parameters indicate longitudinal BP and the survival time were positively associated, implies higher values of the BP associated with a worse survival. Therefore, due to significance of association between the longitudinal and survival outcomes, joint model analysis were suggested over separate models analysis as they incorporate all information simultaneously and provide valid and efficient inferences.
5.1. Limitations of the study
Even though this study contributes as an input for the policy makers towards the decrement of the maternal morbidity and mortality, it has its own limitations. First, recall bias might be expected for the last menstrual period. Second, because of resource limitation, we only incorporated one hospital as study area. Finally, since the study was institutional based study, the result will not be generalizable to the general population of the catchment area.
Ethical and Consents
The research thesis has checked and approved by ethical clearance committee of University of Gondar, and the medical director’s office of Arerti Primary Hospital granted permission to use the patients’ data for this study. For the purpose of confidentiality, there were no linkages with individual patients and all data had no personal identifier and were kept confidential.
Availability of Data and Materials
Yes it is applicable.
Authors contributions
Dawit Baye Haile: Contributed in the conceptualization of the research problem, study design, write the thesis, analysis of the data, interpretation of the final result.
Aragaw Eshetie Aguade (PhD): Contributed in guidance, consultation, continued follow up, encouragement from the beginning to the end of the study.
Moges Zerihun Fetene: Contributed in guidance, consultation, continued follow up, encouragement from the beginning to the end of the study and formulate the manuscript.
We all authors of the paper carefully read, edited and finally approved the final manuscript.
List of abbreviations
ANC – Antenatal Care
AR – Auto-regressive
BP – Blood Pressure
DBP –Diastolic Blood Pressure
PE – Preeclampsia
SBP – Systolic Blood Pressure
Disclosure statement
No potential conflict of interest was reported by the author(s).
Additional information
Funding
Notes on contributors
Dawit Baye Haile
Dawit Baye completed his Master’s Degree in Biostatistics from University of Gondar, Ethiopia. He is currently engaged in teaching statistics course, research supervision on Dilla University College of Natural and Computational Science Department of Statistics. Aragaw Eshetie received the B.S.c. And M.Sc. Degree in Statistics from the Addis Ababa University, Ethiopia, and a Ph.D. degree in Statistics from the Andhra University, Indias. He has more than 15 years of experience in teaching, research and community engagement. Currently, he works as an assistant professor at the University of Gondar, College of natural and computational sciences, Department of Statistics, Gondar, Ethiopia.
Moges Zerihun Fetene
Moges Zerihun completed his Master’s Degree in Biostatistics from University of Gondar, he completed his first degree bachelor of statistics from Dilla University, Ethiopia. He is currently engaged in teaching statistics course, research supervision as well as community engagement on University of Gondar College of Natural and Computational Science Department of Statistics.
References
- American College of Obstetricians and Gynecologists. (2013). Hypertension in pregnancy. Report of the American College of Obstetricians and Gynecologists’ task force on hypertension in pregnancy. Obstetrics and Gynecology, 122(5), 1122–14. https://doi.org/10.1097/01.AOG.0000437382.03963.88
- Baschat, A. A., Dewberry, D., Seravalli, V., Miller, J. L., Block‐Abraham, D., & Blitzer, M. G. (2018). Maternal blood‐pressure trends throughout pregnancy and development of pre‐eclampsia in women receiving first‐trimester aspirin prophylaxis. Ultrasound in Obstetrics & Gynecology, 52(6), 728–733. https://doi.org/10.1002/uog.18992
- Belay, A. S., & Wudad, T. (2019). Prevalence and associated factors of pre-eclampsia among pregnant women attending anti-natal care at Mettu Karl referal hospital, Ethiopia: Cross-sectional study. Clinical Hypertension, 25(1), 1–8. https://doi.org/10.1186/s40885-019-0120-1
- Bergen, N., Zhu, G., Yedenekal, S. A., Mamo, A., AbebeGebretsadik, L., Morankar, S., & Labonté, R. (2020). Promoting equity in maternal, newborn and child health–how does gender factor in? Perceptions of public servants in the Ethiopian health sector. Global Health Action, 13(1), 1704530. https://doi.org/10.1080/16549716.2019.1704530
- Bodnar, L. M., Catov, J. M., Simhan, H. N., Holick, M. F., Powers, R. W., & Roberts, J. M. (2007). Maternal vitamin D deficiency increases the risk of preeclampsia. The Journal of Clinical Endocrinology & Metabolism, 92(9), 3517–3522. https://doi.org/10.1210/jc.2007-0718
- Bookari, K., Yeatman, H., & Williamson, M. (2017). Informing nutrition care in the antenatal period: Pregnant women’s experiences and need for support. BioMed Research International, 2017, 1–16. https://doi.org/10.1155/2017/4856527
- Chen, L. M., Ibrahim, J. G., & Chu, H. (2011). Sample size and power determination in joint modeling of longitudinal and survival data. Statistics in Medicine, 30(18), 2295–2309. https://doi.org/10.1002/sim.4263
- Choudhury, M., & Friedman, J. E. (2012). Epigenetics and microRNAs in preeclampsia. Clinical and Experimental Hypertension, 34(5), 334–341. https://doi.org/10.3109/10641963.2011.649931
- Cochran, W. G. (1972). Relative accuracy of systematic and stratified random samples for a certain class of populations. The Annals of Mathematical Statistics, 164–177.
- Faucett, C. L., & Thomas, D. C. (1996). Simultaneously modelling censored survival data and repeatedly measured covariates: A Gibbs sampling approach. Statistics in Medicine, 15(15), 1663–1685. https://doi.org/10.1002/(SICI)1097-0258(19960815)15:15<1663::AID-SIM294>3.0.CO;2-1
- Garomssa, H., & Dwivedi, A. D. (2008). Maternal mortality in Ambo Hospital: A five year retrospective review. Ethiopian Journal of Reproductive Health, 2(1).
- Gaym, A., Bailey, P., Pearson, L., Admasu, K., & Gebrehiwot, Y., & Ethiopian National EmONC Assessment Team. (2011). Disease burden due to pre‐eclampsia/eclampsia and the Ethiopian health system’s response. International Journal of Gynecology & Obstetrics, 115(1), 112–116. https://doi.org/10.1016/j.ijgo.2011.07.012
- Ghulmiyyah, L., & Sibai, B. (2012, February). Maternal mortality from preeclampsia/eclampsia. In Seminars in perinatology (Vols. 36, No. 1, pp. 56–59). WB Saunders.
- Laird, N. M., & Ware, J. H. (1982). Random-effects models for longitudinal data. Biometrics, 38(4), 963–974. https://doi.org/10.2307/2529876
- Mo, M., Shen, Y., Si, S., Xin, X., Shao, B., Wang, S., Muyiduli, X., Chen, Z., & Yu, Y. (2019). Feature of trajectory of blood pressure among pregnant women with gestational hypertension. Journal of Hypertension, 38(1), 127–132. https://doi.org/10.1097/HJH.0000000000002197
- Mohammednur, A., & Abdo, N. (2019, January– February). Modeling pregnancy induced hypertension among pregnant woman in Jimma University specialized hospital. IOSR Journal of Mathematics, 15(1 Ser. I), 22–28.
- Musa, J., Mohammed, C., Ocheke, A., Kahansim, M., Pam, V., & Daru, P. (2018). Incidence and risk factors for pre-eclampsia in Jos Nigeria. African Health Sciences, 18(3), 584–595. https://doi.org/10.4314/ahs.v18i3.16
- Nakimuli, A., Chazara, O., Byamugisha, J., Elliott, A. M., Kaleebu, P., Mirembe, F., & Moffett, A. (2014). Pregnancy, parturition and preeclampsia in women of African ancestry. American Journal of Obstetrics and Gynecology, 210(6), 510–520. https://doi.org/10.1016/j.ajog.2013.10.879
- Rizopoulos, D. (2012). Joint models for longitudinal and time-to-event data: With applications in R. CRC press.
- Rumbold, A., Duley, L., Crowther, C. A., & Haslam, R. R. (2008). Antioxidants for preventing pre‐eclampsia. Cochrane Database of Systematic Reviews, (1). https://doi.org/10.1002/14651858.CD004227.pub3
- Skjærven, R., Wilcox, A. J., & Lie, R. T. (2002). The interval between pregnancies and the risk of preeclampsia. New England Journal of Medicine, 346(1), 33–38. https://doi.org/10.1056/NEJMoa011379
- Tessema, G. A., Tekeste, A., & Ayele, T. A. (2015). Preeclampsia and associated factors among pregnant women attending antenatal care in Dessie referral hospital, Northeast Ethiopia: A hospital-based study. BMC Pregnancy and Childbirth, 15(1), 1–7. https://doi.org/10.1186/s12884-015-0502-7
- World Health Organization. (2011). WHO recommendations for prevention and treatment of pre-eclampsia and eclampsia: Evidence base (No. WHO/RHR/11.25).
- Wulfsohn, M. S., & Tsiatis, A. A. (1997). A joint model for survival and longitudinal data measured with error. Biometrics, 53(1), 330–339. https://doi.org/10.2307/2533118
- Yang, D., Zhu, L., Ran, B., Pu, Y., & Hui, P. (2016). Modeling and analysis of the lane-changing execution in longitudinal direction. IEEE Transactions on Intelligent Transportation Systems, 17(10), 2984–2992. https://doi.org/10.1109/TITS.2016.2542109
- Yang, L., Yu, M., & Gao, S. (2016). Joint models for multiple longitudinal processes and time-to-event outcome. Journal of Statistical Computation and Simulation, 86(18), 3682–3700. https://doi.org/10.1080/00949655.2016.1181760