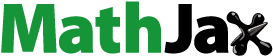
Abstract
The purpose of this paper is to investigate the impact of government consumption expenditure as a share of GDP on economic growth in developing countries. The paper uses threshold panel model to examine nonlinear relationship between the government consumption expenditure share and economic growth in 21 low-income countries and 11 low-middle income countries during 1981–2007. The results confirm nonlinear relationship, in which the threshold share of government consumption expenditure for the low and low-middle income countries is 16.2 and 16.9% with the confidence intervals of [13.7–17.3%] and [16.5–16.9%], respectively. The results indicate that, after passing the threshold, the effect of government consumption expenditure share on economic growth changes from insignificantly positive to significantly negative.
Public Interest Statement
Government size, which is defined as the ratio of government consumption expenditure to gross domestic product, has influences on economic growth. However, there are different studies that support both negative and positive impact. This paper investigated the nonlinear relationship between government size and economic growth in low and low-middle income countries. The findings indicate that the impact of government consumption expenditure share on economic growth is reversed around 16%. After passing the share, the government size has negative and significant effect on economic growth, while it has positive but not significant effect before this share. This result can be useful for analyzing fiscal policies and economic growth in developing countries.
1. Introduction
Government size, which is defined here as the ratio of government consumption expenditure to GDP, has negative and positive impacts on economic growth. On the one hand, enlargement of government results in a boost in economic growth rate through regulating protections of property rights, standardization, and providing infrastructures and public goods. On the other hand, it leads to deceleration of economic growth through disincentive effects of taxation and borrowing and increased inefficiencies. Thus, the final impact of government size on economic growth depends on the magnitude of negative and positive effects.
By the late 1970s and for the first time, Arthur Laffer introduced a curve similar to the inverted U-shaped curve for expressing the relationship between tax revenues and tax rate which was named as Laffer curve (Laffer, Citation2004). After that, Barro (Citation1990) reached such a curve regarding government size (the share of government consumption expenditure to total output) and economic growth using an endogenous growth model. The curve was known in the growth literature as “Barro curve.” In 1995, Richard Armey introduced a quadratic function; i.e. an inverted U-shaped curve for determining the optimal government size (the share of public expenditure to total output). The curve was named “Armey curve” consequently (Armey, Citation1995).
The purpose of this paper is to investigate the impact of government consumption expenditure share on economic growth, which is considered by Barro (Citation1990), instead of the public expenditure share by Armey (Citation1995). This paper has two main contributions. First, the nonlinear effect of the government size on economic growth in low and low-middle income countries is investigated, while past empirical studies focused on developed countries or investigated linear effect in developing countries. Second, the paper uses threshold panel approach (Hansen, Citation1996, Citation1999a) to identify the asymmetric relationship which is interesting to apply in financial studies.
The rest of the paper is organized as follows. In Section 2, theoretical and empirical studies of the impact of government size on economic growth are reviewed briefly. Section 3 describes threshold panel method. Section 4 presents empirical model and then Section 5 presents empirical findings. Finally, conclusion is presented in Section 6.
2. Review of theoretical and empirical studies
In the new growth theory, for the first time, Barro (Citation1990) entered government sector in a simple endogenous growth model with constant returns and infinite horizons. He assumed that government revenues from proportional tax are spent for public services, in a way that all producers are benefited equally and there is not any cumulative effect. Thus, government spending is entered as a production factor in the production function. In this framework, Barro (Citation1988) concluded that “The economy’s growth rate and saving rate initially rise with the ratio of productive government expenditures to GNP, g/y, but each rate eventually reaches a peak and subsequently declines.” The curve corresponding to this relationship is known as “Barro curve” in the growth literature and considered as a base for determining the optimal government expenditure.
Mourmouras and Lee (Citation1999) extended Barro’s work by combining Barro’s production function and consumer with finite horizons (Blanchard, Citation1985) and reached the Barro curve. Also, considering the Barro’s endogenous growth model in a two-country world, with the presumption of perfect capital mobility and finite horizons, Ghosh and Mourmouras (Citation2002) deduced that the effect of government expenditure share on economic growth and trade balance is similar to the Barro curve. After that, Kosempel (Citation2004) extended the Mourmouras and Lee’s model (Citation1999). He assumed a situation in which government spends its expenditures in two ways. First, government spends a portion of its revenues to provide free services to consumers (e.g. services provided via parks, museums, art galleries, and healthcare). These services are directly entered in consumer’s utility function. Second, government spends a portion of its revenues to provide free services to producers (e.g. services provided via constructing roads, airports, railroads, research and development institutes, and programs to improve the skills of labor force). Therefore, as in Barro (Citation1988) and Mourmouras and Lee (Citation1999), these expenditures are entered in the production function. Based on the results, Kosempel (Citation2004) indicate that Barro curve is approved for the second-type expenditures, but not for the first-type expenditures. Although increasing the share of first-type expenditures leads to increased utility of households, it always causes decline in economic growth.
In empirical studies, most of researches used linear methods. Ram (Citation1986) has carried out a comprehensive study using cross-sectional and time series data for 115 developing and developed countries. He concluded that the results of time series are consistent with cross-sectional estimations and the overall impact of government size on economic growth is positive almost in all cases. Guseh (Citation1997) examined the impact of government size on economic growth using data on 59 developing middle-income countries. He concluded that the impact of government size on economic growth is negative. Ghali (Citation1998), using data on 10 countries of the Organization for Economic Co-operation and Development (OECD) and vector error-correction model concluded that government size exerts influence directly or indirectly (via investment and trade) on economic growth. In this study, the ratio of government expenditure to GDP is used as a measure of government size. Gwartney, Lawson, and Holcombe (Citation1998), by investigating the United States, 23 OECD countries, and 60 less developed and high-income countries concluded that in all the cases, the effect of government size on economic growth is negative. They used ratio of government consumption expenditure to GDP as a measure of government size.
Fölster and Henrekson (Citation2001) considered two criteria for government size: the total taxes as a share of GDP and the government consumption expenditure as a share of GDP. By investigating two groups of countries (rich and non-OECD countries), they concluded that the negative effect of government size on economic growth is confirmed by both criteria in the non-OECD countries, while in the rich countries, it is only confirmed by the second criterion. Using data on 19 OECD countries, Dar and AmirKhalkhali (Citation2002) concluded that the effect of government size on economic growth is negative. They used ratio of government consumption expenditure to GDP as a measure of government size. Loizides and Vamvoukas (Citation2005) examined the relationship between government size and real per capita income (based on GNP) in Greece, UK, and Ireland. They concluded that the government size is the Granger cause of national income growth in short term (all three countries) and in long term (Ireland and UK). They used the ratio of government expenditure to GNP as a measure of government size.
Mitchell (Citation2005) studied the trend of government expenditure and economic growth in 2–3 past decades of the United States and 15 selected countries within European Union. He concluded that the extension of government expenditure would not necessarily tend to improve economic activities. For 15 European countries, Romero-Ávila and Strauch (Citation2008) showed that if the government size is measured by the share of total expenditure, consumption expenditure, government revenues, or direct taxes, its effect on economic growth would be negative; but, if the government size is measured by total public investment as a share of GDP, it would bring about positive effects. Wu, Tang, and Lin (Citation2010) investigated the causal relationship between government size and economic growth in 182 countries. Using panel Granger causality for eight sample countries (all countries, OECD countries, non-OECD countries, high-income countries, middle-income countries, low-income countries, high-corruption countries, and low-corruption countries), they concluded that there is a bi-directional causality between government size and economic growth (except in low-income countries). Ghose and Das (Citation2013) investigated the relationship between government size and economic growth in 19 emerging market economies. Using panel co-integration approach and considering different measures for government size and economic growth, they concluded that government size has significant and positive effect on economic growth.
Some researches, based on economic growth and other criteria, determined the optimal size of government. Vedder and Gallaway (Citation1998), using Armey curve, determined the optimal share of government expenditure. According to their results, the optimal government size for America, Canada, United Kingdom, Italy, Sweden, and Denmark are 17.45, 21.37, 20.97, 22.23, 19.43, and 26.14%, respectively. De Witte and Moesen (Citation2009) stated that the Armey curve does not consider causal relations and thus using this curve for determining the optimal government size would be far from reality. Using Data Envelopment Analysis (DEA), they estimated optimal government size as 40%.
Davies (Citation2009) used Human Development Index (HDI) instead of economic growth rate for determining the optimal government size. He considered two criteria for government size: government consumption expenditure as a share of GDP, and government investment expenditure as a share of GDP. Considering 154 countries for seven years (1975, 1980, 1985, 1990, 1995, 2000, and 2002), and categorizing the countries into two groups (all countries and low income countries), he reached the following results: first, the optimal share of consumption and investment in all countries are 17 and 13%, respectively. So the optimal government size in these countries is 30% which is more than the optimal government size with respect to the indicator of economic growth rate (for instance 17.5–26.5% of Vedder and Gallaway’s study). Second, in low-income countries, the share of government consumption expenditure always has positive effect on HDI, while the share of government investment expenditure may have up to 40% negative effect on HDI. Therefore, a certain size cannot be declared for these countries.
Chiou-Wei, Zhu, and Kuo (Citation2010) investigated the nonlinear impact of government size on economic growth in five Asian countries (South Korea, Singapore, Taiwan, Thailand, and Malaysia) drawing on the Solow growth model and using a dynamic smooth transition autoregressive (STAR) model. They used the ratio of government expenditure to GDP as a measure of government size. Chiou-Wei et al. (Citation2010) verified the nonlinear logistic STAR model for four countries including South Korea, Singapore, Taiwan, and Thailand and estimated the threshold share of government expenditure as 10.8, 11, 15.9, and 10.8%, respectively (of course, for Singapore the nonlinear relation was estimated as U-shaped curve).
As mentioned above, empirical studies applied various quantitative methods to investigate the impact of government consumption expenditure on economic growth. These methods are based on statistical models such as quadratic form, vector error correction, threshold autoregressive, and panel data or nonstatistical approaches such as DEA. Each method has particular strengths and weaknesses. In the quadratic form model, the optimal size cannot be estimated or tested directly, and the confidence interval cannot be constructed.
DEA is a mathematical programming model introduced by Charnes, Cooper, and Rhodes (Citation1978) to measure efficiency, and then it expanded in order to be used in various applications. Econometricians, due to the nonstochastic nature of DEA, argued that it produces biased estimates in the presence of measurement error and other statistical noise. In addition, DEA yields estimates that cannot be easily validated with usual diagnostic tools (Coelli, Rao, & Battese, Citation1998). However, Barnum, Gleason, and Hemily (Citation2008) used panel data analysis to estimate the confidence interval and statistical significance for DEA estimates, but this development is in its early stages.
In contrast, the panel data analysis incorporates cross section and time series data, and also it can be modeled as either random or fixed individual effects for countries. For this reason, panel data method is widely used in the growth literature. Using especially threshold panel approach, it is very interesting that nonlinear effect is examined in the growth regression and can be compared to the past empirical studies. This method is described in the next section.
3. Estimation method
According to the Barro curve or the Armey curve, the effect of government size on economic growth looks like an inverted V-shaped curve (it is not linear). Therefore, this curve should also be examined while investigating the effect of government size on economic growth. From the statistical point of view, one method would be to consider that the government size has a threshold exceeding it would invert its effect. Hansen (Citation1999a) has introduced estimation, testing, and construction of the threshold confidence intervals in the nondynamic panel with individual-specific effects.
3.1. Regression with one threshold
A regression function with one threshold and individual-specific fixed effects is considered as(1)
(1)
in which i represents individual, t represents time, y is the dependent variable, x is the column vector of explanatory variables none of which is supposed to be time invariant, and
. Here q is the threshold variable and
is the indicator function. The threshold variable can be an explanatory or any other variable; however, it is supposed that the threshold variable is not time invariant. Being there is a threshold, the observations can be divided into two regimes. To estimate Equation 1, fixed-effects transformations are used,
. Its matrix form is
in which
,
and
Coefficients are estimated using the LS method, and the sum of squared residuals is
(2)
(2)
Chan (Citation1993) recommended that a desirable estimation of the true threshold value can be reached by minimizing the sum of squared residuals, thus
(3)
(3)
After estimating the threshold, and
.
Now, the statistical significance of the threshold should be examined. Considering Equation 1, the null hypothesis is . In the case where this hypothesis is rejected, the threshold will be statistically significant. Based on the likelihood ratio test, the F1 statistic for testing the null hypothesis is
(4)
(4)
Here S0 is the sum of squared residuals of linear regression. The distribution of F1 is nonstandard and depends on the moments of the sample (Hansen, Citation1996). As a result, it is not possible to calculate its critical values in general form. Hansen (Citation1999a, Citation1999b) suggests using the below bootstrap procedure for examining the significance of F1: (1) by minimizing the sum of squared residuals, the threshold value and the corresponding coefficients are estimated; (2) a new sample, with the residuals of first stage, is generated under supposition of the null hypothesis (the explanatory variables are supposed to be nonstochastic, so they do not change). With this sample, the coefficients and residuals are estimated under the null and alternative hypotheses. Then, the simulated F1 statistic is calculated; and (3) the above calculations are repeated many times. Using the simulated F1, critical values of F and bootstrap p-value will be calculated. Finally, p-value is the percentage that the simulated F1 exceeds the actual value. In fact, this will be the estimation of asymptotic p-value under H0. Now, if this percentage is lower than the considered significance level (i.e. 5%), the null hypothesis will be rejected.
While there is a threshold, rejecting the null hypothesis H0 : β1 = β2, Hansen (Citation1997, Citation1999a) indicated that would be a consistent estimation of the true threshold
, but its asymptotic distribution will be nonstandard. He suggested using the likelihood ratio for constructing the confidence interval. The null hypothesis for the true threshold test will be
, so the likelihood ratio would be in the form below
(5)
(5)
It is clear that the probability of rejecting the null hypothesis increases with the value of this statistic. Hansen (Citation1999a) showed that under some assumptions, this statistic converges in distribution to the random variable which have the probability distribution of
and its reverse distribution is
. This function can be used to estimate the critical values. Provided that
, the confidence interval
will be made for the sum of squared residuals and consequently the threshold.
It should be noted that the hypothesis is different from the hypothesis
. The F1 statistic is used to test the presence of the threshold, while the LR1 statistic is used for constructing the confidence interval of the present threshold.
3.2. Regression with more than one threshold
If the first threshold is statistically significant, regression should be estimated using two thresholds and significance of the second threshold should also be tested. The regression function with two thresholds is defined as , in which
and
. Suppose that the smaller threshold is estimated in the previous step. Thus, the second (larger) threshold is estimated by minimizing the sum of squared residuals as
(6)
(6)
Bai (Citation1997) showed that estimating the second threshold is efficient, but estimating the first threshold is not. Therefore, it is necessary to repeat the above method for getting a better estimation of the first threshold. Bai’s correction will be . This is to ensure that both estimations
and
are efficient. After estimating the two thresholds, significance of the second threshold should be examined. The null hypothesis is
. And the F2 statistic for testing the null hypothesis is
(7)
(7)
in which . However, if the larger threshold is estimated in the first step, Equation 6 is rewritten as
(8)
(8)
Bia’s correction will be . Also, the null hypothesis will be
and the F2 statistic related to the null hypothesis is:
(9)
(9)
in which In practice, the both minimizations i.e. Equations 6 and 8 should be carried out and the smallest one should be chosen. Here F2 distribution is again nonstandard, therefore the Hansen’s bootstrap method should be used (Hansen, Citation1999a, Citation1999b). The three bootstrap steps described in Section 3.1 can also be performed for the F2 statistic. However, in this case, residuals of the double threshold model are used to generate new sample, and then the sample is extracted under the new null hypothesis,
or
. If the null hypothesis is not rejected, there will be only one threshold. But if the second threshold is confirmed, then the presence of the third threshold should be examined. Likewise, these rules can be extended for larger numbers of thresholds.
4. Empirical model and data
According to Solow (Citation1957), the aggregate production function is considered as Y = T·f(L, K) in which technological progress is called Hicks neutral or factor augmenting technological progress. Where Y is national economic output, T is the total factor productivity (TFP), K is capital stock, and L is labor force. Dar and AmirKhalkhali (Citation2002), Cameron, Proudman, and Redding (Citation2005), and Chiou-Wei et al. (Citation2010), assumed that the TFP of small economies depends on both the government size and international trade. This approach is based on the premise that the output growth rate is determined by the rates of factor accumulation as well as government size and the share of international trade and, whereby affecting TFP through their impacts on efficiency. Therefore, TFP is defined as T = g(GS, OPN), where GS represents government size and OPN represents openness. Now, the relationship between economic growth and government size can be examined using an econometric framework by following linear and nonlinear specifications;
(1) linear model (no threshold):(10)
(10)
(2) nonlinear model with one threshold:(11)
(11)
(3) nonlinear model with two thresholds:(12)
(12)
Variables are as follows: GGDP denotes the economic growth rate which is calculated based on gross domestic product (at constant 1990 prices in US dollar). GL denotes labor force growth rate. IY denotes the ratio of gross capital formation (at constant 1990 prices in US dollar) to gross domestic product (at constant 1990 prices in US dollar). Since the data related to physical capital of some countries was not available, this variable was used as a proxy of the physical capital growth rate. GS denotes measure of the government size, which is defined as the ratio of government consumption expenditure (at constant 1990 prices in US dollar) to gross domestic product (at 1990 constant prices in US dollar). OPN denotes degree of openness, which is the ratio of sum of exports and imports of goods and services (at current prices in US dollar) to gross domestic product (at current prices in US dollar). OGS denotes the optimal value of GS which is equal to γ in Equation 1. vit denotes error terms which includes individual-specific fixed effects (μi).
The main aim of this study is to investigate the effect of government size on economic growth in low and low-middle income countries, based on the World Bank (http://data.worldbank.org/). The method provided in the previous section is for balanced panel data and its results for unbalanced panel data are not known (Hansen, Citation1999a). Therefore, a large number of these countries were omitted and finally, 32 countries remained in the study. These countries are mainly from Africa and Asia, and their names are given in the Appendix (Table ). Since the effect of government size on economic growth may be different in low-income from low-middle income countries, three groups were considered: (1) low-income countries (LIC); (2) low-middle income countries (LMC); and (3) all countries of the first and second groups (full sample). A summary of information about each group can be found in Table .
Table 1. Information of different groups of the study
Table 2. Linear regression model
Table 3. Tests for thresholds
Table 4. The optimal government size, 95% confidence interval and single-threshold regression
Table 5. Mean government consumption expenditure as a share of GDP
5. Estimation results
Before estimating Equations 10–12, panel unit root tests are used to investigate whether the variables are stationary. In these tests, two types of approaches are considered, one with the assumption of common or homogeneous unit root process such as Levin, Lin, and Chu (Citation2002), and the other with the assumption of individual or heterogeneous unit root process such as Fisher-PP test, which proposed by Maddala and Wu (Citation1999) and Choi (Citation2001). Accordingly, Levin, Lin, and Chu and Fisher-ADF tests were employed here. The results show that the null hypothesis of unit roots is rejected for all of the variables (Table ).Footnote1
In addition, Hausman test, proposed by Hausman (Citation1978), was performed. The test compares fixed versus random effects. The null hypothesis is defined as no correlation between the individual effects and explained variables (the null hypothesis of orthogonality). The results show that the null hypothesis of orthogonality is rejected and hence it suggests that the fixed-effects specification is more appropriate than the random effects (Table ).
At first, the linear model was estimated by Equation 10 and the results are shown in Table . Based on the results, the government size has no significant effect on economic growth in the low-income countries and full sample. However, this effect is significant and negative in the low-middle income countries.
Then, based on Equations 11 and 12, the nonlinear effect was investigated. The presence of thresholds was tested by steps described in Sections 3.1 and 3.2, and the results are presented in Table . According to these results, the presence of a threshold is confirmed at the 1% significance level, but the presence of second threshold was not confirmed in any of them. For each threshold, 10,000 samplings and estimations of the simulated F statistic were repeated; i.e. 10,000 bootstrap replications.
For the estimations to be reliable, availability of enough observations before and after the threshold is necessary. So in each group, a range was determined for the government size and minimization was performed in this range. The government size was arranged from small to large in each group and then a fraction of the smallest and largest ones was omitted. However, these omitted observations were used for estimation, but the threshold variable was not selected in its range. These ranges were considered in a way that data availability before and after the threshold are enough while not belonging to a certain country. In this way, the estimations would be more reliable. These ranges are [9.5–23.2%] for the low-income countries, [9.9–20.5%] for the low-middle income countries, and [9.5–31.4%] for the full sample.
Finally, Equation 11 was estimated based on the optimal government size and the results are presented in Table . The optimal government size is 16.2% for the low income and 16.9% for the low-middle income countries, both of which are significant at the 1% level. The 95% confidence interval is [13.7–17.3%] for the first group and [16.5–16.9%] for the second group. For constructing confidence interval, likelihood ratio was estimated for different threshold values under the null hypothesis H0 : OGS = OGS0. Then based on the likelihood ratio (LR1) around the estimated threshold (that should not be higher than the critical value, c(.05) = 7.3523), the confidence interval of OGS is determined (for details, see c(α) in Section 3.1). The confidence intervals are shown in Table . These confidence intervals are not symmetric. Whenever the likelihood ratio declines more rapidly in one side of the threshold, the confidence interval in that side would be more extended. For example, in the first group, the confidence interval is more extended in the underside. Likewise, in the second group, the confidence interval reaches the threshold in its climax. In the full sample, the confidence interval is more extended in its upper side.
The coefficient of government size after the threshold and also the difference between the coefficients before and after the threshold are significant in all of the three groups, while they are not significant in the linear model in the two groups. In LIC and LMC, before reaching the optimal size (16.2% for LIC and 16.9% for LMC), the government size does not have a statistically significant effect on the growth, provided that the government size does not exceed the threshold. After passing the optimal size, this increase causes a 2% (3.7%) decline in economic growth. Based on these results, it is obvious that the effect of government size on economic growth is not independent on government size. In addition, except the intercepts and coefficients of government size, almost all the other coefficients are not different before and after the threshold, so the effect of these variables is not dependent on the government size.
After verifying the nonlinear relationship, the following policies for low and low-middle income countries become worthy of attention for increasing or decreasing government consumption expenditure. The mean of government consumption expenditure as a share of GDP, for three periods (20-year, 5-year, and 2-year), is presented in Table . In 20 countries, the government consumption expenditure share, at least for the last periods, is lower than the minimum optimal size. This share is higher than the maximum optimal size in 12 countries. But for some countries, the share is almost in the confidence interval of optimal level.
But in these interferences, government revenues are not considered. It is visible that government does not have a “magic source” of money and has to afford its spending through taxes and borrowing. Receiving taxes from economic agents make them discouraged and borrowing increases financial costs of investment, crowds out private investment and tax increase in future (Gwartney et al., Citation1998; Mitchell, Citation2005; Ram, Citation1986). Therefore, in the following proposed policies, only nonlinear effects of government consumption expenditure on the economic growth are considered:
Fixing the share of government consumption expenditure in countries whose government size is near its threshold size (the policy of raising government consumption expenditure proportionate to economic growth). This policy can assist to high and stable economic growth. Tunisia has experienced an average economic growth rate of 4.4% present with relatively stable share of government consumption expenditure (average of 15.9%, except for 1982 and 2007 with low percentage of 14.9).
Maldives has also experienced the same condition in two decades of 1980s and 1990s with higher economic growth. The share of government consumption expenditure has been near the threshold (averaging 17.3%) for the period of 1981–1998, bringing about a 10% economic growth rate for the country, while from 1999 to 2007, the share of government consumption expenditure exceeded the threshold value (averaging 25.6%) and has consequent decrease in economic growth up to 7.6%.
It is surprising that among the countries under study, only Tunisia (4.4 ± 2.3%) and Maldives (except for 1982 and 2005 due to the global economic recession, 10.3 ± 5.5%) and some other countries (Bangladesh: 4.9 ± 1.3%, Indonesia: 5.9 ± 2.2% except for 1998 due to the east Asian recession of 1997 and Uganda: 6.3 ± 1.9%, except for 1984 and 1985) experienced sustainable and high economic growth. With the threshold share of government consumption expenditure from 1999 to 2003, Mali experienced an economic growth rate of 5.2%, although from 2004 afterwards, with re-increase and excessive amount of the share of government consumption expenditure, economic growth falls to 4%.
Cuts in the share of government consumption expenditure if the government size is larger than its threshold size (the policy of reducing the government size). In this case, reducing the government size has positive outcomes, such as increasing efficiency, decreasing economic distortions, boosting private investments, curbing rent-seeking activities, and increasing economic growth. In three countries of Burkina Faso (from 1995), Chad (from 1995), and Djibouti (from 1997), a higher growth rate (6.5, 8 and 2.6% vs. 3, 5 and 1.2%) due to the policy of reducing government size (18, 32 and 29% vs. 20, 47 and 36%, respectively) can be seen than in years prior to the economic reform.
In Comoros, the share of government consumption expenditure decreased from 28.8% in 1981 to 12.2% in 2007. Then, up to 1999, in this country, economic growth increased with decrease in the share of government consumption expenditure (between 1997 and 1999, the government size was equal to that of the threshold value, with the consequent growth rate of 2.6%), but from 2000, with a decrease in the share of government consumption expenditure, the growth rate came down to 1.5%. Algeria experienced three periods: (1) 1981–1985, with the share of government consumption expenditure 19.5% and the growth rate 3.7%, (2) 1986–1991, with the share 17% and the growth rate 5.2%, and (3) 1992–2007, with re-increase in the share (roughly 21%) and the growth rate was 4%.
In 1980s and 1990s, Cote d’Ivoire experienced a growth rate of 3.3% as the result of a linearly decreasing the share of government consumption expenditure (from 30% in 1981 to 17% in 1998). But from 1999, with re-raising the share, economic growth rate came down to 0%. It is also seen that countries like Togo (from 1988), Iran (from 1989), Senegal (from 1994), and Niger (from 1995) succeeded in having higher economic growth by decreasing the share. Togo and Iran had an average growth rate of 2 and 5%, respectively, through reducing the government size to 10%. Senegal and Niger had higher growth rate through reducing the government size (4.5% vs. 2.5% for Senegal and 4% vs. .5% for Niger).
Increasing the share of government consumption expenditure when the government size is smaller than that of the threshold value (the policy of increasing the government size). A large government size is never favorable; however, neither small government is necessarily good. For example, while the share of government consumption expenditure was always under the threshold level for Bangladesh and Indonesia, Bangladesh reached higher economic growth rate by gradual increase in the share, and Indonesia experienced only lower economic growth by cutting this share. Government investment (especially in countries with the lack of fully developed markets of capital, insurance and information) can succeed in improving the performance of production factors, eliminating market failures and favoring the private sector by spillover effects of these factors.
From 1996, Mozambique and Sudan had favorable economic growth of 8 and 9.5%, respectively, (before that, their growth rate was 0 and 2%, respectively) through increasing the share of government consumption expenditure. Also, by increasing the share, Guyana and Sierra Leone in 2000 and 2001 (after the civil war of 1991–2001) get a growth rate of 1.5 and 1%, respectively (in 1980s and 1990s, the share of these two countries were 10 and 8%, and their economic growth, −2 and 0%, respectively).
However, it is still important that the impact of government size on economic growth is influenced by economic and political structures. From policy-makers point of view, mechanism of the impact of government expenditure on economic growth can be considered in the both short run and long run. In the short run, it is a usual phenomenon that policy-makers in developing countries target economic boom through Keynesian policies; i.e. countercyclical fiscal policy is effective against the business cycle. So the expansion of government size can resist recession and hence will actually induce the economy to economic growth. However, if the share of government consumption expenditure is higher than the threshold value, increase in this share cannot trigger economic growth and do not have a positive and favorable reaction (Chen & Lee, Citation2005; Loizides & Vamvoukas, Citation2005).
In the medium and long run, policy-makers in developing countries often interested in choosing the option of government intervention and larger government size strategies for rapid economic growth. But, in fact, this mechanism is under the influence of both the share of government consumption expenditure and the unique features of any country such as political system, efficiency of public sector, and the share of corruption and rent-seeking activities. For these reasons, the policy of increasing this share in developing countries should be implemented with caution, care, and selectiveness. Wide scale intervention of state, nondemocratic political system, inefficient public sector, high corruption, and rent-seeking activities are common features in developing countries. These attributes may lead to the failure of increasing the share in improving the economic condition and higher economic growth.
According to the statistics issued by Heritage Foundation (Citation2010), the level of corruption in majority of the countries under study (except for Sudan and Somalia, whose data are not accessible) is very high. It is surprising that among these countries, Tunisia enjoyed better conditions, attaining a sustainable and favorable growth rate of 4.4% through a relatively fixed threshold share of government consumption expenditure (15.9%). Wu et al. (Citation2010) stated that, in developing countries, government expenditure would not succeed in targeting development and eliminating poverty without improving quality of institutions and lowering the level of corruption. Although in recent years, the government size in most of the selected countries was lower than the threshold value; because of the reasons mentioned above, increasing the share of government consumption expenditure could not boost economic growth.
In addition, majority of the countries under study suffer nondemocratic political systems, and consequent negative effects of the government size. According to the data provided by Freedom House in 2010, among 32 countries, 11 have closed (not free) political system, 17 have partly free political system, and 4 have free political system. Guseh (Citation1997) showed that the impact of government size on economic growth is negative and these in nondemocratic and socialist societies can amount up to three times as much that in democratic societies with market economy. Also, 24 out of 32 countries under the study (75% of the sample) belonged to Africa. Gupta and Verhoeven (Citation2001) indicated that governments in African countries are more inefficient than governments in Asian countries in providing educational and health services (due to inefficient budgetary allocation of resources between sectors). Therefore, an increase in the share of government consumption expenditure will raise inefficiency. Then, improving efficiency in these countries must be done at the first.
Therefore, because of structural problems which are discussed above, it is visible that developing countries (in general) and the countries under study (in particular) must avoid any increase in the share of government consumption expenditure before adopting the following reforms: gradual move to market economy and the least intervention of the state in economic activities, setting democratic political system, privatization of public properties, improving efficiency in public sectors such as education and health, improving the quality of institutions to curb rent-seeking activities and corruption. According to the results by Ram (Citation1986), though, the favorable impact of the government size on economic growth is higher in poor countries, only after the above reforms can one be certain that increasing the government size and investment by government in infrastructures and market failures would bring about higher economic growth.
6. Conclusion
In this study, the effect of government size on economic growth was investigated in 21 low-income countries and 11 low-middle income countries during 1981–2007. Using threshold panel approach (Hansen, Citation1999a) and the Barro curve (Barro, Citation1990), the optimal government consumption expenditure as a share of GDP was estimated. The optimal share for the low-income and low-middle income countries was estimated to be 16.2% with the confidence interval [13.7–17.3%] and 16.9% with the confidence interval [16.5–16.9%], respectively. The estimated threshold government size in this study is smaller than the results of Davies (Citation2009) for high income countries and Herath (Citation2012) for Sri Lanka. Conversely, the results show that the threshold size is larger than the findings of Chen and Lee (Citation2005) and Chiou-Wei et al. (Citation2010) for South-east Asian countries. Therefore, the optimal size depends on the level of income in the selected countries. The results show that the effect of government consumption expenditure share, at least in the selected countries, is nonlinear. After passing the threshold share, the effect of economic growth is changed from insignificantly positive to significantly negative. Therefore, the results can explain why some empirical studies (such as Ghose & Das, Citation2013; Ram, Citation1986; Romero-Ávila & Strauch, Citation2008) estimated positive effect and some other studies (such as Dar & AmirKhalkhali, Citation2002; Fölster & Henrekson, Citation2001; Guseh, Citation1997; Gwartney et al., Citation1998; Romero-Ávila & Strauch, Citation2008) estimated negative effect.
Additional information
Funding
Notes on contributors
Mehdi Hajamini
Mehdi Hajamini is a PhD student of Economics at Ferdowsi University of Mashhad, Iran. His research interest is macroeconomics that focuses on employing nonlinear techniques for the analysis of nonlinear phenomena in macroeconomics.
Mohammad Ali Falahi
Mohammad Ali Falahi is a professor and teaches Econometrics and Macroeconomics in the Department of Economics, Ferdowsi University of Mashhad, Iran. His research interests are in the areas of Applied Econometrics with a special emphasis on time series and panel data models and Energy Economics.
Notes
1. Most panel unit root tests are designed to test the null hypothesis of a unit root for each individual series in a panel which corresponds to a fully nonstationary panel. As Pesaran (Citation2012) and Westerlund and Breitung (Citation2013) demonstrated, formulation of the alternative hypothesis depends on the assumption of homogeneity or heterogeneity of panel and hence there are two candidates for the alternative hypothesis. Under the assumption of homogeneity, in some tests, the parameters are common across cross-sections and so the alternative hypothesis can be described as “each of the series is stationary as a panel” that corresponds to a fully stationary panel (such as Levin et al., Citation2002). While other tests with the assumption of heterogeneity allow to vary freely across cross-sections and so the alternative hypothesis is “at least one of the series in the panel is generated by a stationary process” (such as Fisher-ADF).
References
- Armey, D. (1995). The freedom revolution: Why big government failed, why freedom works, and how we will rebuild America. Washington, DC: Regnery.
- Bai, J. (1997). Estimating multiple breaks one at a time. Econometric Theory, 13, 315–352.10.1017/S0266466600005831
- Barnum, D. T., Gleason, J. M., & Hemily, B. (2008). Using panel data analysis to estimate DEA confidence intervals adjusted for the environment. Journal of Transportation Engineering, 134, 215–223.10.1061/(ASCE)0733-947X(2008)134:5(215)
- Barro, R. J. (1988). Government spending in a simple model of endogenous growth. National Bureau of Economic Research Working Paper, No. 2588.10.3386/w2588
- Barro, R. J. (1990). Government spending in a simple model of endogenous growth. Journal of Political Economy, 98, 103–125.10.1086/jpe.1990.98.issue-S5
- Blanchard, O. J. (1985). Debt, deficits, and finite horizons. Journal of Political Economy, 93, 223–247.10.1086/jpe.1985.93.issue-2
- Cameron, G., Proudman, J., & Redding, S. (2005). Technological convergence, R&D, trade and productivity growth. European Economic Review, 49, 775–807.
- Chan, K. S. (1993). Consistency and limiting distribution of the least squares estimator of a threshold autoregressive model. The Annals of Statistics, 21, 520–533.10.1214/aos/1176349040
- Charnes, A., Cooper, W., & Rhodes, E. (1978). Measuring the efficiency of decision making units. European Journal of Operational Research, 2, 429–444.10.1016/0377-2217(78)90138-8
- Chen, S.-T., & Lee, C.-C. (2005). Government size and economic growth in Taiwan: A threshold regression approach. Journal of Policy Modeling, 27, 1051–1066.10.1016/j.jpolmod.2005.06.006
- Chiou-Wei, S.-Z., Zhu, Z., & Kuo, Y. H. (2010). Government size and economic growth: An application of the smooth transition regression model. Applied Economics Letters, 17, 1405–1415.10.1080/13504850902984295
- Choi, I. (2001). Unit root tests for panel data. Journal of International Money and Finance, 20, 249–272.10.1016/S0261-5606(00)00048-6
- Coelli, T., Rao, D. P., & Battese, G. (1998). An introduction to efficiency and productivity analysis. London: Kluwer.10.1007/978-1-4615-5493-6
- Dar, A. A., & AmirKhalkhali, S. (2002). Government size, factor accumulation, and economic growth: Evidence from OECD countries. Journal of Policy Modeling, 24, 679–692.10.1016/S0161-8938(02)00163-1
- Davies, A. (2009). Human development and the optimal size of government. The Journal of Socio-Economics, 38, 326–330.10.1016/j.socec.2008.07.015
- De Witte, K., & Moesen, W. (2009). Sizing the government. Munich Personal RePEc Archive No. 14785.
- Fölster, S., & Henrekson, M. (2001). Growth effects of government expenditure and taxation in rich countries. European Economic Review, 45, 1501–1520.10.1016/S0014-2921(00)00083-0
- Ghali, K. H. (1998). Government size and economic growth: Evidence from a multivariate cointegration analysis. Applied Economics, 31, 975–987.
- Ghose, A., & Das, S. (2013). Government size and economic growth in emerging market economies: A panel co-integration approach. Macroeconomics and Finance in Emerging Market Economies, 6, 14–38.10.1080/17520843.2012.697075
- Ghosh, S., & Mourmouras, I. A. (2002). On public investment, long-run growth, and the real exchange rate. Oxford Economic Papers, 54, 72–90.10.1093/oep/54.1.72
- Gupta, S., & Verhoeven, M. (2001). The efficiency of government expenditure experiences from Africa. Journal of Policy Modeling, 23, 433–467. Retrieved from http://www.sciencedirect.com/science/article/pii/S0161893800000363
- Guseh, J. S. (1997). Government size and economic growth in developing countries: A political-economy framework. Journal of Macroeconomics, 19, 175–192.10.1016/S0164-0704(97)00010-4
- Gwartney, J. D., Lawson, R. A., & Holcombe, R. G. (1998). The size and functions of government and economic growth. Washington, DC: Joint Economic Committee.
- Hansen, B. E. (1996). Inference when a nuisance parameter is not identified under the null hypothesis. Econometrica, 64, 413–430.10.2307/2171789
- Hansen, B. E. (1997). Inference in TAR models. Studies in Nonlinear Dynamics and Econometrics, 2, 1–14.
- Hansen, B. E. (1999a). Threshold effects in non-dynamic panels: Estimation, testing, and inference. Journal of Econometrics, 93, 345–368.10.1016/S0304-4076(99)00025-1
- Hansen, B. E. (1999b). Testing for linearity. Journal of Economic Surveys, 13, 551–576.10.1111/1467-6419.00098
- Hausman, J. A. (1978). Specification tests in econometrics. Econometrica, 46, 1251–1271.10.2307/1913827
- Herath, S. (2012). Size of government and economic growth: A nonlinear analysis. Economic Annals, LVII, 7–30.
- Heritage Foundation, Index of Economic Freedom. (2010). Heritage foundation economic freedom dataset. Retrieved from http://www.heritage.org/index/
- Kosempel, S. (2004). Finite lifetimes and government spending in an endogenous growth model. Journal of Economics and Business, 56, 197–210.10.1016/j.jeconbus.2003.08.002
- Laffer, A. (2004). The Laffer curve: Past, present, and future. Heritage Foundation Backgrounder, No. 1765.
- Levin, A., Lin, C., & Chu, C. (2002). Unit root tests in panel data: Asymptotic and finite sample properties. Journal of Econometrics, 108, 1–24.10.1016/S0304-4076(01)00098-7
- Loizides, J., & Vamvoukas, G. (2005). Government expenditure and economic growth: Evidence from trivariate causality testing. Journal of Applied Economics, 8, 125–152.
- Maddala, G. S., & Wu, S. (1999). A comparative study of unit root tests with panel data and a new simple test. Oxford Bulletin of Economics and Statistics, 61, 631–652.10.1111/obes.1999.61.issue-S1
- Mitchell, D. J. (2005). The impact of government spending on economic growth. Heritage Foundation Backgrounder, No. 1831.
- Mourmouras, I. A., & Lee, J. E. (1999). Government spending on infrastructure in an endogenous growth model with finite horizons. Journal of Economics and Business, 51, 395–407.10.1016/S0148-6195(99)00014-4
- Pesaran, M. H. (2012). On the interpretation of panel unit root tests. Economics Letters, 116, 545–546.10.1016/j.econlet.2012.04.049
- Ram, R. (1986). Government size and economic growth: A new framework and some evidence from cross-section and time-series data. American Economic Review, 76, 191–203.
- Romero-Ávila, D., & Strauch, R. (2008). Public finances and long-term growth in Europe: Evidence from a panel data analysis. European Journal of Political Economy, 24, 172–191.10.1016/j.ejpoleco.2007.06.008
- Solow, R. M. (1957). Technical change and the aggregate production function. The Review of Economics and Statistics, 39, 312–320.10.2307/1926047
- Vedder, R. K., & Gallaway, L. E. (1998). Government size and economic growth. Washington, DC: Joint Economic Committee.
- Westerlund, J., & Breitung, J. (2013). Lessons from a decade of IPS and LLC. Econometric Reviews, 32, 547–591.10.1080/07474938.2013.741023
- Wu, S.-Y., Tang, J.-H., & Lin, E. S. (2010). The impact of government expenditure on economic growth: How sensitive to the level of development? Journal of Policy Modeling, 32, 804–817.10.1016/j.jpolmod.2010.05.011