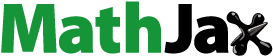
Abstract
This article analyses the technical efficiency of maize production in Zimbabwe’s smallholder farming communities following the fast-track land reform of the year 2000 with a view of highlighting key entry points for policy. Using a randomly selected sample of 522 smallholder maize producers, a stochastic frontier production model was applied, using a linearised Cobb–Douglas production function to determine the production elasticity coefficients of inputs, technical efficiency and the determinants of efficiency. The study finds that maize output responds positively to increases in inorganic fertilisers, seed quantity, the use of labour and the area planted. The technical efficiency analysis suggests that about 90% of farmers in the sample are between 60 and 75% efficient, with an average efficiency in the sample of 65%. The significant determinants of technical efficiency were the gender of the household head, household size, frequency of extension visits, farm size and the farming region. The results imply that the average efficiency of maize production could be improved by 35% through better use of existing resources and technology. The results highlight the need for government and private sector assistance in improving efficiency by promoting access to productive resources and ensuring better and more reliable agricultural extension services.
Public Interest Statement
This article concerns technical efficiency of crop production as an important aspect of pursuing output growth in agriculture in developing countries. Following the fast-track land reform of 2000, Zimbabwe’s smallholder farming communities have grown significantly and now produce the bulk of the country’s maize. However, the sector’s productivity remains low, with yield per hectare averaging only about 0.7 tonnes, seemingly because of technical inefficiencies. This paper contributes to the current debate in finding ways of raising maize productivity in smallholder farming by highlighting key entry points for policy. Findings show that maize output responds positively to increases in inorganic fertilisers, seed quantity, the use of labour and the area planted. This implies that the average efficiency of maize production could be improved through government and private sector assistance in promoting access to productive resources and ensuring better and more reliable agricultural extension services.
1. Introduction and background
Maize production is an important component of food security and livelihoods among smallholder farming communities in Zimbabwe. Being the staple food of the overwhelming majority of people in the country, maize is the most important commodity in terms of food security. The majority of smallholder farmers grow maize primarily for subsistence purposes (Mazvimavi, Ndlovu, An, & Murendo, Citation2012). Since the implementation of the fast-track land reform in 2000, there have been radical changes in the structure of the agricultural sector. An estimated 70% of the population now lives in small-scale farming areas (Mano, Citation2006). This has significant implications for food security, given the critical role of the smallholder sector in producing the staple maize crop. Crop failures and inefficiencies in smallholder maize production might have serious consequences for food security in the country.
Low agricultural productivity and production inefficiency in the smallholder farming community since the land reform have aggravated food insecurity by widening the gap between the demand and supply of food. Per capita maize production is slowly declining because of a significant decline in yield per hectare over time. In the 1990s, the smallholder maize yield was around 1.5 tonnes/ha but after 2000 it dropped to about 0.7 tonnes/ha (Government of Zimbabwe, Citation2002).
Food security could be improved by increasing the production efficiency of smallholder farmers and, hence, the productivity of the staple crop. While it is clear that production inefficiencies are limiting agricultural productivity, the sources of such inefficiencies are diverse. A key requirement of improving productivity (and food security) is for smallholder farmers to use production inputs more efficiently. Understanding the production elasticity of inputs, efficiency, and any socio-economic characteristics of the farmers that influence such efficiency would help improve the design of agricultural policies and programmes, which could in turn help increase food production (Msuya, Hisano, & Nariv, Citation2008). This study therefore aims to assess the technical efficiency of smallholder farmers, the determinants of their technical efficiency, and the production elasticity of inputs in smallholder maize production in Zimbabwe. This could provide guidelines for the design of policies to promote maize production and efficiency in smallholder farming. The aim is to help solve the country’s food security problems by improving the efficiency with which farmers use their existing set of inputs and technologies. The ultimate goal is increasing smallholder maize output, improving household food security, raising farmers’ incomes, and reducing poverty in the country.
This paper uses recent survey data from four districts of Zimbabwe to analyse production elasticity and technical efficiency in smallholder maize production. Based on farm-level data from the 2011 production season, the paper employs a stochastic frontier analysis of production functions to estimate input elasticity, the level of technical efficiency of smallholder maize producers, and farmer characteristics that influence such efficiency. The study contributes to the current debate in finding ways of raising maize productivity in smallholder farming by highlighting key entry points for policy. It is also essential to identify the sources of production efficiency in order to design private public policies.
The rest of the paper is set out as follows: Section 2 provides an overview of the literature on technical efficiency and use of the stochastic frontier methodology, while Section 3 reviews studies on the technical efficiency of farmers in developing countries. Section 4 describes the study sites, data collection and sampling, and Section 5 outlines the econometric method used and the estimation approach. Section 6 discusses the results, and the paper concludes with findings and policy implications in Section 7.
2. Technical efficiency in crop production
Technical efficiency in crop production can be defined as a farmer’s ability to maximise outputs given a set of inputs and technology. The degree of technical inefficiency reflects an individual farmer’s failure to attain the highest possible output level given the set of inputs and technology used. The highest possible output, using the available inputs and technology, is represented by the production frontier. Figure shows a production frontier where the degree of technical efficiency is conditional on the level of inputs used (adapted from Battese, Citation1992). Output (Y) is shown on the y-axis and inputs (X) on the horizontal axis.
Figure 1. Technical efficiency of firms in input-output space.
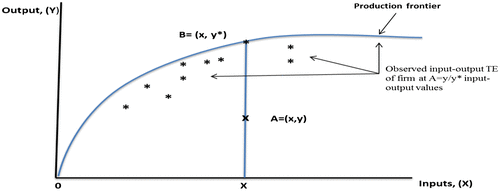
The distinction between technological change and technical efficiency is important. Technological change reflects a shift of the production frontier, as new technologies enable output per unit of input to increase (Bravo-Ureta, Citation2007). Technical efficiency, on the other hand, explains the difference between potential and observed yield for a given level of technology and inputs.
Initial studies to measure technical efficiency for a cross section of producers used the deterministic frontier approach, which assumes that any deviations from the frontier are due to inefficiency. In the maize example, this would imply any farmer producing below the frontier was inefficient. However, the deterministic frontier ignored factors beyond the control of the farmers, such as weather conditions, which could influence efficiency. Thus the results of the deterministic approach were sensitive to the selection of variables and to data errors (Bravo-Ureta, Citation2007). Aigner, Lovell, and Schmidt (Citation1977) and Meeusen and van Den Broeck (Citation1977) independently developed the stochastic frontier approach to address some of the limitations of the deterministic frontier approach. In the new approach, the error term consists of two components, one being random and the other being a one-sided residual term representing inefficiency. The stochastic frontier approach has subsequently been refined in various ways.
This study adopts the stochastic frontier approach to assess the technical efficiency of smallholder maize producers in Zimbabwe. Since the 2000 fast-track land reform, only a few studies of this nature have been conducted in the country; this paper is therefore an important addition to the literature.
3. Empirical studies on technical efficiency in developing countries
Globally, there is a growing body of empirical research on the economic efficiency of farmers in both developed and developing countries (for appraisals see Amaza & Olayemi, Citation2002; Battese, Citation1992; Coelli, Citation1995; Narala & Zala, Citation2010). The empirical literature on the efficiency of farmers focuses on developed countries and Asian economies, and a much smaller number relates to agriculture in developing countries, especially in Africa.
Among these, Heshmati and Mulugeta (Citation1996) estimate the technical efficiency of Ugandan matoke (plantain banana) farms. Using a Cobb–Douglas production function they find decreasing returns to scale and a mean technical efficiency of 65%. There was no significant variation in technical efficiency with respect to farm sizes, and they do not identify the various sources of technical efficiency among the farmers.
Seyoum, Battese, and Fleming (Citation1998) consider the technical efficiency and productivity of maize producers in Ethiopia and compare the performance of farmers within and outside a programme of demonstrating technology. Using Cobb–Douglas stochastic production functions, they show that farmers who participated in the programme were more technically efficient, with a mean technical efficiency of 94%, as against 79% for those outside the project.
The impact of education externalities on production and the technical efficiency of farmers in rural Ethiopia is the subject of Weir and Knight (Citation2000). One limitation of their study, and that of Weir (Citation1999), is that they only assess the level of schooling as a source of technical efficiency. Using standard Cobb–Douglas production function, they find evidence that the source of the externalities of schooling is the adoption and spread of innovations that shift out the production frontier. The mean technical efficiency of cereal crop farmers is estimated at 55%, and a unit increase in the years of schooling increases this by 2.1% points.
Sherlund, Barrett, and Adesina (Citation2002) investigate the efficiency of smallholder rice farmers in Côte d’Ivoire using both parametric and non-parametric methods, while controlling for environmental factors that affect the production process. In addition to identifying factors that influence technical efficiencies, the study finds that the inclusion of environmental variables e.g. rainfall variability in the production function significantly changes the results: the estimated mean technical efficiencies increase from 36 to 76%.
Binam, Tonyè, Wandji, Nyambi, and Akoa (Citation2004) examine factors influencing the technical efficiency of groundnut and maize farmers in Cameroon. Using a Cobb–Douglas production function, they found mean technical efficiencies of around 73 and 77%. They conclude that access to credit, social capital, and distance from the road, and extension services are important factors influencing technical efficiencies.
Mochebelele and Winter-Nelson (Citation2000) evaluated the impact of labour migration on the technical efficiency of farms in the rural economy of Lesotho. Using the stochastic production function (translog and Cobb–Douglas), they found that households that send migrant labour to South African mines are more efficient than those that do not, with mean inefficiencies of 36 and 24%, respectively. In addition, there is no statistical evidence that the size of the farm or the gender of the household head affects the efficiency of farmers. They concluded that remittances facilitate agricultural production, rather than substitute for it. Their study does not, however, consider other household characteristics that might affect technical efficiency (e.g. education, experience, access to credit or capital, and extension services) and the extent to which households that export labour receive remittances. Their interpretation that remittances explain differences in technical efficiencies is based on the presumption that migrant labourers remit to their exporting households, and not on a measure of the extent of such remittances.
Only a few studies focused on the technical efficiency of farmers in Zimbabwe. Mazvimavi et al. (Citation2012) studied the productivity and efficiency of maize production under conservation agriculture, comparing productivity and efficiency levels between conservation agriculture and conventional farming. Using the Cobb–Douglas and trans-log production functions, the study found that output elasticities showed positive responses to the use of labour and seed in conservation agriculture, and negative responses in conventional farming. The responses to land and draught in conservation agriculture were negative, while fertiliser had a larger positive response in conservation agriculture than in conventional farming. Joint frontier estimates indicate that farmers produced 39% more in conservation agriculture than in conventional farming. Technical efficiency levels were similar (about 68%) for both technologies, with two-thirds of farmers achieving efficiency scores in the 60–80% range. These results show sizeable yield gains in conservation agriculture and significant contributions to food production.
Owens, Hoddinott, and Kinsey (Citation2001) explore the impact of agricultural extension on farm production in Zimbabwe, Using panel data and Ordinary least squares regression the research found that, access to agricultural extension services raised the value of crop production by 15%.
Studies on technical efficiency are particularly important to African agriculture, as they provide vital information for improving the design of agricultural policies. More empirical work is needed in Zimbabwe to assess farmers’ performance and the constraints on efficiency, especially following the change in the agrarian structure since 2000.
4. Research sites, sampling and data collection
4.1. Research study sites
The data for the paper is drawn from surveys in smallholder farming communities in Zimbabwe, which covered two provinces and four districts: Mashonaland Central Province (Guruve district) and Mashonaland East Province (Goromonzi, Mudzi and Hwedza districts). Two of the districts, Goromonzi and Guruve, fall in natural farming region II, while Hwedza and Mudzi are in regions III and IV respectively. Table shows the survey areas, their agro-ecological characteristics, rainfall, temperature and main crops.
Table 1. Research districts and natural conditions
4.2. Sampling and data collection
The cross-sectional household baseline study was conducted between November and December 2011. The sampling frame was drawn from farmer households in the four districts, which were selected on the basis of agro-ecological potential and market access. Goromonzi and Guruve are in high-potential agro-ecological zones, while Mudzi and Hwedza are in marginal and low-potential zones. The data collection involved a household survey, using a structured questionnaire. The survey collected information on household composition and characteristics, crop production, household market participation, access to infrastructure, household incomes, ownership of land and non-land assets, livestock ownership, and access to agricultural inputs, extension services and market information. Random sampling was used to select the wards in the four districts and the households to be interviewed from lists provided by resident agricultural extension officers. The research was based on a sample of 522 smallholder maize producers randomly picked from the selected wards.
5. Econometric method
This paper follows the method of estimating a stochastic frontier production function proposed by Aigner et al. (Citation1977) and Meeusen and van Den Broeck (Citation1977). Kumbhakar, Ghosh, and McGuckin (Citation1991) extended the stochastic frontier methodology by openly introducing the determinants of technical efficiency into the model. One advantage of the approach is that it accounts for measurement error in the specification and estimation of the frontier production function. As noted, the stochastic frontier production function differs from the traditional production function in that it consists of two error terms. The first error term accounts for technical efficiency and the second for factors such as measurement error in the output variable, the weather, and the combined effects of unobserved inputs.
The model chosen in this paper has gained preference in application in developing nation’s agriculture. The approach has gained popularity as opposed to the data envelopment analysis. Reasons for this include, first, that the assumption that all deviations from the frontier arise from inefficiency (as assumed by the data envelopment analysis approach) is difficult to accept, given the inherent variability of agricultural production because of uncontrollable factors such as weather, pests and diseases. Second, because many farms are small, family-owned enterprises, farm records are seldom kept. Thus the available data on production are likely to contain measurement errors. In this study, farmers were asked to recall maize production information some months after harvesting, which could have introduced measurement errors.
This paper uses the Cobb–Douglas form of the stochastic frontier production model with a log–log functional form following its merit in application in smallholder farming (see Binam et al., Citation2004; Mazvimavi et al., Citation2012; Mochebelele & Winter-Nelson, Citation2000; Seyoum et al., Citation1998). The production data is maize production in the 2011 season by each of the 522 randomly selected households.
The production function is specified as:(1)
(1)
where yield is the maize output per hectare (kg/ha); β0–β4 are the parameters to be estimated; Inorganicfert is the amount of fertiliser applied per hectare (kg/ha); Seedquant is the maize seed rate per hectare (kg/ha); Labour constitutes family labour plus hired labour (person-days); Areaplanted is the area put under maize for the 2010/2011 production season (ha); εi is the error term, equal to (Vi − Ui); Vi is a two-sided random error component beyond the control of the farmer; Ui is a one-sided inefficiency component.
The farm-specific technical efficiency (TEi) of the ith farmer was estimated using the expectation of Ui conditional on the random variable εi. It follows that:(2)
(2)
so that 0 ≤ TEi ≤ 1.
After obtaining the technical efficiency estimates for each smallholder maize producer, using Equation (2), a kernel density graph was produced of the distribution of technical efficiency in the sample.
Technical inefficiency determinants are specified as:(3)
(3)
where Ui is technical inefficiency; δ0, … δ1 are the parameters to be estimated; is a vector of farmer and household socio-economic characteristics; Wi is a random error.
The stochastic production frontier, defined by Equation (1), and the technical inefficiency model, defined by Equation (3), were jointly estimated by the maximum likelihood method, using Stata, version 12. A half-normal distribution of the inefficiency variance was used in the estimation.
5.1. Presumed relationships between socio-economic characteristics and technical efficiency
A range of factors, including plot-level characteristics and socio-economic, demographic, environmental and non-physical factors, are likely to affect the efficiency of smallholder maize farmers. A number of studies (Amaza & Olayemi, Citation2002; Coelli & Battese, Citation1996; Llewelyn & Williams, Citation1996; Narala & Zala, Citation2010; Parikh & Shah, Citation1994) have investigated the determinants of technical efficiency among producers. They regressed the predicted efficiencies obtained from an estimated stochastic frontier on a vector of farmer-specific factors (e.g. the age of the farmer, the farmer’s level of education and access to extension services) in a second-stage regression. Several of these studies show a positive relationship between technical efficiency and socio-economic characteristics (Coelli & Battese, Citation1996; Kalirajan, Citation1991; Narala & Zala, Citation2010; Parikh, Ali, & Shah, Citation1995).
The identification of the factors that influence the level of technical efficiency is a particularly valuable exercise for policy formulation. The list of variables selected as potential determinants of technical efficiency include both dummy and continuous variables. Dummy variables include the gender of the household head, access to credit, cattle ownership, the region, the level of education of the household head, and the treatment (i.e. whether conservation or conventional agriculture was practised). Continuous socio-economic variables include household size, the frequency of extension services per production season, the frequency of weeding, the age of the household head, farming experience and the size of the farm.
It was expected that the frequency of extension services, access to financial credit, male household headship, weeding frequency, cattle ownership, the level of education of the household head, farming experience and farm size would positively influence technical efficiency, as several studies have found (Coelli & Battese, Citation1996; Kalirajan, Citation1991; Mazvimavi et al., Citation2012; Narala & Zala, Citation2010). Farmers with frequent access to extension services were expected to be more productive and efficient, since they could obtain advice on current technologies, better maize germplasm, and other relevant information on production. Access to credit was seen as another driver of efficiency among smallholder farmers, enabling them to purchase certified seed, fertilisers and other inputs to improve efficiency. Male-headed households were expected to be relatively more productive and therefore more efficient. Considering the importance of weed management in maize production, a positive relationship between weeding frequency and technical efficiency was expected. Cattle ownership, the level of education, farming experience and farm size were also expected to drive technical efficiency, on the understanding that access to productive resources would encourage efficient production.
Household size, the region and the treatment were expected to have an ambiguous influence. A larger household could be a source of labour, which could improve efficiency. But household size could also mean high dependency on the household head, which could contribute to poverty. Poorer households are likely to be inefficient, as their economic status prevents them from acquiring the necessary inputs. Age is expected to be negatively associated with technical efficiency, following Goldman (Citation2013), who finds that older farmers tend to be more technically inefficient than younger ones.
Table gives a summary of variables used to explain variation in technical efficiency and their expected influence.
Table 2. Summary of the variables and their expected influence on technical efficiency
6. Estimation results and discussion
6.1. Summary statistics
Summary statistics of production data and the socio-economic characteristics of the sample are presented in Table . Mean maize output by the smallholder producers is 1,222.24 kg (1.2 tonnes). On average, smallholder maize farmers applied 158.57 kg of inorganic fertiliser (about three 50 kg bags), used 21 kg of seed, and allocated 0.9 ha of land to maize. Each household had an average of about 2.3 ha of arable land and used at least three person-days of labour in the maize fields.
Table 3. Production data and socio-economic characteristics of households
In terms of socio-economic characteristics, the smallholder producers in the sample had fair access to extension services: 58% had received extension service visits, at an average of four visits per season. Access to credit was less common, at only 12% of farmers in the sample. The farmers were predominantly male (76%), and the majority had attained at least secondary education (90%). They were relatively experienced, with 19 years of farming experience on average, and an average age of 51.2 years. Cattle ownership was also a relatively common, at 53% of farmers. Household sizes were relatively large (6 persons), which is a concern given the small tracts of arable land they owned (2.3 ha). Weeding frequency averaged twice per season. Just over a third (35%) of the farmers practised conservation agriculture. The study pooled smallholder farmers from four districts: 30% from Goromonzi, 33% from Guruve, 22% from Mudzi and 15% from Hwedza.
6.2. Estimation results
Table shows the maximum likelihood estimates of the estimated stochastic frontier production function and the determinants of technical efficiency. All the coefficients in the model for productive factors (fertiliser, labour, seed and area under maize) are positive. The coefficients are significant at 1%, except for labour, which is significant at 5%. The coefficient for inorganic fertiliser of 0.22 indicates that maize output is elastic to changes in the application of fertiliser. A 1% increase in fertiliser use should induce a 22% increase in maize output, and vice versa. This is not surprising, since the use of fertiliser tends to increase production. This finding is consistent with those of Goldman (Citation2013).
Table 4. Input elasticity and socio-economic determinants of inefficiency
The positive coefficient for seed application is somewhat surprising, since a high seed application rate would be expected to be negatively associated with output. Goldman (Citation2013) argued that high seed application rates would result in higher plant densities, or overcrowding, which would have a negative effect on output. One explanation could be that farmers lack the resources to purchase all the required inputs, and hence apply seed at below-optimum rates. Should they be able to increase the seed rate, maize output would increase.
Labour had an elasticity of 0.14, which implies that a 1% increase in labour (person-days), ceteris paribus, would lead to a 14% increase in maize output. The significance of labour for maize output is not surprising, since smallholder farmers are known to be resource-constrained and maize production is labour-intensive, which means the farmers rely heavily on manual labour. They would therefore be concerned with maximising their labour output and would tend to emphasise technical efficiency.
The estimated coefficient for land used for maize cultivation is 0.21, showing that a 1% increase in area cultivated would induce a 21% change in maize output. This is again not surprising, since land is a significant factor in maize output. This result is consistent with those of Amaza and Olayemi (Citation2002) and Coelli and Battese (Citation1996).
The inefficiency parameters shown in Table relate to farm-specific characteristics and the farmer’s socio-economic position. The parameters include the gender of the household head, household size, weeding frequency, access to credit, the frequency of extension services, cattle ownership, the age of the household head, farming experience, farm size, a dummy variable for the different districts, the education of the household head and the treatment applied (conservation or conventional agriculture). The gender coefficient is estimated to be negative and statistically significant at 1%, which implies that male farmers are relatively more efficient in maize production. Considering that planting, weeding, harvesting, and other crop management operations are labour-intensive, this result is not surprising. Female farmers also have relatively less access to productive resources. The result could also be explained by the imbalance in resource’s access by gender. In literature, allocation of resources to poor women has a bigger impact on production and productivity; hence our result could imply the relatively low efficiency of women-headed maize farmers could be due to lack of access to productive resources.
The coefficient of household size is estimated to be positive and statistically significant at 10%. This implies that smaller farming households are more efficient, possibly because, as noted, larger household sizes exert pressure on the limited resources available to the smallholder farmer and seem to exacerbate poverty. Poverty-stricken farmers are more likely to be inefficient, as they cannot afford to buy productivity-enhancing inputs such as certified seed and fertiliser. In addition, the bigger the household, the higher the dependency ratio in most cases, which could well contribute to this finding. This result, however, contradicts Wang, Cramer, and Wailes (Citation1996), who find that household sizes are positively related to technical efficiency in Chinese agriculture.
The coefficient for the frequency of extension services is estimated to be negative and statistically significant at the 10% level. This indicates that more frequent extension services tend to increase technical efficiency, as extension agents provide advice on issues such as new technologies and production-related information. This finding is in line with those of Seyoum et al. (Citation1998), Parikh et al. (Citation1995) and Owens et al. (Citation2001).
Farm size was also a significant determinant of the technical efficiency of smallholder maize producers. The coefficient is negative and statistically significant at 10%, and implies that farmers with relatively large tracts of arable land tend to be more efficient. This could be because larger tracts allow farmers to diversify their activities, for instance by growing cash crops (e.g. legumes or tobacco) and keeping small livestock in addition to growing maize. This should increase their income, which would in turn enable them to increase their productivity. This result is consistent with that of Wadud and White (Citation2000), who found technical inefficiency to decrease as farm size increases. In contrast, Coelli and Battese (Citation1996), and Parikh et al. (Citation1995) argue that farmers with small tracts are forced to allocate their scarce resources more efficiently.
A dummy variable to capture the region was also included to explain variations between the various districts. Smallholder maize producers in Goromonzi and Guruve tended to be relatively more efficient (negative coefficients, significant at 1%), but those in Mudzi were relatively inefficient (positive coefficient, significant at 10%). This is not surprising since Goromonzi and Guruve are high-potential zones with favourable temperatures and rainfall patterns (700–800 mm per year), while Mudzi is a low-potential zone with low rainfall and higher temperatures. Farmers in low-potential zones tend to focus on drought-resistant crops, such as groundnuts and cowpeas.
Another significant characteristic of the stochastic frontier production function model is its ability to provide farm-specific estimates of technical efficiency. Figure shows a histogram with a kernel density graph of individual, farm-specific technical efficiency. The technical efficiency indices were derived from the analysis of the stochastic production function.
Figure 2. Kernel density estimates of the technical efficiency of individual smallholder maize producers.
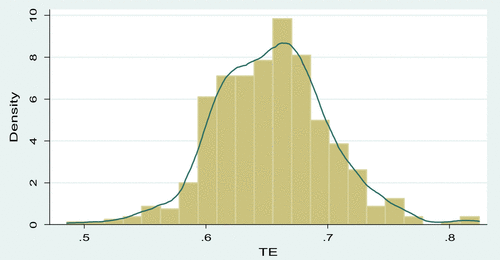
The mean level of technical efficiency for the sample of smallholder farmers is 0.65 (65%), with a standard deviation of 0.0467. This implies that, on the average, they could only achieve about 65% of the potential maximum output from a given mix of production inputs. The distribution of the technical efficiency scores ranges from 0.49 to 0.82. About 90% of the smallholder farmers have technical efficiency scores between 0.6 and 0.75. These results suggest that maize producers in Zimbabwe’s smallholder sector are constrained by a number of factors, such as infrequent extension visits (three times per season on average), smaller farm sizes (about 2 ha on average), relatively large household sizes (about 6 on average), and low agricultural potential in some areas. The potential for increasing the average efficiency among smallholder maize farmers in Zimbabwe is significant, at 35%. This would require improving farmer-specific efficiency factors, such as the frequency of extension services and proper land utilisation practices, given that farm sizes significantly influence efficiency.
7. Conclusions and recommendations
The study shows that inorganic fertiliser, seed and labour (both hired and family labour), as well as the area under maize are the major factors associated with changes in maize output. The effect of inorganic fertiliser on output is positive and the coefficient is statistically significant at 1%. The quantity of seed applied, the use of labour, and the land area allocated to maize production have positive effects on maize output, and their coefficients are statistically significant at 1, 5 and 1% respectively. The model for the inefficiency effects in the frontier function includes gender, household size, the frequency of extension services, farm size and region as significant determinants of technical efficiency. Male household headship, the frequency of extension services, and region1 (Goromonzi) and region2 (Guruve) are negatively associated with technical inefficiency, while household size and region3 (Mudzi) had a positive association.
The policy implications of these findings are that technical efficiency in smallholder maize production could be increased by 35% on average through better use of available resources (e.g. land, fertiliser, seed and labour), given the current state of technology. This could be achieved through improving farmer-specific efficiency factors, which include better and more frequent extension services, smaller household sizes, and the increased involvement of male farmers in maize production. The government could assist in improving the technical efficiency of the smallholder maize producers, who contribute more than 70% of national output, by ensuring better and more reliable support from extension services and empowering women farmers. Efficient extension services would improve crop management practices, for instance, by advising farmers on proper weeding regimes. Women farmers could be empowered by (at least) ensuring that they are not marginalised in accessing credit and other productivity-enhancing inputs. Access to credit, for example, would help ensure their timely access to inputs and thus promote efficiency.
The study, however, could only assess technical efficiency in smallholder maize production in Zimbabwe at a point in time. Given the importance of staple maize production to food security in both rural and urban communities, smallholder farmers would need to operate as efficiently as possible with the available inputs. Results from the analysis contribute to the current debate in Zimbabwe of finding ways of raising maize productivity that has fallen due to the radical changes caused by the land invasions in 2000. Results indicate some key sources of inefficiency in the current maize production system which can be targeted by policy to improve productivity in the maize production sector. Continuous improvement in the technical efficiency of maize production could promote income growth and reduce poverty. Ongoing monitoring of technical efficiency in maize production is therefore necessary to assess changing agricultural contexts and inform policy actions. This calls for more and ongoing research.
Acknowledgement
The authors would like to thank Janine Thorne for editing this article.
Additional information
Funding
Notes on contributors
Nelson Mango
Nelson Mango is a rural development sociologist specialised in agrarian transformation processes, with an emphasis on small-scale farming, technology development and social change, endogenous development and rural livelihoods. Over the years, he has done substantive fieldwork on socio-technical dimensions of maize, zero grazing dairy farming, and soil fertility (re)production in East Africa. He has also worked on livestock, livelihoods and poverty in southern Asia and sub-Saharan Africa using a wide range of methodologies drawn from both quantitative and qualitative research approaches. In the southern Africa region, his research focused on Conservation Agriculture and how to improve farmers’ livelihoods through multi-stakeholder innovation platforms and linking them to extension and business development services. Currently his research work has shifted to East and Central Africa with focus on value chains for nutrition and health, and Policy, Institutions and Markets. Theoretically, he combines actor perspective with broader questions related to political economy.
References
- Aigner, D., Lovell, C. A., & Schmidt, P. (1977). Formulation and estimation of stochastic frontier production function models. Journal of Econometrics, 6, 21–37.https://doi.org/10.1016/0304-4076(77)90052-5
- Amaza, P. S., & Olayemi, J. K. (2002). Analysis of technical inefficiency in food crop production in Gombe State, Nigeria. Applied Economics Letters, 9, 51–54.https://doi.org/10.1080/13504850110048523
- Battese, G. E. (1992). Frontier production functions and technical efficiency: A survey of empirical applications in agricultural economics. Agricultural Economics, 7, 185–208.https://doi.org/10.1016/0169-5150(92)90049-5
- Binam, J. N., Tonyè, J., Wandji, N., Nyambi, G., & Akoa, M. (2004). Factors affecting the technical efficiency among smallholder farmers in the slash and burn agriculture zone of Cameroon. Food Policy, 29, 531–545.https://doi.org/10.1016/j.foodpol.2004.07.013
- Bravo-Ureta, B. E. (2007). Technical efficiency in farming: A meta-regression analysis. Journal of Productivity Analysis, 27, 57–72.https://doi.org/10.1007/s11123-006-0025-3
- Coelli, T. J. (1995). Recent developments in frontier modelling and efficiency measurement. Australian Journal of Agricultural Economics, 39, 219–245.https://doi.org/10.1111/j.1467-8489.1995.tb00552.x
- Coelli, T. J., & Battese, G. (1996). Identification of factors which influence the technical inefficiency of Indian farmers. Australian Journal of Agricultural Economics, 40, 103–128.https://doi.org/10.1111/j.1467-8489.1996.tb00558.x
- Goldman, D. (2013). Technical efficiency of rice production in India: A study using stochastic frontier analysis to estimate technical efficiency and its determinants (Thesis for the Master of Arts in Law and Diplomacy Capstone Project). Tufts University, Medford, MA.
- Government of Zimbabwe. (2002). Crops sector. Harare: Central Statistical Office.
- Heshmati, A., & Mulugeta, Y. (1996). Technical efficiency of the Ugandan matoke farms. Applied Economics Letters, 3, 491–494.https://doi.org/10.1080/758540813
- Kalirajan, K. (1991). The importance of efficient use in the adoption of technology: A micro panel data analysis. Journal of Productivity Analysis, 2, 113–126.https://doi.org/10.1007/BF00156342
- Kumbhakar, S. C., Ghosh, S., & McGuckin, J. T. (1991). A generalized production frontier approach for estimating determinants of inefficiency in US dairy farms. Journal of Business and Economic Statistics, 9, 279–286.
- Llewelyn, R. V., & Williams, J. R. (1996). Nonparametric analysis of technical, pure technical, and scale efficiencies for food crop production in East Java, Indonesia. Agricultural Economics, 15, 113–126.https://doi.org/10.1016/S0169-5150(96)01202-9
- Mano, R. (2006). Zimbabwe smallholder agriculture performance and recurrent food security crisis: Causes and consequences. Paper prepared for the Centre for Applied Social Science (CASS), University of Zimbabwe, Harare.
- Mazvimavi, K., Ndlovu, P. V., An, H., & Murendo, C. (2012, August 18–24). Productivity and efficiency analysis of maize under conservation agriculture in Zimbabwe. Paper presented at the International Association of Agricultural Economics (IAAE) Triennial Conference, Foz do Iguaçu.
- Meeusen, W., & van Den Broeck, J. (1977). Efficiency estimation from Cobb–Douglas production functions with composed error. International Economic Review, 18, 435–444.https://doi.org/10.2307/2525757
- Mochebelele, M. T., & Winter-Nelson, A. (2000). Migrant labor and farm technical efficiency in Lesotho. World Development, 28, 143–153.https://doi.org/10.1016/S0305-750X(99)00116-3
- Msuya, E. E., Hisano, S., & Nariv, T. (2008, January 6–11). An analysis of technical efficiency of maize farmers in Tanzania in the globalization era. Paper presented in the XII World Congress of Rural Sociology of the International Rural Sociology Association, Goyang.
- Mugandani, R., Wuta, M., Makarau, A., & Chipindu, B. (2012). Re-classification of agro-ecological regions of Zimbabwe in conformity with climate variability and change. African Crop Science Journal, 20, 361–369.
- Narala, A., & Zala, Y. C. (2010). Technical efficiency of rice farms under irrigated conditions in Central Gujarat. Agricultural Economics Research Review, 23, 375–381.
- Owens, T., Hoddinott, J., & Kinsey, B. (2001). The impact of agricultural extension on farm production in resettlement areas of Zimbabwe (Working Paper CSAE WPS/2001–6). Oxford: Centre for the Study of African Economies (CSAE), University of Oxford.
- Parikh, A., Ali, F., & Shah, M. K. (1995). Measurement of economic efficiency in Pakistani agriculture. American Journal of Agricultural Economics, 77, 675–685.https://doi.org/10.2307/1243234
- Parikh, A., & Shah, K. (1994). Measurement of technical efficiency in the north-west frontier province of Pakistan. Journal of Agricultural Economics, 45, 132–138.https://doi.org/10.1111/jage.1994.45.issue-1
- Seyoum, E. T., Battese, G. E., & Fleming, E. M. (1998). Technical efficiency and productivity of maize producers in eastern Ethiopia: A study of farmers within and outside the Sasakawa-Global 2000 project. Agricultural Economics, 19, 341–348.https://doi.org/10.1016/S0169-5150(98)00037-1
- Sherlund, S. M., Barrett, C. B., & Adesina, A. A. (2002). Smallholder technical efficiency controlling for environmental production conditions. Journal of Development Economics, 69, 85–101.https://doi.org/10.1016/S0304-3878(02)00054-8
- Wadud, A., & White, B. (2000). Farm household efficiency in Bangladesh: A comparison of stochastic frontier and DEA methods. Applied Economics, 32, 1665–1673.https://doi.org/10.1080/000368400421011
- Wang, J., Cramer, G. L., & Wailes, E. J. (1996). Production efficiency of Chinese agriculture: Evidence from rural household survey data. Agricultural Economics, 15, 17–28.https://doi.org/10.1016/S0169-5150(96)01192-9
- Weir, S. (1999). The effects of education on farmer productivity in rural Ethiopia (Working Paper CSAE WPS99–7). Oxford: Centre for the Study of African Economies (CSAE), University of Oxford.
- Weir, S., & Knight, J. (2000). Education externalities in rural Ethiopia: Evidence from average and stochastic frontier production functions (Working Paper CSAE WPS/2000–04). Oxford: Centre for the Study of African Economies (CSAE), University of Oxford.