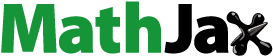
Abstract
In this study, we combine the latent class stochastic frontier model with the complex time decay model to form a single-stage approach that accounts for unobserved technological differences to estimate efficiency and the determinants of efficiency. In this way, we contribute to the literature by estimating “pure” efficiency and determinants of productive units based on the class structure. An application of this proposed model is presented using data on the Ghanaian banking system. Our results show that inefficiency effects on the productive unit are specific to the class structure of the productive unit and therefore assuming a common technology for all productive units as is in the popular Battese and Coelli model used extensively in the literature may be misleading. The study therefore provides useful empirical evidence on the importance of accounting for unobserved technological differences across productive units. A policy based on the identified classes of the productive unit enables a more accurate and effectual measures to address efficiency challenges within the banking industry, thereby promoting financial sector development and economic growth.
JEL classification:
Public Interest Statement
In this paper, we attempt to show that it is important to account for the differences in technology of productive units in order to accurately estimate their efficiency and the determinants of efficiency. In doing this, we use a latent class stochastic frontier model that account for underlying technology differences to derive efficiency as well as determinants. Applying the model using data on the Ghanaian banking system, our results show that inefficiency effects on the productive unit are specific to the class structure of the productive unit and therefore assuming a common technology for all productive units as is normally done in the literature may be misrepresentative. The study therefore provides useful empirical evidence on the importance of accounting for technological differences across productive units. A policy based on the identified classes of the productive unit enables a more accurate and effectual decision-making.
1. Introduction
Estimating efficiency of productive units and its determinants within the stochastic frontier framework is widespread in the applied economic literature. There are many empirical applications of efficiency analysis in agriculture, banking, hospitals, education and municipal services among others due to its importance to managers and policy-makers (see Fried, Lovell, & Schmidt, Citation1993). Generally, studies on the determinants of efficiency have employed the popular Battese and Coelli (Citation1992, Citation1995) complex time decay model (herein after, BC). The Battese and Coelli (Citation1995) model is preferred over the other frontier techniques, in that it overcomes the contradiction inherent in the two-stage approach and allows the simultaneous estimation of the parameters of the stochastic production frontier and the inefficiency effects model.Footnote1 The estimation of the stochastic production frontier functions of these Battese and Coelli (Citation1992, Citation1995) models rest on the assumption that the underlying production technology is common to all productive units. However, productive units in a particular industry may use different technologies. In such a case, estimating a common frontier encompassing every sample observation may not be appropriate in the sense that the estimates from the underlying technology may be biased. If the unobserved technological differences are not taken into account during estimation, the effects of these omitted unobserved technological differences might be inappropriately labelled as inefficiency (see Greene, Citation2005; O’Donnell & Griffiths, Citation2006; Orea & Kumbhakar, Citation2004). As a result, the estimated inefficiency is not likely to represent “pure” inefficiency of the productive unit and therefore the determinants of inefficiency may also be biased.
In order to reduce the likelihood of this type of misspecification, within the stochastic frontier framework, a few studies have combined the stochastic frontier approach with the latent class structure in order to estimate a mixture of frontier functions, i.e. the latent class stochastic frontier model thereby accounting for differences in technology to measure efficiency (see Barros, de Menezes, & Vieira, Citation2013; Caudill, Citation2003; Greene, Citation2005; O’Donnell & Griffiths, Citation2006; Orea & Kumbhakar, Citation2004). Nonetheless, the many articles on the determinants of efficiency using stochastic frontier methods have employed the BC model and therefore do not account for unobserved technological differences (see Apergis & Alevizopoulou, Citation2011; Isshaq & Bokpin, Citation2012; Tahir & Haron, Citation2008 among others). In this study, we combined the latent class stochastic frontier model with Battese and Coelli (Citation1995) complex time decay model to form a single-stage approach (herein after, latent class BC) that accounts for unobserved technological differences to measure efficiency and more importantly examine the determinants of efficiency based on the class structure of the productive unit. In this way, we contribute to the literature by estimating “pure” efficiency and determinants of productive units based on the class structure to promote the formulation of cogent policies for efficient decision-making and management of resources.
An application of this proposed model is presented using data on the Ghanaian banking system. The Ghanaian banking industry consists of 27 banks under the supervision of the Central Bank. It comprises 15 foreign-owned and 12 domestic-owned banks. The foreign-owned banks account for about 51% of total industry assets, while the largest state-owned bank accounts for 11% of the industry assets, 7% share of total industry loans and advances and 11.3% of total industry deposits as of December 2012. Universal banks have operations extending into commerce and corporate lending, international trade financing, treasury financing and loan syndication among several other services that were hereto in the domain of specialized players such as development, merchant and commercial banks. This development was driven by the Banking Act (2004), which led to the abolishing of the specialized banking regime. Under the Act, banks were required to increase the minimum capital requirement to $US8 m. This figure has subsequently been increased to $US30m and $US60m in 2012 and 2013, respectively. The past decade also witnessed the drive towards the computerization of banking operations with the introduction of automated teller machines (ATMs). The number of ATMs across the country stood at 618 as of 2011. This has resulted in the introduction of telephone, SMS and internet banking products (Alhassan, Citation2015).
There are a number of studies on banking sector efficiency in Ghana, however only a few have applied stochastic frontier methods. Most of these studies have employed non-parametric approaches such as the Data Envelopment Analysis (DEA) and have concentrated mostly on efficiency analysis of banks (see Adjei-Frimpong, Gan, & Hu, Citation2014; Saka, Aboagye, & Gemegah, Citation2012) with the exception of Isshaq and Bokpin (Citation2012). As indicated earlier, these studies on Ghana among others do not account for unobserved technological differences in the estimation as well as the determinants of efficiency.
Given the importance of investment in technology in the Ghanaian banking industry, there are significant technological differences among banks primarily with respect to electronic banking, mobile banking services and Point of Sale systems. For instance, internet banking is not as popular; and a few of the banks that offer internet banking services have only now enabled their systems to allow their customers to complete transactions such as online funds transfer (PwC Ghana Banking Survey, Citation2014). Using inefficiency covariates such as inflation, size of bank, bank concentration and banks return on assets, which have been used extensively as determinants of bank efficiency (see Alhassan, Citation2015; Casu, Girardone, & Molyneux, Citation2004; Darrat, Topuz, & Youzef, Citation2002; Isshaq & Bokpin, Citation2012; Saka et al., Citation2012 among others), we employ both the BC and latent class BC to examine the effects of these covariates on bank efficiency. In this way, we are able to show that the inefficiency effects on the productive unit, in this case, banks are specific to the class structure of the bank.
The rest of the study is discussed under five sections. Section 2 discusses the methodology. Sections 3 and 4 present the data and discuss the empirical results, respectively. The last Section 5 concludes.
2. Methodology
In this section, we present a stochastic frontier model that combines the latent class stochastic frontier model with Battese and Coelli (Citation1995) complex time decay model. Following from the application of the proposed model, the presentation is done with application to banks. As per the application to banks, the performance or technical efficiency of banks is defined as the ability of the bank to transform (multiple) resources into (multiple) financial resources (see Bhattacharyya, Lovell, & Sahay, Citation1997). We follow the banking literature and use the intermediation approach proposed by Sealey and Lindley (Citation1977) to define inputs and outputs. In this study, we include loans and advances as output and deposits, staff cost (proxy for labour input) and fixed assets (proxy for capital input) as inputs. In other words, loans and advances of banki at time t is given by:(1)
(1)
where Yit is loans and advances of banki at time t, f (.) is suitable functional form, Dit, Lit and Kit are defined as deposits, labour and capital for banki at time t, respectively. We assume that some banks may lack the ability to employ existing inputs as efficiently as possible and consequently produce less than the optimal output. Therefore, the actual observable output produced by each banki at time t (Yit) is then better described by the following stochastic frontier production function:(2)
(2)
T is a time trend common to all banks and β is an unknown parameter to be estimated. TEit represent technical efficiency and is defined as the exponential of −uit, where uit>0 and is a measure of the shortfall of output from the frontier (technical inefficiency) for each bank in the sample. vit embodies measurement errors, any statistical noise and random variations of the frontier across banks.
Writing Equation (2) in logarithms form, we have:(3)
(3)
Replacing TEit with exp (−uit), Equation (3) can be reformulated as:(4)
(4)
uit > 0, but vit may take any value and is assumed to be a half-normal distribution.
We apply the Cobb-Douglas specificationFootnote2 to characterize the stochastic production frontier as:(5)
(5)
where yit represents the logarithm of Yit and xnit denotes an n-th input variable. For convenience, the Cobb-Douglas production frontier function in Equation (5) can be rewritten as:(6)
(6)
where the proxy for technical change T is included in .
Following from the base model in Equation (6), the popular version of the Battese and Coelli model can be specified as(7)
(7)
where zit is a vector of explanatory variables in addition to time that may affect inefficiency, η is a vector of parameters, t is the period and T is the last period. With respect to zit explanatory variables that affect inefficiency, although there is an increasing concern about the endogeneity issue in the empirical literature, in practice, as Greene (Citation2011) points out, dealing with the issue in non-linear models such as the stochastic frontier analysis is rather complicated.Footnote3 Moreover, as Mutter, Greene, Spector, Rosko, and Mukamel (Citation2013) stressed, the literature on stochastic frontier analysis does not offer clear guidance on how to tackle the endogeneity problem. In this paper, we address the problem of endogeneity using lags of our explanatory variables (see Iyer, Rambaldi, & Tang, Citation2008).
As indicated, in the Battese and Coelli model and other standard stochastic frontier approaches, the frontier function is the same for every firm, therefore inefficiency is estimated relative to the frontier for all observations. However, in the latent class stochastic frontier model, we estimate as many frontiers as the number of classes simultaneously. The latent class stochastic frontier model extended to the Battese and Coelli specification is presented as follows;(8)
(8)
where j indicates class j.
Following from Orea and Kumbhakar (Citation2004) and Greene (Citation2005), the latent class stochastic frontier model in Equation (8) is estimated using maximum likelihood methods. In a latent class model, the unconditional likelihood for productive unit i (banki) is obtained as a weighted sum of their j-class likelihood functions, where the weights are the probabilities of class membership. The probabilities reflect the uncertainty that we might have about the true partitioning in the sample. That is(9)
(9)
where ∏ (i, j) is the prior probability attached to membership in class j. The class probabilities are parameterized as a multinomial logit model,(10)
(10)
where zi is a vector of productive unit (bank) specific variables.Footnote4 The class membership is estimated by j*, the one with the largest posterior probability.
3. Data and variables
The latent class stochastic frontier model extended to the Battese and Coelli framework is applied to a panel of 27 Ghanaian banks over the 2006–2010 period. Following from the banking literature, we use the intermediation approach by Sealey and Lindley (Citation1977) to define outputs and inputs. As indicated, the variables in the stochastic production frontier are loans and advances, which include loans and advances to bank customers as output; the three inputs are deposits made up of customers deposits and deposits from other financial institutions, staff cost (proxy for labour input) consisting of expenditure on employees, and fixed assets (proxy for capital input) made up of book values of fixed assets. The trend variable, t = 1, 2, 3, 4 and 5 for years 2006, 2007, 2008, 2009 and 2010. Following from the review of the literature on banks (Alhassan, Citation2015; Casu et al., 2003; Isshaq & Bokpin, Citation2012; Saka et al., Citation2012 among others), the explanatory variables in the inefficiency model are inflation, total assets, bank concentration and ROA of banks. The data-set is sourced from the Banking supervision Department of the Bank of Ghana. Table present the summary statistics of the input and output variables.
Table 1. Summary statistics of input and output variables (Figures in Ghana Cedi)
4. Empirical results
We first estimate the BC model before proceeding to estimate a latent class BC specification in order to compare our results. In estimating the latent class model, one has to address the problem of determining the number of classes. In this study, we use the testing down strategy suggested by Greene (Citation2003). We test down from 4 to 3 and 3 to 2 classes. The preferred model is that with two classes.
The estimated prior class probabilities are on average 50%. The highest value is obtained for the second class with a prior class probability of 54% (see Table ). The classification resulting from these prior probabilities shows that the largest group (second class) is mainly formed by banks with larger total assets. The average total assets of these banks are much larger than the banks in the other class.
Table 2. Estimated prior probabilities for class membership
A comparison of the inefficiency estimates for the BC model and the latent class BC model using kernel density estimators show that the inefficiency estimates of the BC specification are far larger than that of the latent class BC specification (see Figures and ). Both mean and variation of the distributions for estimated inefficiencies of the Battese and Coelli specification are larger, indicating that the latent class stochastic frontier extension of the Battese and Coelli specification performs better than the Battese and Coelli.
Following from the latent class BC specification, the average efficiency of Ghanaian banking sector as a whole is 0.56. There are however substantial differences in efficiency levels among classes. While the average efficiency in the first class is 0.479, it increases to 0.646 in the second class (see Table ). A further disaggregation of efficiency of banks in different number of class is also presented in Table . Though the sample is made up of commercial banks (that is, banks that are largely engaged in retail banking by providing savings and loans services to individuals and commercial firms), the classification of these banks into classes can be connected to factors such as branch network capacity. For instance, we observed banks in class 1 have an average of 36 branches, while banks in class 2 have an average of 23 branches. In addition, banks in class 2 have a relative concentration of branches in urban areas and leverages on technology-driven banking services such as internet banking, SMS banking, among others, compared to their competitors.
Table 3. Average annual efficiency indexes in different number of class
Table 4. Average efficiency indexes of Banks in different number of class
With regard to the inefficiency effects or the determinants of inefficiency, we examine the effects of these covariates on inefficiency in the BC model and the latent class BC model where efficiency effects are estimated separately for each class. Our results show that the effect of these covariates on inefficiency is not the same across the BC model and the classes of the latent class BC (see Table ). Inflation significantly reduces inefficiencies in the BC model and in the first class of the latent class BC but it is the opposite in the second class. Increases in total assets also reduce inefficiency in the BC model but its effects are not significant in the first class of the latent class BC. The ROA although significantly decreases inefficiency in the BC model, its effects are not significant in both classes of the latent class BC. In effect, the determinants of inefficiency of banks in Ghana in this case are specific to the class structure of the bank when we account for technological differences. This implies that the covariates of efficiency of a productive unit may be specific to the class structure and therefore different policy measures needs to be formulated for different productive units based on the class structure in order to ensure efficiency.
Table 5. Maximum likelihood estimates for parameters of the Cobb-Douglas stochastic frontier production function with efficiency component
5. Conclusions
In explaining inefficiency of productive units in the stochastic frontier framework, most studies have employed the popular Battese and Coelli (Citation1995) complex time decay model. However, the estimation of the stochastic production frontier functions of the Battese and Coelli models rest on the assumption that the underlying production technology is common to all productive units. Nonetheless, productive units in a particular industry may have differences in technologies. In this study, we combined the latent class stochastic frontier model with Battese and Coelli (Citation1995) complex time decay model to form a single-stage approach that accounts for unobserved technological differences to measure efficiency and examine the determinants of efficiency based on the class structure of the productive unit. In this way, we contribute to the literature by showing that the determinants of efficiency of a productive unit is specific to the class structure if there are unobserved technological differences. However, it is important to point out that the stochastic frontier analysis employed for the study does not offer clear guidance on how to tackle endogeneity. This may be a limitation of the study.
Using data on the Ghanaian banking system, our results show that the effects of the covariates of inefficiency on banks in Ghana is specific to the class structure of the bank when we account for technological differences. The findings indicate that inefficiency effects on the productive unit are specific to the class structure of the productive unit and therefore assuming a common technology for all productive units as in the Battese and Coelli model and in many empirical studies (if there are technology differences) may be misleading. Given the importance of accurate policies to promote efficiency and increased output, identifying the class structure of the productive unit would enable policy-makers to put in place appropriate measures to reduce inefficiency and boost productivity within the banking industry thereby enhancing financial development and promoting economic growth.
Additional information
Funding
Notes on contributors
Michael Danquah
Michael Danquah is an economist and lecturer at the Department of Economics, University of Ghana, Legon. He holds a PhD in Economics from Swansea University (UK). His research interests include informality, inclusive growth and stochastic frontier modelling. He has published in journals such as Economic Modelling and Empirical Economics among others. In 2015, he was interviewed by BBC World Service on the Live 8, G8 and the making of Poverty History program.
Peter Quartey
Peter Quartey holds a PhD in Development Economics from the University of Manchester (UK). He is an associate professor in Development Economics and currently the head, Department of Economics, University of Ghana. He is also an economist with the Institute of Statistical, Social and Economic Research, University of Ghana. He was formerly the deputy director, Centre for Migration Studies. He has published extensively and his research interests are private sector development, development finance, migration and remittances and poverty analysis.
Notes
1. Kumbhakar, Ghosh, and McGuckin (Citation1991), Reifschneider and Stevenson (Citation1991) and Huang and Liu (Citation1994) are some of the earlier studies that presented models to overcome this problem in the two-stage approach (i.e. the first stage assumes that inefficiencies are independent and identically distributed, while the second stage contradicts the identical distribution assumption of the first stage) by estimating both the frontier and efficiency effects in one stage.
2. We apply the Cobb-Douglas specification because the estimated variance matrix of the flexible translog specification was singular.
3. Greene (Citation2011) also notes that no accepted approach for estimating unbiased efficiency estimates with endogeneity is currently available for SFA.
4. Following the work by Greene (Citation2003), it is worth noting that the posterior class probabilities do not depend only on the estimated δ parameters above but also on the vector parameters from the production frontier.
References
- Adjei-Frimpong, K., Gan, C., & Hu, B. (2014). Cost efficiency of Ghana’s banking industry: A panel data analysis. International Journal of Business and Finance, 8, 69–86.
- Alhassan, A. L. (2015). Explaining bank productivity in Ghana. Managerial and Decision Economics. doi:https://doi.org/10.1002/mde.2748
- Apergis, N., & Alevizopoulou, E. (2011). Bank efficiency: Evidence from a panel of European banks. Panoeconomicus, 58, 329–341.https://doi.org/10.2298/PAN1103329A
- Barros, C. P., de Menezes, A., & Vieira, J. S. (2013). Measurement of hospital efficiency, using a latent class stochastic frontier model. Applied Economics, 45, 47–54.https://doi.org/10.1080/00036846.2011.579061
- Battese, G. E., & Coelli, T. J. (1992). Frontier production functions, technical efficiency and panel data: With application to paddy farmers in India. Journal of Productivity Analysis, 3, 153–169.https://doi.org/10.1007/BF00158774
- Battese, G. E., & Coelli, T. J. (1995). A model for technical inefficiency effects in a stochastic frontier production function for panel data. Empirical Economics, 20, 325–332.https://doi.org/10.1007/BF01205442
- Bhattacharyya, A., Lovell, C. A. K., & Sahay, P. (1997). The impact of liberalization on the productive efficiency of Indian commercial banks. European Journal of Operational Research, 98, 332–345.https://doi.org/10.1016/S0377-2217(96)00351-7
- Casu, B., Girardone, C., & Molyneux, P. (2004). Productivity change in European banking: A comparison of parametric and non-parametric approaches. Journal of Banking and Finance, 28, 2521–2540.https://doi.org/10.1016/j.jbankfin.2003.10.014
- Caudill, S. (2003). Estimating a mixture of stochastic frontier regression models via the EM algorithm: A multiproduct cost function application. Empirical Economics, 28, 581–598.
- Darrat, A. F., Topuz, C., & Youzef, T. (2002). Assessing cost and technical efficiency of banks in Kuwait. Paper presented at the ERF’s 8th Annual Conference, Cairo, ERF, Cairo. Retrieved from www.erf.org.eg
- Fried, H. O., Lovell, C. A. K., & Schmidt, S. S. (1993). The measurement of productive efficiency: Techniques and applications. New York, NY: Oxford University Press.
- Greene, W. (2003). The economic approach to efficiency analysis. In H. Fried, C. Lovell, & S. Schmidt (Eds.), The measurement of productive efficiency and productivity growth (pp. 92–250). New York, NY: Oxford University Press.
- Greene, W. (2005). Reconsidering heterogeneity in panel data estimators of the stochastic frontier model. Journal of Econometrics, 126, 269–303.https://doi.org/10.1016/j.jeconom.2004.05.003
- Greene, W. (2011). Econometric analysis (7th ed.). Upper Saddle River, NJ: Prentice-Hall.
- Huang, C. J., & Liu, J. T. (1994). Estimation of a non-neutral stochastic frontier production function. Journal of Productivity Analysis, 5, 171–180.https://doi.org/10.1007/BF01073853
- Isshaq, Z., & Bokpin, G. A. (2012). Expansion and efficiency in banking: Evidence from Ghana. Managerial Decision and Economics, 33, 19–28.
- Iyer, K. G., Rambaldi, A. N., & Tang, K. K. (2008). Efficiency externalities of trade and alternative forms of foreign investment in OECD countries. Journal of Applied Econometrics, 23, 749–766.https://doi.org/10.1002/jae.v23:6
- Kumbhakar, S. C., Ghosh, S., & McGuckin, J. T. (1991). A generalized production frontier approach for estimating determinants of inefficiency in US dairy farms. Journal of Business and Economic Statistics, 9, 279–286.
- Mutter, R. L., Greene, W. H., Spector, W., Rosko, M. D., & Mukamel, D. B. (2013). Investigating the impact of endogeneity on inefficiency estimates in the application of stochastic frontier analysis to nursing homes. Journal of Productivity Analysis, 39, 101–110.https://doi.org/10.1007/s11123-012-0277-z
- O’Donnell, C., & Griffiths, W. (2006). Estimating state-contingent production frontiers. American Journal of Agricultural Economics, 88, 249–266.https://doi.org/10.1111/j.1467-8276.2006.00851.x
- Orea, L., & Kumbhakar, S. (2004). Efficiency measurement using a latent class stochastic frontier model. Empirical Economics, 29, 169–183.https://doi.org/10.1007/s00181-003-0184-2
- PwC Ghana Banking Survey. (2014). The future of banking in Ghana… what is next?
- Reifschneider, D., & Stevenson, R. (1991). Systematic departures from the frontier: A framework for the analysis of firm inefficiency. International Economic Review, 32, 715–723.https://doi.org/10.2307/2527115
- Saka, A. N. A., Aboagye, A. Q. Q., & Gemegah, A. (2012). Technical efficiency of the Ghanaian banking industry and the effects of the entry of foreign banks. Journal of African Business, 13, 232–243.https://doi.org/10.1080/15228916.2012.727755
- Sealey, C., & Lindley, J. (1977). Inputs, outputs, and a theory of production and cost at depository financial institutions. Journal of Finance, 32, 1251–1266.https://doi.org/10.1111/j.1540-6261.1977.tb03324.x
- Tahir, I. M., & Haron, S. (2008). Technical efficiency of the Malaysian commercial banks: A stochastic frontier approach. Banks and Bank Systems, 3, 65–72.