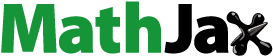
Abstract
We investigate the relationship between idiosyncratic risk and return among four water exchange traded funds—PowerShares Water Resources Portfolio, Power Shares Global Water, First Trust ISE Water Index Fund, and Guggenheim S&P Global Water Index ETF using the Markov switching model for the period 2007–2015. The generated transition probabilities in this paper show that there is a high and low probability of switching between Regimes 1 and 3, respectively. Moreover, we find that the idiosyncratic risk for most of the exchange traded funds move from low volatility (Regime 2) to very low volatility (Regime 1 and 3). Our study also identify that the beta coefficients are positive and entire values are less than 1. Thus, it seems that water investment has a lower systematic risk and a positive effect on the water exchange traded index funds returns during different regimes.
Public Interest Statement
Water is essential for life and has been studied to some extent but not as much in terms of major investments and their associated risks. The issues related to climate change has increased the risk of even more people in the world living with lack of adequate water for drinking. To remove this crisis, water investments in a number of areas are urgently needed. This study provides impetus for further study of the financial aspects of the water industry and its related risks. In particular, this paper contributes by increasing the investor’s understanding of the idiosyncratic risk of water investments. The results and findings will guide investors’ in decision-making.
1. Introduction
Although water is essential for life, it is in severe shortage around the world (Tularam & Properjohn, Citation2011). Water covers about two-thirds of the earth’s surface (Roca & Tularam, Citation2012; Tularam & Marchisella, Citation2014). Recent statistics suggest that more than 2.6 billion people of the world are living with lack of adequate water for drinking (Jin, Roca, Li, Wong, & Cheung, Citation2015a; Roca, Tularam, & Reza, Citation2015; Tularam & Marchisella, Citation2014) and the number has been increasing daily in that 3.9 billion persons could be reached by 2030 (Jin, Li, Roca, & Wong, Citation2015b; Organisation for Economic Co-operation & Development, Citation2008; Roca et al., Citation2015). Presently, more than one billion people are in acute drinking water availability problems in many developing countries including Bangladesh, Pakistan, and much of Africa (Jin et al., Citation2015b; Organisation for Economic Co-operation & Development, Citation2009; Roca et al., Citation2015; Tularam & Illahee, Citation2008; Tularam & Marchisella, Citation2014; Wild, Francke, Menzli, & Schon, Citation2007).
As the global population increases, water demand will unavoidably increase and there will be a critical need to make available affordable clean water as well as adequate sanitation for regional populations (Tularam, Citation2014; Tularam & Illahee, Citation2010; Tularam & Keeler, Citation2006; Tularam & Krishna, Citation2009; Tularam & Singh, Citation2009). The potential impacts of climate change on water availability and related impact on global agriculture will compound the existing problems of water availability. However, the urgently developing interfaces in many countries including much needed trade liberalizations together appear to present much important information on the values, benefits, and costs of investing in water (Roca et al., Citation2015; Tularam, Citation2014).
Clearly, if the water shortages are to be addressed, the existing global water industry needs to examine the rapidly changing market (Jin et al., Citation2015b; Roca & Tularam, Citation2012; Roca et al., Citation2015). There is a major drinking water crisis worldwide and therefore investments in water are urgently needed (Jin et al., Citation2015a; Roca et al., Citation2015). The rapidly changing dynamics of the modern world suggest that water investing can be increasingly popular to investors (Roca & Tularam, Citation2012; Tularam, Citation2014). Investing in water companies and water stocks has been the most commonly adopted approach as alternative to direct investment it seems (Jin et al., Citation2015a). If the water sector expands and the market being rather economically resilient, investments in this industry should lead to fruitful financial returns. Investors could also gain better diversification benefits by involving investments in water within their portfolios (Roca et al., Citation2015).
In recent times, the development of the water market has led to the development of water exchange traded index funds (ETFs). Water EFTs, are baskets of water-related shares aimed at replicating the performance of the water market. They offer investors the flexibility of buying or selling in the whole water market with a single transaction (Roca et al., Citation2015). For example, the American Stock Exchange (AMEX) introduced the Palisades Water Index (ZWI) in 2003, with a starting value of 1,000 points. And from August 2005, they started publishing this index on a regular basis. The ZWI is an equally weighted index comprised of companies engaging in the fundamental activities of the global water industry such as distribution, filtration, pumps and pipes, and treatment (Geman & Kanyinda, Citation2007; Jin et al., Citation2015b; Roca et al., Citation2015). Following the publication of the ZWI, two exchange traded funds (ETFs), namely, Power Shares Water Resources (PHO) and Power Shares Global Water (PIO) were successively introduced to investors to assist them to keep track of companies included in the ZWI (Wong, Roca, & Tularam, Citation2007; Roca et al., Citation2015).
Since March 2012 these two funds have been re-constructed to track the NASDAQ OMX Water Index (Jin et al., Citation2015b). As such, there are other ETFs available to investors, for example, Guggenheim S&P Global Water Index ETF (GGW)), which is based on the S&P Global Water Index; and First Trust ISE Water (FIW), which is based on the ISE Water Index (Atkinson, Citation2009). However, often a number of companies included in these water indices generate only a small portion of their revenue from water-related products or services. Therefore, questions have been raised such as the need to examine whether these water ETFs can be defined as pure water funds (Kearney, Citation2008; Roca et al., Citation2015).
To the best of our knowledge no study has investigated the relationship between idiosyncratic risk and return of ETFs in the water sector using Markov switching model. More importantly, in terms of the motivation of this study, none of studies reported appear to have investigated the changes of the idiosyncratic risk and risk-return relationship of the water ETF across different regimes. Therefore, this study contributes to investor’s understanding of the idiosyncratic risk of water investments. The results will guide investors’ in decision-making. Since the research on idiosyncratic risk of ETF is generally lacking, our study therefore aims to fill in this important gap. The main aim of this article was to investigate the relationship between idiosyncratic risk and return among four water exchange traded funds—PowerShares Water Resources Portfolio (PHO), PIO, First Trust ISE Water Index Fund (FIW), and Guggenheim S&P Global Water Index ETF using the Markov switching model for the period 2007–2015. In particular, we examine the effect of water exchange traded funds regimes on water investment using the Markov switching model.
The rest of this paper is organized as follows. In Section 2, we review the related literature. Section 3 outlines the data and methodologies employed in the study. Section 4 discusses the empirical results followed by the conclusion in Section 5.
2. Literature review
Much literature has focused on the issue of idiosyncratic risk. However, many researchers’ performed studies identifying the stock market unsystematic risk premium. For example, Aaker and Jacobson (Citation1987), Cox and Griepentrog (Citation1988), Barber (Citation1994), and Campbell, Lettau, Malkiel, and Xu (Citation2001), Goyal and Santa-Clara (Citation2003) find that unsystematic risk premium is positively related to return, whereas Cheung and Wong (Citation1992), Bali and Cakici (Citation2008), and Bollen, Skotnicki, and Veeraraghavan (Citation2009) report no significant relationships between the idiosyncratic risk and returns in the US, Australia, and Hong Kong markets, respectively.
Goyal and Santa-Clara (Citation2003) published a paper with the provocative title “Idiosyncratic risk matters!” They reported that there is a positive relation between market return and average idiosyncratic risk for the period of 1963–1999. Later, Bali, Cakici, Yan, and Zhang (Citation2005) expand the Goyal and Santa-Clara’s sampling period using two more years of data to find the relation uncovered by Goyal and Santa-Clara is sample specific. They note that sample is driven by small stocks traded on NASDAQ. A dependence on the weighting scheme is partly attributed to liquidity premium. Wei and Zhang’s (Citation2005) approach does not allow for the possibility that the relation between idiosyncratic risk and return may be different across high and low volatility states. Guo and Savickas (Citation2003) state that Goyal and Santa-Clara’s (Citation2003) did not find further co-movements of average stock volatility with stock market volatility.
Angelidis and Tessaromatis (Citation2009) perform regressions analysis of the monthly value weighted excess market return on lagged value (equally) weighted idiosyncratic volatility. The absence of a relation using value weighted idiosyncratic volatility is consistent with Bali et al. (Citation2005) and Wei and Zhang (Citation2005) research. However, in the case of equally weighted idiosyncratic volatility, it is noted that there is strong evidence of a positive relation only when the stock market is in the low volatility state. While the relation between returns and risk is positive it is not statistically significant in the high volatility state. Huimin, Cheung, and Roca (Citation2010) also find a positive relationship between the idiosyncratic risk and return during low and medium volatility states. Their results are consistent with that of Tang and Shum (Citation2007) and Angelidis and Tessaromatis (Citation2009); in the sense of the idiosyncratic risk premium under different market conditions.
Given the importance to water investment, the issue of idiosyncratic risk and returns has not received appropriate attention in the past literature. The water investment literature indicates that few studies have been done thus far (see Antoniou, Barr, & Priestley, Citation2000; Buckland & Fraser, Citation2000, Citation2001; Buckland & Williams, Citation2013; Geman & Kanyinda, Citation2007; Gilroy, Schreckenberg, & Seiler, Citation2013; Jin et al., Citation2015a,Citationb; Morana & Sawkins, Citation2000; Roca & Tularam, Citation2012; Roca et al., Citation2015). However, other studies on the systematic risk and return of investment in the water industry have been done in great depth (Wong et al., Citation2007). Perhaps the study by Geman and Kanyinda (Citation2007) is the “best” attempt to investigate the water investment. The authors measure the performance and volatility of the World Water Index (WOWAX) (December 2003–June 2006) and find that the index increased by more than 80% during this period (Jin et al., Citation2015a).
3. Data and methodology
3.1. Data
This study utilizes the PHO, PIO, First Trust ISE FIWand Guggenheim S&P Global Water Index ETF (GGW), all obtained from the Thomson Reuters DataStream database. The particular water exchange traded funds have been selected based on the completeness of data starting from the same date, 15 June 2007. The sample period is from 15 June 2007 to 31 August 2015. Daily data utilized is in the form of returns on the price indices; as calculated by the following formula: ; the returns are in US dollars (Figure ).
3.2. Methodology
Quandt (Citation1958), introduced a method of estimating the position of a single switching point for a linear regression system obeying two regimes. Goldfeld and Quandt (Citation1973) presented a particularly useful version of these models which is called a Markov switching model. Hamilton (Citation1989) proposed a multivariate generalization of the univariate Markov switching model. Particularly, we use Calice, Mio, Štěrba, and Vašíček (Citation2015) and Calice, Ioannidis, and Miao (Citation2012). According to them
When both and
are zeros, the two components will be in the high volatility state as
and
similarly if
and
equal 1, the two components will be in the low volatility state since
and
.
3.3. Transition probabilities
The regime generating process in Markov switching model is an ergodic Markov chain with a finite number of states which means that the current value of the process at time t depends only on its previous value at time t − 1 (Calice et al., Citation2012, Citation2015) and Garcia (Citation1998). The transition probabilities from one state to the other are shown below
The transition probability matrix P can be defined as follows:
Markov chain probability of Pij is shown as follows:
3.4. Regime probabilities
This procedure estimates the coefficient matrix, the variance–covariance matrix for each regime, the transition matrix, and the optimal inference for the regimes throughout the sample period. The latter is referred to as the regime probabilities defined subsequently, where T denotes the end period for the estimation.
Three types of regime probabilities are involved. However the choice depends on the differences in the existing results. The three types of regime probabilities are written as follows:
filtered regime probabilities
smoothed regime probabilities.
3.5. The relationship between idiosyncratic risk and return
We test the following Markov switching model by following the studies of Krolzig (Citation1997), Roca and Wong (Citation2008), Guidolin and Hyde (Citation2009), and Huimin et al. (Citation2010). We can regress that model as follows:
where is a multivariate regime switching process driven by a common discrete state variable,
is a vector of intercepts in states, β is the coefficient of market risk premium at lag k, and
is the vector of idiosyncratic return that is assumed to be joint normally distributed with zero mean and state-specific covariance matrix
.
Recently, financial models on the relationship between idiosyncratic risk and returns have been developed by Bali et al. (Citation2005), Roca and Wong (Citation2008), Angelidis and Tessaromatis (Citation2009), and Huimin et al. (Citation2010). We have explored the relation between idiosyncratic risk and returns by regressing daily water ETFs stock returns by implementing of the CAPM with a time-varying coefficient.
where represents the returns on an investment of water ETFs;
,
,
, and
are the time series regression coefficients of
;
in the equation to emphasize age returns across assets;
,
,
, and
are the returns on the CAPM.
is the intercept and
is the error term.
Further, we test the following model to run a regression of the relationship between idiosyncratic risk and returns of water ETFs in regime t by following Angelidis and Tessaromatis (Citation2009) and Huimin et al. (Citation2010) by running OLS time-series regressions. According to them
where represents the returns on an investment of water ETFs,
is the intercept, Xt is the idiosyncratic risk, and ξt is the error term.
4. Empirical results
4.1. Data preliminaries
From Table , it can be seen that stock returns are positive except PIO. The largest positive mean return (0.013%) is for FIW whereas the PIO has the lowest positive mean return (−0.009). The kurtosis values of all water stock exchange traded funds returns are higher than three, thus the returns distribution could be fat-tailed. As the skewness values are in general negative, the skewness values are the asymmetric tail. Since the Jacque-Bera results are statistically significant and reject the null hypothesis of a normal distribution for all stock returns. Nonetheless, our analysis is robust as models are usually robust as well in non-normal cases.
Table 1. Summary statistics for daily returns
4.2. Unit root test
The presence of a unit root in the water ETFs returns are tested using both the augmented Dicky–Fuller (ADF) and Phillips–Perron (PP). Table shows that ADF and PP testing procedures of the data PHO, FIW, and GGW at 1%; and PIO at 5% level of significance. Hence, the ADF and PP tests consistently reject the null hypothesis. Both unit root tests suggest the funds’ returns those of stocks are stationary. Consequently, the returns time series are used in the subsequent analysis without further differencing or testing for co-integration.
Table 2. Unit root tests results
4.3. Regime and transition probabilities
Table presents the corresponding probabilities and characteristics for each of the three regimes in the PHO, PIO, FIW, and GGW models. Table shows the funds stayed most of the time; and the longest time in Regime 2. The three numbers in a particular row show the probability of a regime shifting into Regime 1, 2, and 3, respectively. For example, in row 1, the first number 96.44%, which indicates the probability of Regime 1 shifting into Regime 1, this means Regime 1 staying in itself; while the second number 1.63% shows the probability of Regime 1 switching to Regime 2; 21.37% shows the probability of Regime 2 switching to Regime 3. 30.52% is the probability of Regime 3 staying in itself (and therefore, 13.95%, 41.33%, and 44.71% probability of shifting to Regimes 1, 2, and 3, respectively). Hence, 29.90%, 39.56%, and 30.52% are probabilities of shifting to Regime 1, 2, and 3, respectively.
Table 3. Transition probabilities and characteristics of Markov regime switching models
For the PIO, the probability of staying in Regime 1 is 50.78% (and consequently 27.83% is the probability of switching to Regime 2; and 21.37% probability of switching to regime 3). 33.42% is the probability of Regime 3 staying in itself and hence, 25.12% and 41.43% probability of shifting to Regime 2 and 1, respectively. Therefore, 43.46%, 2.75%, and 97.22% are probabilities of shifting to Regime 1, 2, and 3, respectively, as well.
Similarly, for the FIW, the probability of staying in Regime 1 is 3587% (therefore 45.68% probability of switching to Regime 2; and 43.46% probability of switching to Regime 3); and 20.2345% probability of Regime 3 staying in itself (951025.12% and 2.13% probabilities of shifting to Regime 2 and 1, respectively). Consequently, 43.46%, 02.75%, and 36.30% are probabilities of shifting to Regime 1, 2, and 3, respectively, as well. Again the probability of staying in Regime 1 of GGW is 08.81% (and therefore 39.50% probability of switching to Regime 2; and 51.68% 21.37%) and 02.09% in Regime 3. Consequently, 96.29% and 01.6041.43 are probabilities of shifting to Regime 2 and 1, respectively). Hence, 61.30, 26.94, and 11.75% are probabilities of shifting to Regime 1, 2, and 3, respectively, as well.
Thus, these figures show that there is a high and low probability of switching between Regimes 1 and 3. This means that these regimes are highly and lowly volatile, which further confirms that the water exchange traded funds with the water sector is characterized by more regime stability compared to the funds relationship with the equity market (Roca, Tularam, & Wong, Citation2011).
A graphical representation of the regime probabilities is presented in Figures –.
4.5. Idiosyncratic risk
From Table , it can be seen that idiosyncratic risk for the four ETFs through the three regimes; i.e. SIGMA (σ) for regressions across regimes from the Markov switching model output. The SIGMA (σ) of these ETFs indicates that the movements of ETFs’ returns are in a same direction to their benchmarks over one day. The idiosyncratic risk for most of the exchange traded funds move from low volatility (Regime 2) to very low volatility (Regime 1 and 3). In other words, SIGMA (σ) seems to be assessed in low volatility water exchange traded funds. This result shows that as idiosyncratic risk is not constant during the three regimes for Markov switching heteroscedasticity (MSH), and that the water ETFs have little influence on the idiosyncratic risk. Water ETFs in the same way affect the total risk. Now this question arises: why water ETFs’ idiosyncratic risk is so lowly regime dependent. However, it is noted that Water ETFs with decreasing competitive stress have to shift some of their attention to investors. Further studies are needed to investigate the systematic risk for the financial performance of ETFs and their corresponding benchmark indices during different regimes because of the low volatility of four ETFs.
Table 4. Estimated idiosyncratic risk
4.6. The relationship between idiosyncratic risk and return
From Table it can be seen that during Regime 1, the beta coefficients of four water ETFs are found to be positive. The Three ETFs (PHO, PIO, and FIW) are significant at the 1% level of significance. The beta coefficients are all positive for the four water ETFs at Regime 2 and Regime 3, respectively. All ETFs are significant at the 1% level of significance during Regime 2. However, three ETFs (PHO, FIW, and GGW) are significant at the 1% level of significance during Regime 3. Overall, the results show that water ETFs’ beta coefficients’ entire values are less than 1, which implies that water investment has a lower idiosyncratic risk which coincide with Angelidis and Tessaromatis (Citation2009) and most of the beta coefficients are positive and significant at Regime 1, Regime 2, and Regime 3, respectively. The results indicate that idiosyncratic risk has a positive effect on the water ETFs returns during different regimes. Based on the time series data, as the beta coefficients are significant at the 1% level of significance, the results indicate that idiosyncratic risk has foretelling influence for the forthcoming returns. It is noted that the results of idiosyncratic risk and return are not constant during different regimes. Thus, Markov switching model involving time series analysis was suitable for this study.
Table 5. Empirical coefficients of unsystematic risk
5. Conclusion
The objective of this article was to investigate the idiosyncratic risk and return of the water investment. In particular, we examine the time-varying transition probabilities using the Markov switching model. The study utilizes daily returns of four water ETFs from the data-stream database based on the time series data (period 15 June 2004–31 August 2015). In so doing, the study has taken into account of the regime effects.
In this paper, the ADF and PP testing results show that PHO, FIW, and GGW at 1%; and PIO at 5% level of significance. Both unit root tests confirm that the ADF and PP tests consistently reject the null hypothesis and the funds’ returns those of stocks are stationary.
The transition probabilities show that there is a high and low probability of switching between Regimes 1 and 3, respectively. The transition probabilities show that three regimes are both highly and lowly volatile. The Markov switching model results show that the idiosyncratic risk of the exchange traded funds (ETFs) are not constant across the three regimes and that the water ETFs appear to have little influence on the idiosyncratic risk. Moreover, the “standard error” terms for regressions across regimes outputs are rather low. In a similar manner, water ETFs affect the total risk. We also identify that the beta coefficients are positive and entire values are less than 1 at Regime 1, Regime 2, and Regime 3, respectively. It seems that water investment has a lower systematic risk and a positive effect on the water ETFs returns during different regimes. Thus, as the Markov switching model is changing when the regime either falls or rises, higher idiosyncratic risk of different regimes illuminating greater returns of water ETFs accordingly.
Due to the under diversification of the water investment form, the idiosyncratic risk of the water investment can play an important role in terms of the total risk and it can allow changes across three regimes. This study has important implication for the water investors and international institutions in decision-making need to include water investment in portfolio. Further, this research study can be extended along with a complementary investigation on the financial performance of the water ETFs’ and their corresponding benchmark indices during different stock market regimes.
Additional information
Funding
Notes on contributors
Gurudeo Anand Tularam
Gurudeo Anand Tularam is a senior lecturer in Mathematics/Statistics in the Griffith Sciences-Science, Environment, Engineering and Technology, and a senior researcher at the Environmental Futures Institute, Griffith University, Australia. His research expertise in time series and stochastic calculus methods in business and finance applications such as investment, portfolio analysis, and risk assessment. He has published more than hundred papers in reputed international journals.
Rajibur Reza
Rajibur Reza is a PhD candidate at the Department of Accounting, Finance and Economics, Griffith Business School, Griffith University, Australia.
References
- Aaker, D. A. & Jacobson, R. (1987). The role of risk in explaining differences in profitability. Academy of Management Journal, 30, 277–296.10.2307/256274
- Angelidis, T., & Tessaromatis, N. (2009). Idiosyncratic risk matters! A regime switching approach. International Review of Economics and Finance, 18, 132–141.10.1016/j.iref.2007.09.003
- Antoniou, A., Barr, D. G., & Priestley, R. (2000). Abnormal stock returns and public policy: The case of the UK privatised electricity and water utilities. International Journal of Finance and Economics, 5, 93–106.10.1002/(ISSN)1099-1158
- Atkinson, W. (2009). Water investing. Equities, 58, 32–33.
- Bali, T., & Cakici, N. (2008). Idiosyncratic volatility and the cross section of expected returns. Journal of Financial and Quantitative Analysis, 43, 29–58.10.1017/S002210900000274X
- Bali, T. G., Cakici, N., Yan, X., & Zhang, Z. (2005). Does idiosyncratic risk really matter? The Journal of Finance, 60, 905–929.10.1111/jofi.2005.60.issue-2
- Barber, J. R. (1994). Mutual fund risk measurement and future returns. Quarterly Journal of Business and Economics, 33, 55–64.
- Bollen, B., A., Skotnicki, A., & Veeraraghavan, M. (2009). Idiosyncratic volatility and security returns: Australian evidence. Applied Financial Economics, 19, 573–1579. doi:10.1080/09603100902984327
- Buckland, R., & Fraser, P. (2000). Abnormal returns in the UK water industry since privatisation (Working Paper No. 00-10). Aberdeen Papers in Accountancy, Finance and Management. University of Aberdeen.
- Buckland, R., & Fraser, P. (2001). Political and regulatory risk in water utilities: Beta sensitivity in the United Kingdom. Journal of Business Finance and Accounting, 28, 877–904.10.1111/jbfa.2001.28.issue-7&8
- Buckland, R., & Williams, J. (2013). Volatility and regulation in water supply and distribution: A post-privatization comparison of UK and US water utilities. Business School, University of Aberdeen, and Aberdeen University. Research Archive (AURA). Retrieved July 26, 2014, from http://aura.abdn.ac.uk/bitstream/2164/2968/1/WaterUtilityPaperforAURA.pdf
- Calice, G., Ioannidis, C., & Miao, R. H. (2012). A Markov switching unobserved component analysis of the CDX index term premium. Mimeo: University of Southampton.
- Calice, G., Mio, R., Štěrba, F., & Vašíček, B. (2015). Short-term determinants of the idiosyncratic sovereign risk premium: A regime-dependent analysis for European credit default swaps. Journal of Empirical Finance, 33, 174–189.10.1016/j.jempfin.2015.03.018
- Campbell, J., Lettau, M., Malkiel, B., & Xu, Y. (2001). Have individual stocks become more volatile? An empirical exploration of idiosyncratic risk. The Journal of Finance, 56, 1–43.10.1111/jofi.2001.56.issue-1
- Cheung, Y. L., & Wong, K. T. (1992). An assessment of risk and return: Some empirical findings from the Hong Kong stock exchange. Applied Financial Economics, 2, 105–114. doi:10.1080/758536014
- Cox, L. A., & Griepentrog, G. L. (1988). Systematic risk, unsystematic risk, and property-liability rate regulation. The Journal of Risk and Insurance, 55, 606–627.10.2307/253140
- Garcia, R. (1998). Asymptotic null distribution of the likelihood ratio test in Markov switching models. International Economic Review, 39, 763–788.10.2307/2527399
- Geman, H., & Kanyinda, A. (2007). Water as the next commodity. The Journal of Alternative Investments, 10, 23–30.10.3905/jai.2007.695264
- Gilroy, B. M., Schreckenberg, H., & Seiler, V. (2013). Water as an alternative asset. (Working Paper No. 2012-09). Center for International Economic, Universität Paderborn. Retrieved March 16, 2014, from http://groups.unipaderborn.de/fiwi/RePEc/Working%20Paper%20neutral/WP55%20-%202012-09.pdf
- Goldfeld, S. M., & Quandt, R. E. (1973). A Markov model for switching regressions. Journal of Econometrics, 1, 3–15.10.1016/0304-4076(73)90002-X
- Goyal, A., & Santa-Clara, P. (2003). Idiosyncratic risk matters! The Journal of Finance, 58, 975–1007.10.1111/jofi.2003.58.issue-3
- Guidolin, M., & Hyde, S. (2009). What tames the Celtic tiger? Portfolio implications from a multivariate Markov Switching model. Applied Financial Economics, 19, 463.
- Guo, H., & Savickas, R. (2003). Does idiosyncratic risk matter: Another look (Working Paper). Federal Reserve Bank of St. Louis.
- Hamilton, J. D. (1989). A new approach to the economic analysis of nonstationary time series and the business cycle. Econometrica, 57, 357–384.10.2307/1912559
- Huimin, L., Cheung, A., & Roca, E. (2010). Yes, indeed, idiosyncratic risk matters for socially responsible investments! International Research Journal of Finance and Economics, 54, 63–74.
- Jin, Y., Li, B., Roca, E., &, Wong, V. (2015b). Water as an investment: Liquid yet illiquid. Applied Economics, 731–745. doi:10.1080/00036846.2015.1085646
- Jin, Y., Roca, E., Li, B., Wong, V., & Cheung, A. (2015a). Sprinkle your investment portfolio with water! International Journal of Water, 9, 43–59.10.1504/IJW.2015.067445
- Kearney, H. (2008). Water, water, everywhere—But investors aren’t in synch. On Wall Street, 18, 20.
- Krolzig, H.-M. (1997). Markov switching vector autoregressions. Modelling, statistical inference and application to business cycle analysis. Berlin: Springer.
- Morana, C., & Sawkins, J. W. (2000). Regulatory uncertainty and share price volatility: The English and Welsh water industry’s periodic price review. Journal of Regulatory Economics, 17, 87–100.10.1023/A:1008105405621
- Organisation for Economic Co-operation and Development. (2008). OECD environmental outlook to 2030. Paris: Author.
- Organisation for Economic Co-operation and Development. (2009). Private sector participation in water infrastructure: OECD checklist for public action. Paris: Author.
- Quandt, R. E. (1958). The estimation of the parameters of a linear regression system obeying two separate regimes. Journal of the American Statistical Association, 53, 873–880.10.1080/01621459.1958.10501484
- Roca, E. D., & Tularam, G. A. (2012). Which way does water flow? An econometric analysis of the global price integration of water stocks. Applied Economics, 44, 2935–2944. doi:10.1080/00036846.2011.568403
- Roca, E., Tularam, G. A., & Reza, R. (2015). Fundamental signals of investment profitability in the global water industry. International Journal of Water, 9, 395–424.10.1504/IJW.2015.072155
- Roca, E. D., Tularam, G. A., & Wong, V. S. H. (2011). Markov regime switching modelling and analysis of socially responsible investment funds. Journal of Mathematics and Statistics, 7, 302–313.
- Roca, E. D., & Wong, V. S. H. (2008). An analysis of the sensitivity of Australian superannuation funds to market movements: A Markov regime switching approach. Applied Financial Economics, 18, 583–597. doi:10.1080/09603100601118292
- Tang, Y. N. G., & Shum, W. C. (2007). The risk-return relations: Evidence from the Korean and Taiwan stock markets. Applied Economics, 39, 1905.
- Tularam, G. A. & Keeler, H. P. (2006). The study of coastal groundwater depth and salinity variation using time-series analysis. Environmental Impact Assessment Review, 26, 633–642.
- Tularam, G. A. (2014). Investing in water for a green economy. Australian Planner, 51, 372–373. doi:10.1080/07293682.2014.948987
- Tularam, G. A., & Illahee, M. (2008). Exponential smoothing method of base flow separation and its impact on continuous loss estimates. American Journal of Environmental Sciences, 4, 136–144.
- Tularam, G. A., & Illahee, M. (2010). Time series analysis of rainfall and temperature interactions in coastal catchments. Journal of Mathematics and Statistics, 6, 372–380.10.3844/jmssp.2010.372.380
- Tularam, G. A., & Krishna, M. (2009). Long term consequences of groundwater pumping in Australia: A review of impacts around the globe. Journal of Applied Sciences in Environmental Sanitation, 151–166.
- Tularam, G. A., & Marchisella, P. (2014). Securing water and wastewater systems. International Practices for Protecting Water and Wastewater Infrastructure. Springer.10.1007/978-3-319-01092-2
- Tularam, G. A., & Properjohn, M. (2011). An investigation into water distribution network security: Risk and implications. Security Journal, 4, 1057–1066.
- Tularam, G. A., & Singh, R. (2009). Estuary, river and surrounding groundwater quality deterioration associated with tidal intrusion. Journal of Applied Sciences in Environmental Sanitation, 4, 141–150.
- Wei, S, & Zhang, C. (2005). Idiosyncratic risk does not matter: A re-examination of the relationship between average returns and average volatilities. Journal of Banking and Finance, 29, 603–621.10.1016/S0378-4266(04)00050-0
- Wild, D., Francke, C.J., Menzli, P., & Schon, U. (2007). Water: A market of the future. Zurich: SAM Sustainable Asset Management. Retrieved June 20, 2014, from http://www.bhopal.net/petition/application/views/waterstudy_e.pdf
- Wong, V., Roca, E., & Tularam, G.A. (2007). Socially responsible investments: Do they make a difference to the systematic risk of Australian superannuation funds? 15th Annual Conference on Pacific Basin Finance, Economics, Accounting and Management, Rutgers University.
Bibliography
- Roca, E., Wong, V., & Tularam, G. A. (2010). Are socially responsible investment markets worldwide integrated? Accounting Research Journal, 23, 281–301. Retrieved from http://www.emeraldinsight.com/journals.htm?articleid=1896180&show=abstract
- Tularam, G. A., & Hassan, O. M. ( in press). Water availability and food security—Impact on people’s movement and migration in sub-Saharan Africa (SSA). NY: Taylor and Francis International.
- Tularam G. A. (in press). Water security problems in Asia and longer term implications for Australia Springer. Retrieved from http://link.springer.com/chapter/10.1007/978-3-319-12394-3_7
- Tularam, G. A., & Ilahee, M. (2011). Time series analyses of rainfall and temperature interactions in tropical catchments. In A. B. Nadykto, L. Uvarova, A. V. Latyshev (Ed.), Mathematical models of non-linear phenomena, processes and systems: From molecular scale to planetary atmosphere (400 p.). Albany, NY: State University of New York at Albany, Atmospheric Sciences Research Center. ISBN: 978-1-60876-994-0.
- Tularam, G.A. (2010). Relationship between El Niño southern oscillation index and rainfall (Queensland, Australia). International Journal of Sustainable Development and Planning, 5, 379–391. Retrieved from http://journals.witpress.com/paperinfo.asp?pid=475
- Tularam, G. A., & Ilahee, M. (2007). Environmental concerns of desalinating seawater using reverse osmosis, Journal of Environmental Monitoring, 9, 805–813. Retrieved from http://pubs.rsc.org/en/Content/ArticleLanding/2007/EM/b708455m
- Tularam, G. A., & Ilahee, M. (2007). Initial loss estimates for tropical catchments of Australia, Environmental Impact Assessment Review, 27, 493–504. Retrieved from http://www.sciencedirect.com/science/article/pii/S0195925506001521
- Retrieved from http://lifewater.org/articles-category/nutrition-children-wash/?gclid=CNSxzaK50MgCFQNxvAod9eMOOQ
- Retrieved from http://www.un.org/waterforlifedecade/food_security.shtml