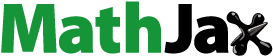
Abstract
This study employed the Cobb–Douglas stochastic frontier production function to measure the level of technical efficiency among smallholder cassava farmers in Central Madagascar. A multi-stage random sampling technique was used to select 180 cassava farmers in the region and from this sample, input–output data were obtained using the cost route approach. The parameters of the stochastic frontier production function were estimated using the maximum likelihood method. The results of the analysis showed that individual farm-level technical efficiency was about 79%. The study found education, gender and age to be indirectly and significantly related to technical efficiency at a 1% level of probability, and to household size at a 5% level. The coefficient for occupational status was positive and highly significant at a 1% level. The results show that the study’s cassava farmers are not fully technically efficient, showing a mean score of .79%, and suggesting that opportunities still exist for increasing efficiency among the farmers. There is a need, therefore, to ensure that these farmers have access to the appropriate inputs, especially land and capital. The results also call for land reform policies to be introduced, aimed at making more land available, especially to the younger and full-time female farmers.
Keywords:
Public Interest Statement
The results of this study reveal that technical efficiency in cassava production in Central Madagascar is relatively high. Although individual levels of technical efficiency range between 24 and 94% with a mean of 79%, it suggests that opportunities still exist for increased productivity of cassava farmers in the zone by increasing the efficiency with which resources are used at the farm level. Important factors indirectly related to technical efficiency are age, education, gender, household size and occupational status. Occupational status had a direct relationship with technical efficiency. The agricultural labour force is female dominated but ageing drastically with educated farmers diverting skills to non-farm livelihoods. Women play a significant role in cassava production in the study area. Therefore, policies designed to improve women access to land, fertilizer, credit, agricultural extension, new technologies and more education will be crucial in increasing technical efficiency.
1. Introduction
Cassava (Manihot esculenta Crantz) is an important staple food and cash crop in several tropical African countries, where it plays a principal role in the food economy (Agwu & Anyaeche, Citation2007). Madagascar ranks 19th in terms of the production of cassava worldwide, with a share of 1.10% of the market, based on a total production figure of 3.11 m metric tonnes. The average farm size in the country is 4,750 ha and the average productivity, 6.55 t/ha (FAO Statistics, Citation2013).
Over 500 million people live on cassava throughout the world, eating its roots or tubers due to its high energy content, and also its leaves which are an abundant source of protein and both vitamins A and B (Kormawa, Tshiunza, Dixon, Udoh, & Okoruwa, Citation2001 and Tchabana, Citation2002). Cassava has the potential to become a key cash crop in many African countries (Qirschot, Ngendello, & Westby, Citation2004). In Madagascar, cassava is cultivated in virtually all regions; however, production levels are poor, taking into account the high demand for the product at local markets and the requirements of a variety of processing industries.
Cassava could be a powerful weapon in the fight against poverty in Africa, as the cash income derived from the crop is more egalitarian than other major staple crops due to its low cash input levels (Nweke, Citation2004). Compared to other major staples, cassava performs well across a wide range of ecological systems, and as a result, can benefit a broader range of farmers, those living across different zones. Cassava is also less expensive to grow, as it tolerates poor soil, adverse weather conditions and pests and diseases, more than other major staples (Nweke, Citation2004). The crop generates an income and provides food for the most vulnerable segments of Madagascan society. Cassava stores its harvestable portion underground until it is needed; therefore, it is also a classic food security crop (Asogwa, Umeh, & Ater, Citation2006).
Technical efficiency refers to the ability of firms to employ the best practices in their production processes, so that not more than the necessary amount of a given set of inputs is used in producing the “best” level of output (Carlsson, Citation1972). Olayide and Heady (Citation1982) defined technical efficiency as the measure of a firm’s success in producing maximum output from a given set of inputs.
Farm efficiency, and the question of how to measure it, is an important subject within the agricultural sectors of developing countries (Hazarika & Subramanian, Citation1999; Shah, Citation1995). There are four major approaches to the measurement of efficiency (Coelli, Rao, & Battese, Citation1998): the non-parametric programming approach (Charnes, Cooper, & Rhodes, Citation1978), the parametric programming approach (Aigner & Chu, Citation1968; Ali & Chaudhry, Citation1990), the deterministic statistical approach (Afriat, Citation1972; Fleming, Fleming, Rodgers, Griffiten, & Johnston, Citation2004; Schippers, Citation2000) and the stochastic frontier approach (Aigner, Lovell, & Schmidt, Citation1977; Kirkley, Squires, & Strand, Citation1995). Among these, the stochastic frontier and non-parametric programming techniques known as data envelopment analysis (DEA) are the most popular approaches. The stochastic frontier approach is preferred when assessing agricultural efficiency because of the inherent stochasticity involved (Coelli, Citation1994; Ezeh, Citation2004).
Frontier efficiency studies over the last three decades have received attention among researchers and policy analysts, as evidenced by the proliferation of the methodology and its application across the globe (Thiam, Bravo-Ureta, & Rivas, Citation2001). Recent empirical findings by Thiam et al. (Citation2001), Bravo-Ureta et al. (Citation2007) and Ogundari and Brümmer (Citation2011) have shown that mean efficiency estimates of agricultural production reported in the primary studies vary across study attributes (or dimensions) such as methodology, data type, model specification and location. Given this, it will be interesting to understand what the literature reveals about the trends in agricultural efficiency levels in Africa and what drives these levels of efficiency over time. Such an understanding will provide an important input to agricultural policy decisions in the region
Given the various cassava programmes and policies implemented over the years to raise farmers’ efficiency and productivity, it has become imperative to empirically analyse the relationship between technical efficiency and socio-economic variables among cassava farmers. Such an analysis will further guide policy-makers in developing policies aimed at improving the welfare of cassava farmers, and such an improvement will give them the potential to expand their cassava production activities. Thus, the broad objective of this study is to analyse the technical efficiency of Malagasy cassava farmers, so as to guide food security policy developments.
2. Methodology
2.1. The study area
Agriculture in Madagascar is heavily influenced by rainfall, which is generally abundant along the east coast, but decreases sharply across the highlands, falling to less than 500 mm per year in the south and south-west. The growing season starts with the first rains in October or November. The cropping calendar varies a lot from region to region according to the different climatic conditions, soils and altitudes. Food crop production is the most important agricultural sub-sector, accounting for around 75% of the cultivated area. Rice is the most common staple food grown, covering as it does 1.34 million hectares throughout the country—with the exception of some semi-arid areas in the south and south-west which use rain-fed and irrigated systems. Other food crops grown include maize (mainly grown in the south and central-east regions), cassava, sorghum (in the south), beans, groundnut, sweet potatoes and a wide variety of vegetables. Nonetheless, the risk-coping strategies used by farmers in the country, including the use of cassava as an energy-giving food, are insufficient to make the farmers food secure (Parikh & Shah, Citation1995; Ramaroson Rakotosamimanana, Arvisenet, & Valentin, Citation2014), and cassava farmers—similar to other farmers—suffer significant losses due to cyclones.
2.2. Sampling procedure
This participatory research study involved 180 farmers living across 12 rural communes selected from 6 districts: Moramanga, Betafo, Ambalavao, Soavinandriana, Analamanga and Vakinankara. The districts are located along the western coast, near to the Mozambique Channel, and along the transverse section of the country, through the central region and over to the eastern coast (Figure ).
A multi-stage random sampling technique was used for the study. Primary data were collected with the aid of a well-structured questionnaire. Cassava collectors and agriculture service agents were interviewed and yield measurements were taken from the farmers’ fields.
2.3. Specification of the stochastic frontier
A stochastic frontier production function is defined by:(1)
(1)
where Yi—output of the ith farm, Xi—vector of input quantities used by the ith farm, β—vector of unknown parameters to be estimated, f()—an appropriate function (e.g. Cobb–Douglas, translog, etc.), Vi—symmetric error, which accounts for random variations in output due to factors beyond the control of the farmer, e.g. weather, disease outbreaks and measurements errors and Ui—a non-negative random variable representing inefficiency in production relative to the stochastic frontier. The random error Vi is assumed to be independently and identically distributed as N(o, σv2) random variables independent of the Uis, which are assumed to be a non-negative truncation of the N(o,σu2) distribution (i.e. half-normal distribution) or have an exponential distribution.
This stochastic frontier model was independently proposed by Aigner et al. (Citation1977) and Meeusen and Van den Broeck (Citation1977). The major advantage of this method is that it provides numerical measures of technical efficiency. The technical efficiency of an individual farmer is defined in terms of the ratio of the observed output to the corresponding frontier output, given the available technology.
Technical efficiency of production for the ith farm at the tth observation is defined by equation (Equation2(2)
(2) ), following Battese and Coelli (Citation1995)
(2)
(2)
where Yi—Observed output and Yi*—Frontier output.
The parameters of the stochastic frontier production function are estimated using the maximum likelihood method. The prediction of the technical efficiencies is based on its conditional expectation, given the model assumptions. This result is also given in the appendix of Battese and Coelli (Citation1993).
2.4. Analytical framework
For this study, the production technology of cassava farmers in Central Madagascar is assumed to be specified by the Cobb–Douglas frontier production function, which is defined as follows:(3)
(3)
where Y—cassava output in kg, X1—labour (man days), X2—organic manure (kg), X3—Planting materials (bundles), X4—farm size (ha), X5—capital input in Ariari made up of depreciation charges on farm tools and equipment, interest on borrowed capital and rent on land, b0, b1, … b5 are estimated regression parameters, while vi and ui are as defined earlier.
In addition, ui is assumed in this study to follow a half-normal distribution, as is the case in most frontier production literature.
2.5. Determinants of technical efficiency
Identifying the determinants of efficiency is a major task within efficiency analysis. In order to determine factors contributing to the observed technical efficiency of cassava production in the study area, the following model was formulated and estimated jointly with the stochastic frontier model in a single-stage maximum likelihood estimation procedure, and using the computer software Frontier Version 4.1 (Coelli, Citation1996).(4)
(4)
where TEi—technical efficiency of the ith farmer, Z1—gender (dummy variable; 1 = male, 0 = female), Z2—age (years), Z3—educational level (years), Z4—occupational status (dummy variable; 1 = full-time farmer, 0 = part-time farmer), Z5—household size, Z6—membership of farmer organizations (dummy variable; 1 = yes, 0 = no), Z7—farm size (ha), Z8—farming experience (years) and a0, a1, a2, …. a8 are estimated regression parameters.
We expect a3, a4, a5, a6, a7 and a8 to be positive, and a1 and a2 to be negative.
3. Results and discussion
3.1. Average statistics for cassava farmers in Madagascar
The averaged statistics for the sample cassava farmers are presented in Table . On average, a typical cassava farmer in Madagascar is 46.05 years old, spends 6 years in education and has 12 years farming experience. The average household size for such farmers is six people. The average cassava farmer cultivates .27 ha, uses about 268.89 kg of organic fertilizer and generates an output of about 2.7t of cassava. Table also shows that an average cassava farmer in the region employs 420.03 mandays of labour, and has 13 years farming experience. Cassava production in the region is dominated by the use of female labour (56.05), the majority (73.88%) of whom work full-time. Only 16.32% of farmers have membership of farmer organizations.
Table 1. Statistics for Cassava Farmers in Central Madagascar
3.2. Estimated production function
The maximum likelihood estimates using Cobb–Douglas stochastic frontier parameters for cassava are presented in Table . The coefficients for labour and organic fertilizer were negative and significant at 1 and 5% levels of probability, respectively. Planting materials was significant at a 10% level. These results imply that that any increase in labour, organic fertilizer and planting material would decrease output by .9748, .1515 and .9163%, respectively. This is in contrast to a priori expectations, probably due to the inefficient use of these inputs. The coefficients for farm size and capital inputs were positive and significant at a 1% level of probability. This implies that any increase in farm size and capital inputs would increase output by .4726 and .3576%, respectively. This is expected and in accordance with a priori expectations.
Table 2. Estimated Cobb–Douglas Stochastic Frontier Production Function for Smallholder Cassava Farmers in Central Madagascar
The estimated variance (σ2) was statistically significant at a 1% level, indicating goodness of fit with the Cobb–Douglas Stochastic Frontier Model. The gamma (γ) was .5501 and significant at 1% level, indicating that only 55.01% of total variation in cassava output was due to technical inefficiency.
3.3. Sources of technical efficiency
The estimated determinants for the technical efficiency of cassava production activities are presented in Table . The coefficient for gender was negative and highly significant at a 1% level of probability. This implies that female cassava farmers are more technically efficient than their male counterparts, in line with the findings of Quisumbing (Citation1996). However, women may be constrained by cultural factors from playing a more active economic role, plus they tend to have a lower level of education and technical development, resulting in lower input levels on the plots they farmed. This results in lower yields and casts doubt on Pareto efficiency assumptions (Adam, Wallace, & Scot, Citation2003).
The coefficient for age was also found to be negative and highly significant at a 1% level. This implies that an increase in age leads to a decrease in technical efficiency; that younger farmers are more technically efficient than their more elderly counterparts. This is because older farmers are less energetic, leading to lower productivity and lower technical efficiency levels. This result is in line with the findings of Mathijs and Vranken (Citation2000), Bozoğlu and Ceyhan (Citation2007), Ajibefun and Daramola (Citation2003) and Ajibefun and Aderinola (Citation2004).
The coefficient for education level was negative and highly significant to a 1% level. This shows that uneducated farmers are more technically efficient than their educated counterparts. This is not expected and is in contrast to the a priori expectation. The reason for this unexpected outcome is probably that the more educated farmers tend to work part-time on their farmers and have other livelihood options. Simonyan, Umoren and Okoye (Citation2011) suggested that for women, there is a strong competing effect in terms of diverting skills to off-farm employment opportunities as their education level increases.
The coefficient for occupational status was positive and highly significant at a 1% level. This implies that the full-time farmers are more technically efficient than their part-time counterparts. The productive effects of having an off-farm income (part-time farmers) are difficult to explain theoretically, but having off-farm incomes could imply that less time is spent on the farm, and so resources are used less efficiently (Tipi, Yildiz, Nargeleçekenler, & Çetin, Citation2009).
The coefficient for household size was negative and significant at a 5% level, indicating that large households will have lower technical efficiency levels. This is in contrast with a priori expectations, probably because with large households, a farmer may have to divert revenue away from farm activities towards looking after the children.
The coefficients for membership of farmer organizations and farm size were negative, but not significant. The coefficient for farming experience was positive but also not significant.
The frequency distributions for technical efficiency are presented in Table . Individual technical efficiency indices ranged from 24% to 94%, with a mean of 79%. In total, 35.56% of the cassava farmers were found to have technical efficiency indices greater than 80%. These technical efficiency levels are consistent with the low variance in farm effects found.
Table 3. Frequency Distribution and Technical Efficiency Indices
The estimates also show that if an average cassava farmer were to become the most technically efficient farmer in the sample, he or she would experience cost savings of 22.34% (1 − .79/.94). The least technically efficient farmers would also have to increase efficiency by 80.85% (1 − .24/.94).
4. Conclusion
This study has analysed the determinants of technical efficiency among smallholder cassava farmers in Central Madagascar. The results show that these cassava farmers are not fully technically efficient. Individual levels of technical efficiency were found to be in the range .24–.94%, with a mean of .79%, suggesting that opportunities still exist for cassava farmers in the country to increase their efficiency levels by improving the ways in which resources are used at the farm level. The study found that the most important factors indirectly related to technical efficiency levels are age, education, gender, household size and occupational status. Occupational status in particular has a direct relationship with technical efficiency. These results call for policies to be introduced aimed at encouraging young people who are agile and stronger than their older counterparts to enter the cassava-growing sector. The study also found that technical efficiency levels could be further increased by improving cassava farmers’ access to inputs such as land and capital, especially among female, full-time farmers.
Corrigendum
This article was originally published with errors. This version has been corrected. Please see Corrigendum (http://dx.doi.org/10.1080/23322039.2016.1169640).
Additional information
Funding
Notes on contributors
B.C. Okoye
B.C. Okoye is an agricultural economist and system analyst. His research programme spanned issues such as food security, production economics, farm management, agricultural marketing, gender productivity and efficiency, environmental and resource economics, adoption studies and applied micro-economics and econometrics.
A. Abass
A. Abass is a food technologist and post-harvest specialist. He has worked as a coordinator–Cassava Value Chain, and Leader of Small-Scale Cassava Processing Project in East and Southern Africa. His research activities have focused on generating positive impacts on smallholders’ livelihoods in West, East and Southern Africa by helping cassava value chain actors to commercialize through increased mechanization levels within the cassava processing sector, and enhanced utilization of the crop for food and in the industry. His latest research activities are focused on enhancing the competitiveness of high-quality cassava flour (HQCF) and developing post-harvest loss prevention strategies for maize and fresh cassava roots.
References
- Adam, Z. C., Wallace, E. H., Scot, R. (2003). Technical efficiency of modern grains production on Chinese farms. A stochastic production frontier approach. Paper prepared for The Human Resource and Labour Economics Workshop. Ames, IA: Department of Economics, Iowa State University.
- Afriat, S. N. (1972). Efficiency estimation of production functions. International Economic Review, 13, 568–598.10.2307/2525845
- Agwu, A. E. & Anyaeche, C. L. (2007). Adoption of improved cassava varieties in six rural communities of Anambra state, Nigeria. African Journal of Biotechnology., 6, 89–98.
- Aigner, D. J., & Chu, S. F. (1968). Estimating the industry production function. American Economic Review, 58, 826–839.
- Aigner, D. J., Lovell, C. A. K., & Schmidt, P. (1977). Formulation and estimation of stochastic frontier production function models. Journal of Econometrics, 6, 21–37.10.1016/0304-4076(77)90052-5
- Ajibefun, I. A., & Aderinola, E. A. (2004). Determinants of technical efficiency and policy implication in traditional agricultural production: Empirical study of Nigerian food crop farmers. Final Report Presentation at Bi-annual Research Workshop of African Economic Research Consortium, Nairobi, Kenya.
- Ajibefun, I. A., & Daramola, A. G. (2003). Efficiency of micro enterprises in the Nigerian economy. AERC Research paper 134. African Economic Research Consortium, Nairobi, 7, 1–42.
- Ali, M., & Chaudhry, M. A. (1990). Inter-regional farm efficiency in Pakistan’s Punjab: A frontier production function study. Journal of Agricultural Economics, 41, 62–74.10.1111/jage.1990.41.issue-1
- Asogwa, B. C., Umeh, J. C., & Ater, P. I. (2006, August 12–18). Technical efficiency analysis of Nigerian cassava farmers: A guide for food security policy. Poster paper prepared for presentation at the International Association of Agricultural Economists Conference, Gold Coast, Australia.
- Battese, G. E., & Coelli, T. J. (1993). A stochastic frontier production function incorporating a model for technical inefficiency effects ( Working Papers in Econometrics and Applied Statistics No 69). Armidale: Department of Econometrics, University of New England.
- Battese, G. E., & Coelli, T. J. (1995). A model for technical inefficiency effects in a stochastic frontier production function for panel data. Empirical Economics, 20, 325–332.10.1007/BF01205442
- Bozoğlu, M., & Ceyhan, V. (2007). Measuring the technical efficiency and exploring the inefficiency determinants of vegetable farms in Samsun province, Turkey. Agricultural Systems, 94, 649–656.10.1016/j.agsy.2007.01.007
- Bravo-Ureta, B. E., Solís, D., Moreira López, V. H., Maripani, J. F., Thiam, A., & Rivas, T. (2007). Technical efficiency in farming: A meta-regression analysis. Journal of Productivity Analysis, 27, 57–72.10.1007/s11123-006-0025-3
- Carlsson, B. (1972). The measurement of efficiency in production: An application to Swedish manufacturing industries 1968. The Swedish Journal of Economics, 74, 468–485.10.2307/3439287
- Charnes, A., Cooper, W. W., & Rhodes, E. (1978). Measuring the efficiency of decision making units. European Journal of Operational Research, 2, 429–444.10.1016/0377-2217(78)90138-8
- Coelli, T. J. (1994). A guide to Frontier 4.1: A computer program for stochastic frontier production and cost function estimation. Armidale: Department of Econometrics, University of New England, 36 pp.
- Coelli, V. J. (1996). Guide to frontier Version 4.1: A computer program for stochasticfrontier production and cost function estimation. Armidale, Australia: Department of Economics, University of New England.
- Coelli, T. J., Rao, D. S. P., & Battese, G. (1998). An introduction to efficiency and productivity analysis. Boston, MA: Kluwer Academic Press.10.1007/978-1-4615-5493-6
- Ezeh, C. I. (2004). A comparative study of Fadama and non-Fadama crop farmers in Osisioma-Ngwa L.G.A, Abia State, Nigeria. Journal of Sustainable Tropical Agriculture Research, 11, 26–31.
- FAO Statistics. (2013). Food and Agricultural Organisation, Data base results. Retrieved June, 2015, from FAO 2013.
- Fleming, E., Fleming, P., Rodgers, H., Griffiten, G., & Johnston, D. (2004). Animal efficiency in an intensive beef production. Armidale: Genetic Breeding Unit, University of New England.
- Hazarika, C., & Subramanian, S. R. (1999). Estimation of technical efficiency in the stochastic frontier production function model—An application to the tea industry in Assam. Indian Journal of Agricultural Economics, 54, 201–211.
- Kirkley, J. E., Squires, D., & Strand, I. E. (1995). Assessing technical efficiency in commercial fisheries: The Mid-Atlantic sea scallop fishery. American Journal of Agricultural Economics, 20, 31–34.
- Kormawa, P., Tshiunza, M., Dixon, A., Udoh, E, & Okoruwa, V. (2001). Varietal characteristics of cassava: Farmers’ perceptions and preferences in semi-arid zone of West Africa. Proceedings of the 8th Triennial Symposium of the International Society for Tropical Root Crops-Africa Branch (ISTRC-AB), IITA, Ibadan.
- Mathijs, E., & Vranken, L. (2000, July 30–August 2). Farm restructuring efficiency in Transitionj: Evidence from Bulgarian and Hungary. Selected Paper, American Agricultural Association Annual meeting, Tampa, FL.
- Meeusen, N., & Van den Broeck, J. (1977). Efficiency estimation from Cobb Douglas production function with composite error. International Economic Review, 18(2), 123–134.
- Nweke, F. (2004, June). New challenges in the cassava transformation in Nigeria and Ghana (Environment and Production Technology Division (EPTD) Discussion Paper No. 118, 118 pp.). Washington, DC: International Food Policy Research Institute.
- Ogundari, K., & Brümmer, B. (2011). Technical efficiency of Nigerian agriculture: A meta-regression analysis. Outlook on Agriculture, 40, 171–180.10.5367/oa.2011.0038
- Olayide, S. O., & Heady, E. O. (1982). Introduction to agricultural production economics (pp. 15–215). Ibadan: University Press, University of Ibadan.
- Parikh, F. A., & Shah, M. K. (1995). Measurement of economic efficiency in Pakistani Agriculture. American Journal of Agricultural Economics, 77, 675–685.
- Qirschot, Q., Ngendello, T., & Westby, A. (2004). Improving cassava processing for the market from field to market. LEISA. Magazine on Low External Input and Sustainable Agriculture., 20, 24–26.
- Quisumbing, A. R. (1996). Male–female differences in agricultural productivity: Methodological issues and empirical evidence. World Development, 24, 1579–1595.10.1016/0305-750X(96)00059-9
- Ramaroson Rakotosamimanana, V., Arvisenet, G., & Valentin, D. (2014). Studying the nutritional beliefs and food practices of Malagasy school children parents. A contribution to the understanding of malnutrition in Madagascar. Appetite, 81, 67–75.10.1016/j.appet.2014.05.032
- Schippers, R. R. (2000). African indigenous vegetables. An overview of the cultivated species. Chathan: Natural Resources Institute/ACP-EU Technical Centre for Agricultural and Rural Cooperation.
- Shah, M. (1995). Measurement of economic efficiency in Pakistani agriculture. American Journal of Agricultural Economics, 1–12.
- Simonyan, J. B., Umoren, B. D., & Okoye, B. C. (2011). Gender differentials in technical efficiency among maize farmers in Essien Udim local government area, Nigeria. International Journal of Economics and Management Sciences, 1, 17–23.
- Tchabana, B. (2002). A cassava parasite un-masked. CORAF Action. Quarterly Newsletter for Research and Agricultural Development in West and Central Africa, 22, 5.
- Thiam, A., Bravo-Ureta, B. E., & Rivas, T. (2001). Technical efficiency in developing country agriculture: A meta-analysis. Agricultural Economics, 25, 235–243.10.1111/agec.2001.25.issue-2-3
- Tipi, T., Yildiz, N., Nargeleçekenler, M., & Çetin, B. (2009). Measuring the technical efficiency and determinants of efficiency of rice (Oryza sativa) farms in Marmara region, Turkey. New Zealand Journal of Crop and Horticultural Science, 37, 121–129.10.1080/01140670909510257