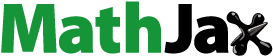
Abstract
The current standardized approach for assessing credit risk under Basel III depends on ratings assigned by credit rating agencies (CRAs). However, this approach presents three problems. First, the definitions of ratings used by CRAs to assess the likelihood of default and recovery rates are not uniform. Second, because CRAs assign ratings according to through-the-cycle ratings, their ratings are less accurate in predicting near-term defaults and react slowly to credit events. Third, CRAs have assigned ratings to few Japanese companies. To improve the standardized approach under Basel III, we propose a new method for the evaluation of credit risk without CRAs. We analyse the influence of companies’ financial and non-financial attributes on default and how a default probability model is constructed using annual reports of companies listed on the Tokyo Stock Exchange spanning fiscal 2003–2009. Results indicate that our model predicts default as accurately as CRAs.
Public Interest Statement
The standardized approach for assessing credit risk under Basel III depends on ratings assigned by credit rating agencies (CRAs). Therefore, we have constructed a new default prediction model that is independent of CRA ratings. Comparison with the historical record reveals that our model anticipates defaults as accurately as credit ratings. The findings are as following. First, our method requires only publicly available data and is accessible to any analyst familiar with routine financial information. Second, it overcomes two acknowledged deficiencies in credit ratings. Third, unlike the existing CRA models, our model pertains to companies that do not issue equity and debt and presents a way of differentiating unrated companies. Fourth, it demonstrates the importance of non-financial indicators like employee tenure for anticipating defaults. Fifth, our method can reveal firm-specific factors that lead to default and allows investors and regulators to identify firms by likelihood of default.
1. Introduction
1.1. Basel Accords and CRA ratings
In 2013, Japan initiated Basel III, the new international capital requirement rule for banks. Basel III addresses quality of capital; however, its standardized approach for measuring credit risk is little improved from Basel II, which was introduced in Japan in 2006.
The Basel Accords impose a standardized approach for assessing credit risk that involves specifying risk weights for each asset category. Basel II assigned a risk weight based on external credit ratings—that is CRA ratings—and assessed risk-weighted assets (RWA) as weighted sum of risk weights and quantity of assets. Therefore, let denote the risk weight of the asset category i,
the quantity of asset i and n the number of asset categories. RWA is then calculated as
(1)
(1)
Minimum capital requirements for credit risk are set at .
Under Basel II and III, banks can measure credit risk using the standardized approach or an internal ratings-based (IRB) approach. The latter requires banks to calculate default probabilities for each asset, thus imposing a difficult compliance burden for small and medium-sized banks. As RWA can be easily calculated using CRA ratings, 92 of Japan’s 117 banks adopt the standardized approach.Footnote1
1.2. Problems of the standardized approach
Although the standardized approach allows banks to easily calculate credit risk, Kirstein (Citation2002) argues that banks lack the incentive to reveal their results truthfully under the IRB approaches and therefore even if banks have better diagnostic skills than CRAs, the standardized approach is better measures of credit risk than the IRB approaches.
However, the standardized approach based on CRA ratings presents three problems.
First, CRAs do not follow uniform criteria for assessing default probabilities and recovery rates. Therefore, their ratings vary even for the same issuers, sometimes by more than two notches (Cantor, Citation2001). Moreover, differences in assigned ratings among Moody’s, S&P and Fitch average 18% for corporates, 15% for banks and 13% for sovereigns (Van Roy, Citation2005).
Second, CRA ratings react slowly to credit events. In general, CRAs rate corporate bond issuers using the through-the-cycle (TTC) approach. The TTC approach eliminates fluctuations in credit risk occasioned by economic cycles and assesses issuers’ medium- and long-term credit risk. Altman (Citation2011) and Löffler (Citation2013) empirically examine the characteristics of CRA ratings and indicate that ratings are stable but underperform other predictors of near-term default and react slowly to credit events. Kiff, Kisser, and Schumacher (Citation2013), simulating ratings using the TTC approach, also obtain identical results.
Third, few Japanese companies have been assigned CRA ratings, as indicated in Table , which displays the number of rated companies listed on the Tokyo Stock Exchange (TSE) on 31 August 2014. As the risk weight for debt of unrated companies is set at 100% under Basel Accords, all unrated companies are perceived to have identical credit risk.
Table 1. The number of the rated companies in TSE
Therefore, to resolve these problems, it is necessary to develop a new method of assessing credit risk without CRAs.
1.3. Purpose of this study
In this study, we construct a new default prediction model that satisfies the standardized approach under the Basel Accords but does not rely on CRA ratings. Our method is easily understood and calculated and can be universally employed using only public information and modest professional knowledge. We examine relationships between information disclosed by listed (non-financial) companies in public financial statements and historical defaults in Japan for fiscal 2003–2009. Furthermore, we compare the results from our models with CRA ratings.
The hypothesis guiding this study is that our method can predict defaults as accurately as CRA ratings and it can differentiate credit risk among unrated companies. If we are correct, our method can predict defaults of all companies issuing financial statements, and risk weights can be calculated using our ratings. It is important because all unrated companies are presumed to have equal exposure to default and our method provides investors and regulators a mechanism that overcomes this deficiency.
This study focuses only on measuring credit risk of corporate issuers; it excludes market, operational and other risks cited in the Basel Accords. In addition, we assume recovery rates to be constant, thus allowing us to measure credit risk solely by likelihood of default.
This study progresses as follows. Section 2 reviews previous literature concerning default prediction models and defaults implied by CRA ratings. Section 3 summarizes Japan’s CRAs and their rating methods. Section 4 describes our data-set, variables and estimation method. Section 5 explains our scoring and rating methods and compares our ratings and those of CRAs to the historical record. Section 6 validates the model’s reliability beyond the sampled period. Section 7 concludes our findings.
2. Literature
An extensive body of literature proposes default prediction models that employ accounting data: Altman (Citation1968) uses discriminant analysis and constructs a z-score model and Ohlson (Citation1980) uses a logit model. Default prediction studies extend Ohlson’s logit model using various explanatory variables for different periods, regions and industries. These models use only accounting data.
Black and Scholes (Citation1973) and Merton (Citation1974) propose models using option pricing frameworks. These contingent claims-based models use market data (e.g. stock prices) but not accounting data.
Campbell, Hilscher, and Szilagyi (Citation2008) and Shumway (Citation2001) use a hazard model to predict default by using market data (e.g. stock prices) and accounting data; these are hybrid models. Beaver, McNichols, and Rhie (Citation2005) explore Shumway’s (Citation2001) model and the stability of coefficients over time in the US.
Recent literature compares default predicting models: Hillegeist, Keating, Cram, and Lundstedt (Citation2004) compare the z-score model, Ohlson model and contingent claims-based models in the US; Xu and Zhang (Citation2009) do the same for Japan; Campbell et al. (Citation2008) compare hazard models and contingent claims-based models in the US; Agarwal and Taffler (Citation2008) compare z-score and contingent claims-based models but exclude hazard models in the UK; and Bauer and Agarwal (Citation2014) compare z-score, hazard and contingent claims-based models in the UK. The results of these studies indicate that the hazard model predicts default most accurately. However, as hybrid and contingent claims-based models use market data, they cannot calculate default probabilities for companies that do not issue stocks and bonds. Moreover, the cited studies focus on the respective models’ assessments of default probability but do not compare them with CRA ratings.
Studies of the predictive reliability of CRA ratings appeared during the 1960s (Horrigan, Citation1966; West Citation1970): Huang et al. (Citation2004) use support vector machines; Hajek and Michalak (Citation2013) employ feature selection procedures; Doumpos, Niklis, Zopounidis, and Andriosopoulos (Citation2015) apply multi-criteria classification to assess the predictive robustness of CRA ratings; in Japan, Katsuda and Tanaka (Citation2008) do the same using neural networks. However, these studies focus only on what variables used by CRAs to determine credit ratings, and they disregard relationships between the empirical default record and CRA ratings.
Altman and Rijken (Citation2004) examine the relationship between CRA ratings and default prediction models using accounting and market data. They observe that CRAs focus on long-term default rates. Over six years, agency rating model parameters closely match those in the default prediction model. To calculate RWA according to the standards required by the Basel Accords, it is important to predict default likelihoods beyond one year.
Hilscher and Wilson (Citation2013) compare the accuracy of the default prediction model proposed by Campbell et al. (Citation2008) and ratings by S&P using US data. They observe that default prediction model is more accurate than CRA ratings and indicate that CRA ratings are an inaccurate measure for default prediction.
To calculate RWA according to the standards required by the Basel Accords without relying on CRAs ratings, we construct a new method of predicting default after one year using financial statement data only. Our model selects default-related variables advocated in earlier studies and adds a non-financial parameter that has been absent in previous studies. Moreover, ours is the only study that uses publicly available accounting data to predict defaults and compares its accuracy against CRA ratings.
3. CRAs in Japan
Seven CRAs are registered under Japan’s Financial Services Agency (FSA): Japan Credit Rating Agency, Ltd. (JCR); Moody’s Japan K.K. (Moody’s); Moody’s SF Japan K.K. (Moody’s); Standard & Poor’s Ratings Japan K.K. (S&P); Rating and Investment Information, Inc. (R&I); Fitch Ratings Japan, Ltd. (Fitch); and Nippon Standard & Poor’s K.K. (S&P). Disregarding overlaps in company groups, Japan has five CRAs (JCR, Moody’s, S&P, Fitch and R&I); while JCR and R&I operate primarily in Japan; Moody’s, S&P and Fitch operate globally.
Table 2. The major indicators through which CRAs assess companies
FSA classifies five CRA risk weightings to corporate debt (Table ).
Table 3. Correspondence that rating and credit category (exposure for corporations)
Table 4. Number of defaulting companies
CRAs assess various factors when affixing ratings, including macroeconomic conditions, industry properties, companies’ industry positions, competitiveness and financial indicators. As a precursor for comparisons with our simple default prediction model, Table identifies the major indicators using which CRAs assess companies.Footnote2
4. Empirical analysis
4.1. Overview of data
We accessed financial and non-financial indicators of each TSE-listed company from eol Japan, an annual report database. Data used in this study are publicly available in each company’s annual report. We examined companies that were continuously listed on the TSE (first section, second section and Mothers) from fiscal 2003 to fiscal 2009. We also identified companies that were listed in fiscal 2003 and had defaulted by fiscal 2009. We affixed default when proceedings under corporate rehabilitation law, bankruptcy and special liquidation were accepted. In this study, we refer to companies that have not defaulted as continuing companies. The data comprise 12,236 observations and 1,771 companies (including 24 defaulting companies). Table outlines the defaulting companies for each fiscal year.
4.2. Variables
Fiscal year is our unit of time, and year-end fiscal 2003 is time . Let
be a binary variable that is 0 if company i is operating at time
and 1 if it defaults between t and
. Let
be the explanatory variable vector indicating the characteristics of company i at time t. Financial and non-financial performance indicators are included in
.
4.3. Estimation method
In analysing the influence of companies’ characteristics on default, we used panel binary logit analysis with a random effect model (RE model) to control for unobserved heterogeneity. Cameron and Trivedi (Citation2005, Chapter 23) offer a detailed explanation of RE models, which is briefly stated here.
We observe financial and non-financial indicators x of N companies at time points and their default state y at
. Thus, the RE model is as follows:
(2)
(2)
where is a logistic function and
is an individual effect. We assumed
and no correlation between
and
.
Therefore, the joint density of the ith observations is as follows:(3)
(3)
where is the probability density function of
(4)
(4)
The log likelihood of all data is indicated as(5)
(5)
In Equation (5) parameters are estimated as the maximum. This procedure is maximum likelihood estimation.
4.4. Estimated results
In examining customary indexes of profitability, safety and financing and company size, we employed the Akaike information criterion (AIC) to select explanatory variables with goodness of fit and statistical significance. Four variables were suitable to our requirements: return on assets (ROA), equity ratio (ER), free cash flow (FCF) and average years of service (AYS). Default prediction models in previous literature seldom use these variables except ER; however, some rating agencies use ROA, ER and FCF in grading. Moreover, fiscal year dummies (D2004, D2005, , D2009) are used to remove macro influences such as the 2007–2008 global crisis. Table outlines the fundamentals of statistics for each variable.
Table 5. The fundamentals of the statistics
Table 6. Results of the panel binary logit analysis
ROA varied narrowly with a mean (standard deviation) of 4.92 (6.19). The mean for ER was about 47.89, thus indicating that sampled companies generally raise capital equally via equity and debt. FCF varies widely with a mean (standard deviation) of 4.79 (56.21). AYS varies narrowly with a mean (standard deviation) of 14.38 (5.13).
The result of the analysis indicated that ROA, ER, FCF and AYS correlated a significantly and negatively with default, while D2007 had a significant positive correlation (Table ).
(a) | ROA is ordinary profit divided by total capital and multiplied by 100. It denotes how successfully companies invest capital. As ordinary profits are operating plus non-operating profits minus non-operating expenses, ROA represents profitability from core businesses and non-core investments such as financial instruments. Our results demonstrated that companies with large ROA—that is, highly profitable companies—are less likely to default. | ||||
(b) | ER is equity capital divided by total capital multiplied by 100. It represents the stability of companies’ capital structures, therefore reflecting their safety. Our results demonstrated that companies with large ER—that is, highly safe companies—are less likely to default. | ||||
(c) | FCF is obtained by subtracting cash flow from investments from operational cash flow. The unit of measure is 1 billion yen. FCF represents surplus funds from operations minus cash invested in the business. Therefore, it reflects entire enterprise’s size and financing. Our results demonstrated that companies with large FCF—that is, large companies well-financed in their entirety—are less likely to default. | ||||
(d) | AYS captures current employees’ average years of service. The unit of measure is one year. AYS represents companies’ years in operation and employees’ average tenure. Our results demonstrated that companies with longer AYS are less likely to default. Few studies featuring default prediction models have used such non-financial indicators. Thus, this is another distinctive contribution of our study. |
As shown in Table , Model (2) has the smallest AIC and therefore the best fit.
5. Scores and ratings of our models
5.1. Scores of our models
This section scores companies using our models following the empirical analysis in Section 4. We calculated scores by multiplying the coefficients obtained from our empirical results times the actual value of variables. Model (1) adopted ROA, ER, AYS and time dummies as explanatory variables, while Model (2) adopted ROA, ER, FCF, AYS and time dummies as explanatory variables.
Equations (6) and (7) provide the formulas for scoring Models (1) and (2), respectively.(6)
(6)
(7)
(7)
If model construction and parameter estimations are robust, our models should demonstrate that companies with high scores for the same fiscal year show greater likelihood of default.
5.2. Ratings by our models
From Equations (6) and (7), we calculated each company’s scores for each sampled year and arranged them in ascending order; we divided them equally into 10 categories and assigned a number grade (1,2,,10) to each. If the model construction and parameter estimations are robust, higher numbers indicate companies that are more prone to default.
Table outlines the empirical results of relationships between ratings and defaults in Models (1) and (2).Footnote4
Table 7. Results of default prediction by ratings of Models (1) and (2) during the sampled period
Models (1) and (2) assigned higher scores—that is higher likelihood of default—(8, 9 and 10) to 21 of 24 companies that defaulted during the sampled period. However, Model (1) rated one defaulting company as 1 in 2005 and two defaulting companies as 4 and 7, respectively, in 2007; Model (2) rated one defaulting company as 5 in 2005 and two as 6 and 7, respectively, in 2007. Our models could not anticipate these defaults because they emerged through conditions exogenous to the models.
Rated 1 by Model (1) and 5 by Model (2) in 2005, IXI’s published financial statements—the basis for both our models—were not credible because the company overstated sales by round-tripping. Rated 7 by Model (1) and 6 by Model (2) in 2007, Inoue Kogyo was driven to default by plunging stock prices and suspicion of fictional capital increases rather than failures in core business. Suruga Corporation, rated 4 by Model (1) and 7 by Model (2) in 2007, was driven to default when its executives were arrested on suspicion of violating the Attorney Act and its stock price collapsed. Thus, our models cannot anticipate defaults due to fraud or factors external to ordinary operations.
5.3. Comparison with CRA ratings
Using historical records for one fiscal year before companies defaulted, this section compares our ratings with those of CRAs. Table classifies ratings by S&P, R&I, JCR and Moody’s into five categories (1, 2, 3, 4 and 5). Our model employed 10 categories. Table outlines the results.
Table 8. Comparison of our ratings and historical record of ratings by CRAs one fiscal year before companies defaulted. Our rating employs 10 categories, while ratings by CRAs are classified into 5 categories
CRAs rated seven companies in the year preceding their default. Our models awarded these companies ratings of 9 and 10—thus indicating higher likelihood of default. For the above-mentioned reasons, however, our models could not assign a low rating to Suruga.
Among the five FSA-approved categories, CRAs generally assign 5 as their lowest rating (highest likelihood of default) and 4 as their second-lowest. However, some CRAs employ 3 as their middle rating among the five categories.
Although few TSE-listed Japanese companies defaulted during the sampled period, the record confirms that our model performed as well or better than CRA assessments.
5.4. Goodness of fit of our models
We evaluate our models’ goodness of fit with the sampled data. To ascertain that our models reliably anticipate default, we evaluate the area under the curve (AUC) of the receiver operating characteristic curve (ROC). Table display these results.
Table 9. AUC of ROC on our ratings and scores in sampled period
Table illustrates that values of AUC of ROC are mostly 0.8 and higher—i.e. approximately 1—and therefore our models satisfactorily display goodness of fit, thus, again indicating that our scores reliably anticipate the likelihood of default. The exception appears in fiscal 2005, when the only default that year, IXI, failed for reasons exogenous to our model as explained above.
6. Model validation outside of sampled period
6.1. CRA Ratings
This section confirms that our models reliably anticipate default for companies outside of sampled period. We calculated companies’ scores in fiscal 2010 and 2011 by substituting the values of variables into Equations (6) and (7). Coefficients were calculated using data from fiscal 2003 to fiscal 2009. The scores were used to classify companies into 10 ratings by fiscal year, in ascending order. If the model construction and parameter estimations are robust, companies with high scores were less likely to default. Our ratings reliably anticipate defaults occurring during fiscal 2010 and 2011, outside of sampled period. Table displays the results for each fiscal year.
Table 10. Results of default prediction by ratings of Models (1) and (2) outside the sampled period
Model (1) reliably anticipated the defaults of three of the four companies that defaulted in 2011 and 2012 as evidenced through their low ratings (10). However, it accorded Elpida Memory, Inc. a score of 7 in 2010, the highest rating.
On the other hand, Model (2), which adopts AYS to reflect companies’ years in operation and employees’ average tenure, assigned low ratings (9 and 10) to all defaulting companies. Model (2) assigned Elpida the second-lowest rating.
By adding a non-financial indicator such as AYS to Model (1)—i.e. Model (2), we could anticipate defaults successfully for periods outside the sample. This is another major contribution of our study.
6.2. AUC of ROC outside sample
We evaluated the goodness of fit of our models for periods outside our sample. To confirm that our score reliably anticipated default, we compared AUC of ROC. Table presents the results.
Table 11. AUC of ROC for our ratings and scores outside the sampled period
Since values of AUC of ROC are 0.9 and higher, approximating 1, our model predicts the default accurately even if outside sampled period and Model (1) could more reliably anticipate defaults than Model (2).
7. Conclusion
Drawing upon Japanese data spanning 2003–2009 for TSE-listed corporations, this study has proposed a model for assessing default risk that satisfies the standardizations set by the Basel Accords without relying on CRAs. Comparison with the historical record reveals that our model anticipates defaults as accurately as credit ratings, even for periods outside our sample. As mentioned in Section 5.2, by the reason of window dressing, our model assigns high rating to a few defaulting companies. However, these incorrect ratings are rate cases and, in most cases, our model can predict the defaults in a high accuracy. Moreover, in the case of window dressing, CRAs is also likely to be deceived and assign high ratings.
Our findings elaborate on previous studies and bear several implications for investors and regulators. First, our method requires only publicly available data and is accessible to any analyst familiar with routine financial information. Second, it overcomes two acknowledged deficiencies in credit ratings—lack of uniformity in assessing likelihood of default and recovery rates, especially in the near term, and slow reaction to credit events. Third, unlike the existing CRA models, our model pertains to companies that do not issue equity and debt and presents a way of differentiating unrated companies. Fourth, it demonstrates the importance of non-financial indicators like employee tenure for anticipating defaults. Fifth, our method can reveal firm-specific factors that lead to default and allows investors and regulators to identify firms by likelihood of default. Thus, through these five aspects, it surpasses CRA ratings and default prediction models that rely on financial and market data (e.g. Campbell et al., Citation2008; Shumway, Citation2001).
However, future research avenues remain. First, a macro environment could alter the probabilities of default indicated by our model; risk-weighted measures corresponding our rating might also need modifications. It is necessary to develop a method for calculating weighted risk that accommodates macroeconomic influences. Future studies could also extend the period sampled to confirm the robustness of our results.
Additional information
Funding
Notes on contributors
Yukiko Konno
Yukiko Konno is an assistant professor at the Institute of Innovation Research, Hitotsubashi University. Her research interests include credit risk management, financial management and quantitative analysis of company activities.
Yuki Itoh
Yuki Itoh is an associate professor at the Graduate School of Social Science, Yokohama National University. His research interests include credit risk management and quantitative risk management.
Notes
1 Japanese Bankers Association (2013) Analysis of financial statements all banks 2012.
2 For details regarding indicators, see Japan Credit Rating Agency (Citation2012), Moody’s Investors Service (Citation2014), Standard & Poor’s Financial Services (Citation2013), Fitch Ratings (Citation2013) and Rating and Investment Information (Citation2012).
3 See Winkelmann and Boes (Citation2009, Chapter 3) for details about AIC.
4 Hereafter, we indicate the fiscal year when we predict defaults. We judged whether defaults actually occurred in the year following the indicated year.
References
- Agarwal, V., & Taffler, R. (2008). Comparing the performance of market-based and accounting-based bankruptcy prediction models. Journal of Banking & Finance, 32, 1541–1551.
- Altman, E. I. (2011). Default recovery rates and LGD credit risk modelling and practice. In A. Lipton & A. Rennie (Eds.), The Oxford handbook of credit derivatives. Oxford: Oxford University Press.
- Altman, E. I. (1968). Financial ratios, discriminant analysis and the prediction of corporate bankruptcy. Journal of Finance, 23, 589–609.
- Altman, E. I., & Rijken, H. A. (2004). How rating agencies achieve rating stability. Journal of Banking & Finance, 28, 2679–2714.
- Bauer, J., & Agarwal, V. (2014). Are hazard models superior to traditional bankruptcy prediction approaches? A comprehensive test. Journal of Banking & Finance, 40, 432–442.
- Beaver, W. H., McNichols, M. F., & Rhie, J.-W. (2005). Have financial statements become less informative? Evidence from the ability of financial ratios to predict bankruptcy. Review of Accounting Studies, 10, 93–122.
- Black, F., & Scholes, M. (1973). The pricing of options and corporate liabilities. The Pricing of Options and Corporate Liabilities, 81, 637–654.
- Cameron, A. C., & Trivedi, P. K. (2005). Microeconometrics: Methods and applications. New York, NY: Cambridge University Press.
- Campbell, J. Y., Hilscher, J., & Szilagyi, J. (2008). In search of distress risk. The Journal of Finance, 63, 2899–2939.
- Cantor, R. (2001). Moody’s investors service response to the consultative paper issued by the Basel Committee on Bank Supervision “A new capital adequacy framework”. Journal of Banking & Finance, 25, 171–185.
- Doumpos, M., Niklis, D., Zopounidis, C., & Andriosopoulos, K. (2015). Combining accounting data and a structural model for predicting credit ratings: Empirical evidence from European listed firms. Journal of Banking & Finance, 50, 599–607. (forthcoming)
- Fitch Ratings. (2013). Corporate rating methodology (Technical report). London: Author
- Hajek, P., & Michalak, K. (2013). Feature selection in corporate credit rating prediction. Knowledge-Based Systems, 51, 72–84.
- Hillegeist, S. A., Keating, E. K., Cram, D. P., & Lundstedt, K. G. (2004). Assessing the probability of bankruptcy. Review of Accounting Studies, 9, 5–34.
- Hilscher, J. & Wilson, M.I. (2013). Credit ratings and credit risk: Is one measure enough?. AFA 2013 San Diego Meetings Paper. Malden, MA: American Finance Association.
- Horrigan, J. O. (1966). The determination of long-term credit standing with financial ratios. Journal of Accounting Research, 4, 44–62.
- Huang, Z., Chen, H., Hsu, C.-J., Chen, W.-H., & Wu, S. (2004). Credit rating analysis with support vector machines and neural networks: A market comparative study. Decision Support Systems, 37, 543–558.
- Japan Credit Rating Agency. (2012). JCR’s rating methodology (Technical report). Tokyo: Author.
- Katsuda, H., & Tanaka, K. (2008). Neural network method as a new corporate rating method for Basel II. Osaka Economic Papers, 57, 32–45. [in Japanese].
- Kiff, J., Kisser, M., & Schumacher, L. (2013). Rating through-the-cycle: What does the concept imply for rating stability and accuracy? (IMF Working Papers No. 13/64). Washington, DC: International Monetary Fund.
- Kirstein, R. (2002). The new Basel Accord, internal ratings, and the incentives of banks. International Review of Law and Economics, 21, 393–412.
- Löffler, G. (2013). Can rating agencies look through the cycle? Review of Quantitative Finance and Accounting, 40, 623–646.
- Merton, R. C. (1974). On the pricing of corporate debt: the risk structure of interest rates. Journal of Finance, 29, 449–470.
- Moody’s Investors Service. (2014). Rating methodology: Global manufacturing companies (Technical report). New York, NY: Author.
- Ohlson, J. A. (1980). Financial ratios and the probabilistic prediction of bankruptcy. Journal of Accounting Research, 18, 109–131.
- Rating and Investment Information (2012). Basic methodologies for R &I’s credit rating (Technical report). Tokyo: Author.
- Shumway, T. (2001). Forecasting bankruptcy more accurately: A simple hazard model. Journal of Business, 74(1), 101–124.
- Standard & Poor’s Financial Services (2013). Criteria - corporates - general: Corporate methodology (Technical report). New York, NY: Author.
- Van Roy, P. (2005). Credit ratings and the standardised approach to credit risk in Basel II (Working Paper Series 0517). Frankfurt: European Central Bank.
- West, R. R. (1970). An alternative approach to predicting corporate bond ratings. Journal of Accounting Research, 8, 118–125.
- Winkelmann, R., & Boes, S. (2009). Analysis of microdata. 2nd edition. New York, NY: Springer.
- Xu, M., & Zhang, C. (2009). Bankruptcy prediction: The case of Japanese listed companies. Review of Accounting Studies, 14, 534–558.