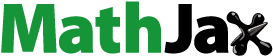
Abstract
Manufacturers of packaged consumer goods strive to develop a new product with a special feature that could provide additional value to consumers. However, it is less clear whether such an effort is still rewarding in terms of margin if manufacturers are losing power to retailers as some have argued. To investigate this issue, we conduct an economic analysis in the Japanese yogurt market incorporating strategic interaction between manufacturers and a retailer as well as between manufacturers by extending the framework employed in the earlier literature to suit the retailer Stackelberg game which can reflect the possible power shift from manufacturers to retailers. We find (1) the manufacturers’ margins on special featured brands are larger than those on the others; (2) however, the manufacturer producing such brands is not able to leverage these brands to exert bargaining power over the retailer; and (3) the retailer obtains as large margins as the manufacturers on these brands. In the course of this research, we successfully portray the symmetrical relationship between manufacturer and retailer Stackelberg games, whereby the vertical Nash game is located in the midpoint of those two games.
Public Interest Statement
The retailer power is said to have increased with respect to manufacturers. Then the question arises is whether manufacturers are still willing to innovate because their efforts to develop a new product would end up benefitting only retailers. In this paper, we mathematically formulate an important game reflecting recent trend of increased power of retailers whereby retailers have controls over pricing. We bring this formulation to the Japanese yogurt market to examine if premium brands are still able to command commensurate profit given the power shift from manufacturers to retailers.
1. Introduction
Manufacturers of packaged consumer goods strive to develop a new product with a special feature that could provide additional value to consumers. Although the higher retail prices of those products would seem to be evidence of high margins for manufacturers, these prices might be higher because the retailer may garner a larger margin at the expense of the manufacturer’s profit given the alleged power shift from manufacturers to retailers.
In this paper, we conduct an economical analysis taking Japanese yogurt market as an example to investigate whether manufacturers’ effort to develop special featured brands still is rewarding in terms of margins. In Japan, some researchers say that the power shift from manufacturers to retailers is irreversible because of (1) the emergence of giant retailers that exert strong buying power and enjoy economy of scale, (2) their sophisticated information systems regarding consumers, and (3) increased retailer concentration (Kim, Citation2010).Footnote1 Formulating a new game theoretic framework to describe this phenomenon and testing it with the real data in yogurt market, albeit a small one, would be of great interest to researchers in the field as well as those working for innovating manufacturers facing similar circumstances.
Our analysis principally follows Che, Sudhir, and Seetharaman (Citation2007). However, while they postulate manufacturer Stackelberg (MS) and vertical Nash (VN) games as two possible vertical strategic interactions between the manufacturers and the retailer, the lack of a retailer Stackelberg (RS) formulation in that paper may limit the scope of the analysis in view of possible power shift from manufacturers to retailers. Thus, we derive an RS formulation to accommodate the market structure favoring retailers. To the best of our knowledge, this is the first instance of an RS formulation in the context of discrete choice model.Footnote2 This study is hence unique, in that it successfully portrays the symmetrical relationship between MS and RS games, whereby the VN game is located in the midpoint of those games.
The rest of the paper is organized as follows. In the next section, we present the general framework of the model and explain the estimation procedure. We describe the data in Section 3. In Section 4, we present and discuss empirical results. In Section 5, we conclude by discussing our research results, the limitations of this study, and an avenue for further research.
2. The Model
In this section, we present the demand and supply models as well as estimation procedure.
2.1. Demand-side specification
We use a multinomial logit model to estimate household brand choice behavior employing the latent class model to capture heterogeneity among households (Kamakura & Russell, Citation1989). The indirect utility of household i () for brand j (
) on shopping occasion
(
) is defined as
(1)
(1)
where vector includes brand dummy variables and the retail price of brand j that household i faces on shopping occasion
,
is the corresponding vector of parameters for households in segment s,
is the attribute similarity index which measures the similarity of two brands and
is the corresponding coefficient (Che et al., Citation2007). Specifically, the attribute similarity index of brand j relative to the previously purchased brand k is defined as
where P is the number of product attributes characterizing the product, and
are indicator variables taking 1 if
and if the two brands have the same level of attribute p, respectively, and
is a perceived importance weight of attribute p to be estimated. The coefficient
in (1) is further parametrized as
where is the base-line state dependence level of those in segment s,
is the vector of the demographic characteristics of household i (i.e. gender and age), and
is the corresponding vector of parameters for
. We note that a positive (negative) value of
reveals inertial (variety-seeking) behavior, that is, a brand consumption experience increases (decreases) the probability of repurchasing the brand on the consecutive purchasing occasion. The term
is a composite measure of unobserved (to the researcher) demand characteristics that affect all households commonly and
are errors distributed iid Gumbel. The outside option (
) is specified as determinant part of the utility being zero.
2.1.1. Demand-side estimation
Since ignoring might result in biased estimation (Berry, Citation1994; Besanko, Dubé, & Gupta, Citation2003; Nevo, Citation2001; Villas-Boas, Citation1999; Villas-Boas, Citation2005), we employ the instrumental variable estimation for price as follows:
where is the instrument for price
,
and
are parameters to be estimated, and
is an error term. They are defined for all brands
and dates
in the study period. For the instrument, we use the average retail prices of yogurt in five stores we exclude from the analysis owing to lack of price information because those prices in other stores would reflect the general economic condition that would have affected retail prices in the target store as well and they would not be correlated to the unobserved demand shock (
) which would include the effect of store-level promotions such as in-store display.Footnote3
The likelihood function of the purchase history of household i belonging to segment s, where
is the set of brand dummy variables, is given by
dropping the parenthesis of , where
is the logit purchase probability of household i who belongs to segment s choosing brand j on shopping occasion
,
is the indicator function taking 1 if household i chooses brand j at time
and 0 otherwise,
is the conditional density function of
given
, and
is the density function of
. Then, the demand-side likelihood function is
where is household i’s probability of membership in segment s.
2.2. Supply-side specification
Following previous studies, we assume that the retailer is a local monopolist and maximizes its joint category profit (Besanko, Gupta, & Jain, Citation1998; Che et al., Citation2007; Sudhir, Citation2001; Villas-Boas, Citation2005).Footnote4 We further assume that there are multiple manufacturers that may produce multiple brands and sell these through the common retailer.
2.2.1. Supply model
As stated, we derived an RS formulation in addition to the MS and VN games as in Che et al. (Citation2007). In an RS game, the retailer first chooses its retail margins anticipating the reaction of the manufacturers in the first stage, and then the manufacturers choose their wholesale prices in the second stage conditional on the observed retail margins. We also consider Bertrand competition and collusion as two horizontal strategic interactions between manufacturers. As a result, we would estimate six different models. In the following, we first derive margins in the general form before we present those under specific games.
2.2.1.1. Profit functions
The profit function of the monopolistic retailer and collusive manufacturers are, respectively, defined as(2)
(2)
and
where ,
, and
are the market share, the wholesale price, and the marginal cost of brand j at time t, respectively, and M is the market size. Then the first-order condition (FOC) of the profit functionsFootnote5 are
(3)
(3)
and(4)
(4)
respectively,Footnote6 with the fixed M removed and the subscript t dropped for convenience.
Stacking (3) vertically for and rearranging them in a matrix form, the retail margins in the general form are obtained as
(5)
(5)
assuming the inverse of the first matrix on the right-hand side of Equation (5) exists. Similarly, by stacking (4) vertically for and rearranging them, the optimal manufacturer margins in the general form can be obtained as
(6)
(6)
The response curves in (5) and
in (6) will be determined in MS, RS, and VN games, respectively, below.
2.2.1.2. Margins in the MS game
We briefly review how retailer and manufacturer margins are derived in the MS game. The game is solved backward and retail margins are derived first. In the second stage of the game, since wholesale prices are already determined before retail prices are, it follows that(7)
(7)
for all . Substituting (7) for (5) yields the optimal retailer margin as
(8)
(8)
where ,
is the matrix whose (j, k) element is
, and
.Footnote7 Note that
and
in (8) can be directly observed and calculated.
On the other hand, in deriving manufacturer margins, the matrix of how a retailer optimally reacts to wholesale price change, in (6), must be indirectly inferred. Since the change in wholesale price of a brand would affect retail prices of all brands, the term
needs to be estimated by totally differentiating the FOC of the retail profit function with respect to the wholesale price as
(9)
(9)
Solving (9) for for all
, substituting them for (6), and rearranging them as a matrix yield the optimal manufacturer margins in the MS game as
(10)
(10)
where and
is the matrix whose (j, h) element is
For the case of Bertrand competition, we have(11)
(11)
instead of (10), where “” denotes element-by-element multiplication and
is a
ownership matrix whose (j, k) element, denoted as
, is an indicator variable taking 1 if brands j and k are made by the same manufacturer and 0 otherwise.Footnote8
2.2.1.3. Margins in the RS game
Now we derive margins in the RS game. In this game, it follows that for all
in the second stage since the retail margin on brand h or
is set prior to wholesale prices being set. Equivalently, we have
(12)
(12)
since and
. Then, from (12) and (14), we have
(13)
(13)
Stacking (13) vertically for and rearranging them, we derive the optimal manufacturer margins in the RS game as
(14)
(14)
To derive retail margins in the RS game, the matrix of how manufacturers optimally react to retail price change in (5) must be inferred. Similar to the MS case, we totally differentiate the FOC of the manufacturers’ profit function in (13) with respect to
and solve the resulting equations for
, the optimal reaction curve of the manufacturer. We then substitute
for (5) to derive retail margins in the RS game as
where is a
matrix whose (l, j) element is
(15)
(15)
and(16)
(16)
For the case of Bertrand competition, we have
where is a
matrix whose (l, j) element is
(17)
(17)
We present the derivation in detail in Appendix 1.
2.2.1.4. Margins in the VN game
Che et al. (Citation2007) substitute (7) for (5) to derive retail margin (8) and substitute (12) for (6) to derive the manufacturer margin (14) in the VN game because conditions (7) and (12) simultaneously hold since the retailer and manufacturers move simultaneously in the game. We note that the margins of the retailer and manufacturers become identical if manufacturers collude in this game. Table presents the formulation of margins under each of the three games.
2.2.1.5. Arriving at VN from two extreme directions
The term in retail profit in the RS-Bertrand game is the matrix whose (l, j) element is
. Notice that these terms are 0 for
when we employ the behavior (7) of manufacturers in the MS game. In other words, retailer profit in the VN game can be obtained by applying the manufacturer behavior in the MS game to the retail margin. Similarly, the term
in manufacturer profit in the MS game is (16) whose (l, h) element is
. Note that the matrix of these terms becomes an identity matrix when we employ the behavior (12) of the retailer in the RS game. This is the symmetrical relationship of MS and RS games we refer to in Section 1.
2.2.1.6. Margins in the forward-looking model
Following Che et al. (Citation2007), we also test forward-looking model. However, because we find that the VN-collusion game best fits the data, we only report the result for that game in the following.
The objective function of the one-period forward-looking retailer is , where
and
are the retailer profit functions defined in (2) for periods 1 and 2, respectively, and the term
is an exogenously given discount rate. Then, the FOC is
(18)
(18)
for where the second subscripts for S and p correspond to the period. The first line in (18) corresponds to the first-period profit function and the second line corresponds to the second-period profit function. After calculating unknown terms in the first line, stacking them for
, and rearranging them, the retailer margins in the VN-collusion forward-looking model are derived as
(19)
(19)
where all the subscripts in the above equation correspond to period 1 or 2 and the term is a
diagonal matrix whose jth diagonal is
. The second-period profit is obtained by the procedure already presented. We omit the manufacturer margins in this case as they are identical to (19).
2.2.1.7. Marginal cost
Following Che et al. (Citation2007), we parameterize the marginal cost as(20)
(20)
where is the brand-specific intercept term,
is the vector of observable cost shifters, and
is the corresponding vector of parameters. The cost shifters used in this analysis will be listed in Section 3.
2.2.9. Supply-side estimation
To estimate parameters in (20) and obtain the likelihood of the supply-model, we exploit the following relationship:
where
and
are estimated margins for the retailer and manufacturers on brand j at time t, respectively,Footnote9 and is the random error term. If we assume that the error terms
follow a normal distribution with mean zero and finite variance to be estimated, the right-hand side of the equation
is also assumed to follow the normal distribution. Then the supply-side likelihood function is(21)
(21)
where is the density function of
. We estimated (21) for all six games and compared the results by Vuong test statistics to select the best-fitting model.
3. Data
We use daily scanner-panel data on the yogurt category between January 2007 and December 2008 in an anonymous retail chain located in western Tokyo, Japan. This market is suitable for our analysis because it already had two well-established brands with a special feature using newly found bacilliFootnote10 and a power shift from manufacturers to retailers was said to already have been observed in the Japanese food industry (Kim, Citation2010). Between two types of yogurt—box type and snack type—we choose the latter for our empirical analysis as the former did not have a brand with a special feature.
We choose the seven top selling brands for our empirical analysis.Footnote11 Table summarizes the data on the brands. The unit of price is Japanese yen per one gram.
As mentioned, two brands (brand 5 and its low-fat version, brand 6) with a special feature had existed during the observation period. After choosing households that only purchased the selected brands at least twice during the period, 183 households who made 15,194 shopping trips and purchased 2,550 units of yogurt remain. The available demographic variables in our data are age and gender. The average age of the shoppers is 59.4 (with standard deviation of 19.6) and 76.5% of them are female.
In addition, we collected weekly data of ingredients (domestic raw milk prices, domestic cream price indexes, and international sugar prices), labor wages for the four prefectures where the seven selected brands had been produced, and international oil price during the study period for the independent variables of marginal cost estimation. We collected domestic raw milk prices and cream price indexes from Jmilk (Citation2014); labor wage in four prefectures from statistical departments of corresponding prefectures; international sugar price from Agriculture & Livestock Industries Corporation (Citation2014); and international oil price from International Monetary Fund (Citation2014).
4. Empirical results
4.1. Demand-side results
We find that the latent class model with four segments is optimal.Footnote12 Table presents parameter estimates of the demand model (with standard errors in parentheses).
In Table , “Brand” entries represent the brand-specific intercepts relative to the outside option, presented under “Demographics” are estimated parameters for , and “Agar Usage" entry is the estimate of importance weight for this attribute in calculating the attribute similarity index.Footnote13 Although the estimates of demographics are generally insignificant, we find some patterns for each segment. For example, Segment is characterized by variety-seeking behavior regardless of the age and gender. Specifically in Segment , a male aged 94 (the maximum age in the sample) would have SD of
. All the other people in this segment would have SD lower than
and thus would be variety seekers. In Segment 1, males of all ages and females aged more than 48 years have a tendency toward inertia. In Segment 3, males of all ages and females aged more than 39 years have a tendency toward inertia. In Segment , females of all ages and males aged less than 34 years are variety seekers.
4.2. Supply-side results
4.2.1. Margins
Table reports the estimated margins in the best-fitting VN-collusion forward-looking model and their standard errors (in parentheses).Footnote14 Since the retailer’s and manufacturers’ margins are identical in this game, we simply report them as “Margins” in Table rather than reporting them separately. The standard errors turn out to be very small because the prices of those brands stay fairly constant during the study period.
Table 1. The margins under each game
Table 2. Product summary of the seven yogurt brands
Table 3. Parameter estimates of the demand model
Table 4. Estimated margins in the VN-collusion forward-looking model
We note that brands 5 and 6 yield the two largest margins, and brand 6 in particular yields the highest margins relative to the average retail prices, implying that brands with the aforementioned special feature could indeed earn a large amount of margins. The implications of these results are discussed in Section 5.
4.2.2. Marginal cost
We find that after including the manufacturer dummy variables, all cost variables except for domestic cream price indices and international oil price become insignificant in the marginal cost estimation in the best-fitting model.Footnote15 International oil price affects marginal cost as oil is required for yogurt-making machine, refrigeration, air conditioning in yogurt factories as well as transportation by refrigerator trucks.
5. Conclusion and discussion
In this paper, we derive the RS game formulation in addition to MS and VN formulations in Che et al. (Citation2007) and show that MS and RS games stand at opposite extremes whereas the VN game lies in between these two games. We then empirically analyze pricing behavior of firms in the Japanese yogurt market under that extended formulation, incorporating heterogeneity among households, state dependence in brand choice, and firms’ forward-looking behavior while correcting for price endogeneity.
We find that the brands with the differentiated feature (i.e. enhancing the health effect of yogurt by newly found bacilli) enable the manufacturer to command larger margins than the other brands, showing that the manufacturer’s effort in this direction can be interpreted as rewarding. However, we also find that the power to charge larger margins does not spill over to the other brands of a manufacturer, as the manufacturer’s margin on brand 4 is in line with those of the others even though brand 4 is produced by the manufacturer producing brands 5 and 6. We also note that that the best-fitting model using a VN framework suggests that even the monopolistic retailer could not have completely controlled prices at least in this category during the study period. The fact that the retailer only earns the same amount of margins as manufacturers is somewhat counter-intuitive given the conventional wisdom of the power shift from manufacturers toward retailers. However, this result is consistent with the findings of Farris and Ailawadi (Citation1992), which questions such conventional wisdom (please see Ailawadi (Citation2001) for a survey of this topic). Nevertheless, that the retailer still earns large margins on these brands might indicate such a power shift. Further research in this area would be necessary.
One of the limitations of this study is the assumption of the monopolistic retailer, as retail competition is shown to affect the relationship between a retailer and manufacturers (Raju & Zhang, Citation2005). In fact, Statistics Bureau of the Ministry of Internal Affairs and Communications (Citation2014) indicates that the average retail prices of yogurt are slightly higher in stores that have no competitors in their neighborhood than in stores with competitors nearby. We leave this issue for future research.
Acknowledgements
We thank an editor and two referees for many constructive comments on earlier versions of the article.
Additional information
Funding
Notes on contributors
Tomohito Kamai
Tomohito Kamai is a PhD candidate in Graduate School of System and Information Engineering, University of Tsukuba. He holds MBA with emphasis in marketing, at William E Simon School of Business, University of Rochester. His interest is in pricing in a differentiated product market.
Yuichiro Kanazawa
Yuichiro Kanazawa is Professor of Statistics at the Faculty of Engineering, Information, and Systems, University of Tsukuba. He holds a PhD in statistics from Yale University. He has publication in the area of applied microeconomics, industrial organization, organizational behavior, and quantitative criminology as well as in statistics proper.
Notes
1 These factors largely overlap with those listed by Kadiyali, Chintagunta, and Vilcassim (Citation2000) as the reasons of the similar power shift in the US market.
2 Choi (Citation1991) introduces an RS formulation but the model in that paper assumes linear demand function.
3 Though our data contained sales information in six stores of the same chain, there was only one store where at least of one purchase of the selected brands of yogurt (for our analysis) was recorded everyday. However, if we combined all five other stores, there were enough data for us to be able to calculate the average retail prices of the seven brands of yogurt we used in this analysis.
4 Sudhir (Citation2001) empirically shows that the retailer earns the maximum profits when it engages in category profit maximization pricing, which supports the assumption widely adopted in the literature.
5 The optimal retail price should not be affected by the price of the other brands; else,
will no longer be optimal. Thus,
becomes 0 if
is assessed at its optimal level.
6 Note that it is assumed for all
, as wholesale price would not affect marginal cost in general.
7 For convenience in comparison to Che et al. (Citation2007), notations and most definitions are the same as those in that paper.
8 We note that the collusion case can be accommodated by expression (11), namely, by setting as an identity matrix.
9 Margins are parametrized as shown since the calculation of margins involves the partial derivative of the logit probability with respect to prices (i.e. and
), since
is the market share of brands which is essentially
times market size.
10 We cannot disclose the name of the bacilli as it would identify the product.
11 The combined market share of the seven selected brands is 44.5%, excluding box-type yogurt. The number is relatively small because there existed 300 brands during the study period and market share of each brand was small. We chose top selling seven brands because the minor brands had many missing daily price information.
12 We increased the number of segments to minimize Akaike Information Criteria (AIC). Although AIC was lower for the five-segment model than for the four-segment model, we chose the latter because the size of fifth segment became 0.7% in the five-segment model, as targeting a segment size less than 0.7% out of a sample size of 183 does not make much sense.
13 “Agar Usage” is whether the yogurt contains agar or not. Agar is used to produce so-called “hard-type” yogurt. We also tested “Raw Milk Usage” (i.e. the proportion of raw milk in yogurt; three levels—none, some, and all) and “Fat Level” (i.e. the amount of fat in yogurt; three levels—less than 3%, between 3% and 4%, and more than 5%) as candidates for the attributes, but they were found to be nonsignificant.
14 We also tested (1) a multinomial logit model without state dependence and (2) a model with state dependence but without forward-looking behavior, in addition to the forward-looking model, but the Vuong test statistics showed that the VN-collusion forward-looking model fitted the data best.
15 The results are available on request.
References
- Agriculture & Livestock Industries Corporation. (2014). Sugar statistical data. Retrieved September 1, 2014, from http://sugar.alic.go.jp/world/w_market.htm
- Ailawadi, K. L. (2001). The retail power-performance conundrum: What have we learned? Journal of Retailing, 77, 299–318.
- Berry, S. T. (1994). Estimating discrete-choice models of product differentiation. The RAND Journal of Economics, 25, 242–262.
- Besanko, D., Dub{\’e}, J.-P., & Gupta, S. (2003). Competitive price discrimination strategies in a vertical channel using aggregate retail data. Management Science, 49, 1121–1138.
- Besanko, D., Gupta, S., & Jain, D. (1998). Logit demand estimation under competitive pricing behavior: An equilibrium framework. Management Science, 44, 1533–1547.
- Che, H., Sudhir, K., & Seetharaman, P. (2007). Bounded rationality in pricing under state-dependent demand: Do firms look ahead, and if so, how far? Journal of Marketing Research, 44, 434–449.
- Choi, S. C. (1991). Price competition in a channel structure with a common retailer. Marketing Science, 10, 271–296.
- Farris, P. W., & Ailawadi, K. L. (1992). Retail power: Monster or mouse? Journal of Retailing, 68, 351–369.
- International Monetary Fund. (2014). IMF primary commodity prices. Retrieved September 1, 2014, from http://www.imf.org/external/np/res/commod/index.aspx
- Jmilk. (2014). Database. Retrieved September 1, 2014, from https://www.j-milk.jp/gyokai/database/index.html
- Kadiyali, V., Chintagunta, P., & Vilcassim, N. (2000). Manufacturer-retailer channel interactions and implications for channel power: An empirical investigation of pricing in a local market. Marketing Science, 19, 127–148.
- Kamakura, W. A., & Russell, G. J. (1989). A probabilistic choice model for market segmentation and elasticity structure. Journal of Marketing Research, 26, 379–390.
- Kim, C. (2010). A change in channel relations in grocery industry and the industrial organization theory approach to distribution channels. The Business review (Osaka City University), 60, 189–208.
- Nevo, A. (2001). Measuring market power in the ready-to-eat cereal industry. Econometrica, 69, 307–342.
- Raju, J., & Zhang, Z. J. (2005). Channel coordination in the presence of a dominant retailer. Marketing Science, 24, 254–262.
- Statistics Bureau of the Ministry of Internal Affairs and Communications, (2014). Distribution of Prices by Type of Outlets, Location, Competitors - Japan, City Groups, Prefectures Retrieved September 2, 2014, from. Japan. http://www.e-stat.go.jp/SG1/estat/GL08020103.do?_toGL08020103_&tclassID=000001016501&cycleCode=0&requestSender=search
- Sudhir, K. (2001). Structural analysis of manufacturer pricing in the presence of a strategic retailer. Marketing Science, 20, 244–264.
- Villas-Boas, J. M., & Winer, R. S. (1999). Endogeneity in brand choice models. Management Science, 45, 1324–1338.
- Villas-Boas, J. M., & Zhao, Y. (2005). Retailer, manufacturers, and individual consumers: Modeling the supply side in the ketchup marketplace. Journal of Marketing Research, 42, 83–95.
Appendix 1
Retailer margins in the RS-collusion case
Stacking the total derivatives of the manufacturer profit function in (13) with respect to vertically for
, we have 0
(A1)
(A1)
for some j since the marginal cost is not affected by the retail price (i.e. for all
). Further we have 0
(A2)
(A2)
since and
0(A3)
(A3)
since for all
and
for all
. Substituting (23) and (24) for (22) and rearranging it as a matrix, we have 0
(A4)
(A4)
Stacking (25) horizontally for and rearranging them, we have for
whose (l, j) element is defined in (15) 0
(A5)
(A5)
We obtain retailer margins in the RS-collusion game by transposing both sides of (26) and substituting it for (5).
Appendix 2
Retailer margins in the RS-Bertrand case
In the Bertrand competition case, we totally differentiate the FOC of the manufacturer profit function in the Bertrand competition 0(B1)
(B1)
instead of (13) with respect to . Stacking the derivatives of (27) vertically for
and rearranging them as a matrix, we have 0
(B2)
(B2)
Stacking (28) horizontally for and rearranging them, we have 0
(B3)
(B3)
with (l, j) element of being (17). We obtain retailer margins in the RS-Bertrand game by transposing both sides of (29) and substituting it for (5).