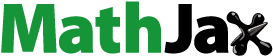
Abstract
Human capital accumulation is one of the key drivers of economic growth especially in developing countries that are trying to catch up in per capita income with the developed world. A high dropout rate of children from school can significantly impede growth. This paper presents evidence on school dropout rate being affected by household characteristics and social background. We investigate dropouts by using a large data-set from the Bangladesh Demographic and Health Survey, 2011. A fractional logit model, a beta regression model, and for comparison a simple OLS model all indicate our empirical strategy to be fairly robust. The large incidence of households having a child quitting school to enter the labor market also allows us to study the dropout using a probit model. We find many interesting results. Children from poor families with less educated parents drop out sooner with a lasting effect on educational outcomes. Households that have more than three children or more than five members also experience greater quit rates. Richer and richest categories of households have the lowest dropouts. Those facing a costly commute to school or having no access to electricity also quit earlier. We also present evidence on several other factors with important policy implications. Our results suggest that any strategy that seeks to encourage human capital accumulation must simultaneously address the school dropout rate through a combination of such factors as promotion of female education and expansion of credit for small businesses.
Public Interest Statement
Human capital accumulation is vital for economic growth in developing countries and their catch-up with the developed world. Bangladesh faces a high rate school dropout. We present evidence on school dropout being affected by household characteristics and social background based on a large data-set from the Bangladesh Demographic and Health Survey (BDHS), 2011. We use a range of statistical models to ensure the soundness of our results. We find that children from poor families with less educated parents drop out sooner with a lasting effect on their educational outcomes. Households that have more than three children or more than five members also experience greater quit rates. Richest households have the lowest dropouts. Children quit school earlier if they come from poor households, particularly those having no electric power at home or if they come from locations where commute to school can be a challenge. A strategy to encourage human capital accumulation must simultaneously address school dropouts by promoting female education and credit expansion for small businesses.
1. Introduction
Martin Luther King Jr. once said, “The job of the school is to teach so well that family background is no longer an issue.” In reality, however, children’s educational outcomes are affected by family, social, and economic backgrounds. In Bangladesh, as in many other developing countries, quality of education is always a subject of policy debate. But a bigger issue is whether a child will go to school to complete middle or high school education or will drop out before graduation due to poverty. Even government subsidies on education such as through free tuition and transportation can be inadequate for schools to retain children if other factors intervene. Examples of these factors could be acute poverty, low parental education, and a larger family size which make children work long hours to contribute to family income.
Education has long been considered a contributor to the development of human potential and social growth (Dewey, Citation1899). Torres (Citation1990) considered education to be a human right, an integral part in obtaining the access to political power and participation. Education is one of the important forces that can change a child’s adult life. So, parents’ understanding of the importance of education is crucial. Parents may not send their children to school if they do not see education as a medium of increasing productivity or opportunity cost of schooling in terms of forgone earnings is too high. On the other hand, even an uneducated parent may have a school going child because the parent understands the value of education through his or her experiences. In the larger population, however, disadvantaged children will face more challenges in attaining a higher degree than will others.
For Bangladesh, household conditions have always been a barrier to education. To study how household conditions affect the decision about whether a child will attend school in Bangladesh, we use data from Bangladesh Demographic and Health Survey (BDHS). To understand factors associated with school dropouts, we estimate relevant OLS and probit models and gain some additional microeconomic insights into schooling in Bangladesh.
2. Brief review of literature
Empirical literature on educational attainment of children focuses on economic and demographic characteristics of the households and social environment in which children live. Becker (Citation1993) argued that investment in human capital occurs within the larger context of household production with household resources serving as the main constraint. Thus, Becker suggests that educational attainment depends on conscious decision-making by families regarding how much to spend on their children’s education relative to spending on other goods.
Pritchett (Citation2013) gives examples of economic significance of school dropouts in Ghana, Tanzania, Turkey, and several other countries. Examining education in Tanzania, for instance, he argues that “… looking at the grade attainment profile, we see a significant drop-out as students move from basic to secondary education. While 68 percent of the cohort completes grade 7, only 29 percent completes grade 8 or higher, so nearly 40 percent of students drop out between grade 7 and 8.”
According to Amuedo-Dorantes and Lopez (Citation2015) who study enforcement of immigration laws and school dropouts among immigrants in the US, “Using data from the October Supplement of the CPS [Current Population Survey] for the years 1995–2010, we find that increased enforcement raises the probability of repeating a grade and dropping out of school for Hispanic youth with likely unauthorized parents, with the effects being concentrated among children ages 6 to 13.”
These findings and others such as Bruns, Filmer, and Patrinos (Citation2011), which has become an ongoing project, show that identification of factors related to household conditions and family structure can be critically important factors in affecting school dropouts. In the case of a developing country such as Bangladesh, low educational attainment because of dropouts is a most relevant subject in human capital accumulation, a factor on which a substantial portion of economic growth depends.
The implication that household poverty keeps education at a low level finds substantial support in the literature. Duncan and Brooks-Gunn (Citation1997) associate household poverty with several related ills such as school dropouts and low academic achievement, teenage pregnancy and childbearing, poor mental and physical health, delinquent behavior, and unemployment in early adulthood. Duncan, Brooks-Gunn, and Klebanov (Citation1994) find children who live in poverty for a longer period of time have substandard educational achievement and poor social and emotional functioning. Low incomes can contribute significantly to lower attainment and accentuate education inequality (Devkota & Upadhyay, Citation2016). Evans and English (Citation2002) claim children from poor households are exposed to more psychosocial stress than others. These findings suggest an important need to control for household income status when investigating school dropouts.
Studying aspects of household characteristics bearing on education, Ermisch and Francesconi (Citation2000, Citation2001) explore the relationship between employment and education of parents and subsequent education of their children. They find that greater time and money made available to a child would lead to a rise in her educational attainment. Children with a working mother during their early stages of life have lower educational attainment than children whose mothers spend more time at home with them. For financially constrained parents, it is unclear whether this means that their time at home is more important than money and other resources generated by more parental time at work away from home. These studies on implicit calculation of the benefit and cost of schooling are particularly important to identify potential predictors.
Haveman and Wolfe (Citation1993) show that parents’ education is a powerful factor affecting their children’s educational attainment. Highly educated mothers motivate their children by instilling in them a drive for education. Although father’s education is significant, the mother has a greater influence on the values that children find important later in their life. Lack of parental education can deprive children of these values and obstruct their path to learning.
There are a number of studies focusing on the correlation between family structure and educational attainment. Boggess (Citation1998) shows that a mother-headed household or mother-stepfather arrangement lowers schooling due to resource allocation that is relatively unfavorable to education. Such an effect, however, turns insignificant after family economic status is controlled for. It seems that income and wealth outweigh the disadvantages of a single parent in education. A single parent family is perhaps the cause of a lower economic status.
Garasky (Citation1995) argues that the impact of family structure on education varies depending on the age of a child. During early childhood, a stable family promotes learning. As the child matures, the type of family structure becomes less critical to attainment because of greater social interaction. As children move into adulthood, they are better equipped to handle separation or divorce, or handle the possibility of living in single headed households themselves. Religion, race, and region, among other factors, also seem to play important roles in determining educational attainment.
Tyler and Lofstrom (Citation2009) have called for a systematic investigation of the underlying causes of school dropouts. They argue that a student’s decision to drop out is influenced by several complex factors and is the outcome of prolonged disengagement from school. School dropout in Bangladesh has been studied by Sabates, Hossain, and Lewin (Citation2010) using a sample of 9,047 children aged between 4 and 15 during 2007 and 2009. Children who dropped out were on average older, had been at the same grade more than one year, belonged to lower income families, had parents with lower educational attainments, performed more household responsibilities, and significantly received less support from parents for their school work.
Overall, the evidence suggests that parental socioeconomic status may have a causal effect on children’s educational outcomes. Yet, most studies establish association of the explanatory variables with schooling rather than causation. Rigorous research on finding causes of dropouts is scarce particularly on developing countries.
We look at data from a large recent survey to study the impact of household conditions on whether the child succeeds in obtaining decent educational attainment or drops out at some point during school. The study adds to the literature by examining household conditions for schooling in the growing but poor country of Bangladesh that has a relatively high dropout rate. A government of Bangladesh report (2010) puts the survival rate to the final grade of elementary school at 45 percent in 2009 implying 55 percent failed to complete their primary education.
3. Data and methodology
3.1. Data
We use data from the 2011 Demographic and Health Survey (DHS) for Bangladesh. The survey covers urban as well as rural population to collect information on demographic pattern, asset ownership, access to public services, housing characteristics, and education and health conditions. A household is defined as a person or a group of people, related or unrelated to each other, who live and share meals together in the same dwelling unit. Other secondary sources were also utilized to collect data.
The survey is based on a two-stage stratified sample of households. In the first stage, 600 Enumeration Areas (EAs) were selected with a probability proportional to the EA size, with 207 clusters in urban areas and 393 in rural areas. A complete household listing operation was then carried out in all the selected EAs to provide a sampling frame for the second-stage selection of households. In the second stage, 17,142 households were given the survey. The data used for this study cover 8,753 households or 51 percent that have one or more children, eligible to attend school, aged 5 to 18 years. The households are selected from the seven regions of Bangladesh. A regional map and the household sample from each region are given in Figure .
3.2. Methodology
We use ordinary fractional logit, beta, least squares (OLS), and probit models to analyze the data. Two variants of the dependent variable of dropout have been used. First, the dropout is calculated as the percentage of all children living in the household who dropped out of school. Since school dropout rate is a continuous dependent variable, bounded by 0 and 100 percent, we use fractional logit, beta regression, and least squares (OLS) regression to check consistency and robustness of our findings. Fractional logit and beta regressions are suitable in analyzing proportions. However, beta regression is restrictive in that it requires that the dependent variable be greater than 0 and less than 1. This contrasts with the nature of our sample which has 39 percent of households having exactly zero dropout and 33 percent that have all of the (one or more) children quitting school.
While a beta regression is feasible if we force an extreme value (0 or 1) to a value that is closest to it within the (0, 1) interval, a fractional logit model is more appropriate for our data. Another variation in data would be to make dropout a binary variable where the value 1 means there is a dropout and the value 0 means none. We can use a probit (or logit) model to analyze a dichotomous variable of this type. A probit regression models the inverse standard normal distribution of the probability of a dropout as a linear combination of predictors.
Regardless of the specification used, the set of explanatory variables for dropout that we consider in our base model includes household-specific demographics and socioeconomic factors together with regional characteristics of the household. Our base model is described by the following equation:
where Region refers to one of the seven administrative regions in the country with Barisal (j = 1) as the reference; Urban is a dummy with urban households receiving the value 1 and rural households 0; the Islam dummy has the value 1 for Muslim households which constitute an overwhelming majority in Bangladeshi population and 0 for all others; MthrAge is a continuous variable for the age of the child’s mother; MthrEdu consists of four dummies for levels of education (no education is the reference level, and others are primary, secondary and higher); FthrAge is a continuous variable for father’s age; FthrEdu has four dummies for levels of education just as for MthrEdu; FthrOccu consists of five types of occupation dummies of which agriculture is the reference occupation, and others are business, service, highly skilled, and “others”; HHAge refers to the age of the household head grouped in four categories with those between 15 and 34 years of age serving as the base and others in the following ranges: 35–54, 55–74, and 75+. Because of great prevalence of joint family arrangements, with high respect accorded to grandparents in Bangladesh, it was necessary to widen the age range and introduce the 75+ category.
Since mother’s age and father’s age in the model are already continuous variables, a difference of few years in the age between household heads was judged to play a more subsidiary role in children’s schooling than if such differences were longer such as decades. HHMale is a sex dummy with a value of 1 if the head is male and 0 if female; NumSons, NumDauters,NumChld5, and FamSize are all continuous variables with actual numbers of sons, daughters, children under 5 years of age, and all members in the household, respectively.
Wealth index is a variable that accounts for the household’s income, living standards, and overall asset and wealth ownership. This variable categorizes households into five wealth quintiles: Poorest, Poorer, Middle, Richer, and Richest. The wealth index is constructed by the DHS Program using the Principal Component Analysis (PCA) and reflects a composite measure of a household’s cumulative living standard—the value is attributed on a scale of 1 to 5 where 5 refers to the richest group. Furthermore, Eleccity is also a binary variable with the value 1 if electric power is available for the household and 0 if not. A large majority of households in Bangladesh still has no access to electric power. Finally, availability of free transportation to school (Bus) is coded as 1 and lack of it as 0.
For the probit model, a binary variable was created to indicate whether the household had one or more children who dropped out of school. If yes, the variable dropout equals 1 and if no, then dropout equals 0.
Table shows the summary statistics for all the variables used in our empirical models.
Table 1. Summary statistics
Table shows that the average number of dropouts is 1.34. The average dropout is almost 50 percent, that is, about a half of the sample households have at least one dropout. The average age of the sample mothers and fathers is 26 and 34, respectively. Around 6 household members live in a typical household. On average, the numbers of boys and girls are about the same, with a difference of less than one percent. Mother’s education (1.39 years) exceeds father’s (1.28 years) by 8 percent. The average wealth index is 2.95 or close to 3 which is in the middle of wealth distribution.
Table A1 in the Appendix A provides the variance inflation factors (VIF) for the regressors used in the empirical model. The VIFs show that none of the variable pairs is too highly correlated and all the VIFs are within the acceptable range. Hence, all regressors can be used in fitting the OLS and probit models without much concern about multicollinearity.
4. Results
We start with a discussion of the results where the dependent variable dropout is measured as a percentage of all school going children who have dropped out of school. The following subsection then analyzes the results for dropout coded as a dummy.
4.1. Results when dropout is continuous
Table provides the OLS results for our model with and without interaction terms. And, Table shows the comparative analyses of the three models used. We examine the possible regional variation in results as well as the effects of economic and social characteristics of the households on the school dropout.
Table 2. OLS model with and without interaction terms
Table 3. OLS, fractional logit and beta models with and without interaction terms
4.1.1. Regions and cities
Regional distribution of the dropout rate shows interesting results. Compared to the base region (Barisal), all others, except for Chittagong for which the coefficient (0.53) has zero explanatory power, have dropout rates that are 10 (Dhaka) to 15 (most others) percentage points higher. Across regions, however, the households in urban areas show a lower dropout than in rural areas by an average of 5.7 points.
4.1.2. Education and occupation
Mother’s education performs better by lowering dropout rate (2.6 percentage points) if she has a secondary education, relative to the base case of no education. Other levels of education are either statistically insignificant or produce a negligible impact. Since the share of highly educated mothers is small, the result effectively suggests that mothers with secondary education, relative to no education or only primary education, are able to keep more of their children at school. Once mother’s education has been controlled for, father’s education, even at the secondary or higher level, does not matter although the variable does have a negative correlation with the dropout rate. On the other hand, father’s occupation explains the dropout differences significantly. In particular, relative to agriculture and related activity which is the reference occupation, business and high-skill work both lower the dropout rates. Having a business (most of the businesses are small) lowers dropout by 4.7 ppts whereas being in the high skill category lowers the rate by 30.0 ppts. It seems as if higher education, which probably is required of most high skill occupations, can matter for dropout reduction only if higher education leads to high skill jobs. Low skill category is insignificant whereas “other” occupations reduce the dropout by 1.9 ppts.
4.1.3. Wealth
When households are divided into five wealth classes of poorest, poorer, middle, richer, and richest with poorest being the reference class, only the top two classes show negative and significant effects on the dropout rate. Even more starkly than in the case of father’s occupation, these two wealth classes have a large difference between them, with the richest class (−54 ppts) showing a 49 ppt lower rate than dropout for the richer class (−4.6 ppts). Interestingly households in the poorer and middle wealth quintiles are insignificant with low t-statistics and the coefficient sizes that are close to zero.
4.1.4. Family demographics
Does having more daughters in the family increase dropouts? Yes, we find the dropout rises by 6.4 ppts for each additional daughter. However, we find no difference between more daughters and more sons since the number of sons also has about the same coefficient (6.9). Once the numbers of sons and daughters are controlled for, other aspects of family demographics mostly do not seem to affect dropouts. These inconsequential factors include Islam as religion, mother’s age, sex of the household head, and the number of children under 5. Also in the regression, two of the three age groups in the variable age of the household head have no significant impact on dropouts. Surprisingly, however, one group of household heads, 75 or older, has a tremendously high dropout percentage of −42 ppts compared to the base group of 15 to 34 years. It is not entirely clear why this age group should have such a large impact when many of the other family characteristics including those of children’s parents have their own controls in the regression. An overwhelming majority of these old people are uneducated. The hypothesis that younger household heads would be associated with fewer dropouts was thus not verified. However, a relevant argument may be that if grandparents are still surviving, their children (school going children’s parents) are relatively young and they care more about their own children’s education than was the case in the earlier decades. Since such data were not collected by the DHS, the test of such a nuanced hypothesis was not possible.
Among the remaining variables in the model, whether a household has electricity at home (65 percent of the households in Bangladesh use electricity) or whether children are provided free transportation to school seems to make no impact on dropouts.
4.1.5. Interactions
It is possible that the marginal effect of a certain variable on dropouts depends on the level of another variable. To explore such interactive effects, we add three interaction terms to the base model: (a) between father’s education and household wealth, (b) between father’s education and father’s occupation, and (c) between father’s occupation and household wealth. The idea was to see whether parental education might affect dropout at different rates depending on the level of wealth of type of occupation. We find, however, that none of the interaction terms provides any further explanatory power and jointly a combination of any two or all three terms fails the F-test. Inclusion of interaction terms, therefore, leaves the coefficients of all other variables to retain their size and significance at about the same levels.
Table presents a comparative assessment of three models: fractional logit, beta, and OLS. All three models are designed to explain the proportion of children who drop out of school, which is a continuous variable taking values anywhere from 0 to 1. The results are similar and hence provide evidence of robustness to model specification. We find that living in a more developed region of the country or in an urban area with high-skill parents will decrease school dropouts. Also, households classified in the richer and richest wealth categories or household heads aged 75 or older have low school dropouts. On the other hand, children living in a larger family or having siblings increase school dropouts. Finally, mother’s or father’s age, father’s education, religion, availability of electricity at home or of school transportation do not influence school dropouts.
4.2. Probit results when dropout is binary
A probit model was also estimated for the dependent variable dropout whose values in data were either 0 for no dropout and 1 for one or more dropouts. Table shows the marginal effects and their significance for the fitted model.
Table 4. Marginal effects from probit model with and without interaction terms
The result for the binary dropout mostly matches with the result for the continuous dropout as discussed in the last subsection. The marginal effects from the probit model indicate changes in the dropout probability in response to a change in explanatory variables. As compared to the reference region of Barisal in the South, the results show a 5 percent greater dropout probability for Dhaka and 6 percent more for all others, except for Chittagong whose coefficient is zero. Under OLS, the results showed a somewhat bigger dropout of 10 percentage points.
While most of the regional coefficients are highly significant, the urban vs. rural location across regions does not provide any further explanatory power to the model. On the other hand, in terms of percentage point changes, mother’s education lowers dropout slightly, by 1.7, if the mother has higher education whereas primary or secondary education seems irrelevant for dropout. This is an interesting difference from the OLS results where only secondary education of the mother seemed to matter for the dropout rate. Father’s occupation also seems to lower the dropout by about 1 percentage point if the occupation is business. Low or high-skilled occupation for the father has no impact on dropouts. Greater the number of sons or daughters, greater is the dropout, whereas more children under five years of age is associated with a marginally smaller dropout. As regards the wealth index, only the households belonging to the middle or higher wealth category show a lower probability of dropout, while the poorer wealth category has a higher dropout probability than is the case with the poorest households.
An important goodness-of-fit measure for the probit model is the percentage of households for which the dropout is correctly predicted (PCP). While Table A2 in the Appendix B gives details, its essence can be described as follows. We use the benchmark of 0.5 (and above) for “success” or correct prediction of dropout, i.e. if the predicted probability for a household is greater than or equal to 0.5 then school dropout is expected to occur in that particular household. The results show that the probit model without the interaction terms correctly predicts the dropout for 80.5 percent of the households, whereas the probit model with the interactions has a success rate of 80.7 percent of the sampled households. As argued earlier, the models with and without interaction terms hardly differ in their predictive power but either way the success rate of the model in tracking the actual data remains high.
Overall, the results for the probit model virtually mirror the OLS results as well. For example, factors that do not seem to matter for the dropout include mother’s or father’s age, father’s education, religion, availability of electricity or of school transportation. These variables are inconsequential regardless of the method used. The OLS and probit do not diverge in sign or significance for most other variables either such as urban-rural location and father’s education.
5. Conclusion
Dropout rates in Bangladesh are high which raise concern about the country’s ability to accumulate human capital for faster economic development. What can be done to stem and reverse the process? This paper has used several alternative model specifications to study the issue. The broader similarity of results across specifications indicates that our results are fairly robust. Whether policy can address dropouts depends on its ability to target social, demographic, and economic factors that have been found important in this study. Examples of such factors are promoting mother’s education up to higher education level, and making it easier to run a business at low interest rates.
There are several unanswered questions in this study that deserve future investigation. Why, for instance, does relatively rich Dhaka have a higher dropout rate than does the division of Barisal to the south? Is it because many members of the poor households migrate to Dhaka, the largest metropolis and capital city, while the relatively young among them quit school to take a job instead so as to contribute to family income? School retention can be a challenge when students who are marginally attached to schooling because of economic reasons often fail the basic competency tests by the end of the year and hence must repeat grades. How does the dropout compare with the dropout rate for an earlier round of DHS and what are the main reasons for the difference? Further, would survey data allow one to identify the effects, for instance, of policy changes made in the mid-1990s that led to substantial increases in female enrollment in schools relative to the counterfactual of no changes in policy? Could a policy mandate raising the minimum age to stay at school above the current minimum of 10 years reduce dropouts if the mandate is combined with an appropriate subsidy scheme? Finally, it would be interesting to apply the model in this paper to other developing countries to see if the factors found influential in Bangladesh remain so in those countries as well.
Acknowledgments
We thank Alok Bohara, Vinish Shrestha, and participants at South Asia Conference, Madison WI, for providing useful comments. We appreciate comments from two anonymous referees that led to substantial improvements in the paper.
Additional information
Funding
Notes on contributors
Nusrat Farah
Nusrat Farah's research interests include economic growth, poverty, education, health, labor, and gender issues. This paper is a part of our research program that studies the evolution of school dropouts in Bangladesh going back up to 2000. Also, we plan to extend our analysis to other developing countries to see if our model can be used to predict dropouts there.
Mukti P. Upadhyay
Mukti P. Upadhyay has studied poverty and inequality closely. His recently published papers include inequality in education and health in a diverse group of developing countries. He also takes keen interest in growth of agricultural productivity and total factor productivity in Asia and Africa, regional trading arrangements in South Asia, women's autonomy in making financial decisions for their families, and several other development issues.
References
- Amuedo-Dorantes, C., & Lopez, M. (2015). Falling through the cracks? Grade retention and school dropout among children of likely unauthorized immigrants. American Economic Review, 105, 598–603.
- Becker, G. (1993). Human capital revisited. In Human capital: A theoretical and empirical analysis with special reference to education (3rd ed., pp. 15–28). Chicago, IL: University of Chicago Press.10.7208/chicago/9780226041223.001.0001
- Boggess, S. (1998). Family structure, economic status, and educational attainment. Journal of Population Economics, 11, 205–222.10.1007/s001480050065
- Bruns, B., Filmer, D., & Patrinos, H. (2011). Making schools work. World Bank. Retrieved from http://siteresources.worldbank.org/EDUCATION/Resources/278200-1298568319076/makingschoolswork.pdf10.1596/978-0-8213-8679-8
- Devkota, S., & Upadhyay, M. (2016). How does education inequality respond to policy: A method and application to survey data from Albania and Nepal. Journal of Economic Studies, 43, 166–177.10.1108/JES-09-2014-0156
- Dewey, J. (1899). The school and society. Chicago, IL: University of Chicago Press.
- Duncan, G. J., & Brooks-Gunn, J. (Eds.). (1997). Consequences of growing up poor. New York, NY: Russell Sage Foundation.
- Duncan, G. J., Brooks-Gunn, J., & Klebanov, P. K. (1994). Economic deprivation and early childhood development. Child Development, 65, 296–318.10.2307/1131385
- Ermisch, J., & Francesconi, M. (2000). The effect of parents’ employment on children’s educational attainment (Working Paper No. 2002-21). Institute for Social and Economic Research. Colchester: University of Essex. Retrieved from https://www.iser.essex.ac.uk/files/iser_working_papers/2002-21.pdf
- Ermisch, J., & Francesconi, M. (2001). Family matters: Impacts of family background on educational attainments. Economica, 68, 137–156.10.1111/ecca.2001.68.issue-270
- Evans, G. W., & English, K. (2002). The environment of poverty: Multiple stressor exposure, psychophysiological stress, and socioemotional adjustment. Child Development, 73, 1238–1248.10.1111/cdev.2002.73.issue-4
- Garasky, S. (1995). The effects of family structure on educational attainment American Journal of Economics and Sociology, 54, 89–105.10.1111/ajes.1995.54.issue-1
- Haveman, R., & Wolfe, B. (1993). Children’s prospects and children’s policy. Journal of Economic Perspectives, 7, 153–174.10.1257/jep.7.4.153
- Pritchett, L. (2013). Education ain’t learning (Chapter 2). Retrieved from https://books.google.com/books?isbn=1933286784
- Sabates, R., Hossain, A., & Lewin, K. M. (2010). School drop out in Bangladesh: New insights from longitudinal evidence. CREATE Research Monograph 49, Brighton: University of Sussex.
- Torres, C. A. (1990). The politics of non-formal education in Latin America. New York, NY: Paeger.
- Tyler, J. H., & Lofstrom, M. (2009). Finishing high school: Alternative pathways and dropout recovery. America’s High Schools, 19, 77–103.
Appendix A
Table A1. Variance Inflation Factors (VIFs) for all regressors
Appendix B.
Table A2. Percent of correct predictions (PCP) under probit model