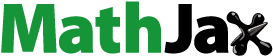
Abstract
This study has evaluated the impact of adoption of improved groundnut seed on the well-being of the farmers of Eastern Ethiopia using a cross-sectional data collected from 301 sample households. To address this objective, both descriptive and econometric analysis methods were employed. In the econometric analysis, Propensity Score Matching was used to measure the impact of adoption of improved groundnut seeds on well-being measured as expenditure per adult equivalent. The results of the study have indicated that adoption of improved groundnut seeds has a positive and significant impact on the welfare of the farmers. Therefore, socioeconomic variables should be addressed to improve the adoption of improved groundnut seeds, which in turn increases the welfare of groundnut producing farmers.
Public Interest Statement
Groundnut contributes to the attainment of nutrition security, maintenance of soil quality, and generation of cash to farm households. To this end, breeders in Ethiopian Universities and Research Centers generate improved seeds that are drought and disease resistant; are resistant to drought and disease and improves productivity and the quality of oil. After generating the improved seeds, it will be availed for farmers and it is up to them to choose whether to adopt the improved seed or stick with the old one. Hence, in this research, we examined why some farmers choose the improved seed—why others don’t. Even for those who adopt the improved seed, the performance of the seeds in the field might not be similar with the results obtained in the research site. Therefore, we also computed the welfare improvement that resulted from the utilization of improved seeds—showing the importance of using improved seeds.
1. Introduction
All developing regions have reached the Millennium Development Goal of reducing poverty by half between 1990 and 2015 except Africa (United Nations, Citation2015). In Africa, though the share of people living on less than $1.90 a day fell from 56% in 1990 to 43% in 2012, the number of poor increased from 284 to 388 million in the same period (Beegle, Christiaensen, Dabalen, & Gaddis, Citation2016). As most of the poor inhabit in rural areas and almost all of them depend on agriculture for their living, the path out of poverty in this continent relies on the performance of the agricultural sector. As emphasized by Department for International Development (Citation2003), a 1% increase in agricultural productivity could reduce the percentage of poor people living on less than $1 a day by between 0.6 and 2% and no other economic activity that generate the same benefit to the poor. Nevertheless, the agricultural sector’s growth in the continent has dawdled behind population growth even in 2001–2010, which was a period globally perceived as a “decade of growth” (Diao, Thurlow, Benin, & Fan, Citation2012).
Particularly there was no progress in Sub-Sahara Africa (SSA) as productivity has not been improved significantly (Tittonell & Giller, Citation2013). The productivity growth in this region is the lowest even compared with other developing regions. Over the past four decades, agricultural productivity growth in the region averaged only 2.4% while the productivity of the rest of developing world improved by 4.0% (Dzanku, Jirström, & Marstorp, Citation2015). SSA is the only developing region not to have experienced significant declines in undernourishment and about one-third of the people in this region are food insecure (de Graaff, Kessler, & Nibbering, Citation2011). Productivity growth in agriculture is thus, viewed as the only pathway to long-term and pro-poor economic development in SSA (Dawson, Martin, & Sikor, Citation2016).
Among the countries from this region, Ethiopia remains to be one of the poorest countries in the world and nearly 30% of households in the country are in extreme poverty (World Bank, Citation2015). More than 30% of the population is undernourished and prevalence of food inadequacy is 41.3% (FAO, Citation2015). Thirty-six percent of Ethiopian farming households are engaged in subsistence farming, living on less than two USD per day (MoA & ATA, Citation2014). Therefore, improving the agricultural production and productivity in the country is not a matter of choice.
Numerous studies, including (Asfaw, Shiferaw, Simtowe, & Lipper, Citation2012; Feleke & Zegeye, Citation2006; Getacher, Mesfin, & Gebre-Egziabher, Citation2013; Teklewold, Kassie, Shiferaw, & Köhlin, Citation2013) argued that the effective way to improve agricultural productivity is through adoption of improved technologies. In addition to productivity improvement, technology adoption can lower per unit cost of production, increase the supply of food, and raise incomes of adopting producers (Moyo, Norton, Alwang, Rhinehart, & Deom, Citation2007). It can also improve nutritional status (Kumar & Quisumbing, Citation2010) and reduces risks of crop failure (Hagos, Jayasinghe, Awulachew, Loulseged, & Yilma, Citation2012). Increasing adoption rates of productivity enhancing technologies is, therefore, essential for boosting crop production and improves the welfare of the rural community. However, the adoption rate of agricultural technologies is very low in Ethiopia (Bingxin, Alejandro, José, & Sinafikeh, Citation2011).
Agricultural technology adoption is one of the most researched areas in Ethiopia (e.g. Ahmed, Citation2015; Teklewold et al., Citation2013). However, most of those studies emphasized on cereals and very few studies have looked at adoption of Legumes in general, and groundnut technologies in particular, though they present an opportunity in reversing the trends in productivity, poverty and food insecurity in SSA (Asfaw & Shiferaw, Citation2010).
Legumes can reduce malnutrition and improve human health, especially for the poor who cannot afford livestock products. For instance, according to the USDA National Nutrient Database for Standard, groundnuts contain 40–50% fat, 20–50% protein, and 10–20% carbohydrates. They are packed with many important B-complex groups of vitamins such as Riboflavin, Niacin, Thiamin, Pantothenic Acid, Vitamin B-6, and Folates. They are also a rich source of minerals like Copper, Manganese, Potassium, Calcium, Iron, Magnesium, Zinc, and Selenium. Groundnuts are also rich in energy, which provide 567 calories per 100 g. This high-energy value, protein content, and minerals make them a rich source of nutrition at a low price.
In addition to nutritional values, it has also environmental advantages for the farmers. Groundnuts improve soil fertility by fixing nitrogen and thereby increasing the productivity of other crops when used in rotation or in intercropping (Ajeigbe et al., Citation2015). The growing demand in both the domestic and export markets could also provide a source of cash for smallholder producers. Despite this fact, the productivity of groundnut is still low in the country at 1.6041 ton/ha (FAOSTAT, Citation2014) and low levels of adoption of productivity enhancing technologies are among the reasons for this level of productivity. Hence, the objective of this study was to identify factors affecting adoption of improved groundnut seeds and to evaluate its impact on the welfare of groundnut producing farmers of Eastern Ethiopia.
1.1. Hypothesis of the research
H1: adoption of improved groundnut seeds has a positive and significant impact on the welfare of the farmers.
H2: The decision by the Groundnut producers of the Eastern Ethiopia to adopt improved groundnut seed is influenced significantly by the plot, household, socioeconomic, institutional and environmental characteristics.
1.2. Profile of groundnut in Ethiopia
Groundnut (Arachis hypogaea L.) also known as peanut is the world’s fourth most important source of edible oil and third most important source of vegetable protein (Govindaraj, Kumar, & Basu, Citation2009; Upadhyaya, Reddy, Gowda, & Singh, Citation2006). It is grown in nearly 100 countries on six continents over 23,712,204 ha of land and developing countries account for over 97% of world groundnut area and 95% of total production (FAOSTAT, Citation2014). The fact that Ethiopia possesses varying climatic conditions results in the cultivation of a wide range oil crops including groundnut.
Though groundnut is introduced to Ethiopia recently in the early 1920s (Daniel, Citation2009), currently it accounts 13.64% of total oil seed produced. According to Central Statistical Agency (Citation2015), in 2014/2015 production year, groundnut covered about 64,643 ha of land at the national level. The total output of groundnut in the same year produced at the national level was 1,037 tonnes. The same source also indicated that 313,564 holders were involved in groundnut production in the same period. The major groundnut producer region in Ethiopia is Oromia region (41,089 ha), followed by Benshangul-Gumuz (14,759 ha) and Amhara (3,161 ha) regional states.
Based on data from FAOSTAT, Table summarizes the groundnut production, the area planted with groundnuts as well as groundnut yield from 2005 to 2014 production period in Ethiopia. In this period, groundnut production has increased from 29,053 tonnes to 103,706 tonnes, which is equivalent to 72% increase. However, this growth should not be attributed to the improvement in productivity alone as land allocated to groundnut production has also increased by 58% in the same period registering average annual growth of 14%.
Table 1. Performance of groundnut in Ethiopia in the last ten years
Within this period, though it lacks consistency, the productivity of the groundnut in Ethiopia has improved remarkably. As indicated in Table , the groundnut productivity growth in Ethiopia is higher than the world average growth and other developing regions. On average, there was annual productivity growth of 6% in Ethiopia within this period and the corresponding figures for the world, Africa, East Africa, and Low Income Food Deficit Countries are 0.8, 0.2, 1.0, and 1.2%, respectively. There is, however, a lot to be done. For instance, the groundnut yield in Egypt in 2014 was twice the Ethiopian yield and the yield for the top major groundnut producing countries Israel, Barbados, and Cyprus were 5, 11, 16 times higher than the yield of Ethiopian, respectively.
Table 2. Groundnut yield growth from 2005 to 2014 in Ethiopia and other developing countries
2. Research methodology
2.1. Description of the study areas
Since Eastern lowland areas of Ethiopia particularly areas such as Babile, Fedis, and Gursum are the major producers of groundnuts for local and commercial consumption (Chala, Mohammed, Ayalew, & Skinnes, Citation2013), this study was under taken in those districts. Babile district lies between 8°9′ and 9°23′N latitude and 42°09′ and 42°55′E longitude. The district has a total area of 3,169.06 km2. It is classified into woinadegaFootnote1 And kola agro-climatic zones, covering about 10 and 90% of the total area of the district, respectively. Fedis district, on the other hand, lies between 8°52′ and 9°14′ N Latitude and 42°02′ and 42°02′ and 42°19′ Longitude with a total area of 1,105.02 km2. About 39% of this district is in woinadega agro ecology and the remaining 61% of the total area of the district is Kolla. Gursum district lies between 9°07′ and 9°32′ N latitude and 42°17′ and 42°38′ E longitude with a total area of 967.31 km2. It is classified into dega, woinadega, and Kolla zones, covering about 15, 35, and 50% of the total area of the district, respectively (Figure ).
2.2. Sampling techniques and sample size
For this study, a multi-stage sampling technique was implemented. In the first stage, three districts namely Babile, Fedis, and Gursum were purposively selected considering their groundnut production intensity. In the second stage, a total of 15 rural kebelesFootnote2 were selected from the three districts mentioned above. Finally, 301 respondents were selected from the selected rural kebeles proportional to the size of groundnut producing formers using simple random sampling technique.
2.3. Method of data collection and data type
Although this principally employed a primary data, both primary and secondary data were collected. The primary data were collected using structured questionnaire that was administered by trained enumerators. In addition to the above way of collecting cross-sectional data from primary source, secondary data were also collected from the bureau of agriculture of the districts and other relevant institutions to verify the cross-sectional data.
2.4. Method of data analysis
To address the objectives of this research, both descriptive and econometric analysis methods were employed. In the descriptive part, measures of central tendency, frequency, and percentages were used; and in the econometric analyses, propensity score matching (PSM) was utilized to examine the impact of adoption of improved groundnut seed on the welfare of the farmers.
2.4.1. Propensity score matching
This technique helps to adjust for initial differences between a cross section of adopters and non-adopters by matching each unit based on similar observable characteristics. An important assumption on which this technique builds is the Conditional Independence Assumption (CIA), which states that selection is solely based on observable characteristics and potential outcomes are independent of treatment assignment (Rosenbaum & Rubin, Citation1983). Under the assumption that initial differences between the two groups determining participation are captured by observable characteristics, the participants’ counterfactual mean outcome had they not been participated is identified by non-participants’ mean outcome.
Besides CIA, another condition in PSM is the common support requirement, which ensures that individuals compared from the two groups are, to begin with, comparable. Specifically, it ensures individuals with the same observable characteristics have a positive probability of being in both groups (Heckman, Ichimura, & Todd, Citation1998). This requirement can be imposed such that estimation is performed on individuals that have common support. The average treatment effect on the treated (ATT) is therefore, given by the difference in mean outcome of matched adopters and non-adopters that have common support conditional on the propensity score. The mean impacts of improved groundnut seed will therefore, be given by:(1)
(1)
where is the treatment effect (effect due to adoption of improved groundnut seed), Y is the outcome (consumption per adult equivalent), Di is whether household i has got the treatment or not (i.e. whether a household adopted improved groundnut seed or not). However, one should note that Y (Di = 1) and Y (Di = 0) cannot be observed for the same household at the same time. Depending on the position of the household in the treatment either Y (Di = 1) or Y (Di = 0) is unobserved outcome (called counterfactual outcome). Due to this fact, estimating individual treatment effect τi is not possible and one has to shift to estimating the average treatment effects of the population than the individual one. Most commonly used average treatment effect estimation is the ATT on the treated, and specified as:
(2)
(2)
As the counterfactual mean for those being treated, E[Y(0)|D = 1] is not observed, one has to choose a proper substitute for it in order to estimate the ATT. By rearranging, and subtracting E[Y(0)|D = 0] from both sides, one can get the following specification for ATT.(3)
(3)
Both terms in the left-hand side are observables and ATT can be identified, if and only if E[Y(0)|D = 1] − E[Y(0)|D = 0] = 0. i.e., when there is no self-selection bias.
There is no way to get adopter with the same score as its counterfactual(s) since the propensity score is a continuous variable. Thus, there is need to search for counterfactual(s) that matches with each adopter depending on its propensity score. Different matching methods are used in the literature. Different matching (Caliper, Kernel, and Nearest Neighbor) estimators, using different algorithms, were used to test the robustness of the differences in ATT among the groups, practitioners, and non-practitioners in the common support region.
2.4.2. Measuring welfare
Welfare can be measured from two perspectives: income and expenditure. Mostly rich countries measure welfare using the former perspective, while poor countries use the expenditure approach. This is because, income is relatively easy to measure in rich countries, while expenditure is complex and hard to quantify. Contrary to this, in less-developed countries income is hard to measure as much of it comes from self-employment, while expenditure is more straightforward and hence easier to estimate (Haughton & Khandker, Citation2009). Provided the information on consumption obtained from a household survey is detailed enough, in this study, welfare is measured by consumption expenditure and is expressed in per adult equivalent terms.
3. Result and discussion
3.1. Characteristics of sample respondents
Out of the total households considered for this study, about 35% of them have adopted improved seed. Table summarizes household, socioeconomic, institutional, and plot characteristics between adopters and non-adopters.
Table 3. Characteristics of sample respondents
Of the total sample households, about 85% were male-headed household. Age of the total sample respondents ranged from 17 to 80 years with a mean of about 38 years. As this study was undertaken in the rural parts of the country, it is not surprising that 64.8% of the sample household heads did not attain formal schooling. On average, the sample respondents have been engaged in farming for 20 years and specifically they have cultivated groundnut for the 17 years.
Regarding socioeconomic variables, the mean adult equivalentFootnote3 family size of the sampled households was 5.47. The mean annual farm income was 9,518.41 ETB.Footnote4 Eighteen percent of respondents were also engaged in non/off farm activities like petty trade, remittance, pension, wage, and rent from assets. On average, respondents own 3.437 units of livestock in terms of tropical livestock unit. The size of land owned ranges from 0.5 to 24 quxiFootnote5 with an average size of 7.744 quxi.
As far as institutional variables are concerned, 55% of the respondents were members of agricultural (input or marketing) cooperatives. Nearly 40% of respondents have social responsibility such as a security guard (Militia), member of the local administration and religious or traditional leadership. Except 5% of the respondents, all of them indicated that they get the extension service though the frequency differs. Frequency of extension contact ranges from zero to 288 days with the mean of 66.31 days per year and 85% of the respondents indicated that the extension service they received from the extension agents is sufficient. Out of the total of the respondents, 63.5% of them got training specific to groundnut production. The sample respondents are, on average, 5.22 and 1.3 km far from market and farmers training center, respectively.
Concerning the plot characteristics, about 79% the plot are found in the kola agro ecological zone. The mean size of the plot was 3.249 quxi. The plots are, on average, 0.109 km away from where the respondents are living. Ninety-eight percent of the plots considered for this study are owned and operated by the respondents and the remaining are either rented in or shared in. About 70% of the plots got good fertility status based on perception of the farmers and 81.3% of them are perceived to be plainly in their slope.
3.2. Econometric results
3.2.1. Adoption of improved groundnut seeds
This sub-section presents the result of the logit regression model, which was used to estimate the propensity score for matching adopter households with non-adopters. The model adequately fitted the data (LR χ2(16) = 74.35, Prob. >χ2 = 0.0000).
The result of the study indicated that the educational level of the household head has a positive effect on the adoption of improved groundnut seed. Educated farmers have more exposure to the external environment and accumulated knowledge through formal learning, which enhances their ability to perceive, interpret, and respond to new events in the context of production. Similar results also found in the work of Wainaina, Songporne, and Matin (Citation2014).
An increase in the groundnut production experience of a household head has a positive relationship with adoption of improved seed. Experienced farmers have knowledge, skills, and attitudes with farming that enables them to easily understand and be familiar with the benefits of the technology better than less experienced counterpart.
The negative relationship between square of age of the household head and adoption of improved seed indicated that younger farmers are more interested to adopt improved groundnut seeds than older and very young farmers are. The knowledge, and the skills as well as the physical capability of farmers are likely to increase as their age increases. However, this tends to decrease after a certain age level. Moreover, older farmers may be more interested in following traditional methods that are familiar to them rather than adopting new practices (Assefa & Gezahegn, Citation2004).
Extension access is a necessary catalyst to technology adoption as it is the major source of agricultural information in Ethiopia. The study also indicated a positive relationship between extension contact and adoption of improved seed. Farmers who have a frequent contact with extension agents have more information that would influence farm household’s demand for new technologies.
Training regarding groundnut production is also found to have a positive relationship with adoption of improved seed. The result is credible as training increases the awareness of farmers and exposes them to new ideas and information about the productivity of inputs, opportunities, input and output management, and prudent handling of cash.
Plot size is also related to adoption of improved seed positively. The same result was also found in the works of Asfaw, Shiferaw, Simtowe and Haile (Citation2011) (Table ).
Table 4. Results of logit regression model estimation
3.2.2. Impact of groundnut seed adoption on consumption per adult equivalent
The common support region in the binary adoption equation, where the values of propensity scores of both treatment and comparison groups can be found, is given in the range between 0.09297288 and 0.94036858. Figure depicts the distribution of households with respect to the estimated propensity scores. The figure shows that most of the treatment households were found on the right side near to middle and partly in the middle while most of control households were found on the left side of the distribution and partly in the middle of the distribution.
Furthermore, Figures and showed that the distribution of estimated propensity scores before and after the imposition of the common support condition for adopter and non-adopter households, respectively. As depicted in these Figures, most of the adopter households had propensity scores around 0.5 while majority of the non-participant households had propensity scores around 0.2.
3.2.3. Estimation of average treatment effect of adoption of improved groundnut seed
Table reports the estimation results for the average treatment effect on the treated (ATT) of the outcome variable using PSM techniques under different matching estimators. In this case, the logarithm value of the annual consumption per adult equivalent was considered as the outcome variable. The impact estimates indicated that adoption of improved groundnut seeds has a positive and significant impact on the welfare of the farmers measured in annual consumption expenditure per adult equivalent. Using the nearest neighbor (NN) algorism, improved groundnut seed adopters were found to have 8.6% higher consumption per adult equivalent than the non-adopters counter factual. Applying a radius algorithm, the result also showed that the adopters’ expenditure is 7.8% higher. Similarly, the stratification algorithm showed that there is a significant difference, amounting to 7.9%, in expenditure per adult equivalent between practitioners and non-practitioners.
Table 5. Estimates of matching methods to measure impact of improved groundnut seeds on household livelihood
4. Conclusion and recommendations
This study has evaluated impact of adoption of improved groundnut seeds and welfare of the rural community using data collected from the Eastern Ethiopia and found evidence that adopters of improved groundnut seed have more consumption per adult equivalent than their non-adopter counterfactual. This confirms the potential direct role of improved groundnut seed adoption on improving rural household welfare. Therefore, the government and other stakeholders should support groundnut producing farmers so as to increase the adoption rate of seed technology by tackling constraints affecting the adoption of the technology.
Accordingly appropriate and adequate extension services should be provided as extension services are the main instruments used in the promotion of demand for modern technologies. Among the several reasons that could explain the negative effect of age on the adoption is the fact that older farmers tend to stick to their old production techniques and are usually less willing to accept change. Therefore, development agents and other participants should aware the elders about the benefits of adopting improved varieties through practical demonstration.
Education, training, and farming experience are also found to be crucial factors in determining the farmers’ decision to adopt the technology. This underscores the importance of human capital development through improving farmers’ access to agricultural knowledge, skill, and experience. Thus, government and other stakeholders have to give due attention to training farmers through strengthening and establishing both formal and informal types of framers’ education, farmers’ training centers, technical and vocational schools. Besides, development agents, local leaders, and other participants should create the room for experience sharing among farmers regarding the importance of improved technologies. The size of groundnut plots also has a positive relationship with adoption of improved seeds. Though the Ethiopian constitution prohibits sale of rural land, farmers can increase their plots by either sharing or renting in.
Acknowledgment
We are also thankful to the Haramaya University for its logistical support.
Additional information
Funding
Notes on contributors
Musa H. Ahmed
Musa H. Ahmed is staff member of Haramaya University. He has MSc in Agricultural Economics. He has experience in productivity analysis and impact evaluation.
Hiwot M. Mesfin
Hiwot M. Mesfin is a lecturer at Haramaya University. She has BSc in Agricultural Economics and MSc in Rural Development. She is the team leader for the socioeconomic research group of the national groundnut project. She has experience in gender and technology adoption researches as well as value chain analysis.
Seltene Abady
Seltene Abady is an agronomist and coordinator for National groundnut project at Haramaya University.
Wendmagegn Mesfin
Wendmagegn Mesfin is a PhD candidate at the United Nations University-MERIT. His research engagements are the areas of smallholder farming, risk analysis and vegetable production.
Amare Kebede
Amare Kebede is a Seed Science and Technology expert in the school of plant sciences at Haramaya University. We utilize our expertise to compute the welfare impact of adoption of improved groundnut seeds.
Notes
1. Dega ecological zone lies between 2,300 and 3,200 m altitude, 900 and 1,200 mm/year of rainfall, and average annual temperature of 11.5°C. woinadega lies in the altitude of 1,500–2,300/2,400 m, rainfall of 800–1,200 mm/year, and average annual temperature of 20.0–17.5/16.0°C, Kola’s altitude is from 500–1,500/1,800 m with rainfall of 200–800 mm/year and annual temperature of 27.5–20°C (Ministry of Agriculture, Citation2000).
2. Kebele is the smallest administrative hierarchy in Ethiopia.
3. Refer Appendices A1 and A2 to see how adult equivalent and tropical livestock unit are calculated.
4. One USD was equivalent with 21.21 ETB in the time of data collection.
5. Quxi is a local measurement unit equivalent with 1/8 of a hectare.
References
- Ahmed, M. H. (2015). Adoption of multiple agricultural technologies in maize production of the Central Rift Valley of Ethiopia. Studies in Agricultural Economics, 117, 162–168.10.7896/issn.2063-0476
- Ajeigbe, H. A., Waliyar, F., Echekwu, C. A., Kunihya, A., Motagi, B. N., Eniaiyeju, D., & Inuwa, A. (2015). A farmer’s guide to profitable groundnut production in Nigeria (Technical Report). Patancheru: ICRISAT.
- Asfaw, S., & Shiferaw, B. (2010, 19–23). Agricultural technology adoption and rural poverty: Application of an endogenous switching regression for selected East African Countries. Poster Presented at the Joint 3rd African Association of Agricultural Economists (AAAE), Cape Town.
- Asfaw, S., Shiferaw, B., Simtowe, F., & Haile, M. G. (2011). Agricultural technology adoption, seed access constraints and commercialization in Ethiopia. Journal of Development and Agricultural Economics, 3, 436–477.
- Asfaw, S., Shiferaw, B., Simtowe, F., & Lipper, L. (2012). Impact of modern agricultural technologies on smallholder welfare: Evidence from Tanzania and Ethiopia. Food Policy, 37, 283–295.10.1016/j.foodpol.2012.02.013
- Assefa, A., & Gezahegn, A. (2004). Adoptions of Improved Technologies in Ethiopia (Research Report, 3.). Ethiopian Development Research Institute.
- Beegle, K., Christiaensen, L., Dabalen, A., & Gaddis, I. (2016). Poverty in a rising Africa. World Bank Publications.10.1596/978-1-4648-0723-7
- Bingxin, Y., Alejandro, N., José, F., & Sinafikeh, A. (2011). International Food Policy Research Institute Ethiopia Strategy Support Program II (ESSP II) (ESSP II Working Paper 31). Addis Ababa, Ethiopia.
- Central Statistical Agency [CSA]. (2015). Statistical report on area and crop production, Addis Ababa, Ethiopia. Addis Ababa: Author.
- Chala, A., Mohammed, A., Ayalew, A., & Skinnes, H. (2013). Natural occurrence of aflatoxins in groundnut (Arachis hypogaea L.) from eastern Ethiopia. Food Control, 30, 602–605.10.1016/j.foodcont.2012.08.023
- Daniel, E. (2009). Groundnut research. In Presentation for Workshop (pp. 1–3). Ethiopia: Werer Agricultural Research Center.
- Dawson, N., Martin, A., & Sikor, T. (2016). Green revolution in Sub-Saharan Africa: Implications of imposed innovation for the wellbeing of rural smallholders. World Development, 78, 204–218.10.1016/j.worlddev.2015.10.008
- de Graaff, J., Kessler, A., & Nibbering, J. W. (2011). Agriculture and food security in selected countries in Sub-Saharan Africa: Diversity in trends and opportunities. Food Security, 3, 195–213.10.1007/s12571-011-0125-4
- Department for International Development [DID]. (2003). Agriculture and poverty, reduction: Unlocking the potential (ADFID policy paper). London: Author.
- Diao, X., Thurlow, J., Benin, S., & Fan, S. (Eds.). (2012). Strategies and priorities for African agriculture: Economywide perspectives from country studies. Washington, DC: International Food Policy Research Institute.
- Dzanku, F. M., Jirström, M., & Marstorp, H. (2015). Yield gap-based poverty gaps in rural Sub-Saharan Africa. World Development, 67, 336–362.10.1016/j.worlddev.2014.10.030
- FAO. (2015). Food security indicators. Retrieved from [email protected]
- FAOSTAT. (2014). Retrieved from http://www.faostat.fao.org
- Feleke, S., & Zegeye, T. (2006). Adoption of improved maize varieties in Southern Ethiopia: Factors and strategy options. Food Policy, 31, 442–457.10.1016/j.foodpol.2005.12.003
- Getacher, T., Mesfin, A., & Gebre-Egziabher, G. (2013). Adoption and impacts of an irrigation technology: Evidence from household level data in Tigray, Northern Ethiopia. African Journal of Agricultural Research, 8, 4766–4772.
- Govindaraj, G., Kumar, G. D., & Basu, M. S. (2009). Benefits of improved groundnut technologies to resource-poor farmers: A participatory approach1. Agricultural Economics Research Review, 22, 355–360.
- Hagos, F., Jayasinghe, G., Awulachew, S. B., Loulseged, M., & Yilma, A. D. (2012). Agricultural water management and poverty in Ethiopia. Agricultural Economics, 43, 99–111.10.1111/agec.2012.43.issue-s1
- Haughton, J. H., & Khandker, S. R. (2009). Handbook on poverty and inequality. Washington, DC: World Bank Publications.
- Heckman, J. J., Ichimura, H., & Todd, P. (1998). Matching as an econometric evaluation estimator. Review of Economic Studies, 65, 261–294.10.1111/roes.1998.65.issue-2
- Kumar, N., & Quisumbing, A. R. (2010). Access, adoption, and diffusion: Understanding the long-term impacts of improved vegetable and fish technologies in Bangladesh (Discussion Paper 00995). Washington, DC: International Food Policy Research Institute (IFPRI).
- Ministry of Agriculture [MoA]. (2000). Agro-ecological zonations of Ethiopia. Addis Ababa: Author.
- MoA and ATA. (2014). Ethiopian national agricultural mechanization strategy. Addis Ababa: Author.
- Moyo, S., Norton, G. W., Alwang, J., Rhinehart, I., & Deom, C. M. (2007). Peanut research and poverty reduction: Impacts of variety improvement to control peanut viruses in Uganda. American Journal of Agricultural Economics, 89, 448–460.10.1111/j.1467-8276.2007.00991.x
- Rosenbaum, P. R., & Rubin, D. B. (1983). The central role of the propensity score in observational studies for causal effects. Biometrika, 70, 41–55.10.1093/biomet/70.1.41
- Storck, H., Emana, B., Adnew, B., Borowiccki, A., & Woldehawariat, S. (1991). Farming systems and resource economics in the tropics: farming system and farm management practices of smallholders in the Hararghe Highland (Vol. II). Wissenschaftsverlag Vauk, Kiel, Germany.
- Teklewold, H., Kassie, M., Shiferaw, B., & Köhlin, G. (2013). Cropping system diversification, conservation tillage and modern seed adoption in Ethiopia: Impacts on household income, agrochemical use and demand for labor. Ecological Economics, 93, 85–93.10.1016/j.ecolecon.2013.05.002
- Tittonell, P., & Giller, K. E. (2013). When yield gaps are poverty traps: The paradigm of ecological intensification in African smallholder agriculture. Field Crops Research, 143, 76–90.10.1016/j.fcr.2012.10.007
- United Nations [UN]. (2015). The millennium development goals report 2015. New York, NY: Author.
- Upadhyaya, H. D., Reddy, L. J., Gowda, C. L. L., & Singh, S. (2006). Identification of diverse groundnut germplasm: Sources of early maturity in a core collection. Field Crops Research, 97, 261–271.10.1016/j.fcr.2005.10.010
- Wainaina, P., Songporne, T., & Matin, Q. (2014). Improved seeds, fertilizer or natural resource management? Evidence from Kenya’s smallholder maize farmers. Paper prepared for presentation at the EAAE 2014 Congress Ljubljana, Slovenia.
- World Bank. (2015). Indicators. Washington, DC: Author.