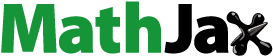
Abstract
This paper examines the determinants of European bank risk-taking during major financial crisis. Using a sample of banks from 26 countries over the period 2005–2015, we examine the nature of the relationship between bank risk, bank characteristics, regulatory, institutional and macroeconomic variables. We use a dynamic panel data modeling structure to capture the potential discrepancies in risk-taking behavior. We subdivide our sample into two sub-samples (East Europe and West Europe countries). We show that macroeconomic and regulatory variables seem to have non-negligible impact on bank risk-taking attitudes. We document that the relationship between bank risk, internal and external factors differs across samples.
Public Interest Statement
The main purpose of this article is to improve the explanatory determinants of credit and insolvency risk behavior in the European banking industry during the financial crisis. The author’s objectives include highlighting the role played by the bank bank-specific and macroeconomic variables in explaining the risk excess. This study is considered the first of its kind conducted in the context of the financial crisis. We use a panel data of 26 European countries over the period 2005–2015. We subdivide our sample into two sub-subsamples (Easteurope and Westeurope countries). The empirical findings show that the relationship between bank risk, microeconomic, macroeconomic and institutional factors differs across samples.
1. Introduction
The eruption of the 2008 banking crisis and the subsequent great recession have stimulated interests in the analysis and understanding of bank stability. The 2007 subprime crisis, widespread rapidly to most international financial markets, has lead to the outbreak of a major global financial turmoil. This major crisis has impacted heavily several international banking industries and led to bankruptcy movement of major international financial institutions such as Lehman Brothers, Bear Stearns, and Merrill Lynch. Several factors have contributed to the emergence of this major financial turmoil in particular financial innovations, executive greedy behavior and a lack of control and supervision. Indeed, due to a deliberate financial liberalization policy, banks have been engaged in a fierce competition characterized by the adoption of new financial products, a sharp credit growth and a savage increase in bank executive compensations.
As a consequence, this major financial crisis, characterized by higher uncertainties, has resurrected the debate about the prevalence of regulatory policy and appropriate regulatory measures to stabilize the system. The recent global financial crisis has emphasized the importance of the early identification of riskier banks, as this allows for solving the problems at a lower cost (Baselga-Pascual, Trujillo-Ponce, & Cardone-Riportella, Citation2015). A better understanding of the determinants of bank risk is needed due to the bank bailout costs associated with the current global financial crisis and the large output losses experienced in several European countries. As stated by Baselga-Pascual et al. (Citation2015), an improved understanding of the determinants of bank risk in the euro area is important for regulators and supervisors interested in benchmarking and validation issues related to the new EU banking rules. However, they may also be of interest to a wide range of financial market participants, including borrowers, shareholders, and bondholders.
Indeed, academics and policy-makers alike have been increasingly interested in the study of the determinants of bank risk-taking behavior (credit risk and insolvency risk). The extent to which banks are vulnerable to risks has been found to be related with a variety of factors, including the banks’ own characteristics such as capitalization and size (Afonso, Santos, & Traina, Citation2014; Baselga-Pascual et al., Citation2015; Berger & DeYoung, Citation1997; Chen, Jeon, Wang, & Wu, Citation2015; Drakos, Kouretas, & Tsoumas, Citation2014; Foos, Norden, & Weber, Citation2010; Williams, Citation2014), bank regulation (Agoraki, Delis, & Pasiouras, Citation2011; Barth, Caprio, & Levine, Citation2004; Laeven & Levine, Citation2009), competition (Agoraki et al., Citation2011; Beck & Demirgüç-Kunt, Citation2013; Boyd & De Nicoló, Citation2005) and the deposit insurance coverage (Angkinand & Wihlborg, Citation2010; Demirgüç-Kunt & Detragiache, Citation2002).
Measurement of bank risk can encompass a variety of dimensions. Since policy makers are primarily concerned about bank failures, we consider bank risk related to the bank’s probability of default. Therefore, we use two well-known accounting-based proxies of bank risk: the non-performing loan ratio (NPLr) and proximity to default (Zscore). The NPLr is defined as the proportion of non-performing loans to gross loans and expresses the quality of a loan portfolio. It has been usually used in the literature as a measure of bank soundness (Agoraki et al., Citation2011; Baselga-Pascual et al., Citation2015; Berger & DeYoung, Citation1997; Delis & Kouretas, Citation2011; Drakos et al., Citation2014; Festić, Kavkler, & Repina, Citation2011; Williams, Citation2014). A higher (lower) value for the NPLr indicates a higher (lower) probability of the bank defaulting. As a complementary indicator, we use the Z-score, which has also been frequently used in the empirical literature to reflect a bank’s probability of insolvency (Agoraki et al., Citation2011; Baselga-Pascual et al.,Citation2015; Demirgüç-Kunt & Huizinga, Citation2010; Drakos et al., Citation2014; Köhler, Citation2015; Konishi & Yasuda, Citation2004; Laeven & Levine, Citation2009; Williams, Citation2014).
The Zscore metric is defined as the number of standard deviations that a bank’s return on assets must fall below the mean for the bank to become insolvent. According to Delis and Staikouras (Citation2011), a higher Zscore indicates that a bank is farther from default. Bikker and Metzemakers (Citation2005) and Laeven and Majnoni (Citation2003) suggested that the Zscore is a better measure of bank risk than the NPLr since non-performing loans are traditionally backward looking and highly pro-cyclical. Delis and Staikouras (Citation2011) stated that this criticism does not concern the Zscore as much since changes in bank riskiness are captured through the variance component of this index. Besides, Agoraki et al. (Citation2011) argued that the Zscore represents a more universal measure of bank risk that captures more than credit risk alone.
This interest lies in the need to insure financial stability and optimal bank management. Reinhart and Rogoff (Citation2010) point out those non-performing loans (NPLs) may be considered as indicators of the eruption of a major banking crisis. They have analyzed the determinants of non-performing loans and have made recourse to explanatory variables such as the level of non-performing loans and bank specific characteristics. It is well-known that bank risks depend largely on the interaction of several factors such as regulatory, macroeconomic, and structural conditions prevailing in the economy as argued in Laeven and Levine (Citation2009); Barrell, Davis, Karim, and Liadze (Citation2010), among others. Salas and Saurina (Citation2002) used macroeconomic as well as microeconomic variables to explain non performing loans of Spanish Commercial and Savings banks from 1985 until 1997.
They found that bank-specific factors may serve as early warning indicators for future changes in non-performing loans (NPLs). The influence of macroeconomic environment on bank risk-taking remains a major issue that has been thoroughly investigated.
This study uses a panel of 280 banks covering the period spanning from 2005 to 2012, which corresponds to a period characterized by excessive volatility in international financial markets, and the occurrence of significant financial and economic events. In this vein, we test the impact of various internal, macroeconomic, regulatory, and institutional variables in order to explore the impact of internal and external environment on the bank risk excess.
This work contributes to the existing literature on the determinants of bank risk-taking behavior in different ways. First, this paper complements the preceding literature by analyzing the bank-specific and macroeconomic factors that influence bank risk of European commercial banks. Second, to control for unobserved heterogeneity as well as for endogeneity issues, we use the generalized method of moments (GMM) estimators, also referred to as the difference GMM and system GMM estimators, developed for dynamic panel data models by Arellano and Bond (Citation1991), Arellano and Bover (Citation1995) and Blundell and Bond (Citation1998). This sophisticated econometric technique has only been used in recent studies on the determinants of bank risk-taking (Agoraki et al., Citation2011; Baselga-Pascual et al., Citation2015; Chen et al., Citation2015; Delis & Staikouras, Citation2011; Drakos et al., Citation2014; Haq & Heaney, Citation2012; Louzis, Vouldis, & Metaxas, Citation2012). Third, we show significant and interactive effects of microeconomic and macroeconomic factors on bank risk-taking not only EU banks taken as a whole but also when adopting a regional subdivision namely two sub-samples regions (East Europe and West Europe) to compare differences on factors that may explain bank risk excess between each region. Peripheral countries are included in West European sample. Recent literature provides evidence that peripheral sovereigns are the main source of instability in the European banking industry (Arghyrou & Kontonikas, Citation2012; Beirne & Fratzscher, Citation2013; Black, Correa, Huang, & Zhou, Citation2013; De Santis, Citation2012 and Gorea & Radev, Citation2014).
Fourth, we exploit the impact of regulatory and institutional environment on the European bank risk-taking. Most authors reviewed macroeconomic environment to the GDP growth and the inflation rate as important variables that can be influence the excess of bank risk. Specifically, we include two indexes (political stability and quality of regulation) individually in the models (Kaufmann, Kraay, & Mastruzzi, Citation2006) which capture different aspects of the institutional environment. We show that these features have a profound influence on the level of bank risk-taking in different European regions and especially on credit risk level.
Fifth, we consider some additional robustness tests that go beyond our main results. We test for robustness of these main results in two ways: we recourse to external data sources for a historical period (2005–2012) and thus we have a sample size that enables us to test for robustness in a panel setting including hence time and country effects. Then, we introduce series of macro institutional indices in our model to test the robustness of the results.
Finally, we have combined both microeconomic and macroeconomic variables to explain the bank risk-taking excess. However, Louzis et al. (Citation2012) used bank specific and macroeconomic variables separately.
In the following section, we propose a literature review of the determinants of bank risk-taking behavior. In section 3, we present our data. Section 4 describes the econometric methodology and empirical model. Section 5 reports the key results relating bank risk-taking to the proposed measures. Section 6 concludes this research.
2. Literature review
Risk-taking behavior in the banking sector has been a central theme in the literature and in particular the behavior of banks in term of risk and information asymmetry. Greuning and Bratanovic (Citation2004) showed that credit risk and insolvency risk are the most important risks of banks. The factors influencing bank risk according to the literature fall into two main groups. First, there is a group of risk determinants that are specific to each bank and that are in many cases, the direct result of managerial decisions. These determinants include capitalization, deposit insurance coverage, size, competition, and regulatory quality. The second group of determinants includes factors relating bank risk to the macroeconomic environment within which the banking system operates, such as economic growth and inflation.
2.1. Bank specific variables
2.1.1. Bank size
The relationship between bank size and bank risk has resulted in a substantial literature. On the one hand, several authors show the existence of a negative relationship between size and risk (Saunders, Strock, & Travlos, Citation1990). On the other hand, González (Citation2005) found a positive and significant relationship between size and bank risk-taking.
This result is consistent with the “too-big-to-fail” argument, indicating that large banks are encouraged to undertake more risky investments as they naturally possess the benefits of a comprehensive security net. Stiroh (Citation2006) argued that major banks have less risk. In addition, Saunders et al. (Citation1990); Galloway, Lee, and Roden (Citation1997); de Haan and Poghosyan (Citation2012) indicate that regulatory protection occurs especially in the case of large banks, under the “too-big-to-fail” theory. Indeed, large banks are encouraged to take more risks than smaller banks in terms of size. These banks have great potential to diversify and reduce their risk. They may also be more sensitive to market movements than those having small size.
2.1.2. Bank capitalization
The capital turns out to be an important variable in determining bank risk-taking. Prior literature uses the ratio of equity to total assets. High capitalization could be used as a cushion, involving a low risk of insolvency. Then, a capital constraint is one of the most important criteria to obtain a robust financial system. Indeed, capital regulation is crucial in the prudential framework. High capital level generates a decrease in the probability of bank risk. Capital requirements may influence risk-taking in various ways. Bolt and Tieman (Citation2004) show that strict capital requirement leads banks to adopt a strict credit policy. Delis, Tran, and Staikouras (Citation2011) argue that bank capitalization is negatively related to bank risk-taking. This finding seems to be intuitive since higher equity capital, as a consequence of stricter capital requirements, implies more prudent bank behavior. Low bank capitalization leads to an increase in non-performing loans. The justification lies on the moral hazard incentives on the part of banks’ managers, who increase their loan portfolio risk when their banks are delicately capitalized as in Berger and DeYoung (Citation1997), among others.
2.1.3. Competition
Several studies have tried to measure the effect of competition on bank risk behavior. Empirical literature showed that banks with large market power are often associated with low credit risk and low default probability. Keeley (Citation1990) found that deregulation measures of the US banking system in the 1970s and 1980s have increased competition, leading to increase in failure risk. Boyd and De Nicoló (Citation2005) suggested that allowing for competition in credit markets is likely to increase stability. Furthermore, Allen and Gale (Citation2004) argued that the relationship between competition and stability in banking sector changed considerably. In addition, Barth et al. (Citation2004) and Schaeck, Cihak, and Wolfe (Citation2006) showed that higher competition is often associated with banking stability. Beck and Demirgüç-Kunt (Citation2013) showed that greater competition is generally associated with a larger impact on banks’ risk-taking activities.
2.1.4. Deposit insurance coverage
Angkinand and Wihlborg (Citation2010) suggested that the existence of explicit deposit insurance coverage schemes reduce market discipline and encourage banks to take excessive risks. Indeed, it is important to protect banks against risks and massive withdrawals with appropriate deposit insurance systems. Demirgüç-Kunt and Detragiache (Citation2002) analyzed the relationship between deposit insurance and banking crises. They found an increase in the likelihood of crises in economies with deposit insurance system. Caprio and Levine (Citation2002) showed that deposit insurance reduce controlling incentives among depositors and debt-holders. This limited responsibility allows shareholders to retain as much profit as possible while recovering part of their losses from deposit insurance fund.
2.1.5. Regulatory quality
Delis and Staikouras (Citation2011) found that supervisory intensiveness reduces bank risk-taking and also reinforces the impact of market discipline in reducing bank risk. Nevertheless, Delis and Staikouras (Citation2011) found that capital requirements, even when reinforced with supervisory activity are not effective in reducing bank risk. Furthermore, Klomp and Haan (Citation2012) found that bank regulations act to reduce bank risk conditional upon ownership structure and size, and that these impacts are most apparent for higher risk banks. Likewise, Laeven and Levine (Citation2009) found that the effect of bank regulations on bank risk is conditional on ownership structure. These authors constructed indices representing the existence of regulations as opposed to the quality of their enforcement. In addition, Williams (Citation2013) attributed a positive relationship between bank capital and risk in Indonesia prior to the Asian financial crisis to poor regulatory governance.
2.2. Macroeconomic variables
Männasoo and Mayes (Citation2009) argued that, during more favorable macroeconomic conditions, a positive relationship between GDP growth and risk assets is acknowledged. Louzis et al. (Citation2012) showed that non-performing loans in the Greek banking system are mainly due to the macroeconomic factors, namely GDP growth.
Jimenez and Saurina (Citation2005) examined the Spanish banking sector from 1984 to 2003.They showed that non-performing loans are determined by GDP growth. Salas and Saurina (Citation2002), Rajan and Dhal (Citation2003) and Fofack (Citation2005) showed that real GDP growth rate is negatively associated with credit risk. Salas and Saurina (Citation2002), Bangia, Diebold, Kronimus, Schagen and Schuerman (Citation2002) and Carey (Citation2002) showed that GDP growth has a significant negative effect on NPLs since the macroeconomic developments make economic agents more able to pay back their debt. Quagliarello (Citation2007) and Louzis et al. (Citation2012) argued that an expansionary phase of the economy features is associated with a low level of NPLs. Demirguç-Kunt and Detragiache (Citation2005) analyzed a sample of 65 of developed as well as developing countries over the period running from 1980 to 1995 using a multivariate Logit model. They showed that inflation is highly significant in increasing the probability of bank risk. Jimenez, Ongena, Peydro and Saurina (Citation2008) used data on Spanish banks for a relatively long period and found that easy monetary policy is associated with higher credit risk. Ioannidou, Ongena, and Peydro (Citation2009) found the same results using monetary policy decision as exogenous variable on the Bolivian banking industry.
3. Data description
We use annual database for commercial banks across 26 European countries over the period spanning from 2005 to 2012. Our data on bank risk determinants are derived from several sources. Bank variables are obtained from the Bankscope database, which provides both listed and unlisted banks. Since we are only focusing on listed banks, the number of banks is reduced to a significant extent. Mainly, the delisted banks are excluded due to the unavailability of data in Bankscope database. Our final sample included 280 commercial banks (see Table ). We use information collected from the World Bank database on banking regulation (Barth, Caprio, & Levine, Citation2001, Citation2006). Besides, World government indicators (WGI) are used to control for macroeconomic and institutional factors that might affect the level of bank risk excess. In addition, the deposit insurance data are obtained from the comprehensive deposit insurance database (Demirguç-Kunt, Kane, & Laeven, Citation2008). The variable definitions and the data sources are described in Table .
Table 1. Sample composition of European countries
Table 2. Definition of selected variables and data sources
The literature proposes several measures of bank risk-taking. The most commonly used are the credit risk defined as non performing loans to gross loans (NPL/GL) and the insolvency risk (Zscore). Therefore, we focus on the ratio of non-performing loans to gross loans as a direct ex-post measure of credit risk (see Salas and Saurina (Citation2002), Barth et al. (Citation2004) and González (Citation2005), among others).
This ratio represents risk excess and is denoted by IRISK, as follows:
(1)
(1)
where NPLi,t and GLi,t denote non-performing loans and gross loans of bank i in period t, respectively. Moreover, the ratio of non-performing loans to gross loans is a commonly measure used in the literature as a proxy for bank default risk (see Covitz, Hancock, & Kwast, Citation2004). Purnanandam (Citation2007) argued that banks with large non-performing loans face the risk of high probability of default. Consequently, they have more incentives to hedge their interest rates. Then, a high value of non-performing loans is an indicator of quality deterioration of bank loan portfolio. In this situation, banks with bad loan portfolios are increasingly urged to hedge their interest rates which may reduce their subsequent risk of overall failure.
In this paper, we use a second dependent variable, which is given by Zscore indicator. This measure of bank risk-taking is used to assess the level of exposure to insolvency risk in financial entities (Blaško & Sinkey, Citation2006; Boyd, Graham, & Hewitt, Citation1993; García-Marco & Robles-Fernández, Citation2008; Hannan & Hanweck, Citation1988; Konishi & Yasuda, Citation2004; Nash & Sinkey, Citation1997). A lower value of Zscore, indicates a higher probability of insolvency. This indicator considers risk of failure to be essentially dependant on the interaction of the income generating capacity, the potential size of return shocks, and the level of capital reserves available to absorb sudden shocks (García-Marco & Robles-Fernández, Citation2008). The Zscore is expressed as follows:(2)
(2)
where ROAit is the return on assets of bank i in period t, Ei indicates expected value,indicates standard deviation, and CAPit is the averaged ratio of equity capital to total assets for the entity i in period t. Recent literature on risk-taking uses extensively the Zscore variable (see Konishi and Yasuda (Citation2004); Laeven and Levine (Citation2009); Angkinand and Wihlborg (Citation2010); Houston, Lin, Lin, and Ma (Citation2010); Delis et al. (Citation2011); Forssbæck (Citation2011); Agoraki et al. (Citation2011), among others).
According to García-Marco and Robles-Fernández (Citation2008), the Zscore indicator reveals the degree of exposure to operating losses, which reduces capital reserves that could be used to offset adverse shocks. Besides, these authors argue that entities with low capital and a weak financial margin relative to the volatility of their returns will score high on this indicator. This indicator is a measure of weakness or strength of financial institutions since it assigns great importance to their solvency and profitability record. Referring to Laeven and Levine (Citation2009) among others, we use the natural logarithm of the Zscore as the insolvency bank risk (LogZscore). According to Roy (Citation1952) and Boyd et al. (Citation1993), among others, Zscore represents a measure of a bank’s distance from insolvency, which is defined as a situation in which losses exceeds equity. A higher Zscore level indicates that bank is more stable.
The dependent variables are represented by the insolvency risk (LogZscore) and the non-performing loans ratio (IRISK). To analyze the effect of bank specific variables, we include bank size (LnTA), loan loss provisions ratio (PROV), bank capitalization ratio (BC), insurance coverage (INSCOV), and the bank competition (HHI). To assess the effect of bank regulation on bank risk, we use the capital regulatory index (Tier 1 ratio). As macroeconomic variables, we use the growth rate (GDP) in order to measure the level of economic development. In addition, we use the inflation rate (INF) as major macroeconomic determinant. Referring to Kaufmann et al. (Citation2006) and the world government indicators (WGI), we use two institutional variables such as political stability (PS) measuring the stability of the political environment and the quality of bank regulation (QR). This indicator measures the ability of the government to formulate and implement regulations that promote competition in the market and the private sector development.
4. Econometric methodology and empirical model
4.1. Econometric methodology
We recall that dynamic models are characterized by the presence of one or more lagged endogenous variables among the explanatory variables.
The general dynamic panel data model may be expressed as follows:
(3)
(3)
where the subscripts i and t denote the cross sectional and time dimension of the panel sample, respectively; yi,t indicates individual i observed in period t; yi,(t-k) is an observation on the same series for the same individual in the k previous periods; is the 1 × k vector of associated polynomials in the lag operator; Xi,t is the k × 1 vector of explanatory variables other than yi,t-k; ηi are an unobserved individual-specific time-invariant effects which allow for heterogeneity in the means of the yit series across individuals; q is the maximum lag length in the model, and υit is a disturbance term which is assumed to be independent across individuals.
A general dynamic panel data specification is given by:
(4)
(4)
with and t = 1, …, T.
Equation (4) is estimated using the Generalized Method of Moments (GMM) as proposed by Arellano and Bond (Citation1991) and generalized by Arellano and Bover (Citation1995) and Blundell and Bond (Citation1998). The GMM estimation of Arellano and Bond is based on the first difference transformation of Equation (4) and the subsequent elimination of individual specific effects:
(5)
(5)
where is the first difference operator.
In Equation (5), the lagged depended variable, is by construction correlated with the error term,
, imposing a bias in the estimation of the model. Nevertheless, yi,t-2, expected to be correlated with
and not correlated with
for
, may be used as an instrument in the estimation of Equation (5), given that ɛi,t are not serially correlated. This suggests that lags of order two and more, of the dependent variable verify the following moment conditions:
(6)
(6)
A second source of bias stems from the possible endogeneity of the explanatory variables and the resulting correlation with the error term. In the case of strictly exogenous variables, all past and future values of the explanatory variable are uncorrelated with the error term, implying the following moment conditions
(7)
(7)
The assumption of strict exogeneity is restrictive and invalid in the presence of reverse causality i.e. when for t < s. For a set of weakly exogenous or predetermined explanatory variables, only current and lagged values of Xi,t are valid instruments and the following moment conditions can be used:
(8)
(8)
The orthogonality restrictions described in Equations (4)–(8) provide the underpinnings of the one-step GMM estimation, which produces, under the assumption of independent and homoscedastic residuals (both cross sectional and over time), consistent parameter estimates. The two-step GMM estimator (Arellano & Bond, Citation1991), used to estimate residuals in order to construct a consistent variance–covariance matrix of the moment conditions, may impose a downward (upward) bias on standard errors (t-statistics) due to its dependence to the estimated residuals. This situation may lead to unreliable asymptotic statistical inference as argued in Bond and Windmeijer (Citation2002) and Windmeijer (Citation2005). This is particularly true in samples with relatively small cross section dimension (see Arellano & Bond, Citation1991; Blundell & Bond, Citation1998).
We test the overall validity of instruments by implementing Sargan specification test, which is asymptotically distributed as chi-square, under the null hypothesis of valid moment conditions (see Arellano & Bond, Citation1991; Arellano & Bover, Citation1995; Blundell & Bond, Citation1998). Furthermore, we assess the fundamental assumption of serially uncorrelated errors, ɛi,t by testing the hypotheses that are not second order auto-correlated.
4.2. Estimation method
We adopt a two step dynamic panel data methodology of as proposed by Arellano and Bond (Citation1991); Blundell and Bond (Citation1998). The GMM technique is used to address the issues of endogeneity, heteroskedasticity, autocorrelation in the data and to monitor individual and time specific effects. The main challenge of this research is to find a valid estimation method. The bank risk heterogeneity caused by the economic or social factors may induce biased estimation unless a special estimation technique is used. Reviewed as a whole, the data signal both cross-sectional (different banks) and longitudinal (different years) characteristics. This type of data can be treated as panel data, which is a specific term to describe a set of data consisting of multiple sites, and periodically observed over a certain time frame.
Panel data models have been mainly used in areas of economics and social studies and the techniques of estimating this type of model have been continuously developed by econometrics. One of the advantages of using the panel data model is that it allows cross-sectional heterogeneity, which is common in bank risk-taking industry. The failure to correct the problems of the heterogeneity, which includes the persistence of bank risk industry and the endogeneity, which is a dynamic mechanism of bank risk, may lead to inconsistent coefficients. To deal with these issues, the dynamic panel data (DPD) models are adopted in this paper. By inclusion of a lagged-dependent variable, the panel data models can allow for dynamic effects (Merkl & Stolz, Citation2009; Salas & Saurina, Citation2002). To account for the persistence of bank risk industry, this paper introduces lagged-dependent variables, extends panel data model easily to models with predetermined or endogenous explanatory variables or instruments. Specifically, dynamic panel model relies on first-differencing or related transformations to eliminate unobserved individual-specific effects, uses lagged values of endogenous or predetermined variables as instrument variables for subsequent first-differences, and can be expected to perform well in situations where the series are close to be autoregressive.
The panel data approach is preferred to operate a richer and more reliable and robust results by comparing the analysis of time series information. A simple linear regression has drawbacks. It does not take into account the problem of persistence as described by Berger, Bonime, Covitz, and Hancock (Citation2000). To specify whether the instruments are valid, we also adopt the specification test suggested by Blundell and Bond (Citation1998). Mainly, we use the Sargan test of over-identifying restrictions, which examines the validity of the instruments. The Sargan test and the second order autocorrelation test (AR2) are the most important criteria used for the estimation and validation of the instruments. Indeed, the Sargan test verifies the independence between the instruments and the residuals (over-identifying restrictions) of the equation which is an essential condition for the validity of instruments. If the null hypothesis of the Sargan test, for validly over-identifying restrictions, cannot be rejected, then the instrumental variables are valid. On the contrary, if we reject the null hypothesis, then the instrumental variables are inappropriate. The second test examines the hypothesis that the errors exhibit no second-order serial correlation.
4.3. Empirical model
As explained in previous sections, both the bank credit risk and bank insolvency risk are persistent and dynamic processes, and are related to their previous conditions. The inclusion of historical bank risk (lagged-dependent variables) information allows the model to capture the dynamic trend of its performance and further to improve model accuracy. At first, this paper focuses on estimation methods for the simple AR(1) model:
(9)
(9)
(10)
(10)
where IRISK is a proxy for the credit risk; α0 and are constant; LogZscore is a proxy for bank insolvency risk, respectively, on series for bank i in time t,
and Zscorei,t−1 denote the one period lagged credit and insolvency risks, Hi,t indicates the internal bank factors; It are some external bank factors, ηiis an unobserved bank specific time-invariant effect which allows for the heterogeneity in the IRISKi,t series across banks, and υi,t is a disturbance term.
Several empirical studies have used this measure of bank credit risk (Agoraki et al., Citation2011; Angkinand & Wihlborg, Citation2010; Brissimis, Delis, & Papanikolaou, Citation2008; Delis et al., Citation2011; González, Citation2005; Louzis et al., Citation2012). This measure determines the quality of bank assets. The second measure represents a more universal measure of bank risk-taking and reflects the insolvency risk position. A key assumption throughout this paper is that the disturbance υi,t is independent across banks. The number of banks for which data are available (N) is assumed to be large whilst the number of time periods for which data are available (T) is assumed to be small, and asymptotic properties are considered as N becomes large with T fixed.
The individual effect (ηi) is treated as being stochastic, which here implies that it is correlated with the lagged-dependent variables ( and Zscorei,t−1) unless the distribution of ηi is degenerate. Initially, this study further assumes that the disturbance (υi,t) is serially uncorrelated. These jointly imply that the explanatory variables
Zscorei,t−1 are positively correlated with the error term (ηi + υi,t) due to the presence of the individual effects, and this correlation does not vanish as the number of individuals in the sample gets larger. In consideration of the issue of this model, this paper uses the method illustrated in Bond (Citation2002). Besides, following Roodman (Citation2009) suggestion, time dummies are included in this model.
5. Empirical results
In this paper, we find some interesting and consistent findings. Descriptive statistics on the different variables used in this analysis are reported in Tables and . We note that the mean IRISK and mean LogZscore are 6.202 and 2.873 (global sample) and 5.914 and 3.167 (south Europe), respectively. The standard deviations are 8.167 and 1.034 (global sample) and 8.387 and 1.111 (south Europe), respectively. Note that the high standard deviations of credit and risk variables indicate the existence of substantial cross-sectional variation in the bank risk levels of European commercial banks. These summary statistics are similar to those reported by Laeven and Levine (Citation2009), in looking at a smaller sample (287 banks in 33 countries). They report a mean LogZscore of 2.85 and a standard deviation of 0.99. The macroeconomic variables (GDP and Inflation rate) reflect both crisis and normal periods. In particular, their minimum and maximum values suggest the volatility of the country’s economy during the study period. The same conclusions are observed for the mean IRISK and the mean LogZscore of the East and West banks regions.
Table 3. Summary statistics (Full sample full period)
Table 4. Summary statistics (East Europe and West Europe regions)
Empirical results are rather robust as shown in Tables –. We conclude some interesting and consistent findings. Firstly, bank size has a negative and significant effect on credit risk. Indeed, the increase in bank size generates a decrease in credit risk. This result implies that banks with larger sizes are not considered in controlling the insolvency risk in the global sample of European banks. This relationship is similar to that found by Salas and Saurina (Citation2002) and Hu, Li, and Chiu (Citation2004). Salas and Saurina (Citation2002) found a negative relationship between bank size and non-performing loans and argued that bigger size allows for more diversification opportunities. Hu et al. (Citation2004) showed that bank size is negatively related to non performing loans of commercial banks in Taiwan. Besides, bank capitalization has a significant negative effect on credit risk. This result is likely due to the fact that capitalization decisions of European banks are generally based on the credit risk absorption objective (Aggarwal & Jacques, Citation2001; Murinde & Yaseen, Citation2004; Rime, Citation2001; Van Roy, Citation2003). This finding is similar to that found by Rajan and Dhal (Citation2003) who argued that a high level of capital detention should minimize the probability of bank credit risk.
Table 5. GMM system estimation results (Global sample)
Table 6. GMM system estimation results (East Europe)
Table 7. GMM system estimation results (West Europe)
However, we note a positive impact on the insolvency risk. Indeed, Kanatas and Besanko (Citation1996) and Koehn and Santomero (Citation1980) showed that a high level of capitalization ratio increases insolvency risk. Several recent theoretical studies focus on the effect of competition on bank risk-taking with mixed predictions (see e.g. Allen & Gale, Citation2000; Boyd & De Nicoló, Citation2005). The bank competition index (HHI) seems to be an important factor in reducing the exposure to credit and insolvency risk. Indeed, this index has a negative but insignificant effect on credit risk. This result is similar to that given provided by Agoraki et al. (Citation2011). Results show that provisions and insurance coverage are associated with a higher level of credit and insolvency risk. This finding is similar to that of Hasan and Wall (Citation2004) who show existence of a positive relationship between non performing loans and provisions for a sample of banks from 24 countries between 1993 and 2000. The positive relationship between insurance coverage and bank risk-taking is consistent with the empirical evidence in Kane (Citation2000), Cull, Senbet, and Sorge (Citation2001), Demirgüç-Kunt and Detragiache (Citation2002) and Laeven (Citation2002).
The coefficients of macroeconomic, regulatory, and institutional variables remain negative and have a significantly impact on credit risk, while those coefficients remain positive and significantly influence the insolvency risk. Some other macroeconomic variables, such as GDP growth rate and inflation rate, have a strong negative effect on the level of non-performing loans. Results for East European and West European samples are reported in Tables and . We conclude that determinants of bank risk-taking behavior are similar for both East European and West European regions in part the Peripheral countries. There is a considerable and significant impact of both internal and external bank determinants on bank risk excess. This result can be explained by the emergence of financial crisis which has influenced the banking stability and has generated a high level of bank risk, especially in the European banking industry
A large shank of literature dealing analyzing bank risk highlights the importance of external environment and especially macroeconomic and institutional environment to explain the bank risk excess. Also, recent financial crisis has generated renewed interest into how the institutional environment and regulatory environment influence bank risk-taking. Therefore, there is widespread interest in understanding these factors. As it is expected, bank regulatory variables have a significant effect on bank risk-taking which is consistent with previous studies (Demirgüç-Kunt & Detragiache, Citation2002).
For all macroeconomic variables, the estimated coefficients are statistically significant and have the expected sign compatible with the theoretical arguments surveyed in section (2). The coefficients of macroeconomic, regulatory, and institutional variables remain negative and significantly impact on credit risk, while coefficients of these variables remain positive and significant effect on the insolvency risk. As the crisis worsens in the period after 2007, the environment stability is compromised. Results indicate that there is an insignificant impact of the most control and external variables on the insolvency risk. Note that only capitalization, provisions, and quality of regulation have significant effect on this category of risks. This finding means that a higher (lower) level of provisions generates higher (lower) level of insolvency risk (Hasan & Wall, Citation2004). By estimating the impact of regulatory factors on bank risk such as Tier 1 ratio, we note the existence of a variation in the coefficients according to the region and the risk measure. The GDP growth and inflation rates have a significantly negative impact on the credit risk. In this vein, a higher GDP growth rate lowers credit risk and increases bank soundness.
The relationship between political stability and credit risk as well as between quality regulation and credit risk is positive and highly statistically significant, which means that a strong institutional environment generates higher bank risk excess. Results in Table show that both inflation and GDP variables have a significant impact on bank risk-taking excess. First, there is a negative and significant relationship between inflation and the two measures of bank risk-taking. This result contradicts those of Demirguç-Kunt and Detragiache (Citation2005), Jimenez, Ongena, Peydro and Saurina (Citation2008) and Ioannidou et al. (Citation2009). Nonetheless, the GDP growth rate is positively and significantly related to both credit and insolvency risks. This means that banks tend to substantially increase their risky assets during the economic booming periods.
The results confirm our findings that stronger macroeconomic and institutional environment induce more bank insolvency risk-taking and reducing bank credit risk-taking. We conclude that bank specific factors such as Bank size (LnTA), bank competition (HHI), and bank capitalization (BC) are significant and related negatively to credit risk. This means that low values of all these variables leads to an increase in credit risk-taking. In this line of research, Salas and Saurina (Citation2002) found a negative relationship between bank size and non-performing loans and argue that bigger size allows for more diversification opportunities. Rajan and Dhal (Citation2003) report similar empirical evidence. Indeed, a high level of capital detention should minimize the probability of bank credit risk.
Moreover, we show that macroeconomic variables, specifically real GDP growth rate and inflation rate, have a strong effect and are related negatively to the level of non-performing loans. This data grouping does not provide information on the existence or not of a regional differential in bank risk-taking behavior. Thus, the global analysis assumes the existence of a typical behavior and omit country differences. In the Euro area, monetary policy rates are identical across countries. However, there are significant differences in terms of GDP and inflation rate. In this research, we propose a data regional disaggregation in order to analyze regional differences in terms of bank risk attitudes. Statistically, we subdivide our sample into two sub-samples: East Europe and West Europe.
As it is shown in Tables and related to the East Europe and West Europe samples results, we show the same findings as for global sample. We conclude that determinants of bank risk-taking behavior are similar both for East Europe and West Europe. There is a considerable and significant impact of both internal and external bank determinants on bank risk excess. While, macroeconomic and institutional environment play a significant and a strong effect on bank risk. This result can be explained by the emergence of financial crisis during the studied period which influenced banking stability and generates a high bank risk level of financial institutions in the world and especially in the European banking industry.
Results indicate that there is a strong and significant link between bank risk-taking and variables measuring external environment. Tables clearly indicate that both strength institutional and regulatory variables have a profound effect on the level of bank risk-taking. Indeed, we show a negative and significant coefficients of institutional variable (QR) which provides information on the quality of banking regulation which means that banks are less exposed to risk excess facing a good banking regulatory quality.
6. Conclusion
Our analysis aims to explore and analyze the fundamental determinants of bank risk-taking behavior in the European banking industry. This research explores how bank internal and external factors influence the level of European bank risk-taking. We use a dynamic panel data approach in a two-step system GMM as proposed by Blundell and Bond (Citation1998). The results found are estimated using heteroskedasticity robust standard errors. We have looked at a sample of 280 banks in 26 European countries during 2005 to 2012 and using lagged values as instruments. Results suggest that the estimated relationship between different internal and external factors and bank risk-taking are robust. Nonetheless, the results may be heavily influenced by the structure of the banking industry characterized by large size banks. We point out that, bank capitalization and bank size have negative and significant effects on insolvency risk. In addition, a large size of bank minimizes credit risk.
Also, we find that a more competitive environment help banks to diversify and to face low level of bank credit risk, meaning that competition has a negative effect on risk-taking excess. Consequently, most bank specific variables, used in this study, have a significant impact on the whole sample of European bank risk-taking.
Macroeconomic variables (GDP growth rate and inflation rate) have a strong effect on the non-performing loans ratio (see e.g. Barrell et al., Citation2010; Laeven & Levine, Citation2009).
By subdividing the whole sample into two sub-samples (East Europe, West Europe; Peripheral countries), we take into account regional differences in terms of bank risk-taking behavior. Results indicate that the impact of bank specific factors differs from one region to another. This may be explained by differences in levels of bank regulation, size of the economy, institutional environment, and financial situation. Specifically, estimation results indicate that the coefficients of macroeconomic variables are fairly stable across different regions. Again, results regarding bank risk-taking are robust for both macro- and micro-measures, and the external environment seems to influence the bank risk-taking excess.
Acknowledgements
The authors would like to thank the editor and the anonymous referees for their useful comments and suggestions.
Additional information
Funding
Notes on contributors
Wiem Ben Jabra
Wiem Ben Jabra is an assistant of Finance at the University of Sousse, Tunisia, Institut des Hautes Etudes Commerciales de Sousse, Tunisia, and received his PhD in Management Sciences (since 2014). His research interests are banking and finance, financial crisis, risk management, and financial econometrics.
Zouheir Mighri
Zouheir Mighri is an Assistant Professor of Quantitative Methods at the University of Sousse, Tunisia, and received his PhD in Management Sciences (since 2011). His current research interests are time series econometrics, spatial econometrics, financial econometrics, panel data econometrics, quantitative finance, and empirical economics.
Faysal Mansouri
Faysal Mansouri is a Professor of Quantitative Methods at the University of Sousse, Tunisia, Institut des Hautes Etudes Commerciales de Sousse. He received his PhD in Industrial Economics from the University of Illinois Urbana-Champaign (1992). His research has been published in several journals and frequently, he participates in conferences and meetings. His major research interests lie in the field of entrepreneurship, microeconomics of banking, industrial economics, environmental economics, regional economics, and risk management.
References
- Afonso, G., Santos, J., & Traina, J. (2014). Do “too-big-to-fail” banks take on more risk? Federal Reserve Bank of New York Economic Policy Review, 20, 41–58.
- Aggarwal, R., & Jacques, K. T. (2001). Assessing the impact of prompt corrective action on bank capital and risk. Federal Reserve Bank of New York Economic Policy Review, 23–32.
- Agoraki, M. K., Delis, M. D., & Pasiouras, F. (2011). Regulations, competition and bank-risk taking in transition countries. Journal of Financial Stability, 7, 38–48.10.1016/j.jfs.2009.08.002
- Allen, F., & Gale, D. (2000). Financial Contagion. Journal of Political Economy, 108, 1–33.10.1086/262109
- Allen, F., & Gale, D. (2004). Competition and financial stability. Journal of Money, Credit and Banking, 36, 453–480.10.1353/mcb.2004.0038
- Angkinand, A., & Wihlborg, C. (2010). Deposit insurance coverage, ownership, and banks’ risk-taking in emerging markets. Journal of International Money and Finance, 29, 252–274.10.1016/j.jimonfin.2009.08.001
- Arellano, M., & Bond, S. R. (1991). Some tests of specification for panel data: Monte carlo evidence and an application to employment equations. The Review of Economic Studies, 58, 277–297.10.2307/2297968
- Arellano, M., & Bover, O. (1995). Another look at the instrumental variable estimation of error-components models. Journal of Econometrics, 68, 29–51.10.1016/0304-4076(94)01642-D
- Arghyrou, M. G., & Kontonikas, A. (2012). The EMU sovereign-debt crisis: Fundamentals, expectations and contagion. Journal of International Financial Markets, Institutions and Money, 22, 658–677.10.1016/j.intfin.2012.03.003
- Bangia, A., Diebold, F., Kronimus, A., Schagen, C., & Schuerman, T. (2002). Ratings migration and the business cycle with applications to credit portfolio stress testing. Journal of Banking and Finance, 26, 445–474.
- Barrell, R., Davis, E. P., Karim, D., & Liadze, I. (2010). Bank regulation, property prices and early warning systems for banking crises in OECD countries. Journal of Banking and Finance, 34, 2255–2264.10.1016/j.jbankfin.2010.02.015
- Barth, J. R., Caprio, G., Jr, & Levine, R. (2001). The regulation and supervision of bank around the world: a new database. In R. E. Litan & R. Herring (Eds.), integrating emerging market countries into the global financial system. Brookings-Wharton Pap. Finan. services (pp. 183–240). Washington, DC: Brookings Institution Press.
- Barth, J. R., Caprio, G., & Levine, R. (2004). Bank regulation and supervision: What works best? Journal of Financial Intermediation, 13, 205–248.10.1016/j.jfi.2003.06.002
- Barth, J. R., Caprio, G., & Levine, R. (2006). Rethinking bank regulation: Till angels govern. Cambridge: Cambridge University Press.
- Baselga-Pascual, L., Trujillo-Ponce, A., & Cardone-Riportella, C. (2015). Factors influencing bank risk in Europe: Evidence from the financial crisis. The North American Journal of Economics and Finance, 34, 138–166.
- Beck, T., & Demirgüç-Kunt, A. (2013). Islamic vs. conventional banking: Business model, efficiency and stability. Journal of Banking and Finance, 37, 433–447.10.1016/j.jbankfin.2012.09.016
- Beirne, J., & Fratzscher, M. (2013). The pricing of sovereign risk and contagion during the European sovereign debt crisis. Journal of International Money and Finance, 34, 60–82.10.1016/j.jimonfin.2012.11.004
- Berger, A., Bonime, S. D., Covitz, D. M., & Hancock, D. (2000). Why are bank profits so persistent? The roles of product market competition, informational opacity and regional/macroeconomic shocks. Journal of Banking and Finance, 24, 1203–1235.10.1016/S0378-4266(99)00124-7
- Berger, A., & DeYoung, R. (1997). Problem loans and cost efficiency in commercial banks. Journal of Banking and Finance, 21, 849–870.10.1016/S0378-4266(97)00003-4
- Bikker, J. A., & Metzemakers, P. (2005). Bank provisioning behaviour and procyclicality. Journal of International Financial Markets, Institutions and Money, 15, 141–157.10.1016/j.intfin.2004.03.004
- Black, L., Correa, R., Huang, X., & Zhou, H. (2013). The systemic risk of European banks during the financial and sovereign debt crisis. Journal of Banking and Finance, 63, 107–125.
- Blaško, M., & Sinkey, J. (2006). Bank asset structure, real-estate lending, and risk-taking. The Quarterly Review of Economics and Finance, 46, 53–81.10.1016/j.qref.2004.11.002
- Blundell, R. W., & Bond, S. R. (1998). Initial conditions and moment restrictions in dynamic panel data models. Journal of Econometrics, 87, 115–143.10.1016/S0304-4076(98)00009-8
- Bolt, W., & Tieman, A. F. (2004). Banking competition, risk and regulation. Scandinavian Journal of Economics, 106, 783–804.10.1111/sjoe.2004.106.issue-4
- Bond, S. (2002). Dynamic panel data models: a guide to micro data methods and practice. Portuguese Economic Journal, 1, 141–162.
- Bond, S., & Windmeijer, F. (2002). Finite sample inference for GMM estimators in linear panel data models: A comparison of alternative tests. London: Mimeo, Institute for fiscal studies.
- Boyd, J. H., & De Nicoló, G. (2005). The theory of bank risk taking and competition revisited. The Journal of Finance, 60, 1329–1343.10.1111/j.1540-6261.2005.00763.x
- Boyd, J. H., Graham, S. L., & Hewitt, R.S. (1993). Bank holding company mergers with nonbank financial firms: Effects on the risk of failure. Journal of Banking and Finance, 17, 43–63.10.1016/0378-4266(93)90079-S
- Brissimis, S. N., Delis, M. D., & Papanikolaou, N. I. (2008). Exploring the nexus between banking sector reform and performance: Evidence from newly acceded EU countries. Journal of Banking and Finance, 32, 2674–2683.10.1016/j.jbankfin.2008.07.002
- Caprio, G., & Levine, R. (2002, April 5). Corporate Governance of Banks: Concepts and International Observations. Paper Presented in the Global Corporate Governance Forum research Network Meeting.
- Carey, M. (2002). A guide to choosing absolute bank capital requirements. Journal of Banking and Finance, 26, 929–951.
- Chen, M., Jeon, B. N., Wang, R., & Wu, J. (2015). Corruption and bank risk-taking: Evidence from emerging economies. Emerging Markets Review, 24, 122–148.10.1016/j.ememar.2015.05.009
- Covitz, D. M., Hancock, D., & Kwast, M. L. (2004). A reconsideration of the risk sensitivity of U.S. banking organization subordinated debt spreads: A sample selection approach, Federal Reserve Bank of New York. Economic Policy Review, 10, 73–92.
- Cull, R., Senbet, L. W., & Sorge, M. (2001). Deposit insurance and financial development (Policy Research Working Paper No. 2221). Washington DC: World Bank.10.1596/prwp
- de Haan, J., & Poghosyan, T. (2012). Size and earnings volatility of US bank holding companies. Journal of Banking and Finance, 36, 3008–3016.10.1016/j.jbankfin.2012.07.008
- Delis, M. D., & Kouretas, G. P. (2011). Interest rates and bank risk-taking. Journal of Banking and Finance, 35, 840–855.
- Delis, M. D., & Staikouras, P. (2011). Supervisory effectiveness and bank risk. Review of Finance, 15, 511–543.
- De Santis, R. A. (2012). The euro area sovereign debt crisis: Safe haven, credit rating agencies and the spread of the fever from Greece, Ireland and Portugal (Unpublished Working Paper. ECB Working Paper No. 1419).
- Delis, M. D., Tran, K. C., & Staikouras, E. G. (2011). Quantifying and explaining parameter heterogeneity in the capital regulation-bank risk nexus. Journal of Financial Stability, 8, 57–68.
- Demirgüç-Kunt, A., & Detragiache, E. (2002). Does deposit insurance increase banking system stability? An empirical investigation. Journal of Monetary Economics, 49, 1373–1406.10.1016/S0304-3932(02)00171-X
- Demirgüç-Kunt, A., & Huizinga, H. (2010). Bank activity and funding strategies: The impact on risk and returns. Journal of Financial Economics, 98, 626–650.10.1016/j.jfineco.2010.06.004
- Demirguç-Kunt, A., Kane, E. J., & Laeven, L. (2008). Deposit insurance around the world: Issues of design and implementation (1st ed., Vol. 1). The Mit Press10.7551/mitpress/9780262042543.001.0001
- Demirgüç-Kunt, A., Karacaovali, B., & Laeven, L. (2005). Deposit insurance around the world: A comprehensive database (Policy Research Working Paper No. 3628). Washington, DC: The World Bank.
- Drakos, A. A., Kouretas, G. P., & Tsoumas, C. (2016). Ownership, interest rates and bank risk-taking in Central and Eastern European countries. International Review of Financial Analysis, 45, 308–319.
- Festić, M., Kavkler, A., & Repina, S. (2011). The macroeconomic sources of systemic risk in the banking sectors of five new EU member states. Journal of Banking and Finance, 35, 310–322.10.1016/j.jbankfin.2010.08.007
- Fofack, H. (2005). Non performing loan in Sub-Saharan Africa, Causal analysis and macroeconomic implications (World Bank Policy Research Working Paper No. 3769). Washington, DC: World Bank.
- Foos, D., Norden, L., & Weber, M. (2010). Loan growth and riskiness of banks. Journal of Banking and Finance, 34, 2929–2940.10.1016/j.jbankfin.2010.06.007
- Forssbæck, J. (2011). Ownership structure, market discipline, and banks’ risk-taking incentives under deposit insurance. Journal of Banking and Finance, 35, 2666–2678.10.1016/j.jbankfin.2011.02.024
- Galloway, T., Lee, W., & Roden, D. (1997). Banks’ changing incentives and opportunities for risk taking. Journal of Banking and Finance, 21, 509–527.10.1016/S0378-4266(96)00052-0
- García-Marco, T., & Robles-Fernández, M. D. (2008). Risk-taking behaviour and ownership in the banking industry: The Spanish evidence. Journal of Economics and Business, 60, 332–354.10.1016/j.jeconbus.2007.04.008
- González, F. (2005). Bank regulation and risk-taking incentives: An international comparison of bank risk. Journal of Banking and Finance, 29, 1153–1184.10.1016/j.jbankfin.2004.05.029
- Gorea, D., & Radev, D. (2014). The euro area sovereign debt crisis: Can contagion spread from the periphery to the core? International Review of Economics and Finance, 30, 78–100.10.1016/j.iref.2013.10.003
- Greuning, H. V., & Bratanovic, S. B. (2004). Analyzing and managing banking risk: A framework for assessing corporate governance and financial risk. Washington, DC: The World Bank.
- Hannan, T., & Hanweck, G. A. (1988). Bank insolvency risk and the market for large certificates of deposit. Journal of Money, Credit and Banking, 20, 203–211.10.2307/1992111
- Haq, M., & Heaney, R. (2012). Factors determining European bank risk. Journal of International Financial Markets, Institutions and Money, 22, 696–718.10.1016/j.intfin.2012.04.003
- Hasan, I., & Wall, L. D. (2004). Determinants of the Loan Loss Allowance: Some Cross-Country Comparisons. The Financial Review, 39, 129–152.10.1111/fire.2004.39.issue-1
- Houston, J. F., Lin, C., Lin, P., & Ma, Y. (2010). Creditor rights, information sharing and bank risk taking. Journal of Financial Economics, 96, 485–512.10.1016/j.jfineco.2010.02.008
- Hu, J. L., Li, Y., & Chiu, Y. H. (2004). Ownership and nonperforming loans: Evidence from Taiwan’s banks. The Developing Economies, 42, 405–420.10.1111/j.1746-1049.2004.tb00945.x
- Ioannidou, V., Ongena, S., & Peydró, J. (2009). Monetary policy, risk taking, and pricing: Evidence from a quasi-natural experiment (Discussion Paper 2009–31). Tilburg: Tilburg University. Center for Economic Research.
- Jimenez, G., & Saurina, J. (2005). Credit cycles, credit risk, and prudential regulation. Madrid: Banco de Espana.
- Jimenez, G., Ongena, S., Peydro, J. L., & Saurina, J. (2008). Hazardous times for monetary policy: What do twenty-three million bank loans say about the effects of monetary policy on credit risk? (CEPR Discussion Paper, No. 6514). Hoboken, NJ: Wiley-Blackwell.
- Kanatas, G., & Besanko, D. (1996). The regulation of bank capital: Do capital standards promote bank safety? Journal of Financial Intermediation, 5, 160–183.
- Kane, E. J. (2000). Designing financial safety nets to fit country circumstances (Working Paper Boston College). The World Bank. 10.1596/prwp
- Kaufmann, D., Kraay, A., & Mastruzzi, M. (2006). Governance Matters V: Aggregate and Individual Governance Indicators for 1996–2005 (World Bank Policy Research Working Paper No. 4012). Washington, DC: The World Bank.
- Keeley, M. C. (1990). Deposit insurance, risk and market power in banking. American Economic Review, 80, 1183–1200.
- Klomp, J., & Haan, J. (2012). Banking risk and regulation: Does one size fit all? Journal of Banking and Finance, 36, 3197–3212.10.1016/j.jbankfin.2011.10.006
- Koehn, M., & Santomero, A. M. (1980). Regulation of Bank Capital and Portfolio Risk. The Journal of Finance, 35, 1235–1244.10.1111/j.1540-6261.1980.tb02206.x
- Köhler, M. (2015). Which banks are more risky? The impact of business models on bank stability. Journal of Financial Stability, 16, 195–212.10.1016/j.jfs.2014.02.005
- Konishi, M., & Yasuda, Y. (2004). Factors affecting bank risk taking: Evidence from Japan. Journal of Banking and Finance, 28, 215–232.10.1016/S0378-4266(02)00405-3
- Laeven, L. (2002). Bank risk and deposit insurance. The World Bank Economic Review., 16, 109–137.10.1093/wber/16.1.109
- Laeven, L., & Levine, R. (2009). Bank governance, regulation and risk taking. Journal of Financial Economics, 93, 259–275.10.1016/j.jfineco.2008.09.003
- Laeven, L., & Majnoni, G. (2003). Loan loss provisioning and economic slowdowns: Too much, too late? Journal of Financial Intermediation, 12, 178–197.10.1016/S1042-9573(03)00016-0
- Louzis, D. P., Vouldis, A. T., & Metaxas, V. L. (2012). Macroeconomic and bank-specific determinants of non-performing loans in Greece: A comparative study of mortgage, business and consumer loan portfolios. Journal of Banking and Finance, 36, 1012–1027.10.1016/j.jbankfin.2011.10.012
- Männasoo, K., & Mayes, D. G. (2009). Explaining bank distress in Eastern European transition economies. Journal of Banking and Finance, 33, 244–253.10.1016/j.jbankfin.2008.07.016
- Merkl, C., & Stolz, S. (2009). Banks’ regulatory buffers, liquidity networks and monetary policy transmission. Applied Economics, 41, 2013–2024.10.1080/00036840802360245
- Murinde, V., & Yaseen, H. (2004). The impact of basle accord regulations on bank capital and risk behavior: 3D evidence from the Middle East and North Africa (MENA) region. University of Birmingham.
- Nash, R. C., & Sinkey, J. F. (1997). On competition, risk, and hidden assets in the market for bank credit cards. Journal of Banking and Finance, 21, 89–112.10.1016/S0378-4266(96)00030-1
- Purnanandam, A. (2007). Financial distress and corporate risk management: Theory and Evidence. Journal of Financial Economics, 87, 706–739.
- Quagliarello, M. (2007). Banks’ riskiness over the business cycle: a panel analysis on Italian intermediaries. Applied Financial Economics, 17, 119–138.
- Rajan, R., & Dhal, C. D. (2003). Non-performing loans and terms of credit of public sector banks in India: An empirical assessment. Occasional Papers, 24, 81–121.
- Reinhart, C. M., & Rogoff, K. S. (2010). This time is different: Eight centuries of financial folly. Princeton, NJ: Princeton University Press.
- Rime, B. (2001). Capital requirements and bank behaviour: Empirical evidence for Switzerland. Journal of Banking and Finance, 25, 789–805.10.1016/S0378-4266(00)00105-9
- Roodman, D. (2009). How to do xtabond2: An introduction and difference and system GMM in Stata. The Stata Journal, 9, 86–136.
- Roy, A. (1952). Safety first and the holding of assets. Econometrica, 20, 431–449.10.2307/1907413
- Salas, V., & Saurina, J. (2002). Credit risk in two institutional regimes: Spanish commercial and savings banks. Journal of Financial Services Research, 22, 203–224.10.1023/A:1019781109676
- Saunders, A., Strock, E., & Travlos, N. G. (1990). Ownership structure, deregulation, and bank risk taking. The Journal of Finance, 45, 643–654.10.1111/j.1540-6261.1990.tb03709.x
- Schaeck, K., Cihak, M., & Wolfe, S. (2006). Are more competitive banking systems more stable? (IMF Working Paper, WP/06/143). Washington, DC: International Monetary Fund.
- Stiroh, K. J. (2006). New evidence on the determinants of bank risk. Journal of Financial Services Research, 30, 237–263.10.1007/s10693-006-0418-5
- Van Roy, P. (2003). The impact of the 1988 Basle Accord on Banks capital ratios and credit risk taking: An international study (Working Paper). Brussels: European center for advanced research in economics and statistics (ECARES). Université libre de Bruxelles.
- Williams, B. (2013). Income volatility of Indonesian banks after the Asian financial crisis. Journal of the Asia Pacific Economy, 18, 333–358.10.1080/13547860.2013.778159
- Williams, B. (2014). Bank risk and national governance in Asia. Journal of Banking and Finance, 49, 10–26.10.1016/j.jbankfin.2014.08.014
- Windmeijer, F. (2005). A finite sample correction for the variance of linear efficient two-step GMM estimators. Journal of Econometrics, 126, 25–51.10.1016/j.jeconom.2004.02.005