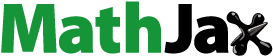
Abstract
This paper applies a sentiment index to check the influence of regional developed countries like Japan and Germany on the sentiments in regional developing countries like Pakistan and Turkey, respectively. The index has been created using a principal component approach with modified proxies. The results indicate that there is a significant influence of developed markets on developing markets also the sentiment index created in this study is good indicator regarding the return pattern of the stock exchange. This study has followed the footsteps of previous studies for methodology and the time period which is used ranged from 29 December 2004 to 31 December 2014. The weekly data has been collected with Wednesday prices so to avoid the white noise, start-of-the week and end-of-the week effect.
Public Interest Statement
This research is beneficial for academicians and practitioners. It is an application of behavioral finance on the real-world scenario. It has explained that stock markets are prone to the sentiments of investors and subject to the influence of other stock markets as well. The study is helpful for the professional investors like brokers as they can consider the sentiments of the markets while investing. Similarly, the academic scholars can also investigate other variables and different relationships with the sentiment index to expand the scope of behavioral finance. This article has unveiled that behavioral finance and economics are complementary subjects with technical and classical finance to study stock market behavior and equity premium puzzles. The scholars interested in the realm of behavioral finance can use our findings in their research on the same subject. This study is beneficial as well as an attempt for the development of the scope of behavioral finance.
1. Introduction
1.1. Traditional vs. behavioral finance
The investment decisions of the financial market participants have been studied under the bipolar views of Traditional Finance and Behavioral Finance. The traditional finance theoretical framework, based upon the Efficient Market Hypothesis (EMH), is led by Fama (Citation1970), which state that prices in the financial markets always reflect all available information, assuming that investors are rational and arbitrage can be done. Contrary to this, behavioral finance mainly focuses on the psychology of investor and limits to arbitrage (Barberis & Thaler, Citation2003).
Behavioral finance emerged as complementary subject, in response to a lot of anomalies which remained unexplained and difficult to be answered in the paradigm of Traditional Finance. For example: Shiller’s (Citation1989) suggested that fluctuations in fundamentals alone cannot account for the aggregate price movements. Bartov, Radhakrishnan, and Krinsky (Citation2000) showed the effect that stock prices underreact to timely announcements of corporate earnings. Cutler, Poterba, and Summers (Citation1991) showed that stock prices experience some of their largest one-day moves. Jegadeesh and Titman (Citation1993) observed a unique trend in prices of individual stocks held for more than a period of one year, i.e. past winners remain winners while past losers remain losers. Still, exceeding one year, this momentum was followed by reversals. Rouwenhorst (Citation1998) observed that European and emerging markets exhibit almost similar patterns. It was also observed that small firms exhibit more momentum than the large firms (Jegadeesh & Titman, Citation1993; Lee & Swaminathan, Citation2000), De Bondt and Thaler (Citation1985) research showed that investors overreact, Poterba and Summers (Citation1988) concluded analogous reversals for national stock price indexes, Basu (Citation1997) challenged the myth of efficiency of security prices and many other studies as highlighted in Sewell (Citation2001, Citation2007).
This shows some irrational behaviors that are found in the stock markets around the globe. These irrational behaviors are out of the scope of Traditional Finance Theories and Traditional Finance alone is insufficient to explain the structure and dynamics of asset prices in the stock markets whereas Behavioral Finance offers a promising and plausible alternative way for these phenomena. The study of these anomalies comes under the scope of Behavioral Finance’s two prime subtopics i.e. Micro Behavioral Finance and Macro Behavioral Finance. Each of these two subtopics corresponds to a distinct set of issues within the traditional finance versus behavioral finance debate. Within the scope of Behavioral Finance Micro, it is examined whether individual investors are perfectly rational or cognitive and emotional errors can impact their financial decisions. While the scope of Behavioral Finance Macro, covers the market efficiency and examine whether they subject to behavioral effects or not (Pompain, Citation2006). As behavioral Finance Macro deals with the stock markets and their behaviors globally, therefore, it can also be termed as International Behavioral Finance and the study falls under the scope of International Behavioral Finance as it is going to examine the behaviors of emerging markets like Pakistan and Turkey, and to check the influence of international markets like Japan and Germany, on these emerging markets. With this background, this paper studies a behavioral finance theme known as sentiments, the concept and emergence is discussed in the following paragraphs.
1.2. Investor sentiments
The traditional finance’s definition of a rational investor is not only well defined but also helps in explaining the investor’s choices and develops accurate beliefs through the Bayesian logics. But it is not the case with behavioral finance’s bias named as Investors’ Sentiments. There has been no consensus made in the literature on single and widely accepted definition of investor sentiment. It can only be assumed that it means investors are inclined toward erroneous beliefs while making their choices.
In simple words, investors who are subjected to sentiments; might develop their beliefs, which might be statistically incorrect, through news or from inappropriate noisy signals (Zhang, Citation2008). In general, investor sentiments means the propensity of an individual, to trade on basis of emotions, moods, and beliefs instead of facts and technical analysis. The concept of Sentiments, in itself, is classified into a wide spectrum and can be used in a variety of ways by researchers and financial analysts (Baker & Wurgler, Citation2007; Barberis, Shleifer, & Vishny, Citation1998; Brown & Cliff, Citation2004; Qiu & Welch, Citation2004; Zhang, Citation2008). For example, many researchers accredit investor’s sentiment as tendency to trade on bases of noise instead of information, while some particularly employed it to make reference to investor optimism or pessimism. The term is also associated with the emotions and moods (Bormann, Citation2013), thus some of the researchers stated it as risk aversion or investor’s fear, while some regarded it in the terms of beliefs. The definitions exist in the literature; vary from statement to statement about this explicit psychological bias that is more model specific rather than theoretical (Shefrin, Citation2007). The measures developed in this regard are ranged from academic intentions to daily indices used by traders.
Since there are numerous measures like closed-end fund discount, investor intelligence surveys, ratio of odd-lot sales to purchases, implied volatility of index options, consumer confidence indices, market liquidity, net mutual fund redemptions, it may be concluded that consensus about the accuracy and efficiency of these measure is not found among the researchers and the investors. The cause of this disagreement is that the investors employ these sentiment measures as investment tools, while the researchers’ purpose is to form arguments for or against the market efficiency hypothesis. Sentiment is measured under two subdivisions in literature: (1) market-based proxies for sentiment and (2) direct surveys. In the first approach, the financial proxies such as closed-end fund discount or put–call ratio etc. are used as the proxy for extracting sentiment indirectly from market data. While in the second approach, sentiment is measured by using questionnaires and surveys directly from the investors. The Yale School of Management’s Stock Market Confidence Index and the University of Michigan’s Consumer Confidence Index are examples for this measure. This study is going to use the former on the data collected from the stock markets of different countries.
1.3. Research question
This study tries to find the answers regarding the measurement of Sentiments also it focuses on whether stock exchanges of developing countries are affected by the stock exchanges of developed countries. Is Sentiment Index created by using new proxies able to explain the behavior of the stock market?
1.4. Objectives of the study
The objectives this study will achieve are as follow:
• | Studying the significance of Sentiment Index with new proxies regarding stock returns in the stock market of developing countries. | ||||
• | Check whether stock exchanges of developing countries are influenced by stock exchanges of developed countries. |
1.5. Significance of the study
Sentiments have been checked with different approaches on different markets in different geographical locations. Still there exists a gap of significance of these approaches in the sphere of developing and developed countries. This study will try to fill this gap and open some new dimensions in understanding the sentiments of the investors and how they made their decisions while investing in a stock market. The markets of USA, Germany, Turkey, Japan, and Pakistan have been chosen for the study for two reasons, the first is that these markets can easily be divided into developed and developing stock markets and second reason is that a comparison of local and international markets will reveal where Pakistan is standing in international scenario.
2. Literature review
Investor sentiment has been regarded as a myth by traditional financial theories and it has also received little attention by researchers prior to 1990. It has been connected with different attributes and therefore, the literature offers no universal definition. Investor sentiment mostly refers to an overall attitude of investors toward the financial market. It represents feelings, moods, beliefs, or expectations of investors which influence their decision-making. In early literature of sentiments, Smidt (Citation1968) linked it to speculative bubbles, Zweig (Citation1973) related it to cognitive biases of the investors, while Black (Citation1986) called investors’ expectations about the returns of assets as noise. De Long et al (Citation1990a) consider it as investors’ belief about future cash flows and investment risks which are unjustified by existing fundamentals. For Shleifer (Citation2000), it is the common judgment errors made by a substantial number of investors. Shleifer and Summers (Citation1990) define the sentiment as part of investors’ expectations about the returns of assets. In view of Brown and Cliff (Citation2004), it is the expectations of market participants in relation to a trend meaning that a bullish (bearish) investor expects returns to be above (below) average which is corresponding with the view of Smith, Shoben, and Rips (Citation1974). Baker and Wurgler (Citation2006) define sentiment as the investor propensity to speculation; that is, sentiment drives the demand for speculative investments. It actually stimulates the investors to trade illogically and make them either overestimate or underestimate the stock performance. According to Zhang (Citation2008), sentiment can be defined as any erroneous belief that individuals have about an economic variable, e.g. Asset prices. The term “noise traders” is used for irrational traders who trade on the bases of beliefs or some pseudo-signals (Shleifer & Summers, Citation1990). These investors cannot properly judge the quality of information and therefore trade more on emotions in contrast with rational investors. Most of the models that investigate its effect on stock market pricing, adopt the assumption that noise traders play an important role in stock markets. In general, a consensus among economist is that irrational traders can induce large price movements and create excess volatility and over the last decades, a large body of research shows that investor sentiment influences stock prices (Shleifer & Summers, Citation1990). Verma and Verma (Citation2006) made a separation between rational and irrational investor sentiment on the bases of noise components of investor sentiment and their relative effects on volatility. Hence Sentiment may be categorized as irrational evaluation of asset characteristics (Baker & Stein, Citation2004; Shleifer, Citation2000). According to Baker and Wurgler (Citation2007), the question is no longer whether investor sentiment affects stock prices but rather how to measure and quantify its effects. In the literature of behavioral finance, there are two main measures of investor sentiment. A direct measure, which is based on surveys and questionnaires, and an indirect measure, modeled with the market data as proxies. Both measures have their advantages and disadvantages.
The empirical results found in the study of De Long et al (Citation1990b) predicted that stocks disproportionally held by sentiment (noise) traders are disproportionally subject to investor sentiment. Lee, Shleifer, and Thaler (Citation1991) by adopting the assumption of Zweig (Citation1973) examined close-end mutual fund discounts as proxy for individual sentiments and indicated a negative correlation with fund’s returns. They however only look at individual investor sentiment and not at sentiment of institutional investors. The study of Neal and Wheatley (Citation1998) showed that the small firms’ expected returns and the fund discounts are positively related, while there is no correlation between the large firms’ expected returns and the fund discounts. Fisher and Statman (Citation2000) compared sentiment of individual investors with sentiment of institutional investors; they used data from the American Association of Individual Investors (AAII), an agency which conducts a monthly survey among members. They compare sentiments by direct measure and examine if it predicts stock returns. They indicated high consumer confidence as an indicator for low subsequent returns. Wang (Citation2001) has developed an investor sentiment index for the each type of trader which is based on their current positions and historical values. Lee, Jiang, and Indro (Citation2002) utilized Investors’ Intelligence of New Rochelle as a reliable forecaster of market movements. Another measure of sentiment proposed by Brown, Goetzmann, Hiraki, Shirishi, and Watanabe (Citation2003) was based on daily flow of mutual funds in Japanese and US stock market. They concluded that the sentiment factor is constant and should be priced and also found negative correlation in Japanese but positive correlation in the US market, indicating a foreign vs. domestic sentiment factor. It has also been argued that overconfidence might give rise to difference of opinions among investors (Scheinkman & Xiong, Citation2003). Brown and Cliff (Citation2004, Citation2005) proposed a sentiment index, using principal component analysis (PCA) and combined several sentiment proxies. In addition, they examine causal relation between their sentiment index and expected returns using vector auto regression (VAR). Chan and Fong (Citation2004) employed a parsimonious method for testing the forecasting capability of announcements on the individual investors’ sentiment for future returns. Qiu and Welch (Citation2004) stated their hypothesis that sentiment changes disproportionally influence small stocks using Michigan Consumer Confidence Index, they have given a detailed description of sentiment variables that are used in behavioral finance. Baker and Wurgler (Citation2006) developed a composite sentiment index in which six commonly used proxies for investor sentiment, i.e. trading volume based on turnover, the dividend premium, the closed-end fund discount, the number and first-day returns on IPOs, and the equity share in new issues, were used. They separated the sampling period into two regimes i.e. high sentiment regime and low sentiment regime. The methodology of Baker and Wurgler (Citation2006) has been widely accepted and used to check the sentiments in many stock exchanges. But recently, a new market sentiment index has been made by Chen, Chong, and Duan (Citation2010). They use the principal-component method to form a linear index with factors such as the market turnover, short selling volume, relative strength index, money flow index, market Hong Kong Interbank Offered Rate (HIBOR), and the indices of foreign equity markets as Sun and Zhang (Citation2009) find interdependence of the financial markets of China and Hong Kong and also, the conditional correlation between China and Hong Kong has overshadowed their conditional correlations with the United States. Shu (Citation2010) studied the influence of mood on financial market behavior. This study showed that both stocks and bills’ price positively correlate with investors’ mood and also that higher asset prices are associated with better mood. This paper is going to follow the footsteps of Chen et al. (Citation2010) and Chen, Chong, and She (Citation2014) to examine the influence of foreign developed countries on the local developing country with some modification in the index created by them. Also the study of Rehman (Citation2013) is followed to check the relationship of sentiment index and returns of developing stock markets.
3. Research methodology and models
The goal of this research is to develop a sentiment index using a PCA for the regional developing country and check the influence of the foreign developed stock markets on the regional developing market and the relationship between this index and stock market returns. The index has been created by following the methodology of Chen et al. (Citation2010) and Chen et al. (Citation2014) for the development of the modified index while Rehman (Citation2013) is followed to examine the relationship between stock market returns and sentiment index. The methodology has been used for Turkey and Pakistan separately.
3.1. Investor sentiment measure
3.1.1. Market data as proxies approach
Many researchers support in favor of using market data as proxy for sentiment, although it is a step for quantifying the actual beliefs of investor. Zweig (Citation1973), Malkiel (Citation1977), Chen, Kan, and Miller (Citation1993), Berk and Stanton (Citation2004) and Ross (Citation2005) have provided rational explanations for using proxy for investor sentiment in many studies. Kumar and Lee (Citation2006) have constructed a sentiment index for retail investors. Baker & Wurgler, Citation2007 developed a sentiment index in which six commonly used proxies for investor sentiment i.e. trading volume based on turnover, the dividend premium, the closed-end fund discount, the number and first-day returns on IPOs, and the equity share in new issues are used and they stated that when the sentiment is low (high), speculative stocks have greater (lower) future returns on average which is inconsistent with classical asset pricing theories. Even though various indices are used as proxy for investor sentiment, they all reflect and capture different information. The selection of these proxies is sometimes arbitrary, depending upon the purpose of the study and the availability of data. This study has adopted the methodology of Chen et al. (Citation2010) and Chen et al. (Citation2014) with a little modification in the variable of short selling because the data on short selling are not available in Pakistan as well as in Turkey, rest of all other proxies are same.
3.1.2. Model for investor sentiment index
The model used in this research for the sentiment index goes like;(1)
(1)
where SMIm,t is the first principal component estimated by the above mentioned linear combination of the standardized variables, STURN is respective stock exchange turnover, MFI is money flow index, IBOR is the interbank offer rate, RSI means relative strength index, is the Change in local market Index, RWBMK,t−1 is the return of the world benchmark stock exchange at t − 1 time period and RRBMK,t−1 is the return of the regional benchmark stock exchange at t − 1 period. The description of the variables is as follows:
3.1.2.1. Respective stock exchange turnover ratio
The turnover ratio is included in the measurement model because it is measures the trading activity of the stock market. In a previous study, it has been suggested that larger turnover is associated with a price rise where small turnover is associated with price fall (Ying, Citation1966). Simply saying the turnover in bullish market period is high while in bearish market period is low.
where VMweek is the average volume for a week and VMyear is average volume of year. STURN has been calculated on running/ moving basis i.e. by dropping the one previous value and adding one next value.
3.1.2.2. Money flow index
The money flow index is associated with the information about daily stock prices and turnover. Joubert and Mason (Citation1992) have suggested that an increase in the money flow indicate the end of a market trend. To calculate the Money flow index, the following calculations have been done:
When the daily price is lower in the previous day, the money flow is defined as positive money flow and when the daily price is higher in the previous day, the money flow is defined as negative money flow. The money flow index for week period has been calculated as follows:
3.1.2.3. Relative strength index
The Relative Strength Index has been included in the model as it indicates the buying and selling activities in the market. It is considered that the RSI above 80 shows an overbought market whereas RSI below 20 shows oversold market. The RSI for the period of week has been calculated as follows:
where (Pt,i − Pt−1,i)+ = |Pt,i − Pt−1,i| if Pt,i − Pt−1,i > 0, otherwise = 0.
3.1.2.4. Interbank offer rate
It is the rate at which banks borrow money from each other. In Pakistan, KIBOR is used and in Turkey TLIBOR is used. The Interbank Offer rate is used as a proxy for investment cost in the model.
3.1.2.5. Change in the local market index
It is the change in the local market index to capture the mood swings of the investors as in the methodology of Chen et al. (Citation2010) and Chen et al. (Citation2014) used short-selling volume for this but we have no availability of short selling data in Pakistan and Turkey. This proxy has been adopted from the study of Daszynska-Zygadlo, Szpulak, and Szyszka (Citation2014) as they have also used it in their sentiment index. As per their study, the change of the investors’ mood is depicted by the change of the market index and calculated as follows:
3.1.2.6. Performance of other markets
According to the study of Lee (Citation2006), the stock exchanges of USA and Japan significantly affect the Hong Kong market. Similarly Keong (Citation2010) has found a minor influence of Chinese market on Hong Kong stock market. The same idea has been adopted for this study to check the influence of the returns of the regional benchmark stock exchange and world benchmark stock exchange on local market. Therefore, the returns of the world benchmark and regional benchmarks have been added to see the influence of other markets on the local developing market.
3.1.2.6.1. Return of the world benchmark stock exchange
USA has been taken as the world benchmark market in this study. One period lagged value of the USA market has been taken to see the impact of this news on the sentiments of a developing country.
3.1.2.6.2. Return of the regional benchmark stock exchange
Germany and Japan have been the regional benchmark for Europe and Asia, respectively. One period lagged value of the market returns has taken so to capture its impact on the regional developing market.
3.1.3. Relationship of sentiment index and stock market returns
This index is regressed on the volatility series of the stock exchanges of Turkey and Pakistan by following the methodology of Rehman (Citation2013). The following equation is used for Pakistan and Turkey separately to see the relationship between respective sentiment indices and returns of the market;(II)
(II)
where Ym,t is the market returns of stock market and SMIm,t is the sentiment index. Ym,t is calculated as:
where Pt is the closing price of the index at time t and Pt−1 is the closing price one period before.
3.2. Data and data sources
First of all, the countries have been selected from Asia and Europe. They have been divided into developing and developed countries as per the classification made by the United Nations Organization.Footnote1 Pakistan and Japan are selected from Asia and Turkey, and Germany from Europe. Then the developed country has been taken as the regional benchmark for the developing country. USA has been taken as the world benchmark. All the data in this study has been collected from Quandl, Yahoo Finance, Google Finance, Wall Street Journal, Federal Reserve Bank’s Economic Data, and the respective stock Markets of USA (S&P-500 Index), GERMANY (DAX-30 Index), TURKEY (Borsa Istanbul-100 index), JAPAN (Nikkei-225 Index), and PAKISTAN (KSE-100 Index). Ten years data from 12-29-2004 to 12-31-2014 has been analyzed to achieve the results for the behavior in developing and developed stock markets. The cycle of weekly data has been adopted to avoid white noise effect in the results (James, Citation2013). The reasons for using weekly (Wednesday) price data are to avoid first or last day-of-the week and non-synchronous trading effects (Bhar & Nikolova, Citation2009; Chiang & Doong, Citation2001; Ng, Citation2000).
4. Empirical analysis of data and results
4.1. Descriptive statistics for sentiment index
Table shows the descriptive statistics for the variables chosen as proxy for the making the sentiment index for Pakistani (PK) and Turkish (TR) stock market. The mean value for STURN in Pakistani market is 97.556 with the median of 90.839 and the highest value is 314.701 making this market highly volatile regarding the returns of the market, while on the other hand, Turkish market is a stable market regarding the returns of the market, with the mean of STURN to be 104.192 with median of 102.508 and the highest value 212.826, whereas the oscillating momentum and market strength index indicators i.e. the MFI and RSI are indicating that Pakistani stock market is bit stable in terms of price fluctuations as means of these values fall in the region of 10 to 90. Still the market is un-predictive in terms of price fluctuations as the maximum and minimum values of RSI are above 70 and below 30 showing the trend of overbought and oversold securities, respectively, whereas the MFI and RSI of the Turkish market indicate that this market somewhat behaves like the Pakistani Stock exchange in terms of price fluctuations. The kurtosis of all the variables shows these variables are somewhat distributed normally during the course of time period of the study with a little positive and negative skewness, whereas, the kurtosis of the Index Change of Local Market and the Foreign Market Returns are way above the threshold (3.00) indicating that the returns are not distributed normally during the time frame. Total number of observations is 522 as weekly observations have been collected for a time period of 10 years. The graphical representation of the above said variables can be seen in Figures –.
Table 1. Descriptive statistics of the chosen variables
4.2. Principle component analysis of the variables chosen for sentiment index
Table (a) and (b) shows the principle component analysis of the chosen variables and it explains that the and the first principal component is explaining 33.80% of the relationship in the case of Pakistani market while it is explaining 31.50% of the relationship in case of Turkish market. The index created by using first principal component makes the index for Pakistan and Turkey is as follows:
Table 2. PCA of the six variables
The graphical representation of the index can be seen in Figures –.
4.3. The relationship between sentiment index created and the chosen variables
The variable proxies chosen for the study are regressed with the sentiment index for examination of the relationship between the index and the proxies. The following Table shows that the stock market turnover, money flow index, relative strength index, change in the local index, and lagged return of the foreign markets show a positive impact or they are directly related with the sentiment index in both countries where as the interbank offer rates of both the countries shows the negative impact or inverse relationship. It indicates that whenever the interest rate increases the investors invest their funds in other financial investments rather to risk them in stock markets. The higher values of foreign markets indicate their strong impact on the investors’ behavior. The values in the bracket are t-statistics significant at 95 level of confidence interval.
Table 3. The relationship among variables and the sentiment index
4.4. The relationship between sentiment index created and the market returns
The indices created for each country have been regressed with the respective returns of the country to see whether or not these can explain the return pattern of a market. The results are as follows:
Table shows the regression results for the equation . The time period taken is from 5 January 2005 to 31 December 2014. The values in the bracket are t-statistics significant at the 95 level of confidence interval. It also indicates that the returns and the sentiment index are positively related and this also shows that the index created in this study is a good predictor for return behavior of a developing country. The graphical representation is attached in Figures –.
Table 4. The relationship between sentiment index and returns of respective markets
5. Conclusion
In this paper, the investment behavior among developing markets has been checked by following the footsteps of a working paper by Chen et al. (Citation2010) where they have created a sentiment index using a new PCA approach for investors’ sentiments. The market proxies such as stock market turnover, money flow index, relative strength index, interbank offer rates, change in the regional market stock index, and performance of the foreign markets have been selected in this study as variables to build an index for sentiments. The research elaborates a significant strength of these variables to be taken as proxies of investors sentiments. The index significantly shows influence of the foreign stock exchange on the local stock exchange, furthermore, the return patterns of the developing country has been regressed upon this index to check whether any relationship exist between the developed index and the stock market returns, the results clearly explain that there is a strong relationship between the index and return pattern of the developing country’s stock market. Hence it is concluded that the index can be used as a reference for investor sentiments.
5.1. Suggestions and limitations
Suggestions for further research include study of the financial markets of the emerging countries to examine neighborhood market’s influence and to check if geographical distance can be a factor to influence investor sentiments. Furthermore, time period and data-set would be suitable to conduct additional test of herding in the sphere of developing and developed countries to investigate whether this produces different results. The study is limited in a sense that only few countries have been chosen for the analysis due to limited resources. The scope of the subject is very vast and there is a further space for more research.
Additional information
Funding
Notes on contributors
Muhammad Zia Ur Rehman
Muhammad Zia Ur Rehman has held positions of Lecturer of Finance at National Textile University Faisalabad, PhD Scholar at International Islamic University Islamabad and Co-PI at the HEC (Higher Education Commission) Research Project.
He specializes in corporate behavioral finance, global financial markets, micro structure of financial markets, innovative research epistemology and international Finance. He is a prolific research scholar. He has several international and national publications having focus of finance and business research.
Notes
1. Classification of countries of the world on the basis of development by United Nations can be checked here www.un.org/en/development/desa/policy/wesp/wesp.../2012country_class.pdf.
References
- Baker, M., & Stein, J. (2004). Market liquidity as a sentiment indicator. Journal of Financial Markets, 7, 271–299.10.1016/j.finmar.2003.11.005
- Baker, M., & Wurgler, J. (2006). Investor sentiment & the cross-section of stock returns. The Journal of Finance, 61, 1645–1680.10.1111/j.1540-6261.2006.00885.x
- Baker, M., & Wurgler, J. (2007). Investor sentiment in the stock market. Journal of Economic Perspectives, 21, 129–151.10.1257/jep.21.2.129
- Barberis, N., & Thaler, R. (2003). A survey of behavioral finance. A Handbook of the Economics of Finance, 1, 1053–1128.
- Barberis, N., Shleifer, A., & Vishny, R. (1998). A model of investor sentiments. Journal of Financial Economics, 49, 307–343.10.1016/S0304-405X(98)00027-0
- Bartov, E., Radhakrishnan, S., & Krinsky, I. (2000). Investor sophistication and patterns in stock returns after earnings announcements. The Accounting Review, 75, 43–63.10.2308/accr.2000.75.1.43
- Basu, S. (1997). The conservatism principle and the asymmetric timeliness of earnings. Journal of Accounting & Economics, 24, 3–37.10.1016/S0165-4101(97)00014-1
- Berk, J., & Stanton, R. (2004). A rational model of the closed-end fund discount (Working Paper). Cambridge, MA: NBER.10.3386/w10412
- Bhar, R., & Nikolova, B. (2009). Return, volatility spillovers and dynamic correlation in the BRIC equity markets: An analysis using a bivariate EGARCH framework. Global Finance Journal, 19, 203–218.10.1016/j.gfj.2008.09.005
- Black, F. (1986). Noise. The Journal of Finance, 41, 528–543.10.1111/j.1540-6261.1986.tb04513.x
- Bormann, S. K. (2013). Sentiment indices on financial markets: What do they measure? (Economics Discussion Papers, 58). Kiel Institute for the World Economy. Retrieved from http://www.economics-ejournal.org/economics/discussionpapers/2013-58
- Brown, G. W., & Cliff, M. T. (2004). Investor sentiment and the near-term stock market. Journal of Empirical Finance, 11(1), 1–27.10.1016/j.jempfin.2002.12.001
- Brown, G. W., & Cliff, M. T. (2005). Investor sentiment and asset valuation. The Journal of Business, 78, 405–440.10.1086/jb.2005.78.issue-2
- Brown, S. J., Goetzmann, W. N., Hiraki, T., Shirishi, N., & Watanabe, M. (2003). Investor sentiment in Japanese and U.S. daily mutual fund flows (Working Paper). New Haven, CT: Yale University.10.3386/w9470
- Chan, S. Y., & Fong, W. (2004). Individual investors’ sentiment & temporary stock price pressure. Journal of Business Finance & Accounting, 31, 823–836.10.1111/jbfa.2004.31.issue-5-6
- Chen, H., Chong, T. T. L., & Duan, X. (2010). A principal-component approach to measuring investor sentiment. Quantitative Finance, 10, 339–347.10.1080/14697680903193389
- Chen, H., Chong, T. T. L., & She, Y. (2014). A principal component approach to measuring investor sentiment in China. Quantitative Finance, 14, 573–579. doi:10.1080/14697688.2013.869698
- Chen, N., Kan, R., & Miller, M. H. (1993). Are the discounts on closed-end funds a sentiment index? The Journal of Finance, 48, 795–800.10.1111/j.1540-6261.1993.tb04741.x
- Chiang, T. C, & Doong, S. C. (2001). Empirical analysis of stock returns and volatility: Evidence from seven Asian stock markets based on TAR-GARCH model. Review of Quantitative Finance and Accounting, 17, 301–318.10.1023/A:1012296727217
- Cutler, D., Poterba, J. M., & Summers, L. H. (1991). Speculative dynamics. The Review of Economic Studies, 58, 529–547.10.2307/2298010
- Daszynska-Zygadlo, K., Szpulak, A.. & Szyszka, A. (2014). Investor sentiment, optimism and excess stock market returns. Evidence from emerging markets, Business and Economic Horizons (BEH), 10, 362–373.
- De Bondt, W. F. M., & Thaler, R. H. (1985). Does the stock market overreact? The Journal of Finance, 40, 793–805.10.1111/j.1540-6261.1985.tb05004.x
- De Long, J. B., Shleifer, A., Summers, L., & Waldmann, R. J. (1990a). Noise trader risk in financial markets. Journal of Political Economy, 98, 703–738.10.1086/261703
- De Long, J. B., Shleifer, A., Summers, L., & Waldmann, R. J. (1990b). Positive feedback investment strategies and destabilizing rational speculation. Journal of Finance, 45, 374–397.
- Fama, E. F. (1970). Efficient capital markets: A review of theory & empirical work. The Journal of Finance, 25, 383–417.10.2307/2325486
- Fisher, K. L., & Statman, M. (2000). Investor sentiment and stock returns. Financial Analysts Journal, 56, 16–23.10.2469/faj.v56.n2.2340
- James, F. (2013). Characterization and impact of low frequency wind turbine noise emissions. Electronic Theses and Dissertations. Paper 4722.
- Jegadeesh, N., & Titman, S. (1993). Returns to buying winners and selling losers: Implications for stock market efficiency. The Journal of Finance, 48, 65–91.10.1111/j.1540-6261.1993.tb04702.x
- Joubert, D. J., & Mason, A. F. (1992). Investment basics-XXV volume and the bull-bear cycle. Investment Analysts Journal, 35, 49–50.
- Keong, T. W. (2010). The time-series analysis for interactions among returns on S&P500, CSI300 and HSI before and after bankruptcy of Lehman Brothers (UM E-theses collection).
- Kumar, A., & Lee, C. (2006). Retail investor sentiment and return co-movements. The Journal of Finance, 61, 2451–2486.10.1111/j.1540-6261.2006.01063.x
- Lee, C., Shleifer, A., & Thaler, R. H. (1991). Investor sentiment and the closed-end fund puzzle. The Journal of Finance, 46, 75–109.10.1111/j.1540-6261.1991.tb03746.x
- Lee, C. M. C., & Swaminathan, B. (2000). Price momentum and trading volume. The Journal of Finance, 55, 2017–2069.10.1111/0022-1082.00280
- Lee, K. Y. (2006). The contemporaneous interactions between the U.S., Japan, & Hong Kong stock markets. Economics Letters, 90, 21–27.10.1016/j.econlet.2005.04.004
- Lee, W. Y., Jiang, C. X., & Indro, D. C. (2002). Stock market volatility, excess returns, and the role of investor sentiment. Journal of Banking and Finance, 26, 2277–2299.10.1016/S0378-4266(01)00202-3
- Malkiel, B. G. (1977). The valuation of closed-end investment-company shares. The Journal of Finance, 32, 847–859.10.1111/j.1540-6261.1977.tb01993.x
- Neal, R., & Wheatley, S. M. (1998). Do measures of investor sentiment predict returns? The Journal of Financial and Quantitative Analysis, 33, 523–547.10.2307/2331130
- Ng, A. (2000). Volatility spillover effects from Japan and the US to the Pacific-Basin. Journal of International Money and Finance, 19, 207–233.10.1016/S0261-5606(00)00006-1
- Pompain, M. M. (2006). Behavioral finance & wealth management: How to build optimal portfolios that account for investor biases. Hoboken, NJ: Wiley.
- Poterba, J. M., & Summers, L. H. (1988). Mean reversion in stock prices. Journal of Financial Economics, 22, 27–59.10.1016/0304-405X(88)90021-9
- Qiu, L., & Welch, I. (2004). Investor sentiment measures (NBER Working Paper Series). Cambridge, MA: NBER.10.3386/w10794
- Rehman, M. U. (2013). Investor’s sentiments and stock market volatility: An empirical evidence from emerging stock market. Pakistan Journal of Commerce and Social Sciences, 7, 80–90.
- Ross, S. A. (2005). Neoclassical finance. Princeton, NJ: Princeton University Press Princeton.
- Rouwenhorst, K. G. (1998). International momentum strategies. Journal of finance, 53, 267–284.
- Scheinkman, J., & Xiong, W. (2003). Overconfidence and speculative bubbles. Journal of Political Economy, 111, 1183–1220.10.1086/378531
- Sewell, M. (2001). Behavioural finance. Retrieved from http://www.behaviouralfinance.net/behavioural-finance.pdf
- Sewell, M. (2007). Behavioural finance. Retrieved from www.behaviouralfinance.net/behavioural-finance.pdf
- Shefrin, H. (2007). Risk & return in behavioral SDF-based asset pricing models. Journal of Investment Management, 6(3), 1–18.
- Shiller, R. J. (1989). Market volatility. Cambridge, MA: MIT Press.
- Shleifer, A. (2000). Inefficient markets: An introduction to behavioral finance. Oxford: Oxford University Press.10.1093/0198292279.001.0001
- Shleifer, A., & Summers, L. H. (1990). The noise trader approach to finance. Journal of Economic Perspectives, 4, 19–33.10.1257/jep.4.2.19
- Shu, H. (2010). Investor mood and financial markets. Journal of Economic Behavior & Organization, 76, 267–282.10.1016/j.jebo.2010.06.004
- Smidt, S. (1968). A new look at the random walk hypothesis. The Journal of Financial and Quantitative Analysis, 3, 235–261.10.2307/2329812
- Smith, E. E., Shoben, E. J., & Rips, L. J. (1974). Structure and process in semantic memory: A featural model for semantic decisions. Psychological Review, 81, 214–241.10.1037/h0036351
- Sun, T., & Zhang, X. (2009). Spillovers of the U.S. subprime financial turmoil to mainland China and Hong Kong SAR: Evidence from stock markets (Working Paper No. 09/166). Washington, DC: IMF.
- Verma, R., & Verma, P. (2006). Noise trading and stock market volatility. Journal of Multinational Financial Management, 17, 231–243.
- Wang, C. (2001). investor sentiment and return predictability in agricultural futures markets. Journal of Futures Markets, 21, 929–952.10.1002/(ISSN)1096-9934
- Ying, C. C. (1966). Stock market prices and volumes of sales. Ecommetrica, 34, 676–686.
- Zhang, C. (2008). Defining, modeling, and measuring investor sentiment (Doctoral thesis). University of California, Berkeley.
- Zweig, M. E. (1973). An investor expectations stock price predictive model using close-end fund premiums. The Journal of Finance, 28, 67–78.10.1111/j.1540-6261.1973.tb01346.x