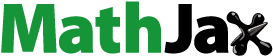
Abstract
The present paper investigates the adverse selection problem by examining the relationship between accident occurrences and deductible choice utilizing a seemingly unrelated probit model that allows for best controls for unobserved heterogeneity and endogeneity. While this microeconometric analysis does not consider a multivariate model and considers only two types of contracts, namely, those with high and low deductibles, it does suggest important implications from applying a recursive bivariate probit. We employ new cross-sectional data on a Tunisian insurance portfolio containing 31,125 policyholders. The results support some evidence for residual adverse selection in the studied insurance portfolio. Moreover, the results suggest the presence of a wealth effect in the decision of the contract choice.
Public Interest Statement
Adverse selection is a phenomenon that is endemic to various insurance line of business, including car insurance. It occurs whenever people make insurance purchasing decisions based on their own knowledge of their degree of risk or likelihood of making a claim on the insurance coverage. This empirical study provide a useful application allowing a better understand of this phenomena. Using statistical and econometric approaches we examine the relationship between accident occurrences and deductible choice. These later, allow a test for the presence of adverse selection in the studied market and provide information about the factors influencing the probability of being involved in car accidents.
1. Introduction
Theoretical studies of insurance markets have extensively underlined the potential importance of asymmetric information and documented its undesirable implications on the development and sustainability of the insurance industry. Two major asymmetric information problems are discussed: moral hazard and adverse selection. In particular, adverse selection has attracted special importance in the theory since the initial paper of Stiglitz (Citation1977). In that paper, the author discusses the presence of adverse selection in a monopoly insurance market in the case of single-period contracts. The impact of adverse selection on welfare has been discussed sufficiently by Rothschild and Stiglitz (Citation1976). These two original studies have been extensively discussed and extended, See, for example, Wilson (Citation1977), Salanié (Citation1997), Spence (Citation1978), Dionne, Doherty, and Fombaron (Citation2001), and more recently, Einav, Finkelstein, and Levin (Citation2010), Einav, Finkelstein, and Schrimpf (Citation2010), and Handel (Citation2013).
Despite the extensive theoretical research on the adverse selection problem in insurance markets, its empirical relevance, however, remains an issue of considerable debate. In this perspective, the main empirical subject is to discuss adverse selection as a considerable resource allocation problem in many markets. In particular, in car insurance markets, risk classification is mostly related to adverse selection; however, the presence of different deductibles can also be explained by proportional transaction costs with different observable risks.
The deductible is chiefly defined as the amount “deducted” from an insured loss. It is an essential part of the insurance contract and represents a sharing of the risk between the insurance company and the policyholder. In the existing literature about insurance, it is usually assumed that a policyholder who chooses a low deductible is exposed to less risk, but is faced with a higher level of expected expenditure. Thus, an individual’s decision to choose a low (high) deductible provides a lower (upper) bound for his coefficient of absolute risk aversion. Related to this topic about the deductible choice, a tricky empirical investigation is to verify whether the existence of various deductibles in an insurance portfolio is explained by residual adverse selection. Otherwise, does the choice of a deductible by a policyholder reveal information about its risk level?
Several current empirical studies have rejected the hypothesis of adverse selection in automobile insurance markets. These studies include that of Chiappori and Salanie (Citation2000), who apply various parametric and nonparametric methods on French automobile insurance data on contracts and accidents and find no evidence for the presence of asymmetric information in that market. Dionne, Gouriéroux, and Vanasse (Citation2001) demonstrate that risk classification is sufficient, in the sense that there is no residual adverse selection on risk types in the automobile insurance portfolio studied. Cohen (Citation2005) employs a non-linear model to test the relationship between the risk of policyholders and deductibles, but she did not find any correlation for beginning drivers.
Conversely, some empirical studies suggest the presence of the adverse selection phenomenon in insurance markets; Puelz and Snow (Citation1994) and Cohen (Citation2001) offer some evidence for adverse selection in US automobile insurance markets, but only for experienced drivers. Grun-Réhomme and Benlagha (Citation2007) employ a bivariate model on French data and demonstrate that the adverse selection problem exists for experienced drivers but not for new policyholders. Shi and Valdez (Citation2011) utilize a portfolio of contracts of an insurer in Singapore and apply a copula approach to test the dependence between the risk level of policyholders and deductibles; he found evidence of a significant positive risk-deductible correlation. Li, Liu, and Peng (Citation2013) employ a data-set for the Taiwanese automobile insurance market to investigate bundled automobile insurance coverage and the occurrence of claims. They found that vehicle physical damage insurance is the major automobile coverage and that this type of coverage affects the decision to purchase voluntary liability insurance coverage as a complement.
It is also interesting to note that the existing literature about adverse selection usually raises the problem of the coexistence of the moral hazard problem in different markets with the adverse selection (Benlagha, Charfeddine, & Karaa, Citation2012; Chassagnon & Chiappori, Citation1997; Fuller, Citation2014; Keane & Stavrunova, Citation2016; Koufopoulos, Citation2009). These theoretical studies stress on the importance of studying the adverse selection and the moral hazard jointly. However, the dissociation between the two asymmetric information forms seems to be technically challenging and problematic.
In this paper, with respect to the nature of exploited data, we will only focus on the adverse selection phenomenon.
Two major problems are identified in these empirical studies. First, the problem of heterogeneity of the insurance portfolio is neglected in most of the empirical studies. Indeed, the presence of a large number of policyholders with no accidents could affect the hypothesis of homogeneity assumed when investigating the relationship between risk and deductible choices. Second, the test of the adverse selection hypothesis is usually conducted by utilizing bivariate or multivariate models, which could generate an endogeneity bias in the estimation results.
The current article contributes to the literature by examining the adverse selection problem by investigating the relationship between accident occurrences and deductible choices utilizing a seemingly unrelated probit model that allows for best controls for unobserved heterogeneity and endogeneity.
While this microeconometric analysis does not consider a multivariate model and considers only two types of contracts (high and low deductibles), it does suggest important implications from applying a recursive bivariate probit.
First, employing this modelling approach allows a better appreciation for the heterogeneity in different insurance risk classes. In fact, the heterogeneity in a class of risk units can be considered a main source of underestimation of the pure premiums paid by policyholders in a class.
This potential underestimation causes a large gap between expected losses and real amounts of recoveries paid by the insurer. Consequently, the benefits of insurers may decrease.
Second, the recursive bivariate probit model helps us to correct for unobserved heterogeneity, which has an immense implication for the insurer because as mentioned by Chiappori and Salanie (Citation2000), the elimination of heterogeneity reduces the problem of residual adverse selection in a class composed by a large number of unit risks.
Therefore, our empirical approach relies on first estimating a seemingly unrelated probit model that does not include the deductible choice as an endogenous variable. This allows us to determine whether a joint estimation is suitable but does not evaluate the impact of the deductible choice on accident occurrences. Then, we test for exogeneity using a maximum-likelihood simultaneous estimation of the two probit equations, a method also known as recursive bivariate probit, initiated by Maddala (Citation1983).
The rest of the paper is organized as follows. The Section 2 presents the seemingly unrelated probit and the recursive models to be estimated. Then, in Section 3, we present the data. The estimation results are discussed in Section 4. Finally, concluding remarks and directions for future research are suggested in Section 5.
2. Methodology
The key variables under investigation are dichotomous and correspond to accident occurrences and deductible choices. Thus, a latent variable would be appropriate to empirically test their relationship. Because decision variables are likely to be related over time, unobservable variables may affect both accident occurrences and deductible choices. Therefore, our empirical strategy relies on first estimating a seemingly unrelated probit model which does not contain the deductible choice as an endogenous dummy. This allows us to determine whether a joint estimation is appropriate but does not evaluate the impact of the deductible choice on accident occurrences. Then, we test the presence of exogeneity by applying maximum-likelihood simultaneous estimations of the two probit equations, a method also identified as recursive bivariate probit, proposed by Maddala and Lee (Citation1976) and Maddala (Citation1983) and discussed and applied by Greene (Citation1998, Citation2003) or as a seemingly unrelated probit model with endogenous dummy variables by Fabbri, Monfardini, and Radice (Citation2004).
The latent model generally supposes normality of responses within latent classes but the mixed distribution may contain non-normal marginal and joint distributions of response probabilities. The model helps us to correct for some unobserved heterogeneity that may otherwise give rise to “omitted variable bias” and is expected to raise the efficiency of the estimation. Finally, to provide additional insight into the nature of the joint choices made by individuals, we calculate the marginal effects of covariates on the probabilities of choosing each type of outcome and on the joint probabilities of each combination of alternatives. The marginal effect allows us to simulate changes in policyholders’ characteristics, which may link deductible choices to individual risk, measured by the accident occurrences.
2.1. The seemingly unrelated probit
We assume that accident occurrence is a latent variable presented by and that
is the latent variable measuring the deductible choices. Because these two latent variables are not directly observable, we specify the two-equation model written as
(1)
(1)
where X1i denotes the observed independent variables explaining the accident occurrence, α1represents parameter associated to each independent variable, and ɛ1i corresponds to a random error term.
We also assume that the deductible choice can be modelled as:(2)
(2)
where X2i denotes the observed independent variables explaining the deductible choice, α2 represents parameter associated to each independent variable, and ɛ2i corresponds to a random error term.
It is noted that the two variables are potentially explained by the same exogenous variables; thus, the error terms of the two models are dependent and distributed as a bivariate standard normal. In the context of dependency and bivariate normal distribution, we obtain:
If the error terms of both equations are affected by similar components, ɛji = μi + ηji, then, although they are likely to be normally distributed, they will not be independent, but will depend on the value of μi.
To test whether the two models have to be jointly estimated, we propose to apply a Wald test for the null hypothesis ρ = 0.
2.2. Modelling adverse selection with presence of endogeneity
To model the problem of adverse selection with the presence of endogeneity, we pursue the tradition of the simultaneous equation models proposed by Maddala (Citation1983). It draws upon a reduced form equation for the potentially endogenous variable (accident occurrence) and a structural form equation for the dichotomous deductible choice variable written as follows:(3)
(3)
In this model, and
correspond to the latent variables measuring, respectively, accident occurrences and deductible choices. X1i and Z2i are exogenous variables, and α1, β1 and β2 are parameters of the behavioural function.
In this specification, the error terms are assumed to be dependent and distributed as a bivariate normal so that ,
, and
.
To test the evidence on the correlation between the unobserved explanatory variables of both equations, we must use the Wald test. This statistic test suggests that if ρ = 0, then is exogenous for the second equation.
3. Data
To empirically investigate the adverse selection with the presence of the heterogeneity problem, we employ new cross-sectional data of a Tunisian insurance portfolio. The used data are very informative for two main reasons. First, the studied company is among the largest companies operating in car insurance branch in Tunisia. The used portfolio concerns 54,040 policyholders. After preliminary analysis, we performed our empirical investigation on 31,125 policyholders. Secondly, our data cover the year 2009 after the implementation of the new no-claims bonus class. This may reflect the behaviour of the policyholders after the implementation of a new insurance regulation. We must emphasis on the fact that the data used are cross sectional and the variables reflect a microeconomic characteristics of policyholders. Thus, the data cannot be considered as ancient because the microeconomic behaviour does not change speedily as the case of time series panel data.
The variables used in this study can be divided into four major groups.
3.1. Contracts
The insurance company proposes four types of contracts that differ by the amount of the deductible:
• | Third Party Liability: the contract is compulsory for all drivers. The premium paid for this contract is low. | ||||
• | Full coverage contracts: the studied insurance offers three types of full contracts that differ by the amount of the deductible. It is noted that the difference between different deductibles is insignificant; thus we define a dummy variable for the contract choice (deductible choice) as follows: |
3.2. Characteristics of the driver
• | Gender: We define a dummy variable written as |
• | Age of the driver: we utilize three dummy variables describing beginning drivers, experienced drivers and seniors. | ||||
• | Occupation: for the occupation, many possible classifications can be used to distinguish between professions for policyholders. In this study, we propose seven dichotomous variables defined as follows: |
• | The residence area: we use four dummy variables to capture the effect of the immediate environment of the policyholders. |
3.3. Characteristics of the car
• Car’s origin: We define five dummy variables to capture the car’s country-of-origin effect.
• Use of the car: we define a dichotomous variable written as
3.4. Past involvement in accidents
• Accident number (N): The number of accident the drivers was involved in.
• No claim bonus rate (NCBR): is a discrete variable varying between 1 and 8. The no claim bonus rate is a system used to encourage drivers who have not been involved in any accidents for two consecutive insured years and to immediately punish drivers who have been involved in an accident. For example, an insured beginner driver must be classified in a class with no claim bonus rate equal to 8. If this driver commits an accident during the year, then he remains in the same class. If he is not involved in any accidents for two consecutive insured years, he will receive two points, and he will be reclassified in class 6.
4. Empirical results
4.1. Preliminary evidence
In this section, we analyse data to detect the marginal behaviour of various variables. We also make a bivariate analysis to investigate possible relationships between variables.
4.1.1. Univariate analysis
The studied insurance portfolio is composed of approximately 17.06% women and 82.94% males. Among these insurants, 60% utilize their vehicles for commuting and family use, and 40% of them use cars for commercial activities. It is observed that approximately 29% of policyholders live in big Tunisia, and 30% of them on the Coast. Concerning the occupation of drivers, approximately on half of them are employees, 21% are officials, and approximately 8% are unemployed.
The summary statistics also demonstrate that the average premium rate paid by policyholders is approximately 320 TND (176.55 USD), and the maximum premium paid is approximately 6000 TND (3,310.27 USD). Moreover, the number of accidents varies from 0 to 8 accidents, with an average value of 0.20, indicating a low frequency of accidents.
Tunisian’s vehicle fleet is composed of approximately 58.81% French cars, followed by German vehicles with 17.31% and Italian ones with approximately 12.62%.
Our data-set also includes information on the responsible accident number reported by the policyholder to the insurer. In this analysis, we describe the number of accidents for the whole portfolio, experienced drivers and beginner drivers.
As Table indicates, for the whole portfolio, we demonstrate that 12.32% of policyholders experienced at least one accident during the calendar year, for the beginner drivers, 10.82% had at least one accident and 13.73% of experienced drivers experienced at least one accident. According to this statistical result, we state that experienced drivers are likely to be more risky than young drivers. This result appears to be controversial compared to the literature (see, for example, Cohen (Citation2005), Grun-Réhomme and Benlagha (Citation2007)). However, this result could be explained by the risk aversion phenomenon. Because having used their car for a long time, drivers become more confident in driving, and then, they become less vigilant, and as an immediate consequence, there is an increase in the probability of their causing an accident. Controversially, beginner drivers are prudent, mostly in their first years of driving, leading to a decrease in the probability of their accident occurrence.
Table 1. Distribution of number of accidents
It is also noted that the distribution of the accident number is likely to be the same for beginner and experienced drivers. Generally, this distribution can be statistically adjusted to the zero inflated Poisson or a negative binomial distribution.
4.1.2. Bivariate analysis
To obtain a preliminary suggestion about the relationship between the deductible choice and accident occurrence, we analyse a two-way frequency table of policyholders’ coverage choices and accident occurrences (Table ). According to the deductible choice, for the whole portfolio, 79.25% of policyholders purchased comprehensive insurance contracts with the highest coverage (low deductible), and 20.75% of them desired the third party insurance with the lowest coverage (high deductible).
Table 2. Frequency table for coverage choice and accident occurrence
Regarding to accident occurrences, as usually expected in car insurance portfolios, the preponderance of policyholders have no accidents during the calendar year. In our case, as Table presents, approximately 88% of policyholders have no accidents, and approximately 12% of them incurred at least one accident.
For beginner drivers, among the 26.61% of policyholders who prefer third party coverage, 7.69% incurred at least one accident during the year, and among 73.39% of policyholders who purchased comprehensive coverage, 11.97% incurred at least one accident. We also note that the association between the deductible choice and accident occurrence is statistically significant at the 1% level.
For experienced drivers, among the 15.25% of policyholders who purchased the higher deductible, they had no accident, and 13.59% had at least one accident during the studied period, and among the 84.75% of policyholders who purchased the lowest deductible, 13.74% incurred at least one accident. We note that the association between deductible choice and the accident occurrence is not statistically significant.
To sum up the preliminary analysis of our data-set, we observe a strong association between deductible choice and accident occurrence for beginner drivers. This association could be regarded as the presence of residual adverse selection in the insurance portfolio. Thus, under this condition, the policyholders would self-select insurance policies according to their own risk types. The high-risk insured will choose high coverage and be charged a high premium rate, while the low-risk insured will desire low coverage and be charged a lower premium rate.
This preliminary analysis is important; however, a simple correlation does not consider the coexistence of many variables impacting the deductible choice and accident occurrences. Moreover, when modelling the relationship between these variables, many technical problems, such as heterogeneity and endogeneity, have to be considered. In the rest of this paper, we test the adverse selection hypothesis in the presence of such problems.
4.2. Seemingly unrelated probit
Table reports the results of the joint estimation of the probability of a policyholder choosing third party liability and accident occurrence employing the seemingly unrelated probit model exclusive of the deductible choice as an endogenous dummy described in Section 2.1.
Table 3. Seemingly unrelated probit model exclusive of the deductible choice as an endogenous dummy
As expected, the coefficient ρ is negative and significantly different from zero at the 5% level. This indicates that a joint estimation procedure might improve the efficiency of the estimates when there are common factors affecting the deductible choice and accident occurrences. Expressly, the unobserved heterogeneities of the deductible choice and accident occurrences are correlated. This implies that the two variables (errors) are correlated and the probability of one variable will be dependent on the probability of the other.
Compared to univariate probit and logit estimations, we find significant changes in some coefficients while no evidence of sign reversal was observed.
The results also highlight some determinants for accident occurrences and for the deductible choice.
For the first equation, the use of car is positive and significant at the 1% significance level. Therefore, on average, accident occurrences increase when we move from the family use of car to the business use.
In addition, the probability of incurring accidents decreases when we move from the coast regions to other geographic zones. Moreover, the results also demonstrate that the estimated parameter associated with the variable occupation 4 is significant and negative. Thus, the number of accidents decreases when we move from insurants with craft occupations to the other insurants.
Finally, the results prove that the probability of incurring accidents by beginners and seniors is positive and significant at the 1% level. Therefore, we may define two classes of risk, the first composed of beginner and senior drivers and the second composed of experienced drivers.
From results of the second equation, we observe that gender association is negative and significant at the 1% level. Thus, the probability of choosing the third party liability contract decreases when we move from male drivers to woman drivers.
It is also noted that the use of cars is significant at the 1% significance level. Therefore, policyholders who use cars for business prefer a contract with low deductibles.
It is interesting to note also that the no claim bonus rate is significant; policyholders with high no claim bonus rates tend to buy a contract with higher deductibles.
Finally, the results demonstrate that the origin 1, origin 2 and origin 3 are significant and positive. Thus, if the car was made in France, in Italy or in Germany, the policyholder would tend to buy a full insurance contract.
As mentioned above, this first modelling demonstrates that a joint estimation procedure might improve the efficiency of the estimates when there are common factors affecting the deductible choice and accident occurrences. However, this significant correlation in the two variables’ error terms does not mean that the two variables are correlated. Thus, we employ a recursive bivariate probit estimation to test whether the two variables are joint.
4.3. The recursive bivariate probit estimation
Table reports the results of the joint estimation of the probability of a policyholder choosing third party liability and accident occurrences employing a recursive bivariate probit estimation with the deductible choice as an endogenous dummy, as described in Section 2.2.
Table 4. Seemingly unrelated probit model with deductible choice as endogenous dummy
The results demonstrate that the p-value associated with the test of ρ = 0 equals 0.930 for the seemingly unrelated bivariate probit model, suggesting that the two endogenous variables are not jointly determined. That is, accident occurrences did not depend on the deductible choice.
This result can also be viewed as a presence of heterogeneities in both accident occurrences and deductible choices. However, the ρ test for the recursive bivariate model for both the accident occurrence and the deductible choice equations, as measured by the premium paid by policyholders, suggests that the two variates are jointly determined; thus, each equation should be analysed in the recursive bivariate probit model.
These findings may have an important implication for the insurance industry. Because the two endogenous variables are not jointly determined, the estimation and the analysis of equations can be performed separately.
The results of the recursive model also demonstrate that the sets of significant variables are basically the same as those obtained in the first modelling. However, we essentially discuss the deductible choice, the gender and the no-claim bonus rate as independent variable in the first equation.
First, for the accident occurrence equation, the results demonstrate that the deductible choice is significant at the 1% significance level. This result suggests some evidence for residual adverse selection in the studied insurance portfolio. Consequently, policyholders with high levels of risk buy insurance contracts with low deductibles or higher coverage and, conversely, policyholders with low levels of risk buy insurance contracts with high deductibles.
Moreover, for the first equation, the results highlight other determinants of accident occurrences. In fact, the parameter associated with gender is negative and significant at the 1% significance level. Therefore, on average, accident occurrences decline when we shift from the group composed of male to the group of women.
The results also demonstrate that the no-claim bonus rate variable is positively significant in explaining accident occurrences. It is well known that the no-claim bonus rate is strongly correlated with the expected number of accidents for the reference period. Conversely, our results exhibit a weak relationship between these two variables, by approximately 0.9%. This result can be argued by an asymmetry in the Tunisian no-claim bonus rate system.
4.4. Marginal effects of the deductible choice
The significant variables of the two models are relatively analogous. Moreover, the marginal effect estimates of the two models are similarly quite comparable.
The marginal effect results demonstrate that the probability of selecting third party liability declines by 0.22 when we consider women policyholders rather than males. Thus, women drivers are likely to be more risk averse than male drivers for because they tend to buy contracts with low deductibles.
Moreover, the results demonstrate that the probability of choosing a contract with a low deductible increases by 0.01% when a policyholder moves from a low risk class to a higher one.
In addition, the probability of selecting third party liability rises by approximately 11% when we shift from the coastal regions to other geographic zones. This can be argued by a wealth effect because the income of the working population is likely to be greater than that in other regions.
It is also noted that the probability of purchasing third party liability increases by 2.4% for unemployed policyholders. This result corroborates the proposal of the presence of the wealth effect in the decision of the contract choice (Table ).
Table 5. Marginal effects of the deductible choice
5. Conclusion
This paper examines the presence of the adverse selection problem by investigating the relationship between accident occurrences and the deductible choice. We account for potential heterogeneity in insurance contract choices by employing a seemingly unrelated probit model.
Our findings suggest some evidence for residual adverse selection in the studied insurance portfolio. Consequently, policyholders with high levels of risk buy insurance contracts with low deductibles, and, conversely, policyholders with low levels of risk buy insurance contracts with high deductibles.
In our empirical model, we found evidence that supports the need for correcting the endogeneity of the deductible choice on the accident number. Indeed, accounting for the deductible choice as an endogenous variable had a significant effect on the determinants of the accident number.
Our estimates also suggest the presence of a wealth effect on the decision of the contract choice. Therefore, policyholders with high incomes purchase insurance contracts with low deductibles and, conversely, policyholders with low incomes tend to purchase insurance contracts with high deductibles.
The general implication of our results is that the presence of heterogeneity could generate a bias when testing the presence of asymmetric information in a portfolio. The heterogeneity could also negatively impact the estimation of the different factors explaining the probability of accident and the deductible choice. We consider that our study has important implications for insurers and for the policy-makers, and suggest that the relevant authorities should pay particular attention to the model used in the estimation and the prediction of the accident occurrence and the choice of contracts by the policyholders. Indeed, the implemented model has to take into account the problem of heterogeneity to get accurate prediction of the variables explaining the accident occurrence and the choice of deductible. Moreover, the accurate predictions of the accident occurrence and the deductible choice are crucial for the insurer in developing a pricing policy. It is noticed that the insurer have to estimate a fair premium that must be acceptable by the insured and must generate profit for the insurer. Hence, neither the over-estimation nor the under-estimation of the premium can promote a powerful and stable insurance market.
Finally, in this empirical study, major caveats must be considered. First, our empirical results are based on data for a unique insurance portfolio, which could bring their validity and robustness into question. Although we have, mostly, found that our estimate results are consistent with previous empirical studies, (e.g. Cohen, Citation2001; Grun-Réhomme & Benlagha, Citation2007; Karaa & Benlagha, Citation2015; Puelz & Snow, Citation1994). Some bias might still be present because of the presence of over-dispersion in the claim number due to the problem of non-reporting or the phenomenon of hit and run. However, some studies in the literature suggest that adverse selection must be jointly investigated with the moral hazard problem, whereas testing for the presence of moral hazard requires data on drivers before and after concluding the insurance contracts; a dynamic panel data model is also suitable. This modelling is left for future work.
Funding
The authors received no direct funding for this research.
Additional information
Notes on contributors
Noureddine Benlagha
Noureddine Benlagha completed his PhD at the university of Paris II Assas in France, his Master degree at Paris II Assas university in France and his undergraduate studies at Université de Tunis Almanar in Tunisia.
Before joining the College of Business and Economics at Qatar, Noureddine has taught a broad number of courses at both the undergraduate and graduate levels et al. Imam Mohammad Ibn Saud Islamic University (KSA), University of Sfax (Tunisia), University of Paris II Assas (France) and at the Institute of higher education of Paris (France).
His research interests include, Information Economics, Insurance and risk management, Micro-econometrics, Financial markets and Time series. He has published widely on these topics in internationally respected peer-reviewed journals such as Applied Economics, Insurance and Risk Management, Applied Econometrics and international Development, Global Finance Journal and presented at major conferences such as Eurostat, The French Society of Statistics, and the Midwest Economics Association (USA).
References
- Benlagha, N., Charfeddine, L., & Karaa, I. (2012). Modelling accident occurrence in car insurance implementation on Tunisian data. Asian-African Journal of Economics and Econometrics, 12, 395–406.
- Chassagnon, A., & Chiappori, P. A. (1997). Insurance under moral hazard and adverse selection: The case of perfect competition. mimeo, DELTA. Retrieved from https://www.researchgate.net/publication/5067432
- Chiappori, P. A., & Salanie, B. (2000). Testing for asymmetric information in insurance markets. Journal of Political Economy, 108, 56–78.10.1086/262111
- Cohen, A. (2001). Asymmetric information and learning in the automobile insurance market. Cambridge, MA: Harvard University, Department of Economics.
- Cohen, A. (2005). Asymmetric information and learning: Evidence from the automobile insurance market. Review of Economics and Statistics, 87, 197–207.10.1162/0034653053970294
- Dionne, G., Doherty, N., & Fombaron, N. (2001). Adverse selection in insurance markets handbook of insurance (Vol. 22, pp. 185–243). Boston, MA: Kluwer Academic.
- Dionne, G., Gouriéroux, C., & Vanasse, C. (2001). Testing for evidence of adverse selection in the automobile insurance market. Journal of Political Economy, 109, 444–453.10.1086/319557
- Einav, L., Finkelstein, A., & Levin, J. (2010). Beyond testing: Empirical models of insurance markets. Annual Review of Economics, 2, 311–336.10.1146/annurev.economics.050708.143254
- Einav, L., Finkelstein, A., & Schrimpf, P. (2010). Optimal mandates and the welfare cost of asymmetric information: Evidence from the UK annuity market. Econometrica, 78, 1031–1092.
- Fabbri, D., Monfardini, C., & Radice, R. (2004). Testing exogeneity in the bivariate probit model: Monte Carlo evidence and an application to health economics. Bologna: Department of Economics, University of Bologna.
- Fuller, D. L. (2014). Adverse selection and moral hazard: Quantitative implications for unemployment insurance. Journal of Monetary Economics, 62, 108–122.10.1016/j.jmoneco.2013.10.003
- Greene, W. H. (1998). Gender economics courses in liberal arts colleges: Further results. The Journal of Economic Education, 29, 291–300.10.1080/00220489809595921
- Greene, W. H. (2003). Econometric analysis (5th ed.). Upper Saddle River, NJ: Prentice Hall.
- Grun-Réhomme, M., & Benlagha, N. (2007). Choix de contrat et sinistralité chez les jeunes conducteurs. Assurances et gestion des risques, 74–4, 505–532.
- Handel, B. (2013). Adverse selection and inertia in health insurance markets: When nudging hurts. American Economic Review, 103, 2643–2682.10.1257/aer.103.7.2643
- Karaa, I., & Benlagha, N. (2015). Testing for asymmetric information in Tunisian automobile insurance market. Mediterranean Journal of Social Sciences, 6, 455.
- Keane, M., & Stavrunova, O. (2016). Adverse selection, moral hazard and the demand for Medigap insurance. Journal of Econometrics, 190, 62–78.10.1016/j.jeconom.2015.08.002
- Koufopoulos, K. (2009). Optimal securities under adverse selection and moral hazard. Journal of Mathematical Economics, 45, 341–360.10.1016/j.jmateco.2009.03.003
- Li, C. S., Liu, C. C., & Peng, S. C. (2013). Bundled automobile insurance coverage and accidents. Accident Analysis and Prevention, 50, 64–72.10.1016/j.aap.2012.03.026
- Maddala, G. S. (1983). Limited-dependent and qualitative variables in econometrics (pp. 257–290). New York, NY: Cambridge University Press.10.1017/CBO9780511810176
- Maddala, G. S., & Lee, L.-F. (1976). Recursive models with qualitative endogenous variables. Annals of Economic and Social Measurement, 5, 525–545.
- Puelz, R., & Snow, A. (1994). Evidence on adverse selection: Equilibrium signaling and cross-subsidization in the insurance market. Journal of Political Economy, 102, 236–257.10.1086/261930
- Rothschild, M., & Stiglitz, J. (1976). Equilibrium in competitive insurance markets: An essay on the economics of imperfect information. The Quarterly Journal of Economics, 90, 629–649.10.2307/1885326
- Salanié, B. (1997). Théorie des contrats. Economica, 65–86.
- Shi, P., & Valdez, E. A. (2011). A copula approach to test asymmetric information with applications to predictive modeling. Insurance: Mathematics and Economic, 49, 226–239.
- Spence, M. A. (1978). Product differentiation and performance in insurance markets. Journal of Political Economy, 10, 427–447.
- Stiglitz, J. (1977). Monopoly, non-linear pricing, and imperfect information: The insurance market. Review of Economic Studies, 44, 407–430.
- Wilson, C. A. (1977). A model of insurance markets with incomplete information. Journal of Economic Theory, 16, 167–207.10.1016/0022-0531(77)90004-7