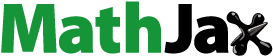
Abstract
In line with term structure theory, empirical studies suggest that it is difficult to beat the random walk in forecasting long-term interest rates. We ask whether consumer survey data on both mortgage interest rates and expected inflation help beat the random walk in forecasting the 30-year fixed rate mortgage. Using the vector autoregressive (VAR) modeling framework with the mortgage rate and consumer survey data as variables, we generate the mortgage rate forecasts for 1988–2016. Our forecast evaluation test results indicate that the VAR forecasts generally embody useful predictive information above and beyond that contained in the random walk forecasts for 2008–2016. The evidence is weaker for 1988–2007 in the sense that the VAR forecasts fail to outperform the random walk (but still contain distinct useful predictive information). In line with the notion that consumers are “economically” rational, our findings suggest that consumer survey data have become more informative due to the uncertainty created by the 2008 financial crisis.
Public Interest Statement
Long-term interest rates, including the 30-year fixed rate mortgage, are difficult to forecast. Both theory and empirical evidence suggest that the best forecast of the future rate is today’s rate (a naïve forecast). Despite the inherent difficulty, market participants and policy-makers are regularly engaged in producing more accurate forecasts than the naïve benchmark. This study is another attempt at providing accurate forecasts of mortgage rates for 1988–2016, using consumer survey data on both mortgage interest rates and expected inflation, derived from the long-running Michigan Survey of Consumers. Our results indicate that the consumer survey data are more helpful in producing accurate forecasts of mortgage rates for 2008–2016 than for 1988–2007. One explanation for this difference may be that the uncertainty created by the 2008 economic crisis has induced consumers to become more attentive by closely following relevant information and producing more informed assessments.
1. Introduction
Term structure theory suggests that long-term interest rates approximately follow a random walk (Pesando, Citation1979, Citation1980). This implies that such rates rapidly and fully reflect all relevant information so that future rate changes deviate from zero only in response to unexpected shocks. In line with this notion, empirical studies (including Baghestani, Citation2009; Brooks & Gray, Citation2004; Kolb & Stekler, Citation1996; Reichenstein & Shetty, Citation1989) show that professional forecasters fail to beat the random walk in forecasting long-term US Treasury bond rates.
Theoretically, in the absence of time-varying term premiums with the assumption that the term structure is determined according to the pure expectations theory, Reichenstein (Citation2006, p. 117) shows that:(1)
(1)
where is the long-term interest rate on an m-period bond at time t, and
is the long-term interest rate in t + 1 expected at time t. Similarly, rt is a one-period short-term interest rate at time t and rt+m is the short-term interest rate in t + m expected at time t. With m = 120 months for the 10-year treasury rate, the right-hand side term in Equation (1) is nearly zero and the future rate is expected to be today’s rate.
The mortgage industry uses the 10-year treasury rate as a benchmark in pricing fixed rate mortgages (Darrat, Dickens, & Al-Khazali, Citation2006). Accordingly, such rates often move in tandem with the 10-year treasury rate and thus display a random walk behavior (Baghestani, Citation2008a; Reichenstein, Citation1989). Following this argument, Baghestani (Citation2008b) shows that the consensus forecasts of the US 30-year fixed rate mortgage from the Blue Chip panel of experts fail to outperform the random walk forecasts for 1992–2004.
In this study, we utilize consumer survey data on both mortgage interest rates and expected inflation (derived from the long-running survey conducted by the University of Michigan) and ask whether they help produce a more accurate forecast of the 30-year fixed rate mortgage than the random walk benchmark. Using the vector autoregressive (VAR) modeling framework with the mortgage rate and consumer survey data as variables, we generate the mortgage rate forecasts for 1988–2016. Our forecast evaluation test results indicate that these forecasts generally embody useful predictive information above and beyond that contained in the random walk forecasts for 2008–2016. The evidence is weaker for 1988–2007 in the sense that the VAR forecasts fail to outperform the random walk (but still contain distinct useful predictive information). In line with the notion that consumers are “economically” rational (Baghestani, Citation1992), our findings suggest that consumer survey data have become more informative due to the uncertainty created by the 2008 financial crisis.
The format of this study is as follows: Section 2 describes the data in addition to the VAR and random walk forecasts. Section 3 presents the forecast evaluation test results. Section 4 concludes.
2. Data and alternative forecasts
The data on the US 30-year fixed rate mortgage come from the Federal Reserve Bank of St. Louis database. The consumer survey data are derived from the University of Michigan Surveys of Consumers (MSC). Every month since January 1978, the MSC selects a nationally representative random sample of at least 500 US consumers to probe sentiment on personal finances, buying attitude, business conditions, and expectations. We focus on consumers’ beliefs about both mortgage interest rates and expected inflation. In particular, one question asks, “Generally speaking, do you think now is a good time or a bad time to buy a house?” with the follow-up question “Why do you say so?” For the respondents who answer that it is a good time to buy a house, there are six options to select as reasons: Prices are low; prices will increase; interest rates are low; interest rates are rising (borrow in advance); it is a good investment; and times are good (prosperity). For the respondents who answer that it is a bad time to buy a house, there are five options to select as reasons: Prices are high; interest rates are high; it is a bad investment; there are uncertainties in the future (uncertain future); and I cannot afford it. For the purpose of this study, we utilize the number of respondents selecting the option that “interest rates are low” and the number of respondents selecting the option that “interest rates are high.”Footnote1 Baghestani, Kaya, and Kherfi (Citation2013) find that the interest rate options have directional predictability for US home sales. In addition, Baghestani (Citation2017) shows that consumer home buying attitudes can explain the behavior of US home sales in both tranquil and uncertain periods within 1978–2015.
As for expected inflation, the MSC asks: “During the next 12 months, do you think that prices in general will go up, or go down, or stay where they are now?” and “By about what percent do you expect prices to go up, on the average, during the next 12 months?” Using the individual responses, the MSC calculates and reports the consensus (mean) response as the measure of expected inflation. Ang, Bekaert, and Wei (Citation2007, p. 1206) note that “it is striking that the Michigan survey, which is conducted among relatively unsophisticated consumers, beats time-series, Phillips curve, and term structure forecasts.” The importance of expected inflation follows the Fisher (Citation1930) equation, which maintains that the nominal interest rate is the sum of the real interest rate and expected inflation. Baghestani (Citation2010) shows that expected inflation calculated as the past rate of change in the GDP deflator over 10 years helps beat the random walk in forecasting the US 10-year treasury rate.
In this study, we formulate two VAR forecasting models. The first one includes three variables: the change in the mortgage rate, the change in expected inflation, and the number of survey respondents who select the option that “interest rates are low” and thus it is a good time to buy a house. For the January 1982–January 1987 (1982.01–1987.01) sample period, the multi-equation Schwarz information criterion selects a VAR(2) model. That is, each of the three equations in the model includes a constant term and two lags of each variable on the right-hand side. We utilize the 1982.01–1987.01 parameter estimates of the model to generate the forecasts of the mortgage rate for 1987.02–1988.01. The values for 1987.02, 1987.04, 1987.07, and 1988.01 are, respectively, the 1-, 3-, 6-, and 12-month-ahead forecasts made at the end of 1987.01. Re-estimating the model for 1982.01–1987.02, we then use the updated parameter estimates to generate the forecasts of the mortgage rate for 1987.03–1988.02. The values for 1987.03, 1987.05, 1987.08, and 1988.02 are, respectively, the 1-, 3-, 6-, and 12-month-ahead forecasts made at the end of 1987.02. This procedure is repeated until the last set of forecasts is generated for 2016.12–2017.11 using the 1982.01–2016.11 parameter estimates. The values for 2016.12, 2017.02, 2017.05, and 2017.11 are, respectively, the 1-, 3-, 6-, and 12-month-ahead forecasts made at the end of 2016.11. Accordingly, the sample periods for the 1-, 3-, 6-, and 12-month-ahead forecasts are, respectively, 1987.02–2016.12, 1987.04–2017.02, 1987.07–2017.05, and 1988.01–2017.11. For the sake of consistency, however, we focus on the 1988.01–2016.12 period for all forecast horizons.
The second VAR model also includes three variables: the change in the mortgage rate, the change in expected inflation, and the number of survey respondents who select the option that “interest rates are high” and thus it is not a good time to buy a house. Again, for the 1982.01–1987.01 sample period, the multi-equation Schwarz information criterion selects a VAR(2) model. That is, each of the three equations in the model includes a constant term and two lags of each variable on the right-hand side. We utilize the same procedure outlined above to generate the 1-, 3-, 6-, and 12-month-ahead forecasts of the mortgage rate for 1988.01–2016.12. With the forecast horizon f (=1, 3, 6, and 12 months), the f-month-ahead VAR forecast of the mortgage rate (evaluated below) is a simple average of the two f-month-ahead forecasts obtained from the two VAR models described above.
As for the random walk forecasts, we set the 1-, 3-, 6-, and 12-month-ahead random walk forecasts equal to the actual mortgage rate for the last week of month t ending on Thursday.Footnote2 This ensures that the random walk forecasts are comparable to the VAR forecasts, making our forecast evaluation test results valid. Finally, we evaluate the forecasts separately for the 1988.01–2007.12 and 2008.01–2016.12 sample periods. The latter period includes the recent financial crisis.
3. Forecast evaluation results
Our evaluation focuses on answering the following three questions:
(1) | Are the VAR forecasts more informative than the random walk forecasts? | ||||
(2) | Are the mortgage rate forecasts unbiased? | ||||
(3) | Are the mortgage rate forecasts directionally accurate? |
Figure presents the forecasting timeline. As noted, At, At+1, …, and At+12 are the actual mortgage rates for the respective months. With the forecast horizon f (=1, 3, 6, and 12 months), PVt+f and Rt+f are, respectively, the VAR and random walk forecasts of At+f made at the end of month t. Figure presents the time plot of the actual mortgage rate for 1988.01–2016.12. As can be seen for 1988.01–2007.12 (2008.01–2016.12), the mortgage rate has a mean value of 7.69% (4.39%) with a high rate of 11.05 (6.48) and a low rate of 5.23 (3.35).
Figure 1. Timeline of the forecasts.
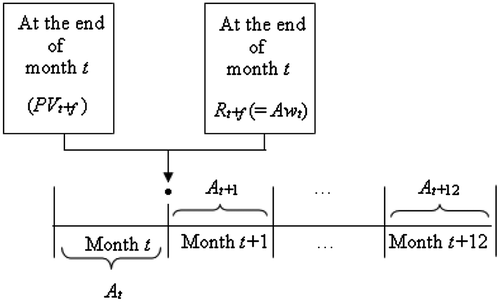
3.1. Are the VAR forecasts more informative than the random walk forecasts?
To answer, we estimate the following encompassing test equation:(2)
(2)
where the actual mortgage rate in month t (At) is known at the time of the forecast. We subtract At from both the actual (At+f) and forecast series to alleviate problems due to the non-stationarity of the series (Fair & Shiller, Citation1990). The VAR and random walk forecasts contain distinct predictive information when the estimates of γ1 and γ2 are both positive and significant. The VAR forecast contains more predictive information than the random walk when the estimate of γ1 is positive and significant and the estimate of γ2 is either insignificant or negative; the converse is also true. The VAR and random walk forecasts contain similar predictive information when the estimates of γ1 and γ2 are both insignificant. With the forecasts made in month t, the error term ut+f, while generally heteroscedastic, follows an (f − 1)-order moving-average process under the null hypothesis of rationality. In evaluating the 1-month-ahead forecast, we thus utilize the White (Citation1980) procedure to obtain the standard errors corrected for heteroscedasticity. In evaluating the 3-, 6-, and 12-month-ahead forecasts, we utilize the Newey and West (Citation1987) procedure to obtain the standard errors corrected for both the inherent serial correlation and heteroscedasticity.
Rows 1–4 of Table report the OLS parameter estimates of Equation (2) along with the correct standard errors for 1988.01–2007.12. As can be seen in each row, the estimates of γ1 and γ2 are both positive and significant. This means that the 1-, 3-, 6-, and 12-month-ahead VAR and random walk forecasts contain distinct useful predictive information, and combining the two forecasts for each forecast horizon produces a forecast that is more informative than the individual forecasts (Granger & Ramanathan, Citation1984). To keep it simple, we generate the combined forecasts (denoted as PCt+f) by taking a simple average of the VAR forecasts (PVt+f) and the random walk forecasts (Rt+f) for 1988.01–2007.12.Footnote3 In order to see whether the combined forecasts are more informative than the random walk forecasts, we estimate the following encompassing test equation:(3)
(3)
Table 1. Encompassing test results: VAR vs. random walk forecasts
where, again, At is subtracted from both the actual and forecast series to alleviate problems due to the non-stationarity of the series. Table reports the OLS parameter estimates of Equation (3) along with the correct standard errors. As can be seen, the estimates of δ1 are positive and significant but the estimates of δ2 are all insignificant. Thus, the 1-, 3-, 6-, and 12-month-ahead combined forecasts for 1988.01–2007.12 are more informative than the random walk forecasts.
Table 2. Encompassing test results: Combined vs. random walk forecasts (1988.01–2007.12)
Rows 5–8 of Table further report the OLS parameter estimates of Equation (2) along with the correct standard errors for 2008.01–2016.12. As can be seen in row 5, the estimate of γ1 is insignificant but the estimate of γ2 is positive and significant. This means that the 1-month-ahead random walk forecast is more informative than the corresponding VAR forecast. However, for the 3-, 6-, and 12-month-ahead forecasts in rows 6–8, the estimates of γ1 are positive and significant but the estimates of γ2 are all insignificant. This means that the 3-, 6-, and 12-month-ahead VAR forecasts embody useful predictive information above and beyond that contained in the random walk forecasts.
Given the results in Tables and , in what follows, we focus on evaluating the combined forecasts for 1988.01–2007.12 and the VAR forecasts for 2008.01–2016.12. Table reports the root mean squared forecast errors (RMSE) in addition to Theil’s U coefficients. The U coefficient is the mean squared forecast error of the combined (VAR) forecast divided by the mean squared forecast error of the random walk forecast for 1988.01–2007.12 (2008.01–2016.12). Consistent with the encompassing test results for 1988.01–2007.12, the 1-, 3-, 6-, and 12-month-ahead combined forecasts produce lower RMSEs than the corresponding random walk forecasts, with the U coefficients all below one. Consistent with the encompassing test results for 2008.01–2016.12, the same is true for only the 3-, 6-, and 12-month-ahead VAR forecasts.
Table 3. Relative forecast accuracy
3.2. Are the mortgage rate forecasts unbiased?
We examine unbiasedness by estimating:(4)
(4)
(5)
(5)
where Pt+f is a general representative for the combined and VAR forecasts, and (At+f − Pt+f) is the forecast error. The forecast is said to be unbiased if we cannot reject the individual null hypotheses that α = 0, β = 1, and α′ = 0 (Holden & Peel, Citation1990).
Rows 1–4 of Table report the OLS parameter estimates of Equations (4) and (5) with the correct standard errors for 1988.01–2007.12. As can be seen, the 1-, 3-, 6-, and 12-month-ahead combined forecasts are all unbiased since we cannot reject the individual null hypotheses that α = 0, β = 1, and α′ = 0. Rows 5–8 report the OLS parameter estimates of Equations (4) and (5) with the correct standard errors for 2008.01–2016.12. As can be seen in rows 6–8, the 3-, 6-, and 12-month-ahead VAR forecasts are also unbiased since we cannot reject the individual null hypotheses that α = 0, β = 1, and α′ = 0. However, the 1-month-ahead forecast in row 5 fails to be unbiased since we reject the null hypothesis that β = 1. Despite the mixed results on unbiasedness, the null hypothesis that α′ = 0 cannot be rejected for any of the forecasts in rows 1–8. This means that the combined forecasts (for 1988.01–2007.12) and the VAR forecasts (for 2008.01–2016.12) are all free of systematic bias. In line with this conclusion, the size of the mean error (ME) estimates in rows 1–8, ranging from 0.008 to 0.086, are all small compared to the corresponding mean absolute error (MAE) estimates, ranging from 0.125 to 0.632.
Table 4. Unbiasedness test results
3.3. Are the mortgage rate forecasts directionally accurate?
To investigate directional accuracy, we employ the procedure first introduced by Merton (Citation1981) and Henriksson and Merton (Citation1981) and later refined by Schnader and Stekler (Citation1990), among others. We start with presenting the two-by-two contingency table in Table . As noted, the actual change is (At+f − At) and the predicted change is (Pt+f − At). The numbers of correct sign predictions are n1 and n2, and the numbers of incorrect sign predictions are n3 and n4. We use these statistics to calculate πAll, πUp, and πDown. In particular, with n (=n1 + n2 + n3 + n4) defined as the sample size, πAll = (n1 + n2)/n is the overall directional accuracy rate; πUp = n1/(n1+n3) is the proportion of correctly predicted upward moves; and πDown = n2/(n2+n4) is the proportion of correctly predicted downward moves.
Table 5. Contingency table
Rows 1–4 of Table report these statistics for the combined forecasts for 1988.01–2007.12. In testing the null hypothesis of no (directional) association between the actual and predicted changes, we use Fisher’s exact test and the χ2 tests with and without Yate’s continuity correction (Sinclair, Stekler, & Kitzinger, Citation2010). As indicated by superscript b, we reject the null hypothesis for only the 1- and 3-month-ahead combined forecasts, meaning that these forecasts are directionally accurate. For the 1-month-ahead combined forecast in row 1, πAll = 0.71, πUp = 0.66, and πDown = 0.75. We employ the χ2 test described in Berenson, Levine, and Rindskopf (Citation1988, sec. 11.4.1) to test the null hypothesis that the proportion of incorrectly predicted upward moves (1 − πUp) equals the proportion of incorrectly predicted downward moves (1 − πDown). With the test p-value = 0.146 (> 0.10), reported in the last column of Table (row 1), we cannot reject the null hypothesis and thus conclude that the 1-month-ahead combined forecast implies symmetric loss and is, therefore, of value to a user who assigns similar cost (loss) to incorrect downward and upward moves in the mortgage rate. For the 3-month-ahead combined forecast in row 2, πAll = 0.59, πUp = 0.43, and πDown = 0.70. With the test p-value < 0.001, reported in the last column of Table , we reject the null hypothesis of no asymmetry and thus conclude that the 3-month-ahead combined forecast implies asymmetric loss and is, therefore, of value to a user who assigns high (low) cost to incorrect downward (upward) moves in the mortgage rate.
Table 6. Directional accuracy test results
Rows 5–8 of Table report the statistics for the VAR forecast for 2008.01–2016.12. As indicated by superscript b, we reject the null hypothesis for only the 1- and 3-month-ahead VAR forecasts, meaning that these forecasts are directionally accurate. For the 1-month-ahead VAR forecast in row 5, πAll = 0.62, πUp = 0.43, and πDown = 0.75, and for the 3-month-ahead VAR forecast in row 6, πAll = 0.69, πUp = 0.29, and πDown = 0.90. With the test p-values < 0.001, reported in the last column of Table (rows 5 and 6), we reject the null hypothesis of no asymmetry and thus conclude that the 1- and 3-month-ahead VAR forecasts imply asymmetric loss and are, therefore, of value to a user who assigns high (low) cost to incorrect downward (upward) moves in the mortgage rate.
4. Conclusions
Accurate forecasts of mortgage rates are important not only to housing market borrowers and lenders but also to policy-makers who are mindful of the strong link between the interest rate-sensitive housing market activity and business cycles (Bernanke, Citation2007). However, theory and empirical evidence suggest that long-term interest rates follow a random walk, meaning that the best near-term forecast of a long-term interest rate is today’s rate.Footnote4 In this study, we ask whether consumer survey data on both interest rates and expected inflation help beat the random walk in forecasting the 30-year fixed rate mortgage. Numerous studies have investigated the predictive information content of consumer sentiment for macroeconomic indicators with mixed results. The findings of Carroll, Fuhrer, and Wilcox (Citation1994) and Bram and Ludvigson (Citation1998) suggest that consumer sentiment contains useful predictive information for consumer spending. Croushore (Citation2005) replicates these studies with real-time data and shows otherwise. Batchelor and Dua (Citation1998) find consumer sentiment useful only in predicting the 1991 recession. More recent studies by Dees and Brinca (Citation2013), Christiansen, Eriksen, and Møller (Citation2014), Kellstedt, Linn, and Hannah (Citation2015), and Lahiri, Monokroussos, and Zhao (Citation2016), however, present evidence in support of consumer sentiment as a reliable predictor of economic indicators.
In this study, we use the vector autoregressive (VAR) modeling framework with the 30-year fixed rate mortgage and consumer survey data on both mortgage interest rates and expected inflation to generate the forecasts of the mortgage rate for 1988–2016. Our test results indicate that these forecasts generally embody useful predictive information above and beyond that contained in the random walk forecasts for 2008–2016. The evidence is weaker for 1988–2007 in the sense that the VAR forecasts fail to outperform the random walk (but still contain distinct useful predictive information.) Such findings lend support to the notion that consumers are “economically” rational. In particular, Baghestani (Citation1992, p. 282) points out that “To economically rational agents, the optimal forecast is that which balances the predictive benefit against the cost of gathering and processing information.” Accordingly, it is reasonable to argue that the uncertainty created by the 2008 economic crisis has induced consumers to become more attentive by closely following relevant information and producing more informative responses to the survey questions. This argument is consistent with Lahiri et al. (Citation2016, p. 1273) who show that consumer sentiment improves the accuracy of consumption forecasts (primarily through the services component). They further note that “the effect of sentiment is found to be stronger during the last recession when all categories of consumption seem to have been affected.” Additional evidence is provided by Baghestani (Citation2015) who uses the MSC data on both expected inflation and consumer sentiment to predict US gasoline prices for 2003–2014. He notes that the forecasts “are particularly more accurate for the period since 2008, reinforcing the notion that consumers are ‘economically’ rational” (p. 27).
Alternatively, one may argue that long-term interest rates have become easier to forecast due to the unusually low federal funds rates as a result of the Federal Reserve’s quantitative easing (QE) in response to the 2008 financial crisis. Jarrow and Li (Citation2014) show that the QE reduced the short- to medium-term (<12 years) forward rates but had almost no impact on longer term forward rates. Given that the mortgage industry uses the 10-year treasury rate as a benchmark in pricing the 30-year fixed rate mortgage (Darrat et al., Citation2006), the question of whether the mortgage rate has become easier to forecast awaits subsequent research.
Additional information
Funding
Notes on contributors
Hamid Baghestani
Hamid Baghestani has a PhD in Economics from the University of Colorado, Boulder, 1982. He is currently a professor of Economics at the American University of Sharjah, UAE. His research interests include time series analysis, macroeconometric modeling and forecasting, energy economics, monetary economics, and financial markets. He has published widely on these topics in internationally respected peer-reviewed journals such as Applied Economics, Energy Economics, Energy Policy, Journal of Business, Journal of Forecasting, Journal of Industrial Economics, Journal of Macroeconomics, and Oxford Bulletin of Economics and Statistics.
Notes
1. One question on the survey asks: “No one can say for sure, but what do you think will happen to interest rates for borrowing money during the next 12 months—will they go up, stay the same, or go down?” Using the individual responses, the MSC calculates and reports the index values (=down − up + 100) which represent the expected change in interest rates. Our results show that this index does not help produce accurate forecasts of mortgage rates. Dräger, Lamla, and Pfajfar (Citation2016, p. 90) analyze these data and conclude that “consumers have more difficulties in giving consistent expectations when interest rates would be expected to decrease.” Similarly, Baghestani and Kherfi (Citation2008, p. 731) conclude that, “for 1984–2005, consumers assigned much cost (loss) to incorrect predictions when interest rates were rising and almost no cost (loss) to incorrect predictions when interest rates were falling.”
2. The data on the 30-year fixed rate mortgage come from the Federal Reserve Bank of St. Louis website (https://fred.stlouisfed.org). These data are available on a monthly and a weekly basis. The weekly data end on Thursday. Also, the MSC data for month t are generally released before the end of month t to the fee-paying Thomson Reuters subscribers. The historical MSC data are available on the Michigan Surveys of Consumers website (http://www.sca.isr.umich.edu).
3. Generating the combined forecasts as a simple average of the VAR and the random walk forecasts is justifiable, since the estimates of γ1 (ranging from 0.429 to 0.525) and the estimates of γ2 (ranging from 0.392 to 0.436) in rows 1–4 of Table are not significantly different from 0.50.
4. As noted by Pesando (Citation1979), short-term interest rates do not necessarily display a random walk behavior. Existing evidence suggests that professional (consensus) forecasts of short-term interest rates can beat the random walk benchmark, although there is room for improving the accuracy of such forecasts as demonstrated by Baghestani (Citation2005).
References
- Ang, A., Bekaert, G., & Wei, M. (2007). Do macro variables, asset markets, or surveys forecast inflation better? Journal of Monetary Economics, 54, 1163–1212.10.1016/j.jmoneco.2006.04.006
- Baghestani, H. (1992). On the formation of expected inflation under various conditions: Some survey evidence. The Journal of Business, 65, 281–293.10.1086/jb.1992.65.issue-2
- Baghestani, H. (2005). Improving the accuracy of recent survey forecasts of the T-bill rate. Business Economics, 40, 36–40.10.2145/20050204
- Baghestani, H. (2008a). A random walk approach to predicting U.S. 30-year home mortgage rates. Journal of Housing Economics, 17, 225–233.10.1016/j.jhe.2008.06.001
- Baghestani, H. (2008b). Consensus vs. time-series forecasts of U.S. 30-year mortgage rates. Journal of Property Research, 25, 45–60.10.1080/09599910802397073
- Baghestani, H. (2009). Forecasting in efficient bond markets: Do experts know better? International Review of Economics and Finance, 18, 624–630.10.1016/j.iref.2008.10.007
- Baghestani, H. (2010). Forecasting the ten-year U.S. treasury rate. Journal of Forecasting, 29, 673–688.10.1002/for.1165
- Baghestani, H. (2015). Predicting gasoline prices using Michigan survey data. Energy Economics, 50, 27–32.10.1016/j.eneco.2015.04.015
- Baghestani, H. (2017). Do consumers’ home buying attitudes explain the behavior of US home sales? Applied Economics Letters, 24, 779–783.10.1080/13504851.2016.1229401
- Baghestani, H., Kaya, I., & Kherfi, S. (2013). Do changes in consumers’ home buying attitudes predict directional change in home sales? Applied Economics Letters, 20, 411–415.10.1080/13504851.2012.709597
- Baghestani, H., & Kherfi, S. (2008). How well do US consumers predict the direction of change in interest rates? The Quarterly Review of Economics and Finance, 48, 725–732.10.1016/j.qref.2007.06.002
- Batchelor, R., & Dua, P. (1998). Improving macro-economic forecasts: The role of consumer confidence. International Journal of Forecasting, 14, 71–81.10.1016/S0169-2070(97)00052-6
- Berenson, M. L., Levine, D. M., & Rindskopf, D. (1988). Applied statistics: A first course. New York, NY: Prentice Hall.
- Bernanke, B. S. (2007). Housing, housing finance, and monetary policy. Speech at the Federal Reserve Bank of Kansas City’s Economic Symposium, Kansas.
- Bram, J., & Ludvigson, S. C. (1998). Does consumer confidence forecast household expenditure? A sentiment index horse race. New York, NY: Federal Reserve Bank of New York Economic Policy Review.
- Brooks, R., & Gray, J. B. (2004). History of the forecasters: Naïve forecasts are better than the consensus WSJ forecast. The Journal of Portfolio Management, 31, 113–117.10.3905/jpm.2004.443329
- Carroll, C. D., Fuhrer, J. C., & Wilcox, D. W. (1994). Does consumer sentiment forecast household spending? If so, why? American Economic Review, 84, 1397–1408.
- Christiansen, C., Eriksen, J. N., & Møller, S. V. (2014). Forecasting US recessions: The role of sentiment. Journal of Banking & Finance, 49, 459–468.10.1016/j.jbankfin.2014.06.017
- Croushore, D. (2005). Do consumer-confidence indexes help forecast consumer spending in real time? The North American Journal of Economics and Finance, 16, 435–450.10.1016/j.najef.2005.05.002
- Darrat, A. F., Dickens, R. N., & Al-Khazali, O. (2006). Interactions between mortgage and other capital markets in the USA: Has financial deregulation made a difference? Applied Financial Economics, 16, 335–345.10.1080/09603100500186606
- Dees, S., & Brinca, P. S. (2013). Consumer confidence as a predictor of consumption spending: Evidence for the United States and the Euro area. International Economics, 134, 1–14.10.1016/j.inteco.2013.05.001
- Dräger, L., Lamla, M. J., & Pfajfar, D. (2016). Are survey expectations theory-consistent? The role of central bank communication and news. European Economic Review, 85, 84–111.10.1016/j.euroecorev.2016.01.010
- Fair, R. C., & Shiller, R. J. (1990). Comparing information in forecasts from econometric models. American Economic Review, 80, 375–389.
- Fisher, I. (1930). The Theory of Interest. New York, NY: A.M. Kelly.
- Granger, C. W., & Ramanathan, R. (1984). Improved methods of combining forecasts. Journal of Forecasting, 3, 197–204.10.1002/(ISSN)1099-131X
- Henriksson, R. D., & Merton, R. C. (1981). On market timing and investment performance. II. Statistical procedures for evaluation forecasting skills. The Journal of Business, 54, 513–533.10.1086/jb.1981.54.issue-4
- Holden, K., & Peel, D. A. (1990). On testing for unbiasedness and efficiency of forecasts. The Manchester School, 58, 120–127.10.1111/j.1467-9957.1990.tb00413.x
- Jarrow, R., & Li, H. (2014). The impact of quantitative easing on the US term structure of interest rates. Review of Derivatives Research, 17, 287–321.10.1007/s11147-014-9099-7
- Kellstedt, P. M., Linn, S., & Hannah, A. L. (2015). The usefulness of consumer sentiment: Assessing construct and measurement. Public Opinion Quarterly, 79, 181–203.10.1093/poq/nfu056
- Kolb, R. A., & Stekler, H. O. (1996). How well do analysts forecast interest rates? Journal of Forecasting, 15, 385–394.10.1002/(ISSN)1099-131X
- Lahiri, K., Monokroussos, G., & Zhao, Y. (2016). Forecasting consumption: The role of consumer confidence in real time with many predictors. Journal of Applied Econometrics, 31, 1254–1275.10.1002/jae.v31.7
- Merton, R. C. (1981). On market timing and investment performance. I. An equilibrium theory of value for market forecasts. The Journal of Business, 54, 363–406.10.1086/jb.1981.54.issue-3
- Newey, W. K., & West, K. D. (1987). A simple, positive semi-definite, heteroskedasticity and autocorrelation consistent covariance matrix. Econometrica, 55, 703–708.10.2307/1913610
- Pesando, J. E. (1979). On the random walk characteristics of short- and long-term interest rates in an efficient market. Journal of Money, Credit, and Banking, 11, 457–466.10.2307/1992047
- Pesando, J. E. (1980). On forecasting long-term interest rates: Is the success of the no-change prediction surprising? The Journal of Finance, 35, 1045–1047.10.1111/j.1540-6261.1980.tb03521.x
- Reichenstein, W. (1989). Martingales and efficient forecasts of effective mortgage rates. Journal of Real Estate Finance and Economics, 2, 317–330.
- Reichenstein, W. (2006). Rationality of naïve forecasts of long-term rates. The Journal of Portfolio Management, 32, 116–119.10.3905/jpm.2006.611812
- Reichenstein, W., & Shetty, S. (1989). Rationality of no-change forecasts of US Treasury interest rates. Advances in Quantitative Analysis of Finance and Accounting, 2, 93–103.
- Schnader, M. H., & Stekler, H. O. (1990). Evaluating predictions of change. The Journal of Business, 63, 99–107.10.1086/jb.1990.63.issue-1
- Sinclair, T. M., Stekler, H. O., & Kitzinger, L. (2010). Directional forecasts of GDP and inflation: A joint evaluation with an application to Federal Reserve predictions. Applied Economics, 42, 2289–2297.10.1080/00036840701857978
- White, H. (1980). A heteroskedasticity-consistent covariance matrix estimator and a direct test for heteroskedasticity. Econometrica, 48, 817–838.10.2307/1912934