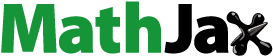
Abstract
This study explores the long-run and short-term relationship between the Mexico, Indonesia, South Korea, and Turkey (MIST) equity markets and the developed stock markets such as US, UK, Germany, Japan, Hong Kong, and Singapore. To start with, the author employs static bivariate and multivariate Johansen cointegration tests to test for long-run relationship between each of MIST equity markets and the developed stock markets. Subsequently, the author employs the recursive multivariate Johansen cointegration tests to garner a better understanding of the evolution of extent of integration between MIST and the developed stock markets. Static and Recursive Johansen Test findings reveal lack of consistent cointegrating relationship between MIST and developed markets (DM). Consequently, MIST equity markets do offer portfolio diversification avenues for international investors. On the short-term front, the time-varying correlations for each MIST-DM pair of stock indices were examined using the Dynamic Conditional Correlation (DCC) specification of the Multivariate GARCH. Of all the developed stock markets considered for this study, Mexico is found to exhibit high DCC with US and least amount of DCC with Japan while Indonesia is found to exhibit high DCC with Singapore and Hong Kong and least amount of DCC with US. Lastly, when it comes to South Korea, it exhibits the least amount of DCC with US and high DCC with Hong Kong, Singapore, and Japan.
Public Interest Statement
International Investors are constantly on the lookout for emerging markets that would enable them to better diversify their portfolios. Effective portfolio diversification is not only about investing in stock markets that are insulated from global turbulence but also about investing in those markets that are likely to serve as growth engines of the world. It is in this context that the recent emergence of equity markets pertaining to Mexico, Indonesia, South Korea, and Turkey (collectively referred to as MIST markets) from the shadows of the well-known BRICS markets offer an interesting avenue for exploration. International investors pertaining to developed equity markets such as US, UK, Germany, Singapore, Hong Kong, and Japan are more likely to assess (a) the extent of interdependence of MIST with the developed stock markets in the long-run; and (b) the comovement of MIST with the developed equity markets in the short-term. Consequently, this paper delves into these aspects in adequate detail keeping-in-view the fact that interrelationships are a dynamic phenomenon.
1. Introduction
In an effort to help global investors benefit from growth beyond the BRIC nations, a group of 11 nations (N11) that are likely to make increased contribution toward global GDP in the time to come were identified by Goldman Sachs. These nations include Mexico, Indonesia, South Korea, Turkey, Bangladesh, Egypt, Nigeria, Pakistan, Philippines, Vietnam, and Iran. Of the 11 nations, the economies of Mexico, Indonesia, South Korea, and Turkey (collectively called as MIST economies) accounted for 73% of the total N-11 GDP as of 2011. Taking cognizance of the emergence of N11 economies from the shadows of the BRICs, the prominent role played by the MIST economies amidst N11 group, and the growing expectation that these economies would serve as the growth engines of the world in the time to come, it is all the more critical for international investors looking for international portfolio diversification avenues to understand the long-run and short-term relationship between the equity markets pertaining to the MIST economies and the developed stock markets of the world. Consequently, this study is aimed at examining the time-varying cointegration and comovements of MIST equity markets with the developed stock markets of the world namely US, UK, Germany, Japan, Hong Kong, and Singapore. Section 2 offers a snapshot of literature on stock market integration, the significance of this study and its role in the broader scheme of things. Section 3 details the data utilized for this study. The mathematical underpinnings behind the different methodologies employed in this study and their respective outcomes are made available in Section 4. The author concludes in Section 5.
2. Literature review
Over the years, financial market integration, the evolution of the same, the reasons behind such integration and consequent implications for international portfolio diversification has attracted huge amount of academic attention. The literature in this arena is getting richer day by day as the recent 2008–2009 financial crisis has once again reinvigorated researchers’ quest to reassess the extent of stock market integration and to understand the dynamics behind the integration (or lack-thereof) of financial markets when punctuated by financial crises. In other words, the October 1987 crash, 1997 Asian crisis, the 1998 Rouble crisis, 1999 Brazilian crisis, and the recent 2008–2008 financial crisis have all been instrumental in advancement of literature pertaining to stock market integration. In this section, the author attempts to offer a snapshot of the burgeoning literature on stock market integration, taking into account the nature and scope of study on hand.
Earlier studies on financial market integration (Grubel, Citation1968; Hilliard, Citation1979; Levy & Sarnat, Citation1970; Ripley, Citation1973) that were aimed at exploring avenues for portfolio diversification from the point of view of international investors, found that the international markets offered the right incentives owing to lower correlation of foreign asset returns with respect to domestic assets held by investor. Foreign markets that are independent from the vagaries of the domestic market would offer international investors, an opportunity to garner exposure to factors that may be absent in the domestic market, and in the process improve risk-adjusted returns of their international portfolio. However, as the world gets more integrated, it accentuates integration of financial markets, which in-turn lead to decrease in potential diversification benefits from the point of an international investor (Pretorius, Citation2002).
Search for independent (interdependent) financial markets paved way for extensive studies of stock markets pertaining to emerging economies, for possible regional-level and world-level integration. Masih and Masih (Citation1997) found high level of integration among the stock markets pertaining to Taiwan, South Korea, Singapore, US, UK, Germany, and Japan from 1982–1994. In a subsequent study, Masih and Masih (Citation1999) found high level of integration amidst stock markets pertaining to Thailand, Malaysia, US, UK, Japan, Hong Kong, and Singapore from 1992 to 1997. Palac-McMiken (Citation1997) found cointegration amidst monthly ASEAN markets of Indonesia, Malaysia, Philippines, Singapore, and Thailand between 1987 and 1995. Chowdhury (Citation1994) found significant price and variance linkages between Asian Newly Industrialized Economies (NIEs), Japan, and US from 1986 to 1990. On the contrary, Huang, Yang, and Hu (Citation2000) found no cointegration amidst developed markets such as US and Japan, and Asian markets such as China, Hong Kong, and Taiwan. Likewise, Elyasiani, Perera, and Puri (Citation1998) found no interdependence between Sri Lanka and Asian developed markets for the time period 1989–1994.
On the Latin-American front, Choudhry (Citation1997) and Christofi and Pericli (Citation1999) found high level of inter-relationship amidst stock markets pertaining to Brazil, Chile, Columbia, Argentina, Venezuela, and Mexico from 1992–1997. Raj and Dhal (Citation2008) studied the extent of integration between India, Singapore, Hong Kong, Japan, US and US, and their results indicate prevalence of a weak multivariate co-integrating relationship amidst these markets, but absence of any bivariate co-integrating relationship between India and each of the other markets. On the European front, Gilmore and McManus (Citation2002), Gilmore and McManus (Citation2003) found no long-term relationship amidst Central and Eastern European (CEE) stock markets (Budapest, Prague and Warsaw) and developed stock markets (US and Germany). On the contrary, Voronkova (Citation2004) proved the prevalence of cointegration amidst Central European markets and between CEE and mature markets, when structural changes in the long-run relationship were accounted for. On similar lines, Syriopoulos (Citation2004) found CEE markets to be strongly linked to their mature counterparts, as opposed to their neighbors. Recently, Syriopoulos (Citation2007) found prevalence of long-term relationship amidst Central European emerging markets (Poland, Czech Republic, Hungary, and Slovakia) and developed stock markets (US and Germany) from 1999–2003. Further, Syriopoulos and Roumpis (Citation2009) find prevalence of long-term interdependence between equity markets pertaining to South Eastern European countries and developed stock markets (US and Germany). On the contrary, Égert and Kočenda (Citation2007) find no evidence of cointegration amidst Western, CEE stock markets using intra-day data from June ‘03 to February ‘05.
The burgeoning literature on stock market integration spanning different geographical locations is reflective of the prolonged, persistent academic quest to explore opportunities for short-term and/or long-term portfolio diversification for international investors. Effective portfolio diversification would entail not just investing in stock markets that are insulated from the global vagaries, but also investing in those markets that will serve as the growth force of the world in the time to come. In this context, the 2003 research paper by Goldman Sachs Investment Bank (Wilson & Purushothaman, Citation2003) wherein the economies of Brazil, Russia, India, and China (BRICs) were identified as the growth engines of the world, subject to these countries maintaining policies and developing institutions that are supportive of growth, attracted the attention of academia and industry alike. Bhar and Nikolova (Citation2007, Citation2009) found that, of all the BRIC economies, India showed the highest level of regional and global integration, followed by Brazil, Russia, and China. Negative relationship was found between conditional volatility of India with the Asia-Pacific region and that of China with the world, indicating avenues for portfolio diversification. However, the progressive integration of BRIC economies both regionally and globally called for international investors to invest in specific areas of growth within the BRIC economies as opposed the country indices. As a vindication of 2003 prediction by Goldman Sachs, the International Monetary Fund’s (October) 2012 world economic outlook noted that for the first time, the emerging economies enjoyed longer expansions and shorter downturns than advanced economies in the past decade.
Contrary to the events surrounding the Asian financial crisis, the BRIC economies showed resilience when confronted with the global financial crisis of 2008–2009 (Mallaby, Citation2012; December 4). Also, the average growth rate of the BRIC economies was four times faster than US from 2001 to 2010. Having said so, in light of recent underperformance of the BRICs where growth has slowed, and in an effort to help investors to benefit from growth beyond the BRIC nations, a group of 11 nations (N11) that are likely to make increased contribution to global GDP in the time to come were identified by Goldman Sachs (Martin, Citation2012, August 7). These nations include Mexico, Indonesia, South Korea, Turkey, Bangladesh, Egypt, Nigeria, Pakistan, Philippines, Vietnam, and Iran.Footnote1 Of the 11 nations, the economies of Mexico, Indonesia, South Korea, and Turkey (collectively called as MIST economies) accounted for 73% of the total N-11 GDP as of 2011.Footnote2 In light of the emergence of N11 economies from the shadows of the BRICs, the prominent role played by the MIST economies amidst N11 group, and the growing expectation that these economies would serve as the growth engines of the world in the time to come, it is all the more critical for international investors to understand the relationship between MIST and developed equity markets. Consequently, this study is aimed at (a) examining the long-run relationship between MIST equity markets and developed stock markets using static and recursive Johansen cointegration tests; and (b) examining the short-term comovements of MIST and developed equity markets using multivariate GARCH models.
3. Data utilized
The different national indices considered for this study include Mexican Bolsa IPC Index (Mexico), Jakarta Stock Exchange Composite Index (Indonesia), Korea Stock Exchange KOSPI Index (South Korea), Istanbul Stock Exchange National 100 Index (Turkey), S&P 500, FTSE100, DAX, FTSE STI, Hang Seng, and Nikkei 225. But for Istanbul National 100 Index, daily adjusted closing prices pertaining to all indices were downloaded from finance.yahoo.com. Daily closing prices pertaining to Istanbul National Index 100 were downloaded from Istanbul Stock Exchange website. But for Istanbul National 100 Index, data pertaining to all indices were available in their local currencies. In the case of Istanbul National 100 Index, closing data were made available by Istanbul Stock Exchange on euro-denominated basis based on Turkish Central Bank’s daily buying exchange rates on bank notes. Further, it has to be noted that, since July 1994, Istanbul Stock Exchange has two trading sessions in a day. For this study, Istanbul National 100 closing prices pertaining to the afternoon session (2:00 PM to 4:00 PM local time) are considered. Should afternoon session closing prices be unavailable, then closing prices pertaining to the morning session (9 AM to 12:30 PM) of the same day were considered. The time period of the study was 2nd December 2002 to 30th November 2012. Missing data were imputed using linear interpolation. Descriptive statistics of daily data in levels and daily logarithmic returns are shown in Tables and , respectively.Footnote3 The order of integration of all-time series considered for this study was examined using Augmented Dickey-Fuller test, Phillip-Perron test, and KPSS test. Test results indicated that all-time series considered were I(1) at levels/logarithmic levels and I(0) when transformed into logarithmic returns.Footnote4 Since all the time series considered for this study were found to be of the same order of integration, this offered an opportunity to examine the extent of cointegration (if any) between each of the MIST equity markets and the developed stock markets of the world.
Table 1. Summary statistics of daily data in levels
Table 2. Summary statistics of daily logarithmic returns
4. Methodologies employed and study’s findings
4.1. Exploring long-run relationship
To start with, bivariate Johansen (Citation1988, Citation1991) cointegration test is employed to test for extent of cointegration between MIST equity markets and the developed stock markets. The starting point for Johansen test is a Vector Autoregression model of order p.(1)
(1)
where zt is an n × 1 vector of variables that are I(1) and ɛt is zero mean white noise vector. The above VAR framework can be re-written as follows(2)
(2)
where and
Then the rank of coefficient matrix is determined. If the coefficient matrix has a reduced rank of r < n, then there exists n × r matrices of α and β each with rank r such that π = αβ′ and β′z is stationary, r is the number of cointegration relationships, the elements of α are known as the adjustment parameters in the vector error correction model and each column of β is a cointegrating vector. The Johansen methodology is concerned with testing the hypothesis of matrix π, using two different likelihood ratio tests namely the trace test and maximum eigenvalue test. The trace test tests the null hypothesis of r cointegrating vectors against the alternative of n cointegrating vectors. The maximum eigenvalue test tests the null hypothesis of r cointegrating vectors against the alternative of r + 1 cointegrating vectors.(8)
(8)
(9)
(9)
Should the test statistic exceed the critical value, it offers sufficient grounds to reject the null hypothesis and accept the alternative. Bivariate Johansen test outcomes, made available in Tables and , indicate absence of cointegration between any of the MIST equity markets and any of the developed equity markets.
Table 3. Bivariate Johansen test outcomes
Table 4. Bivariate Johansen test outcomes
In addition, multivariate Johansen cointegration tests were employed over the entire sample period to test for any cointegration between each MIST equity market and all developed stock markets in one go. Multivariate Johansen test results indicated absence of multivariate cointegration between each MIST equity market and all developed stock markets of the world.Footnote5
While the bivariate and multivariate Johansen tests employed above help in ascertaining cointegration or lack-thereof between MIST and the developed stock markets, it is a static assessment that fails to account for break points or regime switches. In order to garner a better understanding of the evolution of extent of integration between MIST and the developed equity markets, recursive multivariate Johansen cointegration tests were employed between each MIST equity market and all developed stock markets. The preliminary trace statistic pertaining to such recursive Johansen cointegration tests were based on a base period of one year (4 December 2002 to 3 December 2003). Subsequently, additional observations are considered one at a time toward the end of the evolving base period, and the trace statistic is re-estimated on an on-going basis until the last trace statistic is derived over the full sample period, which lasts from 4 December 2002 to 30 November 2012. All such recursive trace statistics generated over time were scaled by the 5% critical values.
Figures – depict the time-varying scaled trace statistic of multivariate Johansen frameworks comprising of a MIST equity market and all developed stock markets. Further, each of these Figures – contains two panels A and B. Panel A depicts the recursive scaled trace statistic for a Johansen multivariate framework represented by Equation (2), while panel B depicts the recursive scaled trace statistic of a reduced form representation of Equation 2, which gives a more cleaner estimation of the cointegrating vectors.Footnote6
For a multivariate Johansen framework to possess r unique cointegrating vectors, recursive scaled trace static of these r cointegrating vectors need to be consistently higher than one for a protracted period of time. Similarly, recursive scaled trace statistic of less than one in-connection with the remaining (n − r) vectors is reflective of the number of common trends in the multivariate framework comprising of n variables. Consequently, Panel A of Figures –, which reveal recursive scaled trace statistic that is greater than one for a protracted period of time for one of the seven vectors, is reflective of utmost one unique cointegrating relationship.
A cleaner reduced-form estimation of the recursive trace statistic, as given by panel B of Figures –, uncovers absence of any cointegrating relationship between each of the MIST equity markets and all of the developed markets, for most of the time. Panel B of these figures also uncovers transient cointegrating relationships in these multivariate frameworks which, more-or-less coincides with the timeline of the recent global financial crisis (mid-2007 to 2009).
To sum up, the findings pertaining to the static as well as the recursive Johansen Cointegration tests, reveal lack of consistent cointegrating relationship between MIST equity markets and all of the developed markets considered for this study. This is not to say that the MIST equity markets are not impacted by turbulence in global markets. However, such turbulence in global financial markets that manifest as transient cointegrating relationship between MIST and the developed stock markets do not last for a protracted period of time. In light of the lack of consistent cointegrating relationship between MIST and developed equity markets at all time, MIST equity markets do offer portfolio diversification avenues for international investors pertaining to the developed stock markets.
4.2. Exploring short-term relationship
Having examined the extent of integration between MIST and the developed stock markets, the rest of this section is devoted to examining the short-term dynamics between MIST and developed markets (DM). To be specific, the time-varying correlations for each MIST-DM pair of stock indices were examined using the Dynamic Conditional Correlation specification of the Multivariate GARCH model developed by Engle (Citation2002). The following DCC-GARCH model for a two-dimensional vector process for stock returns pertaining to each MIST-DM pair was employed.
where It − 1 is the information set at time t − 1. Each univariate error process has the specification ri, t = h1/2εi, t and the conditional variance , and it follows a univariate GARCH(1,1) process as shown below.
The conditional correlations are allowed to be time-varying by following the GARCH (1,1) model given below.
where is the time-varying covariance between standardized residuals.
is the unconditional covariance between
maximum likelihood estimation; and η and τ are non-negative scalars that must satisfy a stability constraint of the form η + τ < 1.
Finally, the dynamic conditional correlations between the two variables that constitute the multivariate frameworks are derived from the time-varying conditional covariances and conditional variances as shown below.
The parametric estimates pertaining to bivariate DCC-GARCH (1,1) models involving Mexico (Indonesia) and the developed stock markets considered for this study, the algorithm that yielded convergence for such estimations, the number of iterations it took for convergence and the volatility persistence for each univariate process estimated as part of the DCC-GARCH methodology, is made available as Appendices 1 and 2.Footnote7,Footnote8
All parametric estimates pertaining to the returns and volatility equations were found to be significant at 1% level for all 24 DCC GARCH(1,1) models estimated. Further, the sum of estimated coefficients (η + τ) of variance equation is close to unity, which implies that volatility exhibits a highly persistent behavior. The time-varying dynamic conditional correlations (DCC) obtained from each of the 24 DCC-GARCH models were then plotted. DCC plots involving Mexico and Indonesia are made available as Figures and , respectively.Footnote9
Of all the developed stock markets considered for this study, Mexico is found to exhibit high DCC with US and least amount of DCC with Japan while Indonesia is found to exhibit high DCC with Singapore and Hong Kong and least amount of DCC with US. Lastly, when it comes to South Korea, it exhibits the least amount of DCC with US and high DCC with Hong Kong, Singapore, and Japan.
5. Conclusion
The purpose of this paper is to examine long-run and short-term relationship between MIST and the developed stock markets. On the long-run front, the author employed bivariate and multivariate static Johansen Cointegration test, and the test findings indicated (a) absence of any bivariate cointegrating relationship between MIST-DM pairs and (b) absence of multivariate cointegrating relationship between each of MIST markets and all of the developed stock markets. Subsequently, the author employed the recursive multivariate Johansen cointegration tests to garner a better understanding of the evolution of extent of integration between MIST and the developed stock markets. The recursive scaled trace statistic pertaining to these estimations uncovered (a) absence of any cointegrating relationship between each of the MIST equity markets and all of the developed stock markets for most of the time, and (b) transient cointegrating relationships in these multivariate frameworks which more-or-less coincides with the timeline of the recent global financial crisis (mid-2007 to 2009).
Put simply, the findings pertaining to static and recursive Johansen tests reveal lack of consistent cointegrating relationship between MIST and developed equity markets. Consequently, MIST equity markets do offer portfolio diversification avenues for international investors pertaining to the developed stock markets.
On the short-term front, dynamic conditional correlation specification of bivariate MGARCH (1,1) model was employed, so as to examine the time-varying co-movements of each of MIST equity markets with each of the developed stock markets. This led to estimation of 24 bivariate DCC-GARCH models between different MIST and developed stock markets. DCC-GARCH model outcomes indicated significance of all parametric estimates pertaining to the returns and variance equations of all models, and prevalence of volatility persistence in all DCC models.
Of all the developed stock markets considered for this study, Mexico is found to exhibit high DCC with US and least amount of DCC with Japan while Indonesia is found to exhibit high DCC with Singapore and Hong Kong and least amount of DCC with US. Lastly, when it comes to South Korea, it exhibits the least amount of DCC with US and high DCC with Hong Kong, Singapore, and Japan.
The findings pertaining to this study are not only valuable to investing community, but it also goes a long way in contributing toward the vast burgeoning literature on global stock markets integration and comovements.
Funding
The author received no direct funding for this research.
Acknowledgments
The author would like to express his gratitude toward IFMR Sri City for offering requisite resources and for ensuring an academic environment that is conducive for research pursuits.
Additional information
Notes on contributors
Vinodh Madhavan
Vinodh Madhavan currently serves as an Associate Professor in Finance and Accounting (F&A) Area at IFMR Sri City. He successfully completed the Doctor of Business Administration program at Golden Gate University (GGU), San Francisco in Dec ‘09. Based on faculty recommendations, he was awarded the “2009-2010 Outstanding Graduate Student – Doctor of Business Administration” Award at GGU. Subsequent to his doctoral days, Dr Vinodh Madhavan has served as an Adjunct Faculty at GGU, as Malcolm S. M. Watts III Research Fellow at Technical Securities Analysts Association of San Francisco, and as an Assistant Professor at IIT Kharagpur, IIM Lucknow and at IMT Ghaziabad.
Notes
1. Iran is not open to foreign investors, owing to sanctions imposed by US and European Union.
2. It must be noted that the recent underperformance of the BRICs cannot be construed as irretrievable loss of their long-term potential. Jim O’ Neill of Goldman Sachs predicts that BRIC nations, despite that current lean patch, would grow an average of 6.5% a year through 2020, followed by 5.5% growth of the N11 group.
3. In the interest of brevity, descriptive statistics of daily data in logarithmic levels and time series plots of all indices considered for this study is not made available here. Interested readers may approach the author for the same.
4. Unit root tests were employed with and without a trend, and the test outcomes were qualitatively the same in either case.
5. In the interest of brevity, multivariate Johansen test outcomes are not made available here. Interested readers may obtain a copy of these results by contacting the author.
6. For a mathematical exposition of the reduced form representation of Equation (2), the readers may refer to Juselius (Citation2006).
7. Initially, the BFGS algorithm due to Broyden, Fletcher, Goldfarb, and Shanno was employed for all DCC-GARCH models. In the absence of convergence, the BHHH optimization algorithm due to Berndt, Hall, Hall, and Hausman was subsequently employed. In the event of no convergence despite employment of BFGS, followed by BHHH, a preliminary SIMPLEX algorithm for a certain number of iterations followed by the BFGS algorithm is employed. At this juncture, if the model still failed to converge, then a preliminary SIMPLEX algorithm for a certain number of iterations followed by BHHH algorithm was employed.
8. In the interest of brevity, bivariate DCC-GARCH model outcomes pertaining to other MIST - DM pairs, such as and limited to, South Korea – DM and Turkey- DM pairs, is not presented here. Interested readers may obtain a copy of these results by contacting the author.
9. In the interest of brevity, the DCC plots involving South Korea and Turkey are not presented here. Interested readers may obtain a copy of these results, by contacting the author.
References
- Bhar, R., & Nikolova, B. (2007). Analysis of mean and volatility spillovers using BRIC countries, regional and world equity index returns. Journal of Economic Integration, 22, 369–381.10.11130/jei.2007.22.2.369
- Bhar, R., & Nikolova, B. (2009). Return, volatility spillovers and dynamic correlation in the BRIC equity markets: An analysis using a bivariate EGARCH framework. Global Finance Journal, 19, 203–218.10.1016/j.gfj.2008.09.005
- Choudhry, T. (1997). Stochastic trends in stock prices: Evidence from Latin American markets. Journal of Macroeconomics, 19, 285–304.10.1016/S0164-0704(97)00016-5
- Chowdhury, A. R. (1994). Stock market interdependencies: Evidence from the asian NIEs. Journal of Macroeconomics, 16, 629–651.10.1016/0164-0704(94)90004-3
- Christofi, A., & Pericli, A. (1999). Correlation in price changes and volatility of major Latin American stock markets. Journal of Multinational Financial Management, 9, 79–93.10.1016/S1042-444X(98)00047-4
- Égert, B., & Kočenda, E. (2007). Interdependence between Eastern and Western European stock markets: Evidence from intraday data. Economic Systems, 31, 184–203.10.1016/j.ecosys.2006.12.004
- Elyasiani, E., Perera, P., & Puri, T. N. (1998). Interdependence and dynamic linkages between stock markets of Sri Lanka and its trading partners. Journal of Multinational Financial Management, 8, 89–101.10.1016/S1042-444X(98)00020-6
- Engle, R. (2002). Dynamic conditional correlation – A simple class of multivariate GARCH models. Journal of Business and Economics Statistics, 20, 339–350. doi:10.1198/073500102288618487.
- Gilmore, C. G., & McManus, G. M. (2002). International portfolio diversification: US and Central European equity markets. Emerging Markets Review, 3, 69–83.10.1016/S1566-0141(01)00031-0
- Gilmore, C. G., & McManus, G. M. (2003). Bilateral and multilateral cointegration properties between the German and Central European equity markets. Studies in Economics and Finance, 21, 40–53.10.1108/eb028768
- Grubel, H. G. (1968). Internationally diversified portfolios: Welfare gains and capital loss. American Economic Review, 58, 1299–1314.
- Hilliard, J. (1979). The relationship between equity indices on world exchanges. The Journal of Finance, 34, 103–114.10.1111/j.1540-6261.1979.tb02074.x
- Huang, B., Yang, C., & Hu, J. W. (2000). Causality and cointegration of stock markets among the United States, Japan and the South China growth triangle. International Review of Financial Analysis, 9, 281–297.10.1016/S1057-5219(00)00031-4
- Johansen, S. (1988). Statistical analysis of cointegration vectors. Journal of Economic Dynamics and Control, 12, 231–254.10.1016/0165-1889(88)90041-3
- Johansen, S. (1991). Estimation and hypothesis testing of cointegration vectors in Gaussian vector autoregressive models. Econometrica, 59, 1551–1580.10.2307/2938278
- Juselius, K. (2006). The cointegrated VAR approach: Methodology and applications. Oxford: Oxford University Press.
- Levy, H., & Sarnat, M. (1970). International diversification of investment portfolios. American Economic Review, 60, 668–675.
- Mallaby, S. (2012). Beware membership of this elite club. Financial Times, 4 December. Retrieved April 22, 2013, from https://www.ft.com/cms/s/0/51058960-3d44-11e2-b8b2-00144feabdc0.html#axzz2RCJCPUvu/
- Martin, E. (2012). Goldman sachs’s MIST Topping BRICS as smaller markets outperform. Retrieved April 22, 2013, from https://www.bloomberg.com/news/2012-08-07/goldman-sachs-s-mist-topping-brics-as-smaller-markets-outperform.html/
- Masih, A. M., & Masih, R. (1997). Dynamic linkages and the propagation mechanism driving major international stock markets: An analysis of the pre- and post-crash eras. The Quarterly Review of Economics and Finance, 37, 859–885.10.1016/S1062-9769(97)90008-9
- Masih, A. M., & Masih, R. M. (1999). Are Asian stock market fluctuations due mainly to intra-regional contagion effects? Evidence based on Asian emerging stock markets. Pacific Basin Finance Journal, 7, 251–282.10.1016/S0927-538X(99)00013-X
- Palac-McMiken, E. D. (1997). An examination of ASEAN stock markets: A cointegration approach. Asean Economic Bulletin, 13, 299–311.10.1355/AE13-3B
- Pretorius, E. (2002). Economic determinants of emerging stock market interdependence. Emerging Markets Review, 3, 84–105.10.1016/S1566-0141(01)00032-2
- Raj, J., & Dhal, S. (2008). Integration of India’s stock market with global and major regional markets. Regional Financial Integration in Asia: Present and future Bank of International Settlements (Paper No. 42). Basel: Bank for International Settlements.
- Ripley, D. M. (1973). Systematic elements in the linkage of national stock market indices. The Review of Economics and Statistics, 55, 356–361.10.2307/1927959
- Syriopoulos, T. (2004). International portfolio diversification to Central European stock markets. Applied Financial Economics, 14, 1253–1268.10.1080/0960310042000280465
- Syriopoulos, T. (2007). Dynamic linkages between emerging European and developed stock markets: Has the EMU any impact? International Review of Financial Analysis, 16, 41–60.10.1016/j.irfa.2005.02.003
- Syriopoulos, T., & Roumpis, E. (2009). Dynamic correlations and volatility effects in the Balkan equity markets. Journal of International Financial Markets, Institutions, and Money, 19, 565–587.10.1016/j.intfin.2008.08.002
- Voronkova, S. (2004). Equity market integration in Central European emerging markets: A cointegration analysis with shifting regimes. International Review of Financial Analysis, 13, 633–647.10.1016/j.irfa.2004.02.017
- Wilson, D., & Purushothaman, R. (2003). Dreaming with BRICs: The path to 2050. Goldman Sachs.
Appendix 1
Bivariate DCC-GARCH (1,1) Results: Mexico and developed equity markets
*Significance at 10%.
**Significance at 5%.
***Significance at 1%.
Appendix 2
Bivariate DCC-GARCH(1,1) results: Indonesia and developed equity markets
*Significance at 10%.
**Significance at 5%.
***Significance at 1%.