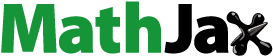
Abstract
This study sought to estimate the level of technical efficiency and total factor productivity of RCBs in Ghana using Stochastic Frontier Analysis (SFA). The total factor productivity (TFP) was decomposed into efficiency change and technical change over the study period. Quarterly data spanning 2009 to 2012 was sourced from the ARB-Apex Bank for a sample of 107 out of 137 RCBs in Ghana. The findings suggested that RCBs have room to improve on their technical efficiency and total factor productivity levels. Technical efficiency change, on average, was the main source of total factor productivity change during the period. The results suggest the shifting of the RCBs production frontier causing improvement in the TFP. The finding implies that efforts to improve efficiency and productivity of RCBs must be focused on improving the operational environment through rigorous efficiency analysis and monitoring by the regulator and management boards. The findings on the relative efficiencies also have implications for investment decision by prospective investors.
Public Interest Statement
An efficient financial institution serves as confidence booster for both investors (shareholders) and customers in the financial sector. Specific knowledge on the level of efficiency and productivity pertaining in the Rural Banks in Ghana is of interest to the regulator and the public that invests and saves with them. Recent cases of collapse of the microfinance institutions in Ghana make it more imperative to study the efficiency and productivity of these Banks and provide empirical evidence needed to deepen prudential requirements, supervision, and regulation. This will improve the operational efficiency and competitiveness of these financial institutions and attract investors into the sector in Ghana.
1. Introduction
Technical efficiency and productivity remain serious issue with regard to the financial and operational sustainability in banking. This is due, in part, to the recent collapse in microfinance institutions in Ghana, where most of them operated without rigorous prudential and regulatory oversights from the central bank of Ghana. The large number of Rural and Community Banks (RCBs) in Ghana demands that their performance be rigorously monitored to avoid any distress call and its negative effects on rural economic activities and development. This study attempts to estimate and benchmark the technical efficiency and sources of productivity of RCBs in Ghana.
Ghana embraced formal financial intermediation system in 1896. The country has since witnessed critical transformations in its financial institutions, especially in the banking industry, from the early days of a command market into a more competitive and market-oriented system. The transformations in the banking sector have been driven by technological, financial, and economic factors (Amidu & Hinson, Citation2006; Nabieu, Citation2013) and also by the motivation of the regulator to remove operational inefficiencies in the industry.
The Bank of Ghana introduced the concept of rural banking in Ghana in 1976. The motive was to mobilize rural financial resources to fund viable rural-based economic ventures whilst promoting rural development (Asiedu-Mante, Citation2011). This was against the bedrock that existing financial institutions in the country were reluctant, incapable, or slow in expanding financial services to rural communities. As a result, Rural and Community Banks (RCBs) were established as unit banks to serve medium-small and micro enterprises, agricultural activities, and households within demarcated rural polity (Nair & Fissha, Citation2010). On the average, a unit RCB serves within a range of 53,000 km2 radius (IFAD, Citation2000). However, recent developments show that most of the RCBs are operating outside their allotted demarcation and established agencies (branches) in the urban areas and thereby competing with the universal (commercial) banks. From the initial one RCB in 1976, the numbers of RCBs in Ghana have increased to 137 RCBs by 2017. It is also estimated that the RCBs network in Ghana has reached over 2.8 million depositors and 680,000 borrowers. Market statistics show that RCBs had a market share of slightly over 65% of depositors and 48% of borrowers in rural areas (Ampah, Citation2010). The financial and non-financial services that RCBs offer to their customers is seen as vital to the development of rural communities and their livelihoods. Literature accounts for the contributions of RCBs to the mobilization of rural savings and loans, the promotion of economic activities, and the enhancement of the socioeconomic lives of rural dwellers (Chowdhury, Citation2009; Steel & Andah, Citation2003).
Aside the contributions rural banking makes to the economy of Ghana, there are concerns of limited capacity and liquidation threats. Fierce competition from the universal banks, the proliferation of savings and loans companies, credit unions, and microfinance institutions are also a challenge to their operational sustainability. The RCBs are unequally distributed, with the fewest in the Upper East, Upper West, and Northern regions of Ghana (Adusei, Citation2016).
RCBs have had a checkered operational performance history. Up to 2007, a total of 23 distressed RCBs were closed down by the Bank of Ghana (BoG). The BoG in 2012 rated the operational performances of 85 RCBs as “satisfactory,” 19 of the RCBs were rated as “mediocre,” and the rest were considered “distressed” RCBs who needed close monitoring and nurturing to avoid being closed down (Bank of Ghana, Citation2012). Therefore, since 1988 there have been numerous interventions and reforms in the rural financial services sector aimed at improving the overall efficiency, productivity and sustainability of RCBs (See Table ). According to Chen, Skully, and Brown (Citation2005) and Matthews and Zhang (Citation2010) reforms improve efficiency and productivity in the banking sector. The prevalence of the interventions and reforms is a suggestion of the magnitude of the problems that bedevils the rural banking industry in Ghana. The reforms, steered by the Bank of Ghana, included regulatory, institutional, and financial restructuring. The outcome was an improvement in the operations of RCBs, though their performance is still considered sub-optimal.
Table 1. Selected interventions in the rural financial sector of Ghana
With the establishment of the Association of Rural Bank (ARB) Apex Bank, there have been continuous efforts to measure and monitor the performance of RCBs. The aim is to identify resource wastes and improve on efficiency and productivity, based on prudential financial norms required of all the formal banks in Ghana. The ARB-APEX Bank Efficiency Monitoring Unit (EMU) and the Bank of Ghana use accounting-based financial ratios approach to measure and rank RCBs performance. However, the accounting ratios approach to performance measurement focuses only on either the input or output side of the financial intermediation (production) process. It does not consider the input–output combinations in the intermediation process. Therefore, it is not able to fully identify inefficiencies and changes in productivity. This study uses a production approach that considers both the inputs and outputs sides to measure the performance of RCBs in Ghana.
Studies on the efficiency and total factor productivity (TFP) of RCBs in Ghana are largely unexplored, in spite of the importance of such knowledge in the management of RCBs toward operational and financial sustainability. It is for this cause that the thrust of this study is placed. We estimate the efficiency and productivity using the parametric Stochastic Frontier Analysis (SFA), which will deal with unobserved heterogeneities in the sample of RCBs, a consideration which is rare in the Ghana literature. Danquah, Barimah, and Ohemeng (Citation2013) used SFA but did not estimate the productivity of RCBs. Abdul-Majid, Saal, and Battisti (Citation2011), Hasan, Kamil, Mustafa, and Baten (Citation2012), Nguyen, Nghiem, Roca, and Sharma (Citation2016), and Silva, Tabak, Cajueiro, and Dias (Citation2017) among others are studies that applied SFA but did not focus on rural banks in their settings.
The rest of the study is structured as follows. Section 2 provides a brief review of the empirical literature on rural banks in general and specific to Ghana. Section 3 describes the data and defines the specific variables used in the estimations. Section 4 contains the empirical results and discussions. Conclusions are offered in section 5.
2. Related literature
2.1. Extant literature on efficiency and productivity
A large number of studies in the economics literature document the theoretical precepts of technical efficiency and productivity (Battese, Citation1992; Caves, Christensen, & Diewert, Citation1982; Farrell, Citation1957). On the empirical front, majority of the bank efficiency and productivity studies are found on commercial banks in developed, transition, and developing countries. Examples of such studies include Semih Yildirim and Philippatos (Citation2007) for transition economies of Europe and Ncube (Citation2009) for South African banking sector efficiency, Sufian (Citation2009) for Malaysian banks, Andrieș and Ursu (Citation2016) for European banks, Zhou, Placca, Jin, Liu, and Wu (Citation2017) for Togo, Zenebe Lema (Citation2017) for commercial banks in Ethiopia, and Silva et al. (Citation2017) for China.
According to Wijesiri and Meoli (Citation2015), the large amount of the empirical studies on technical efficiency and productivity in the banking sectors have employed parametric SFA and non-parametric Data Envelopment Analysis (DEA). The advantages and disadvantages of these methods have been extensively discussed in Hjalmarsson, Kumbhakar, and Heshmati (Citation1996), Murillo-Zamorano (Citation2004), and Silva et al. (Citation2017) for the interested reader. This paper uses the SFA method, which is discussed in section 3.1. Recent studies that have employed the SFA approach in commercial banking include Koetter, Karmann, and Fiorentino (Citation2006), Tahir, Bakar, and Haron (Citation2008), Abdul-Majid et al. (Citation2011), Hasan et al. (Citation2012), Nguyen et al. (Citation2016), and Silva et al. (Citation2017).
In the case of rural banks, a limited number of studies exist. These are mostly found in developing countries including India, Philippines, Indonesia, and Ghana. Almost all of these identified rural banks studies have rather applied the data envelopment analysis (DEA) with the exception of Danquah et al. (Citation2013). Another exception is Ahmed (Citation2014) who assessed the productivity performance of rural banks in India using accounting-based methods. Productivity was estimated in terms of labor, branch, return on asset (ROA) and investment (ROI), profit percent of business volume, etc. for a case study rural bank (Meghalaya Rural Bank) and compared to trends of rural banks in the national context. The study found the case bank relative to the national context utilized its resources efficiently and also that productivity changes are highly affected by the non-profit activities and low payment of loans by rural clients.
On the rural banks studies that used DEA approach, while there has been a consistent definition of the inputs specifications, output specifications appear to vary among the various studies. Reddy (Citation2006) and Khankhoje and Sathye (Citation2008) specified interest and non-interest income as outputs and interest and non-interest expenses as inputs for rural banks in India. Gordo (Citation2013) on the other hand used total loans as output and salary expenses, branches and interest expenses as inputs for rural banks in Philippines. For rural banks in Indonesia, Mongid and Tahir (Citation2010) defining bank total earning assets as outputs and total deposits and total overhead expenses as inputs. These inconsistencies might render it difficult to compare results across these studies and even to studies in the commercial banking sector. For instance, Gordo (Citation2013) found that for the period 1999–2009, Philippine rural banks experienced decline in productivity, which was mainly due to declines in technical efficiency changes with weak technological progress over the study period. The study was however not conclusive as the differences in efficiencies and changes in total factor productivity were not supported statistically. Khankhoje and Sathye (Citation2008) found that restructuring significantly improved the production efficiency of the rural banks in India. In the case of Mongid and Tahir (Citation2010) their results revealed that the estimates of technical efficiency was lower than scale efficiency, which indicates that portion of overall inefficiency, is due to producing below the production frontier rather than producing at an inefficient scale. The study also found that majority of the rural banks explored showed below optimal scale efficiency implying that output could be expanded to reach the optimal scale. Reddy (Citation2006) found significant growth in total factor productivity for rural banks situated in economically developed and low banking density regions. The weaknesses in these empirical studies are that they do not consider heterogeneities among the rural banks and therefore the results might be confounded by unobserved differences, which will bias the results.
2.2. Empirical studies in Ghana
In the case of Ghana, several studies can also be identified that estimated a measure of the technical and cost efficiency for universal banks, credit unions, and rural banks. The empirical discourse has however mostly focused on the mainstream universal banks (Adjei-Frimpong, Gan, & Hu, Citation2014; Frimpong, Citation2010; Saka, Aboagye, & Gemegah, Citation2012 etc.), credit unions (Oteng-Abayie, Owusu-Ansah, & Amanor, Citation2016), and microfinance institutions (Oteng-Abayie, Amanor, & Frimpong, Citation2011) in Ghana. The empirical literature on RCBs in Ghana is limited. To the best of our knowledge, Adusei (Citation2016) and Danquah et al. (Citation2013) are the only known studies that have attempted to estimate the technical efficiency of RCBs in Ghana.
Danquah et al. (Citation2013) estimated the technical efficiency of RCBs using the SFA. They employed the “true” random effect (Greene, Citation2005a) and the random parameter estimators in a panel data framework and characterized loans as output and deposits and fixed assets (physical capital) as inputs. The study used annual data of selected RCBs in Ghana from 2006 to 2011. The estimators selected were used to address the issue of unobserved heterogeneity among RCBs. Thus omitted unobserved heterogeneity in the SFA production model may always show up in the estimated inefficiency. The results also indicated that average technical efficiency of RCBs in Ghana, as a whole is 66% for the true random effects model, and 63% for the random parameter model. Both models indicate increases in efficiency levels from the 2006–2008 period, followed by a decrease in the 2008–2010 period, and a marginal increase from 2010 to 2011. On the other hand, Adusei (Citation2016) used DEA to estimate the technical efficiencies of RCBs and also characterized loans as output and deposits and fixed assets as inputs for the period 2009 to 2013. Though the study used quarterly data for the analysis, it conducted annual trend analysis, which looses the quarterly information depicted by the operations and reporting requirements of RCBs in Ghana. The study concluded that RCBs are on average inefficient. The weakness in Adusei (Citation2016) is that the DEA approach does not deal with the issue of observed and unobserved heterogeneity among RCBs by assuming that RCBs are homogenous despite, for example their regional differences.
Both Danquah et al. (Citation2013) and Adusei (Citation2016) concluded that RCBs were not fully efficient and therefore have efficiency improvement targets to set. This calls for the need to monitor productivity changes over time. From the existing literature, it appears that just estimating a measure of technical efficiency for the institutions are an end to themselves. However, it is important for bank managers to observe the improvement in efficiency and productivity and also know the sources of it. This necessitates the estimation of the total factor productivity (TFP) of RCBs and decomposes the measure into its components for effective decision-making.
The conspicuous gap in the empirical discourse concerning the estimation of the productivity of RCBs in Ghana is filled by the study. Clearly, the study contributes to the body of knowledge and policy by studying both the technical efficiency and productivity of RCBs in Ghana. We extend the limited study on RCBs in the following ways. Firstly, I estimate the technical efficiencies using SFA based on four estimators to deal with unobserved heterogeneity among RCBs. Secondly, I employed the more realistic translog production function which does not restrict all RCBs to be operating a constant returns to scale function. Thirdly, based on the technical efficiency estimates, derive the estimates for the total factor productivity (TFP) changes of the RCBs, and also identify the sources of the TFP changes by decomposing it into technical change and efficiency change. This is completely absent in the Ghana literature. Most importantly and in line with Berger and Humphrey(Citation1997) and Isik and Kabir Hassan (Citation2003), this study provides policy evidence for the banking regulation authorities on the outcomes of the sector reforms and the effects of changes in economic and business cycles on bank performance in Ghana.
3. Methodology and data
3.1. Measuring efficiency using SFA
SFA commonly employs Cobb-Douglas or translog functional forms applied to both cross-sectional and panel data to predict efficiency scores that can be subjected to statistical inference. Panel data stochastic frontier models are normally preferred over cross-sectional data, due to the fact that the latter, although produces unbiased estimates, is noted for inconsistent results requiring strong distributional assumptions on the form of inefficiency. Panel data, on the other hand, yields consistent results without requiring any robust assumptions about inefficiency effect, which makes it possible to develop time varying and time invariant characteristics in an SFA model. This study considers the translog functions to estimate production efficiency due to its known advantages (Greene, Citation2005b)Footnote1. The translog form brings more flexibility in terms of assumption of the shape of factor shares, and it is useful when the researcher is interested in interactive or integration effects. The translog model is commended for its advantage of relaxing the restrictions on demand elasticities and elasticities of substitution, as expressed in Cobb-Douglas functions. A translog specification of a production function is often found to fit data better than the Cobb-Douglas specification (Headey, Alauddin, & Rao, Citation2010).
The general translog function assuming a time trend includes the interactive terms and is represented in Equation (1) as follows:(1)
(1)
where Yi represents the output of the ith bank, Xk represents the vector of the logarithm of input, k, Xm represents the vector of the logarithm of input, m. βm, βk, γm, γk, θm, θk, θkm denote the parameters to be estimated, and ei is the error term.
In this study we considered stochastic frontier models with time-varying specificationsFootnote2, which include the Battese and Coelli (Citation1992) model (BC92), the Battese and Coelli (Citation1995) model (BC95), the Greene (Citation2005a) true random effects model (TRE), and the (2005) true fixed effects model (TFE). All models were estimated using the Maximum Likelihood methods (ML). The analysis was done using the Stata sfpanel command (Belotti, Daidone, Ilardi, & Atella, Citation2013). These models use different assumptions for the distribution of inefficiency terms. However, for the purposes of consistency, all the models were estimated, using a truncated normal distribution about the inefficiency. Again, for each of the models, conditional estimates were based on both the Battese and Coelli (Citation1995) and Jondrow, Knox Lovell, Materov, and Schmidt (Citation1982) measures.
3.2. Empirical SFA model specification
The model used in the study is based on the financial intermediation approach of technology specification proposed by Sealey and Lindley (Citation1977) and typified in efficiency research, including Hermes, Lensink, and Meesters (Citation2009) and Barry, Dacanay, Lepetit, and Tarazi (Citation2011). Berger and Humphrey (Citation1997) describe the appropriateness of the intermediation approach as superior for evaluating the importance of frontier efficiency, since it best illustrates a rural bank as a unit that accepts deposits backed by their capital assets and invests them, and also lends them when required and gains profits in the process.
Specifically, the empirical translog production frontier with a time-trend representation of technical change is specified as follows:(2)
(2)
where v = statistical noise, u = the error due to inefficiency, and betas represent coefficients or factor shares of the production function.
Four SFA models were estimated using the translog (TL) production function in Equation (2). The translog function was selected based on the knowledge from extant literature that the Cobb-Douglas specification is nested in the translog specification (Kaneda, Citation1982). Meanwhile, the translog specification also presents more information on interactive effects useful for policy-making, including performance, exhibiting all the attributes of the Cobb-Douglas specification. Hence, the translog is found more useful in practiceFootnote3. Ultimately, the choice was made on one of the four best-fit models useful for the productivity analysis.
3.3. Productivity analysis - multiplicative Malmquist TFP
Following the SFA analysis to generate the technical efficiency scores, we conducted the productivity analysis to estimate the technical change (TECH), efficiency change (EFFCH) and then total factor productivity change (TFP). Based on estimates of Equation (2), TECH, EFFCH, and TFP for the RCBs in 2009q1–2012q3 could be captured. The TEC is the ratio between TE score from the current period to the previous period. Mathematically,(3)
(3)
To estimate for the technical change (TECH), the partial derivative of the estimated translog function (2) is calculated, with respect to time (T). The TECH index then becomes the geometric mean between each two periods (Coelli, Rao, & Battese, Citation2005).(4)
(4)
Following the multiplicative Malmquist TFP concept, the TFP index is estimated by taking the product of EFFCH and TECH.(5)
(5)
3.4. Description of the data
A balanced panel data analysis was used in the estimation of the technical efficiency and productivity levels of the RCBs. Data for the study was obtained from the quarterly prudential returns of RCBs submitted to the Efficiency Monitoring Unit (EMU) in the Audit Department of the Association of Rural Bank (ARB) Apex Bank and to the Bank Supervision Division (BSD) of the Bank of Ghana. The study considered 107 RCBs (See Table A3 in appendix) in Ghana who have been operating consistently over the period 2009q1 to 2012q3. Sample RCBs are distributed over the 10 regions of Ghana. The data was based on the financial statements (Assets and Liabilities, BSD R2) of the RCBs. The original data-set included all the 137 RCBs, as at the end of the financial year, 2013. However, data was not consistently available for some RCBs for the study period, for which reason they were removed from the sample. The data, therefore, excludes 26 RCBs with less than 15 quarters of data points. The RCBs with missing values on inputs, and outputs variables within the study period were also excluded. Therefore, the total sample data form a strong balanced panel of 107 RCBs by 15 quarters, yielding 1,605 observations, which make up about 81% of all 137 RCBs during the study period (Table ).
Table 2. Regional distribution of RCBs in Ghana
3.5. Inputs and output variables specification
Inputs and outputs selected for the empirical analysis of the technical efficiency of the sampled RCBs in Ghana was based on existing literature, data availability and theoretical strands. Following the financial intermediation approach, net-loan (Lnnloan) is specified in this study as the output variable. Net loans is defined as Gross Loans and Advances less provisions for Loan Losses. The input variables included both net fixed assets and total deposits. The conventional definition of fixed assets describes them as tangible (capital and physical) assets that are in operational use and have a useful recurrent economic life of more than a year. In this study, net fixed asset (LnFxasst) of RCBs is measured as total assets (property and equipment) less accumulated depreciation. Deposits (LnDep) are defined in this study as current deposits, savings deposits, and time deposits from customers and other banks. Natural log values were used. Among the studies that have used these inputs and output variables include Elyasiani and Mehdian (Citation1990), Casu and Molyneux (Citation2003), Isik and Kabir Hassan (Citation2003), Danquah et al. (Citation2013), Sufian and Kamarudin (Citation2014), Danquah and Quartey (Citation2015), and Adusei (Citation2016). The descriptive statistics of the data is reported in Table .
Table 3. Descriptive statistics for input and output variables
It is evident from Table that net loans, total deposits, and fixed assets of the sample RCBs increased steadily during the study period. The respective average annual growth rates are 24, 27, and 29% over the period 2009 to 2012. This implies that the industry was vibrant and kept expanding annually. The growth in fixed assets might point to the increased outreach drive of RCBs through the acquisition of property and equipment (e.g. the establishment of new agencies and acquisition of computers and ICT equipment, among others). This growth in fixed assets is supported by the corresponding increase in both total deposits mobilized and the total loans disbursed over the same period.
4. Empirical results
4.1 Parameter estimates of SFA models
The maximum likelihood estimates of the parameters of the selected translog stochastic frontier production functions (Equation (2)) are presented in Table to enable a comparison of the models. From the results, the parameter estimates appear reasonably consistent in magnitudes across the various models. All the time, parameters are statistically significant and negatively signed, indicating some technical regress. Moreover, the estimates of variance parameters, sigma_u, sigma_v, and lambda (λ) are mostly statistically significant for all the models, suggesting evidence of technical inefficiency in the data as expected (Hjalmarsson et al., Citation1996; Sharma, Leung, & Zalenski, Citation1997; Wadud & White, Citation2000). For instance, lambda (γ), which is significant at 1% in all models shows that null hypothesis of no technical inefficiency effects is rejected. Thus, it can be concluded that a significant amount of the variation in the composite error term (v-u) is due to the inefficiency component.
Table 4. Parameter estimates for the stochastic frontier models
According to Jung and Pyo (Citation2009), using different models with different assumptions underlying might lead to different rankings of estimated efficiency. It is, therefore, important to choose the model that is suitable, considering the available data for the purposes of further analysis. The SFA estimates in Table were subjected to the Likelihood Ratio Test (lrtest) with the null hypothesis to find the stochastic production frontier model that best fits the data (see Table ). The χ2 statistic (LR χ2(66) = 2,499.36) rejects the null hypothesis of no difference among the models at 1% significance level, and judging from the minimized AIC and BIC information criteria, the true fixed effect model (Model 2) is selected for the subsequent prediction of technical efficiency, technical change and total factor productivity.
Table 5. Likelihood-ratio test of models
4.2 Technical efficiency scores derived from SFA
This section presents the results for the SFA technical efficiencies derived from the true fixed effect translog Model 2 for the RCBs. The results are presented in quarterly averages and by individual RCBs and followed with discussions.
4.1 Quarterly mean technical efficiency
The results presented in Figure show that, over the period under study, RCBs’ technical efficiency fluctuated. It can be observed that the mean quarterly measure of technical efficiency was highest in the third quarters of each year, followed by lower efficiency levels in the fourth quarter. The trends show that the technical efficiency level rises from the first quarter and peaks during the 3rd quarter of each year and falls by the 4th, as shown in Figure . This is interesting to note, because the third quarter is known to be the time when RCBs actively lend to businesses to finance trade activities in preparation for the Christmas season and offer individual loans to finance schooling, and other consumption expenditure by working and salaried workers. The trend, therefore, could be explained by the quarterly (seasonal) shifts in economic activities, which affect banking operations. The third quarter is the peak of business activities in the economy of Ghana. The mean technical efficiency for the period is 80.12%, indicating that RCBs could improve on inputs (fixed assets and deposits) used to achieve the same level of outputs (loans).
4.2. Technical efficiency results for individual RCBs
In this section, we present the mean technical efficiency scores of the individual RCBs over the study period, 2009q1 to 2012q3. A couple of comments can be made from Figure . First, it is observed on average that no RCB was fully technical efficient over the study periodFootnote4. Second, there is low variability in the efficiency estimates among RCBs relative to the overall mean score (0.80). This is indicative of the homogeneous characteristics of the RCBs in the industry. From the mean results, RCB 34 appeared the most efficient (0.94), and RCB 44 was the least efficient (0.55), in relative terms. The ranking for RCB 44 is not surprising, as it has currently ceased operation, after the ARB Apex Bank rated it as non-satisfactory. The ranking of RCBs also appears to include about 9 banks that are rated among recent top Ghana Club 100 companies. These include RCBs 65, 3, 58, 63, 73, 86, 98, 23, and 90.
Again, it is observed that most of the RCBs (53) fell within the middle bounds with technical efficiency ranging from 75.54–87.21%. A fairly equal number of RCBs were, however, found at the upper and lower bounds, as shown in Table . Thus a sizable number of RCBs lag behind and need more attention to improve their technical efficiency. About fifty percent of RCBs could be classified as moderate.
Table 6. Technical efficiency bounds and bank classification
4.3 Estimates of total factor productivity (TFP)
The productivity indices were derived from the best-fit SFA model using the multiplicative Malmquist procedure outlined in Equations ((3)–(5)).
4.3.1 TFP changes for individual RCBs
Table A2 in the appendix reports the summary of the estimates of productivity changes and components for the individual RCBs between 2009Q1 and 2012Q3. Several observations can be made from the individual reports. Firstly, on technical efficiency change, 48 out of the 107 RCBs under study suffered a regression in productivity over the study period, whilst 2 RCBs had a stable efficiency change. The rest of the RCBs achieved an improvement in efficiency over the period. Secondly, on technological growth, it is revealed that 10 (9.3%) of the RCBs experienced productivity declines ranging from −2.4 to −0.02%. Also, 95 RCBs (representing 88.8%) attained growth in technical progress (ranging from 0.087 to 3.44%). Two RCBs, however, had no change in their technical growth. Thirdly, 75 (70%) RCBs obtained an improvement in productivity (TFP) ranging from 0.03% to 21.358%. Furthermore, 30 out of the 107 RCBs had a regression in productivity change over the period. The productivity of two RCBs remained unchanged. As identified earlier, the sources of poor productivity performance within the sector were largely due to technical efficiency declines (with the exception of a few banks), at least in the short term.
4.3.2 TFP changes—Quarterly means for all RCBs
Figure provides a graphical presentation of the productivity growth and its components for the study period. These components are classified as the sources of the total factor productivity (TFP) growth. It can be observed that, overall, RCBs on average experienced progress in total factor productivity of 2.3%. There were also observed improvements in TFP for all the quarters within the study period, except for the 2009Q3/2009Q4, 2009Q4/2010Q1, 2010Q3/2010Q4, and 2011Q3/2011Q4 periods. Again, the results showed that during the period, TFP decreased for every third to fourth quarter of the year and rose at every first to second quarters. Banking experience in Ghana showed that, usually, banks intensify their marketing and operational activities from the third to fourth quarter. As a result, the falls in total factor productivity could be due to either managerial inefficiency and/or intensified competition that make some banks relax their credit scoring and client selections.
A further breakdown of the TFP into its components: technical efficiency change (EFFCH) and technical change (TECH) results, showed that, overall, an improvement was observed for all the quarters of the years in technical efficiency change, averaging 1.3% from 2009Q1 to 2012Q1. Also, it is shown that, except for the 2009Q3/2009Q4, 2009Q4/2010Q1, 2010Q3/2010Q4, and 2011Q3/2011Q4 periods, there was efficiency improvement in all the periods. Just as was explained under TFP, decreases in efficiency changes were observed for every third to fourth quarter of the year, and increase for every second to third quarter, from 2009 to 2012. The fall in efficiency changes in the third to fourth quarter confirms the assertion that, on most occasions, the resultant decrease in productivity growth is largely due to managerial inefficiencies.
For TECH, the evidence showed that there was technological growth consistently for all the quarters of the years. Overall, an average percentage of 1% was estimated for technical change for 2009–2012. The improvement in both efficiency change and technological growth of 1.3% and 1%, respectively, indicates that both significantly contributed to the growth in total factor productivity for the period.
Considering quarter-by-quarter trends, it appears that, overall, changes in TFP for the periods 2009Q3/2009Q4, 2009Q4/2010Q1 and from the last quarter of 2011, through to the third quarter of 2012, were powered solely by technical change. Technical efficiency change was also identified as the major source of total factor productivity movements from the first quarter of 2009 through to the third quarter of the same year. However, for the periods between 2010Q4/2011Q1 and 2011Q1/2011Q2, TECH and EFFCH contributed simultaneously to significantly power the movement in total factor productivity.
4.3.3 Cumulative change of TFP and its components
A further analysis, using the cumulative change of TFP for the period, is presented in Figure to compare the endpoints quarters from the beginning period, 2009Q1. Interestingly, the results appear to confirm the initial finding that productivity improved during the period. Cumulatively, there was a 32.3% growth in total factor productivity over the entire period for the RCBs. The RCBs, thus, expanded 32.3% of output in 2012Q3, compared to 2009Q1. The cumulative trends for technological change also indicated an improvement (outward shift of the frontier) of 14.1% between 2009Q1 and 2012Q3, while the cumulative technical efficiency showed a progress (efficiency catch-up) of 18.6%. The implication is that, while relatively less technically efficient RCBs did catch-up with more technically efficient ones in recent quarters, the presence of economies of scale ensured overall improvement in the rural banking sector.
Figure also shows that, at the end of the period under study, with the movement in productivity, though influenced by both EFFCH and TECH from the first quarter of 2009 through to the second quarter of 2011, TFP changes have been propelled by EFFCH. This observation confirms the conclusions drawn from Figure that improvements in TFP during the period, 2009–2012, were caused by the shifting of rural banking production frontiers and efforts to close the gaps between actual and optimal output, represented by EFFCH. As a result, any effort which helped to close the efficiency gaps or even just maintain TE scores, will push up TFP further.
5. Conclusion
In this study, we have estimated and ranked the technical efficiency of 107 RCBs in Ghana, using the SFA method. We also estimated the total factor productivity of the RCBs and decomposed it into efficiency change and technical change. Using a very still unexplored data from the Rural and Community Banks in Ghana for this purpose, a number of important findings emerged from the analysis.
First, the mean technical efficiency score of 80.12%, indicates the fact that the RCBs can, on average, reduce input usage by 19.88%. This average technical efficiency score suggest there is still significant potential for further efficiency improvement in the rural banking industry. Generally, the efficiency estimates were found to follow some quarterly variations aligned with economic activities in Ghana. High technical efficiency tends to be achieved in periods of high economic activities. The results support both Danquah et al. (Citation2013) and Adusei (Citation2016). Secondly, RCBs 63, 65, and 73, which belong to the Ghana Club 100 companies and are known to be high performing banks, were ranked high among the entire sample of RCBs. RCB 44 was ranked the least efficient over the period, and it is interesting to note that the bank has currently folded up due to managerial inefficiency. This yields some consistency with industry ratings. Assessing the number of efficient RCBs according to each estimator, the analysis reveal that 29 out of 107 RCBs were classified as high efficient (leading) RCBs with an efficiency score of 87% and above, whilst 23.4% of the total RCBs were classified as laggards with a technical efficiency score below 76%.
Thirdly, on the productivity front, the estimated indices demonstrate that the RCBs have, on average, experienced technical efficiency improvements during the study period with a quarterly change of 1.33%. This suggests that, on average, the RCBs got closer to the efficiency frontier. However, the results also showed that, though there were periods of technical regress, especially before the third quarter of 2011, such reductions were overturned afterwards. Technical efficiency change, on average, was the main source of productivity growth during the period with the results also suggesting the shifting of the production frontier causing improvement in the TFP.
From the findings, one important conclusion for policy is that there are considerable capacities for RCBs to improve their efficiency and productivity in the industry. Therefore, industry players (regulators and management boards) should continue to tailor resources toward the improvement in the operational environment and maintain optimal level of fixed assets commensurate with the funds mobilization to operate with. The regulator must continuous process of efficiency monitoring using more rigorous frontier analysis. Moreover, the relative efficiencies of the RCBs provide useful information for investors to consider where to put their capital for investment purposes.
Funding
The author received no direct funding for this research.
Additional information
Notes on contributors
Eric Fosu Oteng-Abayie
Eric Fosu Oteng-Abayie has a PhD in Economics and is currently a senior lecturer in the Department of Economics at Kwame Nkrumah University of Science and Technology, Kumasi, Ghana. He has experience in management and research consultant. He has published articles in the area of financial economics, macroeconomics, and research methodology. Eric’s current research focuses on economics of energy, health, and development issues. As a lecturer, he teaches Mathematical Economics, Advanced Macroeconomics, Labour Economics, and Research Methodology.
Notes
1. See Greene (Citation2005b) for more on the choice of functional form of the production function.
2. See Table A1 in the appendix for specifications of the selected models.
3. The results of the two models (CD and TL) were found not to be significantly different.
4. Note however that at some point within the study period, the RCBs achieved full technical efficiency. See maximum values of efficiency scores for each individual RCB in Figure .
References
- Abdul-Majid, M., Saal, D. S., & Battisti, G. (2011). Efficiency and total factor productivity change of Malaysian commercial banks. The Service Industries Journal, 31, 2117–2143. doi:10.1080/02642069.2010.503882
- Adjei-Frimpong, K., Gan, C., & Hu, B. (2014). Cost efficiency of Ghana’s banking industry: A panel data analysis. The International Journal of Business and Finance Research, 8, 69–86.
- Adusei, M. (2016). Determinants of bank technical efficiency: Evidence from rural and community banks in Ghana. Cogent Business & Management, 3, 1199519. doi:10.1080/23311975.2016.1199519
- Ahmed, J. U. (2014). Productivity analysis of rural banks in India: A case of Meghalaya rural bank. The NEHU Journal, XII, 53–76. ISSN: 0972-8406 53.
- Amidu, M., & Hinson, R. (2006). Credit risk, capital structure and lending decisions of banks in Ghana. Banks and Bank Systems, 1, 93–101.
- Ampah, S. K. (2010, September 7). Rural banks in Ghana collapsing. The Ghana Journal. Retrieved from https://www.theghanaianjournal.com
- Andrieș, A. M., & Ursu, S. G. (2016). Financial crisis and bank efficiency: An empirical study of European banks. Economic Research-Ekonomska Istraživanja, 29, 485–497. doi:10.1080/1331677X.2016.1175725
- Asiedu-Mante, E. (2011). Rural banking in Ghana. Accra: Combert.
- Bank of Ghana. (2012). Statistical bulletins. Retrieved from https://www.bog.gov.gh
- Barry, T. A., Dacanay, S. J. I. O., Lepetit, L., & Tarazi, A. (2011). Ownership structure and bank efficiency in six Asian countries. Philippine Management Review, 18.
- Battese, G. E. (1992). Frontier production functions and technical efficiency: A survey of empirical applications in agricultural economics. Agricultural Economics, 7, 185–208. doi:10.1016/0169-5150(92)90049-5
- Battese, G. E., & Coelli, T. J. (1992). Frontier production functions, technical efficiency and panel data: With application to paddy farmers in India (pp. 149–165). Berlin: Springer.
- Battese, G. E., & Coelli, T. J. (1995). A model for technical inefficiency effects a Stochastic Frontier function for panel data. Empirical Economics, 20, 325. doi:10.1007/BF01205442
- Belotti, F., Daidone, S., Ilardi, G., & Atella, V. (2013). Stochastic Frontier analysis using stata. Stata Journal, 13, 718–758.
- Berger, A. N., & Humphrey, D. B. (1997). Efficiency of financial institutions: International survey and directions for future research. European Journal of Operational Research, 98, 175–212. doi:10.1016/S0377-2217(96)00342-6
- Casu, B. & Molynuex, P. A. (2003). Comparative study of efficiency in European banking. Applied Economics, 35, 1865–1876.
- Caves, D. W., Christensen, L. R., & Diewert, W. E. (1982). The economic theory of index numbers and the measurement of input, output and productivity. Econometrica, 50, 1393–1414.10.2307/1913388
- Chen, X., Skully, M., & Brown, K. (2005). Banking efficiency in China: Application of DEA to pre- and post-deregulation eras: 1993–2000. China Economic Review, 16, 229–245. 10.1016/j.chieco.2005.02.001
- Chowdhury, A. (2009). Microfnance as a poverty reduction tool–a critical assessment ( Economic and Social Affairs DESA Working Paper No. 89). New York, NY: United Nations.10.18356/a39cdf00-en
- Coelli, T. J., Rao, D. S., & Battese, G. E. (2005). An introduction to efficiency and productivity analysis. London: Kluwer Academic.
- Danquah, M., Barimah, A., & Ohemeng, W. (2013). Efficiency measurement using a “True” random effects and random parameter Stochastic Frontier models: An application to rural and community banks in Ghana. Modern Economy, 2013, 864–870. doi:10.4236/me.2013.412093
- Danquah, M., & Quartey, P. (2015). Examining the determinants of efficiency using a latent class stochastic frontier model. Cogent Economics & Finance, 3, 1124741. doi:10.1080/23322039.2015.1124741
- Elyasiani, E., & Mehdian, S. M. (1990). A nonparametric approach to measurement of efficiency and technological change: The case of large U.S. commercial banks. Journal of Financial Services Research, 4, 157–168. doi:10.1007/BF00352569
- Farrell, M. J. (1957). The measurement of production efficiency. Journal of the Royal Statistical Society Series A, 120, 253–281.10.2307/2343100
- Frimpong, J. M. (2010). Investigating efficiency of Ghana banks: A non-parametric approach. American Journal of Scientific Research, 7, 64–76.
- Gordo, G. M. (2013). Estimating Philippine bank efficiencies using frontier Analysis. Philippine Management Review, 20, 17–36.
- Greene, W. (2005a). Fixed and random effects in Stochastic Frontier models. Journal of Productivity Analysis, 23, 7–32. doi:10.1007/s11123-004-8545-1
- Greene, W. (2005b). Reconsidering heterogeneity in panel data estimators of the Stochastic Frontier model. Journal of Econometrics, 126, 269–303. doi:10.1016/j.jeconom.2004.05.003
- Hasan, M. Z., Kamil, A. A., Mustafa, A., & Baten, M. A. (2012). A Cobb Douglas Stochastic Frontier model on measuring domestic bank efficiency in Malaysia. PLoS One, 7, e42215. doi:10.1371/journal.pone.0042215
- Headey, D., Alauddin, M., & Rao, D. S. P. (2010). Explaining agricultural productivity growth: An international perspective. Agricultural Economics, 41(1), 1–14. doi:10.1111/j.1574-0862.2009.00420.x
- Hermes, N., Lensink, R., & Meesters, A. (2009). Financial development and the efficiency of microfinance institutions. Retrieved from https://papers.ssrn.com/sol3/papers.cfm
- Hjalmarsson, L., Kumbhakar, S., & Heshmati, A. (1996). DEA, DFA and SFA: A comparison. Journal of Productivity Analysis, 7, 303–327. doi:10.1007/BF00157046
- IFAD. (2000). Ghana: Rural financial services project ( Pre-Appraisal Mission Working Paper: “Re-Packing the Rural Finance Sub-Sector in Ghana Poverty Gender and Rural Informal Sector Perspectives” and Working Paper: “Strategy for Mainstreaming Gender with a Specific Focus on Northern Ghana”.
- Isik, I., & Kabir Hassan, M. (2003). Financial deregulation and total factor productivity change: An empirical study of Turkish commercial banks. Journal of Banking and Finance, 27, 1455–1485. doi:10.1016/S0378-4266(02)00288-1
- Jondrow, J., Knox Lovell, C. A., Materov, I. S., & Schmidt, P. (1982). On the estimation of technical inefficiency in the stochastic frontier production function model. Journal of Econometrics, 19, 233–238. doi:10.1016/0304-4076(82)90004-5
- Jung, S., & Pyo, H. K. (2009). A Stochastic Frontier Analysis on firm-level total factor productivity and technical efficiency in Korea. Retrieved from https://gcoe.ier.hit-u.ac.jp/CAED/papers/id124_Jung_Pyo.pdf
- Kaneda, H. (1982). Specification of production functions for analyzing technical change and factor inputs in agricultural development. Journal of Development Economics, 11, 97–108. doi:10.1016/0304-3878(82)90045-1
- Khankhoje, D., & Sathye, M. (2008). Efficiency of rural banks: The case of India. International Business Research, 1, 140–149. doi:10.5539/ibr.v1n2p140
- Koetter, M., Karmann, A., & Fiorentino, E. (2006). The cost efficiency of German banks: A comparison of SFA and DEA (No. 2006, 10) ( Discussion Paper, Series 2: Banking and Financial Supervision).
- Matthews, K., & Zhang, N. (2010). Bank productivity in China 1997–2007: Measurement and convergence. China Economic Review, 21, 617–628. 10.1016/j.chieco.2010.06.004
- Mongid, A., & Tahir, I. M. (2010). Technical and scale efficiency of Indonesian rural banks. Banks and Bank Systems, 5, 80.
- Murillo-Zamorano, L. R. (2004). Economic efficiency and frontier techniques. Journal of Economic Surveys, 18, 33–77. doi:10.1111/j.1467-6419.2004.00215.x
- Nabieu, G. A. A. (2013). The structure, conduct and performance of commercial banks in Ghana. European Journal of Business and Innovation Research, 1, 34–47.
- Nair, A., & Fissha, A. (2010). Rural banking: The case of rural and community banks in Ghana ( Agriculture and Rural Development Discussion Paper 48).
- Ncube, M. (2009). Efficiency of the banking sector in South Africa (pp. 1–44). Johannesburg: University of the Witwatersrand.
- Nguyen, T. P. T., Nghiem, S. H., Roca, E., & Sharma, P. (2016). Bank reforms and efficiency in Vietnamese banks: Evidence based on SFA and DEA. Applied Economics, 48, 2822–2835. doi:10.1080/00036846.2015.1130788
- Oteng-Abayie, E. F., Amanor, K., & Frimpong, J. M. (2011). The measurement and determinants of economic efficiency of microfinance institutions in Ghana : A Stochastic Frontier approach. African Review of Economics and Finance, 2, 149–166.
- Oteng-Abayie, E. F., Owusu-Ansah, B., & Amanor, K. (2016). Technical efficiency of credit unions in Ghana. Journal of Finance and Economics, 4, 88–96. doi:10.12691/jfe-4-3-3
- Reddy, A. A. (2006). Productivity growth in regional rural banks. Economic and Political Weekly, 1079–1086.
- Saka, A. N. A., Aboagye, A. Q., & Gemegah, A. (2012). Technical efficiency of the Ghanaian banking industry and the effects of the entry of Foreign banks. Journal of African Business, 13, 232–243. doi:10.1080/15228916.2012.727755
- Sealey, C. W., & Lindley, J. T. (1977). Inputs, outputs, and a theory of production and cost at depository financial institutions. The Journal of Finance, 32, 1251–1266. doi:10.1111/j.1540-6261.1977.tb03324.x
- Semih Yildirim, H., & Philippatos, G. C. (2007). Efficiency of banks: Recent evidence from the transition economies of Europe, 1993–2000. The European Journal of Finance, 13, 123–143.10.1080/13518470600763687
- Sharma, K. R., Leung, P., & Zalenski, H. M. (1997). Technical, allocative and economic efficiencies in swine production in Hawaii: A comparison of parametric and nonparametric approaches. Agricultural Economics, 20, 23–35.
- Silva, T. C., Tabak, B. M., Cajueiro, D. O., & Dias, M. V. B. (2017). A comparison of DEA and SFA using micro- and macro-level perspectives: Efficiency of Chinese local banks. Physica A: Statistical Mechanics and its Applications, 469, 216–223. doi:10.1016/j.physa.2016.11.041
- Steel, F. W., & Andah, O. D. (2003). Review of rural and microfinance regulation in Ghana: Implications for development and performance of the industry ( Africa Region Working Paper Series No. 49). Washington, DC: World Bank.
- Sufian, F. (2009). Determinants of bank efficiency during unstable macroeconomic environment: Empirical evidence from Malaysia. Research in International Business and Finance, 23, 54–77. doi:10.1016/j.ribaf.2008.07.002
- Sufian, F., & Kamarudin, F. (2014). The impact of ownership structure on bank productivity and efficiency: Evidence from semi-parametric Malmquist productivity index. Cogent Economics & Finance, 2, 932700. doi:10.1080/23322039.2014.932700
- Tahir, I. M., Bakar, N. M. A., & Haron, S. (2008). Technical efficiency of the Malaysian commercial banks: A Stochastic Frontier approach. Banks and Bank Systems, 3, 65–72.
- Wadud, A., & White, B. (2000). Farm household efficiency in Bangladesh: A comparison of stochastic frontier and DEA methods. Applied Economics, 32, 1665–1673. doi:10.1080/000368400421011
- Wijesiri, M., & Meoli, M. (2015). Productivity change of microfinance institutions in Kenya: A bootstrap Malmquist approach. Journal of Retailing and Consumer Services, 25, 115–121. doi:10.1016/j.jretconser.2015.04.004
- Zenebe Lema, T. (2017). Determinants of bank technical efficiency: Evidence from commercial banks in Ethiopia. Cogent Business & Management, 4, 1268356. doi:10.1080/23311975.2016.1268356
- Zhou, Z., Placca, E., Jin, Q., Liu, W., & Wu, S. (2017). Banks efficiency and productivity in Togo after the financial liberalization: A combined Malmquist index approach. INFOR: Information Systems and Operational Research, 1–15. doi:10.1080/03155986.2017.1337416