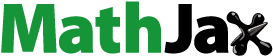
Abstract
This paper states that market sentiments are central to any financial data analysis. A vivid distinction is made between studying financial data in terms of the concept of volatility and in rapport to analysing financial data in terms of market sentiments. The former is an existing approach that is extensively used and the latter is a proposed tactic. Methods of devising constructs for defining relative and absolute market sentiments are also discussed. Patterns of market sentiments in terms of the model parameters are discussed and a few new measures that capture the hypothesized market sentiments are proposed. As an application of the proposed line of approach, this study analyses weekly market sentiments that govern Domestic Company Indices of Botswana Stock Exchange.
Public Interest Statement
Analysis of market sentiments is central to any financial data analysis. Rao and Moseki (Citation2009) advocated market sentiments approach to analyse financial data as opposed to the time series based GARCH model approach which has been well researched over the past several decades. In their work, a clear distinction was made between the existing approach of studying financial data in terms of extensively used concept of volatility and the proposed approach in terms of market sentiments. In this paper, we further explore the types of market sentiments and device constructs for defining relative and absolute market sentiments. A few possible patterns of market sentiments in terms of the model parameters are discussed and new measures which capture the hypothesized market sentiments are proposed. As an application of the proposed line of approach, we analyse weekly absolute market sentiments that govern Domestic Company Indices of Botswana Stock Exchange.
1. Introduction
The natural up and down patterns of the daily stock prices is indicative of the stochastic nature of the financial markets. Despite such price movements investors risk their funds in the stocks with the expectation of making more future returns. The variation in the stock returns due to daily price changes is generally termed as volatility that is measured by the standard deviation of the returns. Investors in stock market are interested in the volatility of stock prices as high volatility could be interpreted as huge losses or profits at the expense of greater uncertainty (Gujarati, Citation2003). Volatility is something good but that can only be bad if the stock price swings are unusually very high and rapid over short time periods as it makes financial planning difficult (Islam, Citation2013). This means that high fluctuations in stock prices can possibly increase both risk and uncertainty about the future returns. Since prediction of stock is part and parcel of stock trading, if the market performance is unstable then investors will be clueless as what the future holds for them and hence increased uncertainty about future price changes. As a result of uncertainty investors can fear to take risk and continue to fund their investments.
A volatile market inhibits individual retailers and companies to raise funds in the capital markets. Islam (Citation2013) cited that uncertainty causes loss of investor confidence that is essential in trading of stocks particularly in encouraging further investment and leverage decision. He further mentions that uncertainty can aggravate volatility. The knowledge of volatility is of crucial importance in many areas of finance in enabling investors to manage risks and optimize profits (Hull, Citation2003). The fluctuations in the asset prices are widely believed to be the cause of changes in the economic and geo-political environmental factors such as stocks, bonds, exchange rates, tradable options and banking instruments among others. The perceived knowledge of the aforementioned contributing factors along with analysis of data gives a player in any stock market a strong and reliable tool to trade.
The financial data analysis is focused on the prediction of the future prices of security and as such they are model-based strategies. These are mainly tailored for the needs of well-informed market players that include individual investors, financial institutions and mutual fund managers. It is widely believed that, security prices exhibit considerable variations from time to time and these variations can be objectively captured by well-defined market volatility phenomena. The concept of volatility is imperative in the analysis of financial time series data. Volatility is the unpredictable change of a variable over time. In the context of finance and economics, volatility refers to the unstable states of economic and financial variables, these variables include stock prices, exchange rates, inflation rates, volume of trade and currency returns. There are several definitions of volatility and the common denominator is variability. The different definitions of volatility are distinct in how they discuss the measurement of this variability. The causes of volatility could be ascribed to several factors; such as local and global economic environments, maturity and delivery date of instruments, changes in trade volume, practices or patterns and new information among others (Hull, Citation2012).
1.1. Objectives and implications of the study
A common assumption in applying GARCH-type models to financial data is that the conditional return series is conditionally normally distributed. In practice, however, the shape of the daily distribution of return series is much broader than the commonly assumed Gaussian distribution. For example, truncated Levy distribution, Student’s distribution, and log normal distribution among others have been used. It is pertinent to indicate that in this paper, we do not assume any specific form of the probability distribution for stock return series. Thus, we adopt a nonparametric approach to pursue the objectives of our study.
The central contribution of this paper is that it suggests an alternative approach for modelling and related analysis of stock returns. In the new approach suggested here, volatility in weekly means of stock returns is conceived to be a function of the mean and its standard error and the perceived volatility is classified into various market sentiment states. Thus, a fairly accurate knowledge of such states of volatility in stock returns will be of great help to a prospective or an existing market player. The main objectives of the paper are as follows:
(1) | To appreciate the distinction between absolute and relative market sentiments that govern stock return data, | ||||
(2) | To devise suitable data-driven rules to classify volatility in stock returns into market sentiment states, | ||||
(3) | To build a probability model for capturing volatility in stock returns in terms of different market sentiment states, | ||||
(4) | To formulate possible hypotheses about the states of market sentiments, | ||||
(5) | To propose different measures to capture the underlying patterns those exist in states of the market sentiments and also to test their significance. |
The implications of the current work are many folds. A few among these are stated below:
(1) | The results in this paper indicate an alternative approach to analyse financial data, namely the market sentiment approach, as opposed to the volatility approach that is in vogue, | ||||
(2) | It is established that the short term movements in a financial data can be analysed in terms of relative market sentiments, while the analysis of absolute market sentiments provide indicators of long-term movements in financial return series, | ||||
(3) | Any decision-making that an investor takes on his investment portfolio is heavily dependent on the direction of the market sentiments. Basically, financial decisions are generally based upon the trade-off between risk and return, it is therefore imperative for investors to make proper decisions in order to avoid too much loss of business. In this paper, we are presenting measures that will assist in decision-making and future planning of investment portfolio, | ||||
(4) | Due to the novelty of the proposed approach, it is hoped that the line of analysis suggested here will be of interest to both academicians and stock market players. |
The paper is structured as follows: After the introduction, second section briefly discusses literature review. The third section outlines methodological framework. In the fourth section, as an application of the proposed model, we analyse Botswana Stock Exchange data from 1999 to 2013, and presents the results and discussions. The probability models are based on the weekly indices. The last section provides conclusions and recommendations, followed by a list of references used in this study.
2. Literature review
The literature on economic and financial variable data analysis is indeed quite extensive. Forecasts of the distribution of such variables like asset returns are of great importance in the context of financial risk management. In pricing options, anticipated volatility over the life of the option is the crucial unknown parameter. One of the most popular tools used to explain volatility clustering in option pricing is the Autoregressive Conditional Heteroscedasticity (ARCH) class of models which was invented by Engle (Citation1982). The theoretical frame work of modelling volatility advocated by Engle captures the time variability of the variance of returns by imposing an autoregressive structure on the conditional second moment of returns. Over the years there have been a number of extensions to the basic ARCH model. The most important of these is the generalised ARCH (or GARCH) model developed by Bollerslev (Citation1987) which includes lagged values of the conditional variance as extra regressors. Subsequently, many other ARCH-type processes have been developed to capture various dynamics. For additional references and expository studies using ARCH type of models and also for exhaustive theoretical treatment of volatility models, we refer to Blake (Citation2005) and Sheldon (Citation1994).
Generally, market sentiments are perceived to be either absolute or relative. Absolute market sentiments can be defined with reference to a base period greater than a month such as a quarter or bi-annual or annual. In this case, market sentiments are constructed based on the entirety of data corresponding to the base period. For example, the weekly market sentiments can be constructed based on the daily stock prices of a given year. It may be pointed out that absolute market sentiments are useful to compare the movements in market sentiments over blocks of years and hence may be of use to players with long-term interest. In other words, absolute market sentiment captures the long-term impact on volatility. Relative market sentiments are defined with reference to shorter base period such a week or month. In this case, market sentiments are more dynamic in the sense that constructs compare weekly or monthly data from time to time. Relative market sentiments are seen to be useful to an active player with short-term interest. A large share of overall movement of an individual stock has been attributed to market sentiments. Forecasting market sentiments in financial data such as stock indices, volume of trade is a formidable area of research in financial economics. There are innumerable causes, as mentioned earlier, that propel market sentiments in a financial data. Classically, market sentiment is monitored by a variety of technical and statistical methods such as the number of advancing vs. declining stocks and new highs vs. new lows comparisons.
There are multiple of studies dealing with stock return volatility from an investor’s perceptive. In most of these studies, researchers preferred to use the terminology investor sentiment rather than the “market sentiment”. For the sake of completeness, we outline a few of these, keeping in mind that none of these studies follow the approach that is advocated in this paper.
Within the scope of behavioural finance, many studies have gone on to analyse factors relating to the mentality of investors, chief among others being volatility. A comprehensive review of the prior studies based on the role of market sentiments in the financial markets was investigated by Shiller (Citation1999) who examined the behaviour of investors in the financial markets; Hirshleifer and Shumway (Citation2003) demonstrated, from the psychological point of view, that since investors have acute shortage of information, investor sentiment is a factor for consideration in the formulation of any investment strategy. In other words, when investors lack complete information regarding their investment, they are believed to make their choices based on unreasonable behaviour and personal considerations.
Lee, Jiang, and Indro (Citation2002) investigated the relationship between volatility, stock returns and investor sentiment. They estimated a GARCH-in-mean model which includes contemporaneous shifts in investor sentiment in the mean equation and lagged shifts in investor sentiment in the conditional volatility variance equation. They used the survey indicators provided by investors’ intelligence to examine the impact of changes in investor sentiment on the conditional volatilities and indices. They found that bullish (bearish) changes in investor sentiment result in downward (upward) adjustments in volatility.
Wang, Keswani, and Taylor (Citation2006) and Lee and Kumar (Citation2006) showed that the index of investor sentiment has no predictive power in the stock market return, or at best very limited ability. This has not diluted the appeal by the majority of studies. Within the prior studies by Brown (Citation1999), Fisher and Statman (Citation2000), Lee et al. (Citation2002), Baker and Wurgler (Citation2004), Baker and Stein (Citation2004), Brown and Cliff (Citation2004) and Lee and Kumar (Citation2006) tend to agree that there is correlation between the investor sentiment index and stock market returns, and this could be used to predict the index of return within the stock market. Yoshinaga and de Castro (Citation2012) estimated a pricing model that relates stock rate of return to market sentiment index and other factors and the results confirm that the sentiment variable plays a relevant role.
Rao and Moseki (Citation2009) analysed the data on Domestic Company Indices of Botswana Stock Exchange using a probabilistic approach to market sentiments. The methodology developed in that paper is applied to weekly and monthly DCI of the Botswana Stock Exchange. The findings in that study are; the weekly and monthly DCI of the BSE are volatile; the market environment in BSE is investor-friendly as the bubble sentiments are the modal sentiments; the variation in monthly DCI is more stable than the weekly DCI; the weekly trading is more speculative than the monthly trading. Finally, from the seller’s point of view, returns from investment are more if the decision to sell scrip is based on weekly volatility analysis rather than monthly volatility analysis. Rao and Moseki (Citation2011) provided an alternative approach, namely a Markovian approach to the study of market sentiments. An application of their methodology to the weekly DCI revealed that “greedy” market sentiment prevailed during the study period as the long-term probability related to the “greedy” sentiment was high and close to 0.5. Rao and Ramachandran (Citation2014) used the methodologies advocated by Rao and Moseki (Citation2009) to analyse exchange rate market sentiments of six major global currencies against the Botswana Pula. In this research, the authors considered relative market sentiments. The study has generated the following results; weekly and monthly exchange rate market sentiments appear to fall into two regimes of symmetric and asymmetric sentiments; all the six currencies exhibited smiling sentiments giving the scenario of asymmetric market sentiments; all the currencies have clusters of sentiment states which are strongly persistent revealing that the exchange rate trading across the currencies and the years are buoyant. In conclusion, the literatures reviewed do not capture the direction of the market sentiments which is a key variable of interest to the traders in the market whenever they want to make investment decisions. An attempt is also made to fill this gap by devising suitable measures.
3. Methodology
3.1. Market sentiment constructs
Because this paper focuses on financial applications, we will use financial notation. Let “t” refer to the trading day and “N” the total number of trading days in a study period. Further, let “w” denote the week, so that W corresponds to the total number of weeks in a study period. Let the number of trading days in a week be denoted by nw. In a typical stock market, nw could vary between 1 and 5. Let for a week w = 1, …, W, denote the equity index of day “t”, t = 1, …, nw, w = 1, …, W. Our analysis will be based on the log returns of stock index (DCI) defined by
For analysing weekly DCI returns, for a week w = 1, …, W, we define the average returns by(1)
(1)
and the sample variance of equity return index for the week w by(2)
(2)
Similarly, for the entire period N, we have the combined mean and the combined sample variance of the equity returns, respectively, given by, (3)
(3)
(4)
(4)
If we assume that rt’s come from a population with finite mean μ and finite variance σ2, then we know that ,
=
and let Z =
. We shall use the statistic Z to define classification rules for the market sentiments.
As in Rao and Moseki (Citation2009), Rao and Ramachandran (Citation2014), we consider the following seven type of market sentiments.
The market sentiments, from the standpoint of a seller in a stock market can be seen to belong to the following categories.
The depressed/fear sentiment. This is a state in which the equity index witnesses a sharp fall, enough to cause panic to a seller holding stocks. This may lead to distress selling and shatter investor confidence. This sentiment may also witness eventual bankruptcy or consolidation or regulatory intervention. Further, raising funds from the capital market may be extremely difficult.
The bearish sentiment. This is a state in which the equity index witnesses a significant downward trend, enough to cause frustration to a seller holding stocks. This may lead to an offloading exercise of stocks and undermine investor confidence. Moreover, raising funds from the capital market may be adversely affected.
The downward sentiment. This is a state in which the equity index witnesses a gradual fall enough to sound a warning to an investor. This may lead to an offloading exercise of stocks and may dent investor confidence. Sustained attempts may be needed to raise funds from the capital market.
The tranquil sentiment. This is a state in which the equity index witnesses normal swings. The investor continues to be an active player in the stock market. This state may also be called “range bound” or “uncertain”.
The upward sentiment. This is a state in which the equity index witnesses a gradual upward swing enough to cause a feeling of encouragement to an investor. The environment for fund raising may be encouraging.
The bullish sentiment. This is a state in which the equity index witnesses a significant upward swing; propelling speculation by a seller holding stocks. Further, raising funds from the capital market may be easy.
The greed/bubble sentiment. This is a state in which the equity index witnesses a sharp rise, enough to prompt a seller either to dispose of his load or to hold on in the hope of further appreciation leading to a bubble like condition. This state assures the seller of massive gains for having waited a while. In addition to this, raising funds from the capital market may be spontaneous.
In order to reflect these seven market sentiments, we associate the following classification rules, based on the statistic Z, with the states Sj, j = 1, 2, 3, 4, 5, 6, 7. Let and
be consistent estimators of μ and σ2, respectively.
Then for a week (w + 1), , the equity index is said to belong to
(5)
(5)
The value of the multiplier of between −3 and 3 are so chosen as the cut off points of the standard normal distribution that in essence reflects a symmetric distribution. These values reflect normal to extreme shift on either side of the middle. So, the whole idea is to try to create symmetric market sentiments of the financial stock market data. The notion of symmetric distribution of asset returns to most investors is an ideal environment in the sense that changes in the security return is the same from either side of the middle. So, multipliers used are equivalent to the action and warning limits of the statistical process control (See, for example, Grant & Leavenworth, Citation1980).
In the approach that we are proposing, we use these values to generate categories of volatility of asset returns we referred as market sentiments. The seven-states market sentiments constructs is general, in a sense that they are capable of reflecting most of the market sentiments usually observed in a vibrant stock market economy. In a less vibrant stock market economy, one may continue to work with smaller set of states. But if the market sentiments appear to be uniform, say, four categories, one may be inspired to use the states defined on the basis of quartiles, and the multipliers of the standard error may correspond to the quartiles of the standard normal distribution. These multipliers will be used to construct four market sentiment states.
Suppose we take , the weekly average of log returns of equity prices as an estimator of μ, and
, the weekly sample variance of log returns as an estimator of σ2. Then, the classification rule (5) with these estimators will define relative market sentiments as considered in Rao and Moseki (Citation2009). On the other hand, if we use the combined estimators
,
, and replacing nw = W, in (5), the classification rules generate absolute market sentiments.
3.2. Modelling market sentiments
Following the assumptions and the notations of Rao and Moseki (Citation2009), in general, the vector of counts of market sentiment states follows a multinomial distribution with the joint probability mass function (p.m.f.) given by
(6)
(6)
for all and
; where, fj denotes the number of occurrences of the state Sj in an independent sequence of the phenomena observed for W weeks and
, j = 1, 2, ..., k. In general, the parameters θj’s in the model given by (6) are unknown and can be estimated by their empirical estimates
(7)
(7)
3.3. Hypotheses about market sentiments
The market sentiments witness different types of patterns with respect to time, namely bullish pattern (increasing); bearish pattern (decreasing); symmetric pattern criss-crossing (evenly around a calm sentiment). While a bullish or a bearish run may be imperative consequence to an economic or geo-political vital event and therefore may be of short duration, a symmetric pattern is perceived to be the rule of vibrant market economies. These patterns can be effectively summarized in the terms of the model parameters as follows:(8)
(8)
(9)
(9)
(10)
(10)
The pattern in (8) is indicative of an ordered increasing sentiment (Bull Run), while (9) refers to that of an ordered decreasing sentiment (Bear Run). The pattern in (10) reflects a symmetric pattern.
As an illustration for the sentiment pattern H1, an apriori knowledge about a bull run may be characterized by . On the other hand, a bear run exhibited by HD will have
.
When θi’s are unknown and follow the sentiment pattern HS given by (10) an appropriate symmetric multinomial distribution model with k = 7, using the classification rule (5) is given by,(11)
(11)
for all and θ4 = 1 − 2(θ1 + θ2 + θ3).
3.4. Measures of market sentiments
The seven-states symmetric multinomial distribution model was used by Rao and Moseki (Citation2009) and Rao and Ramachandran (Citation2014) to estimate measures of market sentiments and also to test hypothesis HS, namely the symmetry of market sentiments of equity indices. Their approach was intuitively based on the classical chi-square measure of discrepancy between the observed and the expected counts and likelihood ratio principle
. The two measures considered by Rao and Moseki (Citation2009), were given by
(12)
(12)
(13)
(13)
It can be easily shown that these discrepancy measures are related to certain members in the family of power divergence statistics proposed by Cressie and Read (Citation1984).
These authors carried out test for symmetry of the market sentiment states for the asset returns of Domestic Company Indices in Botswana and exchange rate of market sentiments of Botswana Pula against six global currencies, respectively. For Rao and Moseki (Citation2009), the test concluded that the weekly and monthly equity index data were highly asymmetric, while for Rao and Ramachandran (Citation2014), it was revealed that two regime paths emerged, one for symmetry and the other for asymmetry.
Here below, we advance a few new measures of market sentiments that can be used for the types of market sentiments hypothesised in (8)–(10) above.
The measures given by (12) and (13) have the inherent disadvantage that they do not indicate the direction of market sentiments. Firstly, for the pattern HS given by (10), a variant of the measure (12) can be defined as follows:(14)
(14)
where is the sign function defined by
, if u > 0,
, if u = 0,
, else. This measure of market sentiments takes into accounts both the magnitude and direction of market sentiments, therefore especially appropriate when the market sentiments are asymmetric. For example, a large positive
would indicate smiling sentiment states, while a large negative
would indicate frowning sentiment. We observe that
is a measure based on the signed dispersion.
3.4.1. A measure of ideal market sentiments
A second measure of market sentiment is surmised on the theory that in an ideal market condition, more often, the sentiments should be concentrated around the calm sentiment state, namely the state S4, when k = 7. An ideal market sentiment results in least speculations and minimal loss or gain to the player. As a measure of ideal market sentiments, the study proposes a measure which is akin to the mean sum of squares of deviations of the sentiment states taken from the calm sentiment state. Let W denote the number of weeks in a year and tw, w = 1, ..., W, be the value of the sentiment state corresponding to a week w. Note that tw will take one of the values from 1 to 7. For example, if t1 = 2; then for the first week, the sentiment state is “2”, if t2 = 6; then for the second week, the sentiment state is “6” and so on. A dispersion measure of an ideal market sentiment is given by;(15)
(15)
It is evident that in an ideal market condition, tw = 4 for all w and therefore . On the other hand, if the sentiments swing on either side of the normal threshold state S4,
will be significantly different from 0. In the two extreme of cases;
. Thus, greater the values of
, more dispersed are the weekly sentiments from the calm sentiment state S4. In other words,
defines risky sentiments. In our context large value of
closer to nine would mean risky market sentiments in the sense that there are appreciable swings in the market sentiment states. It is pertinent to note that the measure
is an absolute measure of swings, but it fails to measure symmetry of market sentiments like the measures
and
3.4.2. A measure of uncertainty of return series
Finally, uncertainties associated with market sentiment models given by (6) and (11) can also discussed in terms of information theoretic entropy. For example, we may use Shannon’s entropy, as in Shannon (Citation1948), for the model (6), which is given by;(16)
(16)
The choice of a logarithmic base corresponds to the choice of a unit for measuring information. Shannon himself discussed logarithm bases 2, 10 and e, but in practice entropy is always calculated using base 2 logarithm. Henceforth, this paper uses base 2 in computations. When θi’s are unknown, we compute , using the empirical estimates of θi’s given by (7). On the other hand, when θi’s are perceived to be known apriori, we may use the formula given by (16) directly. The most rational choice of θi’s is θi = k−1, i = 1, ..., k, in which case the maximum entropy will be equal to
. Thus, for k = 7,
for an ideal band of market sentiments. If one uses the classification rule (5) stated in Section 3.1, using the empirical rule of large sample approximation, apriori, we have, θ1 = 0.00135 = θ7, θ2 = 0.0215 = θ6, θ3 = 0.1359 = θ5, θ4 = 0.6826. In this case,
= 1.423. This paper observes that the classification rule with these apriori probabilities provides the most extreme band of market sentiments. Therefore, it may be argued that model uncertainties as defined by Shannon’s entropy will vary in between the entropy measure corresponding to the extreme (HI or HD) pattern and the symmetric (HS) band of market sentiments. Thus, under this assumption we have;
and this interval may be used to ascertain the significance of any computed entropy for the observed market sentiment counts for the given year. Similarly, with the priori choice of model parameters given by
Using (16), it can be shown that the entropy value for the market sentiment scenario given by (8) and (9) is given by = 2.61.
3.4.3. Divergence measures
Alternatively, one may use the generalized directed divergence measure proposed by Cressie and Read (Citation1984) or Bregman (Citation1967) divergence. For the most rational choice of θi’s, namely θi = k−1, i = 1, ..., k, it can be shown that a certain member of Cressie–Read divergence measure is given by(17)
(17)
Further, it can be shown that the Bregman divergence based on the squared Euclidean distance is given by(18)
(18)
It is easy to show that when the observed frequency of market sentiment states are in perfect synchrony with the rational choice of θi’s.
3.5. Data and basic statistics
The daily stock price index data used in this paper are the daily Domestic Company Indices (DCI) collected from the Botswana Stock Exchange over the period from 11 January 1999 to 31 December 2013. The daily index returns are expressed in the continuously compounded returns calculated as rt = log (pt) − log (pt−1), where pt and pt−1 are the index prices on day t and t − 1 respectively. The market sentiments are calculated for the weeks in the calendar years 1999–2013, assuming that each starting and ending week in a calendar year has at least two trading days. The movement in DCI is classified into the seven-states S1, S2, ..., S7 based on the classification rule described by (5). The data can be downloaded from https://www.bse.co.bw or obtained from the first author. The details of calculations of weekly averages, standard deviations and states to which each weekly DCI belongs to are not reported in this paper but may be obtained from the first author on request. Before proceeding further for formal analysis of market sentiment states, we plot the weekly return series and their volatility over the study period as shown in Figure in order to understand the likely nature of the return series.
It can be observed that the graphs displayed Figure exhibit considerable swings or volatility in the return series over the sample period. The spikes in the return plots are the graphical evidence implying that the volatility is time varying conditional variance. It also indicates the presence of volatility clustering effect in the return series of DCI, that is to say, the series exhibit some periods of high volatility followed by other periods of high volatility and some periods of low volatility followed by other periods of relatively low volatility. Volatility clustering is a sign of positive autocorrelation in the squared returns.
The results from the descriptive statistics reported in Table show that during the period of study, the Domestic Company Indices (DCI) of Botswana stock market observed the weekly mean return of 0.00027. The corresponding volatility of the DCI measured by standard deviation is 0.00122. The high volatility offers the possibility of high rate of returns. The return series for DCI shows positive skewness suggesting that the distribution has a long right tail meaning there are some extreme return values in the right tail of the series. The excess value greater than 3 for kurtosis indicates fat tail characteristics of the security returns distribution. The Jarque–Bera (JB) test of normality statistically and significantly rejects the null hypothesis of normality as indicated by the smallest significance level that is below 0.01. The test suggests that the distribution of the return series is non-normal.
Table 1. Summary statistics
3.5.1. Data stationarity test (unit root test)
In order to assess whether the financial time series (returns) are stationary or not the researchers have applied the augmented Dickey–Fuller (ADF) test. The results show that the ADF test statistics rejected the null hypothesis of the existence of the unit root in the return series as indicated by the highly significant value (<0.0001) of the associated probability of the ADF test statistic. The test summarizes that the return series of DCI is stationary and therefore the application of the stochastic models to return series is appropriate to examine the dynamic behaviour of volatility over time.
4. Results and discussion
Table presents the summary of absolute market sentiments of DCI of BSE for the year 1999–2013. The multinomial model is highly skewed towards the two extreme sentiments (frowning and smiling sentiments). This is an illustration of a discrete bathtub type of a multinomial model. The practical significance of this finding is that the stock market in Botswana is characterized by extreme bulls and bears that present difficulties for potential investors willing to do business as a trader.
Table 2. Weekly absolute market sentiments
Table presents the five measures of weekly absolute market sentiments. It is to be noted that missing values indicate that the corresponding measure is not defined due to zero counts. Based on the measure, , that takes values between 0 and infinity, the empirical observation reveals that the weekly stock return market sentiments more or less appear to fall in two regimes of symmetric and asymmetric sentiments. The findings point to 1999 and 2004 through 2006 as asymmetric, otherwise the rest of the years seem to be symmetric. The summary findings on
whose values range between
, where intuitively small values would indicate symmetry of the market sentiments, indicates that the DCI market experienced periods of bear performance and periods of bull performance for study time periods. The measure reveals that for the study period considered the market movement was more towards the bearish sentiments than the bullish one. Considering the measure
, there is evidence that stock market is branded by symmetric market sentiments for values of measure close to zero and are very small except for years where the measure could not be computed due to zero counts of sentiment states. In other words, lack of vibrancy in the DCI trading resulted in a number of zero counts that caused the measure untenable for some of the years. The other measure that was considered is the
that measures the dispersion of the market sentiments from the calm sentiment, that communicates market swings of sentiment states. The larger the values of the measure close to nine, the riskier it is to invest in equity market. There is empirical evidence that across all the study period there has been no considerable swings experienced in the stock market. On using the measure
, that detects uncertainty or the presence of either increasing or decreasing patterns of the market sentiments over the years considered for the study. From the analysis we may conclude that market sentiments during the years 1999–2013 do not reflect the pattern HI or HD, since the computed
in column (6) of Table are significantly different from the reference value
= 2.61. However, since the computed values are somewhat nearer to the reference value
= 1.423, we may conclude that the market witnessed more or less symmetric pattern HS during the entire study period. The measure
is computable only for some years and not for others because of zero counts of market sentiment states in those years. Using the measure
, it is clearly seen that the market sentiment states are close to the rational choice of equi-symmetry of θi’s for all the years. Turning to the measure
, the market sentiment states are close to the rational choice of equi-symmetry during the entire period of study.
Table 3. Measures of market sentiments
5. Conclusions and recommendations
The study of this paper proposes three new measures to analyse absolute market sentiments and to detect the direction of the market sentiments. Evaluating the stock market returns sentiments helps the trader to develop a well-informed and strong trading strategy. In order to evaluate stock returns perception of volatility is critical. Volatility is observed in the daily/weekly/monthly stock returns. The concept of volatility is not easily understood by a trader. To circumvent this, we advocate the analysis of stock returns based on the concept of market sentiments that is very user-friendly and easy to understand by a trader. The focus of this study has been to analyse weekly stock returns applied to market sentiment measures to have a better understanding of the movement in stock returns in terms of market sentiments.
The DCI data analysis shows that the market sentiments are indeed asymmetric and skewed towards the two extreme sentiments of bears and bulls. This is critical for investors who want to minimize risk and maximize profit from equity markets. The investors would therefore know when to trade and when not because they are now equipped with strategies to avoid major losses. Knowing the direction of the market helps one to make prudent resource allocation to avoid dependency on one investment.
The summary findings of the analysis show a continued existence of symmetric market sentiment behaviour witnessed in almost all blocks of years except for a few cases. This proposes that any trader investing in stocks within those years is likely to run a chance of either gaining or losing by the same quantity. Therefore, in case of departure from symmetry a signed measure is applied to market sentiments to determine the direction of the market that could either be bearish or bullish. The analysis shows that for the block of years where the market sentiments witnessed asymmetry towards frowning state of sentiment possibly indicates that the market seems to be under performing.
Our additional findings indicate that investing in the BSE is not yet risky. This declaration of less riskiness has been affirmed by the application of other two measures of equi-symmetry of market sentiments that pronounced symmetric market sentiments behaviour.
In recommendation, the investors in equity markets must be assisted with knowledge about market sentiments states of equity indices, for extreme sentiment states could mean huge losses or gains, resulting in greater uncertainty and thereby impacting on the much needed development of emerging economies like Botswana. We suggest additional listing of domestic companies on BSE as a way of promoting liquidity in the trading of stocks to allow for provision of a friendly environment conducive from those who want to profit from stock trading.
Additional information
Funding
Notes on contributors
K.K. Moseki
K.K. Moseki is a lecturer in the Department of Statistics, University of Botswana. He is pursuing his PhD in Statistics at the same department. He has published research papers in regional journals. His research interests include financial data analysis, time series, among others.
K.S. Madhava Rao
K.S. Madhava Rao is a professor in the Department of Statistics, University of Botswana. He has published extensively in national, regional and international peer-reviewed journals with high-impact factors. His research areas include nonparametric inference, empirical likelihood, social sciences, agricultural and financial data analysis.
References
- Baker, M. P., & Stein, J. C. (2004). Market liquidity as a sentiment indicator. Journal of Financial Markets, 7, 271–299.10.1016/j.finmar.2003.11.005
- Baker, M. P., & Wurgler, J. (2004). Investor sentiment and the cross-section of stock returns (NBER Working paper No. 10449). Cambridge, MA: National Bureau of Economic Research.10.3386/w10449
- Blake, D. (2005). Financial market analysis (2nd ed.). Chichester: Wiley & Sons Ltd.
- Bollerslev, A. T. (1987). A conditional heteroscedastic time series model for speculative prices and rates of return. The Review of Economics and Statistics, 69, 542–547.10.2307/1925546
- Bregman, L. M. (1967). The relaxation method of finding the common points of convex sets and its application to the solution problems in convex programming. USSR Computational Mathematics and Mathematical Physics, 7, 200–217.
- Brown, G. W. (1999). Volatility, sentiment and noise traders. Financial Analysts Journal, 55, 82–90.10.2469/faj.v55.n2.2263
- Brown, G. W., & Cliff, M. T. (2004). Investor sentiment and the near-term stock market. Journal of Empirical Finance, 11, 1–27.10.1016/j.jempfin.2002.12.001
- Cressie, N., & Read, T. C. (1984). Multinomial goodness-of-fit tests. Journal of the Royal Statistical Society, 46, 440–464.
- Engle, F. R. (1982). Autoregressive conditional heteroscedasticity with estimates of the variance of United Kingdom inflation. Econometrica, 987–1008.10.2307/1912773
- Fisher, K. L., & Statman, M. (2000). Investor sentiment and stock returns. Financial Analysts Journal, 56, 16–23.10.2469/faj.v56.n2.2340
- Grant, E. L., & Leavenworth, R. S. (1980). Statistical quality control. New York, NY: McGraw Hill.
- Gujarati, D. N. (2003). Basic econometrics. New York, NY: McGraw Hill.
- Hirshleifer, D., & Shumway, T. (2003). Good day sunshine: Stock returns and the weather. The Journal of Finance, 58, 1009–1032.10.1111/1540-6261.00556
- Hull, C. J. (2003). Options, futures and other derivatives (5th ed.). Englewood Cliffs: Prentice Hall.
- Hull, J. C. (2012). Options, future and other derivatives (8th ed.). Englewood Cliffs: Pearson Education.
- Islam, M. A. (2013). Estimating volatility of stock index returns by using symmetric GARCH models. Middle-East Journal of Scientific Research, 18, 991–999.
- Lee, C. M. C., & Kumar, A. (2006). Retail investor sentiment and return co-movements. Journal of Finance, 56, 2451–2486.
- Lee, W. Y., Jiang, C. X., & Indro, D. (2002). Stock market volatility, excess returns, and the role of investor sentiment. Journal of Banking and Finance, 26, 2277–2299.10.1016/S0378-4266(01)00202-3
- Rao, M. S., & Moseki, K. K. (2009). A probalistic approach to categorise equity index volatility. Management Dyanamics, 18, 22–34.
- Rao, M. S., & Moseki, K. K. (2011). Analysing volatility in equity indices—A Markov approach for botswana domestic company indices. South African Journal of Industrial Engineering, 22, 83–98.
- Rao, K. S., & Ramachandran, A. (2014). Exchange rate market sentiment analysis of major global currencies. Open Journal of Statistics, 4, 49–69.10.4236/ojs.2014.41006
- Shannon, C. E. (1948). A mathematical theory of communication. Bell System Technical Journal, 27, 623–656.10.1002/bltj.1948.27.issue-4
- Sheldon, N. (1994). Option volatility & pricing: Adavanced trading strategies and techniques. New York, NY: McGraw Hill Professional.
- Shiller, R. J. (1999). Human behavior and the efficiency of the financial system. In J. Taylor & M. Woodford (Eds.), Handbook of macroeconomics (pp. 1305–1340). Amsterdam: Elsevier B.V.
- Wang, Y. H., Keswani, A., & Taylor, S. (2006). The relationships between sentiment, returns and volatility. International Journal of Forecasting, 22, 109–123.10.1016/j.ijforecast.2005.04.019
- Yoshinaga, C. E., & de Castro, Jr., F. F. (2012). The relationship between market sentiment index and stock rates of return: A panel data analysis. BAR, Rio de Janeiro, 9, 189–210.