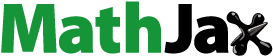
Abstract
This study aims to identify the determinants of adoption of improved maize variety (IMV) among farmers in the northern region of Ghana and subsequently assess the factors influencing the intensity of IMV adoption. The study used two econometric techniques to address its objectives. Firstly, a multinomial logit was employed to identify factors affecting the adoption of IMV. Secondly, Tobit regression was used to analyze the determinants of the intensity of IMV adoption. A fractional regression model through the procedure proposed by Papke and Wooldridge was also used to test the robustness of the results obtained from the Tobit model. Results from the study revealed that variables such as the age of the household head, household size, level of experience, farm workshop attendance, the number of years in formal education, access to agricultural credit, membership of a farmer-based organization, availability of labor and extension contacts influence the adoption of IMV. Moreover, variables such as years in formal education, household size, distance to farm plots, attendance of demonstration fields, membership of a farmer-based organization, farm size, and previous income are significant determinants of the intensity of IMV adoption. The study has implications for achieving food security and poverty reduction through agricultural productivity growth.
Public Interest Statement
Since the emergence of the Green Revolution, investments in agriculture has resulted in the development and dissemination of many improved crop varieties (ICV) for cultivation by farmers worldwide. The development of these ICVs is geared toward increasing farm productivity and income, reducing hunger and malnutrition, and minimizing food insecurity, particularly among developing economies like Ghana. However, research has shown that in smallholder farming communities in developing countries, adoption of ICV including improved maize varieties (IMV) is relatively low. The study, investigates the determinants of adoption and adoption intensity of IMV in the northern region of Ghana. Empirical findings from the study confirm that farmers’ socioeconomic characteristics, institutional and policy factors significantly influence adoption and adoption intensity of IMV. The study, therefore, recommends that farm-level strategies oriented toward the adoption of ICV, in general, is very crucial to enhance productivity growth in the Ghanaian agricultural economy.
1. Introduction
As the world’s population is expected to reach 9.1 billion by 2050, the production of food, mainly staple crops is expected to increase accordingly, especially for the 870 million people who are currently food insecure (International Finance Corporation [IFC], Citation2013). This suggests that the dominant role of agriculture as the primary source of food and employment creation in the developing economies should be stepped up. A study by Alexandratos and Bruinsma (Citation2012) indicated that agricultural production needs an increase of 60% by 2050 to meet the world’s consumption demand. This expected growth means that smallholder farmers who are the principal conduit of agricultural production have a significant role to play. In Sub-Saharan Africa (SSA), a majority of the population is agriculture dependent with about 55% in the rural areas (IFC, Citation2013). Agriculture in Ghana is a crucial sector contributing about 22% to GDP and employing more than 50% of the labor force (Food and Agriculture Organization [FAO], Citation2015). The contribution of agriculture to achieving the Millennium Development Goal (MDG) of halving poverty and hunger by the end of 2015 was quite impressive. For instance, between 1992 and 2013, the national poverty levels in Ghana reduced by more than half (56.5 to 24.2%), thus achieving the MDG 1 target (Cooke, Hague & Mckay, Citation2016).Footnote1 However, the sector remains predominantly small-scale with over 82.5% of rural households involved in producing about 80% of the output through rudimentary method leading to low productivity (FAO, Citation2015; Ghana Statistical Service [GSS], Citation2014a). Also, over 25% of the people, particularly in the northern part of the economy, still live under US$1.25 per day (FAO, Citation2015).
The vital sector in the Ghanaian agricultural economy is the crop sector consisting of major crops such as cocoa, maize, rice, yam, cassava, plantain, and other cereals and fruits. Other crops like oil palm, cotton, coconuts, and soybean are also essential cash crops in the agricultural sector. The sector has been fluctuating regarding its contribution to GDP. The contribution of the agricultural sector reduced from 30.1% in 2006 to 20.5% in 2015 even though there was a significant increase in terms of value (about GH¢5.4 million to approximately GH¢26 million) during the same period, which has resulted from a substantial growth in the crop sub-sector (GSS, Citation2016). This suggests that the agricultural economy has not done enough to catch up with the growth of the economy. Among the cereal crops, maize is considered the most critical crop accounting for about 50% of the total cereal production with an estimated 2.1 million households involve in its cultivation (GSS, Citation2014b). Maize has been cultivated in Ghana since late sixteenth century and was formerly a significant food crop in the southern part of the country. Today, maize is an essential Ghana’s staple food produced by the vast majority of the rural households across the five (of the six) agro-ecological zones of Ghana—Coastal savannah, High rainforest zone, Semi-deciduous rainforest, Forest savannah and the Guinea savannah (Amanor-Boadu, Citation2012).Footnote2 The poultry and livestock sectors largely depend on maize for their survival since it forms a substantial component of livestock and poultry feed. Moreover, the crop is used for the preparation of other materials such as corn starch, corn flakes, maltodextrins, corn oil, corn syrup and products of fermentation and distillation industries. Despite the significant contribution of maize to the Ghanaian economy, growth in the sector has come as a result of expansion in the area of land cultivated rather than productivity.
Since the so-called Green revolution, investments in Agricultural research have resulted in the development and the release of many improved crop varieties (ICV) for cultivation by farmers across the globe (Consultative Group on International Agricultural Research [CGIAR], Citation2011). Studies have shown that these ICV have been one of the strategies to increase agricultural productivity and had accounted for about 50–90% of global crop yield increase (Bruins, Citation2009; World Bank, Citation2007). By implication, ICV can increase farmer’s income and reduce rural poverty (Alene, Menkir, Ajala, Badu-Apraku, & Olanrewaju, Citation2009; Krishna & Qaim, Citation2008). However, adoption of ICV including IMV is relatively low within smallholder farming communities in developing countries including Ghana (Smale, Byerlee & Jayne, Citation2011). Walker et al. (Citation2014) indicated that just about 35% of land under cultivation in Africa are allocated to improved crop varieties. In Ghana, the Council for Scientific and Industrial Research (CSIR) through Crop Research Institute of Ghana (CRI) and Savanah Agricultural Research Institute (SARI) have developed and released many improved maize varieties (IMV) for cultivation by farmers across the country including Tolon district. Despite the releases of all the IMVs in Ghana by CSIR, maize yields are still less than half of the economically attainable yields in the country (Ministry of Food and Agriculture [MoFA], Citation2013). For example, national average yields are 1.9 metric tonnes/hectare while data from different on-station and on-farm trials suggested yield averages of 4–6 tonnes/hectare as achievable yields for the crop (MOFA, Citation2013). The low productivity could partly be ascribed to the low adoption of IMV which limit the revenues of farmers and subsequently lead to poverty and food insecurity. Yet, there is a paucity of studies explaining the economic relationship between farm household socioeconomic factors and adoption of IMV. Moreover, adoption is a location and technology-specific study. Hence, research that focuses on such important crop grown in an area where poverty is still pervasive is crucial. Further, to the best of the authors’ knowledge, no empirical research in Ghana had considered the adoption of both the improved and the local variety of maize. The present study aims to provide an understanding of the determinants of IMV adoption and its intensity in the northern region of Ghana using Tolon district as a case study. The rest of the paper is structured as follows: section I provides information on relevant literature relating to the adoption of agricultural technology in general, and ICV in particular, section II delivers the methodological approach to achieving the objectives of the study. Part III discusses the empirical finding while the last part (IV) concludes the study with recommendations for policy implications.
1.2. Literature review
Diverse approaches have been used in literature to model factors influencing adoption decisions of agricultural technologies, of which dichotomous choice models (Logit, Probit, and Tobit) and multiple response models (Multinomial logit or Multivariate probit) are widely used. A dichotomous regression model (Probit or Logit) is usually used when the data in question is qualitative and explains only the probability of adoption or non-adoption (Madala, Citation2005). Contrary to the logit or probit model, multinomial response models (probit or logit) deal with three or more alternative responses under the assumption of Independent Irrelevant Alternative (IIA), i.e. the relative probability of someone choosing between two options is independent of any additional alternatives in the choice set (Train, Citation2003). Tobit model is employed when the data-set for the dependent variable is censored, and there are continuous effects of the explanatory variables on the dependent variable. The Tobit model is usually used to estimate joint effects of factors influencing probability and intensity of adoption (Adesina, Citation1996; Waithaka, Thornton, Shepherd, & Ndiwa, Citation2007).
Considerable literature exists in explaining factors influencing adoption decisions of IMV using different econometric techniques, some of which are mention above. Most previous and recent studies have shown that household characteristics, farm-specific and institutional factors have a significant influence on adoption of farm technology (Denkyirah et al., Citation2016; Nmadu, Sallawu, & Omojeso, Citation2015; Ouma & De Groote, Citation2011). Thomson, Gelson & Elias (Citation2014) using logit model posited that farmers’ age, maize farming experience, and household labor, among others, significantly explain the adoption of improved maize seed varieties in Southern Zambia. Educational attainment also plays vital roles in enhancing production through farm technology adoption by increasing the capacity of farmers to access market information easily. Gebresilassie and Bekele (Citation2015) used Tobit regression model to study the determinants of allocation of farmland to improved wheat variety in Northern Ethiopia. The study found that farmers with higher years of formal education have a higher probability of allocating a significant proportion of their farmlands to an improved variety of wheat seeds. This is because educated households are better skilled and can quickly synthesize production technologies and market information.
Other relevant variables that have been documented by many studies to have significant effects on agrarian technology adoption are on-farm and off-farm income. Diiro (Citation2013) analyzed the impact of off-farm income on agricultural technology adoption intensity and productivity among rural maize farmers in Uganda. The empirical results revealed that income from off-farm activities induces adoption of improved maize variety. However, farm households without off-farm income were more productive than households with off-farm income. Similar results on the influence of off-farm activities on farm technology adoption were reported by Mmbando and Baiyegunhi (Citation2016) while previous income from rice farm was found to positively and significantly influence the adoption of improved rice varieties among farm households in rural Nigeria (Awotide, Aziz, & Diagne, Citation2016). Other farm households’ assets and farm-specific characteristics have been reported by many pieces of literature to influence adoption of farm technology including IMV positively. For instance, Afolami, Obayelu, and Vaughan (Citation2015) indicated that household ownership of assets such as radio, television and mobile phones are significant sources of information for new farm technology and hence the likelihood of increasing the level of adoption. A study by Awotide, Abdoulaye, Alene, and Manyong (Citation2014) in South-Western Nigeria found a negative and significant relationship between total farm size and adoption of improved cassava variety. Similar results were reported by Teklewold, Kassie, and Shiferaw (Citation2013) and Kassie, Jaleta, Shiferaw, Mmbando, and Mekuria (Citation2013).
Awotide et al. (Citation2016) also examined factors influencing adoption of improved rice varieties (IRV) in rural Nigeria using Tobit regression model, where the dependent variable (intensity of adoption) was defined as the proportion of farmland allocated to improved rice variety. Their empirical results identified factors such as membership of Farmer-based Organization (FBO), the level of training and distance to the seed input shop that positively and significantly affects the intensity of IVR. Regarding the effects of extension services on agricultural technology, a study by Ugwumba and Okechukwu (Citation2014) and Ojo and Ogunyemi (Citation2014) found a positive and significant influence of extension services on an improved variety of cassava among Nigerian farmers. A similar study suggested that credit constrained conditions of farmers explain both probability and the intensity of adoption of sustainable farming practices (Teklewold et al., Citation2013).
2. Methodology
2.1. The study area and data source
The study analyses the adoption and intensity of adoption of maize variety among maize farm households in Ghana using a sample obtained from the farming communities in the Tolon district. The district is predominantly rural communities with the majority being smallholder farm households. Most of these families are crop farmers with maize dominating their farming systems. The population of the district according to the 2010 population census is estimated at 72,990 representing about 2.9% of the total population in the northern region of Ghana (GSS, Citation2014b). The 2010 housing population census also indicated that the district has about 8,110 households, out of which about 7, 304 (97.5%) are into crop farming (GSS, Citation2014b). The major traditional crops cultivated include maize, rice, millet, cowpea, sorghum, cassava, groundnut, and yam. The district is characterized by a single rainy season, which starts in late April with little rainfall, rising to its peak in July-August and declining sharply and coming to a complete halt in October-November. The dry season starts from November to March with day temperatures ranging between 33 and 39 °C, while mean night temperature varies from 20–26 °C. The Mean annual rainfall ranges between 950–1,200 mm.
The data were obtained mainly from primary sources, through the use of structured questionnaires. The survey covered fifteen communities in the Tolon district. These communities includes; Lungbunga, Gundaa, Kpendua, Kunguri, Wantugu, Kasuliyili, Yoggo and Kapligun. The rest are Yepelgu, Tingoli, Nyankpala, Tunayili, Gburimani, Tali and Nambulegu. A multi-stage random sampling technique was used in selecting the district in the Northern region, communities within the district and households within the communities. In the first stage, Tolon district was randomly selected from the many maize-producing districts in the Northern region. In the second stage, 15 maize farming communities were randomly selected from Tolon district and finally, 10–15 farm households were selected from each community. A total of 200 farm households were used for this study.
2.2. Conceptual framework and estimation technique
Adoption can be defined as the use and continue use of innovation (Rogers, Citation1962). The study employed random utility framework in analyzing adoption and intensity of adoption of improved maize variety (IMV). We assumed that a maize farmer is a rational producer and will, therefore, make a rational production decision. Considering that maize farm households have choices of planting only local variety; only IMV or a combination of the two, farm households aim to make maximum profits from their choices by comparing the benefit provided to them by the various choices available to them. Thus, maize farm households will plant only local variety, only IMV or a combination of the two (IMV and local variety) if doing so will maximize his or her utility. The requirement for an ith maize farmer to choose any package, j, over any alternative package m is that πij > πim m ≠ j.
Following previous and recent studies (Baltas & Doyle, Citation2001; Diiro, Ker, & Sam, Citation2015; Hanemann, Citation1984), the expected benefit that a farmer derives from the adoption of package j is a latent variable determined by observed households and farm-specific characteristics and can be specified as,
where Xi is observed covariates and φij is the error term capturing unobserved characteristics. The study adopted the multinomial logit model to examine farm household decisions on IMV and local variety while Tobit model is used to identify factors influencing the intensity of IMV adoption.
2.3. Multinomial logit model
Considering that maize farm households can decide to use only local variety or only IMV or a combination of the two on their farm plots, a multinomial choice model is appropriate for identifying the factors influencing farm households’ decisions to adopt these choices. In multinomial models, there are K alternatives instead of a binary choice and the level of alternatives are the same. Among the choice models, the study employed the multinomial logit (MNL) model because it is widely used in multiple adoption studies and easy to compute. Another advantage of using a MNL model is its computational simplicity in calculating the choice probabilities that are expressible in analytical form. The main limitation of the model is the independence of irrelevant alternatives (IIA) property, which states that the ratio of the probabilities of choosing any two options is independent of the attributes of any other alternative in the choice set. The MNL model allows farmers’ socioeconomic characteristics to have different effects on the relative probabilities between any two choices.
In MNL, the choice probabilities of K alternative for an ith farm household is computed as;(1)
(1)
The probabilities derived from the Equation (1) have positive values. Since the total probability of P(Yi = j) one and because we can only identify K – 1 probability as independent, normalization process is made. Because the multinomial logit is capable of taking multiple choices, one alternative is different from the other. That is, Yi = 1 and Yi ≠ 1 are not the same. Hence, relative risk ratios are used to explain the alternatives (Borooah, Citation2002; Guris, Metin, & Caglayan, Citation2006; Hardin & Hilbe, Citation2001). Relative risk ratio (or risk ratio) compares the probability of a choice (adoption) in each group and is easier to interpret. Considering an individual choosing among k alternatives in a choice set and let Xi be the covariates explaining the choice of the individual. The generalized logit model focuses on the individual as the unit of analysis and uses individual characteristics as explanatory variables. The explanatory variables, been characteristics of an individual, are constant over the alternatives. The probability that individual j chooses alternative k is[2]
[2]
βi, βk are k vectors of unknown regression parameters (each of which is different, even though Xj is constant across alternatives). Since, the k sets of parameters are not unique. By setting the last set of coefficients to null (that is, βk = 0), the coefficients βk is called the relative risk ratio and it represent the effects of the Xi variables on the probability of choosing the kth alternative over the last alternative. In fitting such a model, you estimate K − 1 sets of regression coefficients.
Empirically, the model can be expressed as;(3)
(3)
where the dependent variable Yi is the adoption of the alternatives (local only, IMV only and both local and IMV). X1……………X10 represent the age of the household head, household size, number of years in formal education, farming experience, availability of seed in the local market, participation in agricultural seminars/workshop, presence of agricultural related NGO in the community, access to credit, availability of labor, and number of extension contact. β’s are parameters to be estimated and ɛiis an error term accounting for unobserved characteristics and measurement errors.
2.4. The Tobit model and the fractional response model
Following Taha (Citation2007) and Awotide et al. (Citation2016), among many others, this study makes use of the Tobit model developed by Tobin (Citation1958) to estimate the intensity of adoption measured as the proportion of the total farmland allocated to the cultivation of IMV. Since the dependent variable is a fraction and ranges from zero (0) to one (1), a double censored regression model was used. The higher the fraction the greater the intensity of adoption. The Tobit model is a hybrid of a discrete and continuous dependent variable describing the relationship between the dependent variable and a vector of explanatory variables. The Tobit model assumes that there is a latent unobservable dependent variable Y* which is a linear function of a set of independent variables Xi and an error term δi. The observed variable Yis equal to the latent variable Y*if and only if the latent variable is greater than zero but less or equal to zero if otherwise. The model is specified as;(4)
(4)
where Y* = βiXi + δi and δN(0, σ2).
Empirically, the Tobit model is presented in Equation (5) below;(5)
(5)
Since the variable of interest (intensity of IMV adoption) is a proportional data, a logit transformation regression model proposed by Papke and Wooldridge (Citation1996) was also estimated to test for the robustness of the results obtained from the Tobit model. Following Papke and Wooldridge (Citation1996) applied in Wale (Citation2010), the study performed the logit transformation on the proportion of maize farmland under IMV (dependent variable) which ranges between zero (0) and one (1). The logit transformation model can be specified as,(6)
(6)
However, estimating the intensity of IMV adoption directly with this approach when the dependent variable contains the extreme values of zero (0) and one (1) produces a spurious results. Therefore, the problem of extreme values were accounted for by substituting the values (0 and 1) with close approximations as applied in Grigoriou, Guillaumont, and Yang (Citation2005), Pryce and Mason (Citation2006) and Wale (Citation2010). The ones (1) and zeros (0) in the data-set were substituted with 0.999999 and 0.0000001, respectively, after which an OLS regression was performed on the transformed dependent variable as specified in Equation (7).(7)
(7)
where represents the intensity of IMV adoption in its transformed form, Xi is a vector of variables hypothesized to explain the variations in the intensity of adoption. These variables include, the gender of the respondent, whether the respondent is the household head or not, age of the respondent, household size, respondent’s number of years in formal education, distance to the farm plots in kilometers, access to credit, respondent’s attendance of agricultural related seminar or conference, membership of farmer-based organization (FBO), farm size measured in hectares and previous income from crop (maize).
2.5. Description of explanatory variables used in the empirical models
Previous studies (Adeoti, Citation2009; Awotide et al., Citation2014; Mendola, Citation2007; Simtowe et al., Citation2011; Sisay, Jema, Degye, & Abdi-Khalil, Citation2015) have reported that farm technology including improved maize variety (IMV) adoption is explained by farmer’s socioeconomic characteristics, and institutional variables such as gender, age, household size, number of years in formal education, amount of labor force, extension services, membership of farmer-based organizations, farmer’s attending demonstration farms as well as crop farm income. In Sub-Saharan Africa, farm resources are usually owned and managed by men, which gives men more access to information than women. The expectation is that household headed by men have a higher likelihood of adoption. Farmer’s age can increase or decrease the probability of adopting an innovation. This is because as a farmer ages, his level of risk averseness increases or decreases depending on his/her confidence level (Kaliba, Verkuijl, & Mwangi, Citation2000). Hence, we expect a positive or negative relationship. A more significant number of adults in a household provides the family labor force for the farm business since the adoption of IMV requires extra labor inputs. Thus, farmer’s adoption of IMV is strongly associated with the amount of labor force available. The farm business is more of field work than formal education and hence farmers with longer years in farming are expected to adopt innovation faster than farmers with fewer years of farming. Knowledge gained over time can also help farmers to evaluate the merits of a new technology thereby influencing their decisions on the new product (Simtowe et al., Citation2011). Moreover, farmers who have spent some years cultivating the local variety of maize may find it difficult to switch to IMV as they have become accustomed to the local variety. Production of IMV may require person-hours for the application of inputs such as fertilizer, pesticides and weed control and therefore scarcity of labor may affect the decision of farmers to adopt IMV. We, thus, expect that availability of labor will increase adoption of IMV. IMV seeds readily available enhance the farmer’s adoption; hence, we expect a positive correlation between seed availability and adoption. Further, farmers living close to their farms are expected to intensify their adoption of IMV. This is because living close to the farm saves a lot of time walking to the farm or saves money through transportation. With this, farmers can minimize their production cost and could, therefore, afford to purchase the IMV input.
Institutional factors such as extension services, access to credit, membership of FBOs, and farmer’s attendance to seminar or demonstration fields are hypothesized to influence farmer’s adoption decisions (see Awotide et al., Citation2016; Mmbando & Baiyegunhi, Citation2016; Sisay et al., Citation2015). Extension officers are considered as sources of information on farm innovation. Having access to credit provides a means for farmers to purchase the required inputs to implement a new farm technology. Attending farm seminar or conference and been a member of FBOs allows the farmer to get in touch with their colleagues and share ideas, particularly on a new farm technology. Organization of seminar or demonstration fields serves as a platform for information dissemination and also improves the farmer’s technical skills. Therefore, farmers contact with extension services, access to credit, membership of FBO, and participation in seminar or demonstration field are expected to have positive relations with IMV adoption. Table presents the definitions and measurement of variables contained in the models and their descriptive statistics.
Table 1. Summary definition of variables
3. Results and discussions
3.1. Factors explaining the adoption of improved maize variety
The results from the MNL model used to examine factors influencing the adoption of IMV are presented in Table . The hypothesis that all the regression coefficients are jointly equal to zero was rejected as indicated by the Wald test [χ2(245) = 988.45; ρ = 0.000]. This indicates that the model is appropriate for the sampled data. The base category is the adoption of local variety (non-adoption of IMV), where the results are compared. The results indicate that the estimated coefficients do not differ much across the two alternatives. It must be noted that the coefficients of the variables in Table are the Relative Risk Ratios (RRR). The relative risk ratio of an outcome m relative to the reference group is expected to change by a factor of the respective parameter estimate given that the other variables in the model are held constant. A relative risk ratio greater than one (1) means that if a variable increase by a unit, the relative risk for adopting any of the two packages relative to the reference group (non-adoption) is expected to increase by a factor of the estimated parameter of that variable. The opposite is true if the RRR is less than one (1).
Table 2. Multinomial logit regression of factors influencing adoption of IMV
Age is significant and positively affects the probability of a farmer’s decision to cultivate IMV only as well as a combination of IMV and local variety than just planting a local variety. The relative risk ratio of age which is less than one (1) for adopters of only IMV and adopters of both IMV and local variety implies that older farmers usually prefer to grow only the local variety than cultivating either the improved or the combination of the two. This conforms to our apriori expectation but disagrees with the findings of Islam, Sumelius, and Bäckman (Citation2012) that older and hence experienced farmers readily adopt new technologies. The inverse relationship between age and adoption in the study area can be attributed to the fact that older farmers are used to their conventional ways of farming and usually find it difficult to switch, unlike young people who are associated with a higher risk-taking behavior. Household size is significant and positively related to the probability of the two alternatives (planting only IMV and the combination of the two). The relative risk ratio of [1.13] shows that there is 1.13 times as much probability for adopting either IMV or IMV and local variety among larger households than among smaller households. In other words, increasing the household size by one person will increase their probability of adoption of IMV by 1.13 times with other variables held constant. Indeed, adoption of new IMVs is labor intensive. Hence, large households tend to have free labor supply toward the adoption of the innovation than the smaller households. In a similar study by Sodjinou, Glin, Nicolay, Tovignan, and Hinvi (Citation2015), families with a substantial number of persons adopt organic cotton than those with a smaller number of persons. Similarly, the relative risk ratio (1.034 for IMV and 1.071 for IMV and local variety) for the farmer’s level of experience shows that there is a higher probability of adopting IMV only and the combination of local variety and IMV for farmers with longer years of experience than farmers with less experience in agricultural crop production. Consistent with this study, Ojo and Ogunyemi (Citation2014) found a significant positive relationship between many years in farming and adoption of farm technology.
In a similar vein, there is a positive and significant relationship between adoption of IMV only as well as the combination of IMV and the local variety, and workshop or seminar attendance. Farmers who get the opportunity to attend agricultural conferences, seminars and workshops organized by international and local NGOs are exposed to these varieties, their benefits and cultivation process, which increases their knowledge level and boost their morale of adoption. Thus, farmers with farm seminar or workshop records tend to have a higher probability of switching from local variety to IMV as indicated by a higher relative risk ratio of 3.203 for only IMV and 1.455 for the combination of IMV and local variety. The positive and significant effect of education is consistent with the assertion that educated farmers can easily assimilate information and therefore adopt improved technology much more comfortable than the uneducated farmers (Diiro et al., Citation2015; Gebresilassie & Bekele, Citation2015). The results further indicated that having access to agricultural credit positively and significantly affect farmer’s decisions on cultivating both IMV and local variety over local variety. As reported in Table , a farmer who had access to agricultural credit have 7.70 times probability of adopting the combination of the IMV and the local variety than their counterparts with no access to credit. Fisher and Carr (Citation2015) in their analysis of factors influencing adoption of drought-tolerant maize seed in Eastern Uganda found a positive relationship between access to credit and adoption of drought-tolerant maize variety. However, access to credit has no significant influence on the probability of adopting only IMV over local variety.
Institutional variables such as extension services and farmer’s membership of farmer-based organizations (FBO) are essential sources of information. Farmers get a lot of information with regard to production and marketing from extension officers and through a farmer-to-farmer network. From the results, being a member of an FBO increases the probability of a farmer to adopt an IMV. Also, farmers with regular extension contacts have a higher likelihood of adopting an IMV than those with no extension contacts. The result is consistent with a recent study by Mmbando and Baiyegunhi (Citation2016) who found a positive relationship between these two institutional variables (FBO and extension contacts) and adoption of improved maize varieties in Hai district of Tanzania. Labor significantly affect the probability of adopting IMVs over local variety and the probability of adopting both local and improve varieties over only local variety at 1% level of significance in both cases. This indicates that holding other variables constant, increasing farmers’ capacity to employ a unit of human labor will increase their probability to adopt both local and improve varieties by 2.35 times more than those who cannot hire more human labor (Etoundi & Dia, Citation2008).
3.2. Factors influencing intensity of IMV adoption
Estimated results from the Tobit and transformed OLS regression models are presented in Table . The Tobit model had a sigma value of 1.39019 and is statistically significant at 1% level of significance indicating that the data were appropriate for the model. The log likelihood value of −165 is an indication of a high explanatory power of the Tobit model. The model of the OLS is free of multicollinearity as indicated by Variance Inflation Factor (VIF) mean value of 1.88. The VIF for each independent variable was less than the critical value of 10 indicating non-existence of multicollinearity (Gujarati & Sangeetha, Citation2009). Comparing the two models in Table , the Tobit model gives better results than the OLS model; hence, we focused on the Tobit model to explain the effects of the explanatory variables on the dependent variable (intensity of IMV adoption). The results from the Tobit model presented in Table show that seven out of twelve variables included in the model (Tobit) are significant in explaining the variation in the intensity of IMV adoption.
Table 3. Results of adoption intensity from Tobit and Transformed OLS models
These variables include educational attainment, household size, distance from home to the farm plot, participation in demonstration fields, membership of FBO, farm size, and previous income from maize crop. Many years in formal education is statistically significant and have a positive correlation with the intensity of IMV adoption. Thus, farmers with a relatively high level of education intensify the adoption of IMV than their counterparts with a low level of education. This is not surprising as many studies have reported a positive relationship between adoption of improved farm technology and farmers level of education (see Ahmed, Citation2015; Deepa, Bandyopadhyay, & Mandal, Citation2015; Kebede & Tadesse, Citation2015). Household size had a significant and positive influence on the intensity of IMV. Farming in SSA, particularly in the study area is more intensive as mechanization remains rare. Hence, having larger household size helps in the farm operations since IMV requires some farm cultural practices such as frequent weeding and application of pesticides. The results of this study agree with that of Sodjinou et al. (Citation2015) who reported positive and significant effects of household size and adoption of organic farming. More extended distance from the farmer’s home to the farm plot has the potential to affect the farm business negatively as farmers may feel tired by the time they get to the farm or may have to spend extra money to commute from the house to the farm field. This is seen in the results of the study as the distance from farmers’ house to the farm plot has an inverse correlation with the intensity of adoption.
The probability of farmers adopting and intensifying the IMV is higher in households with larger farm sizes than those with smaller farm sizes. This is because farmers with larger farm sizes are usually into commercial farming and will usually plant IMV for profit maximization. However, Lunduka, Fisher, and Snapp (Citation2012) reported negative and significant effects of farmland holdings and opened-pollinated variety of maize in Malawi. Previous income from maize farm did not meet our apriori expectation. The estimated results show that the probability of farmers intensifying IMV on their farmland is low for farmers who had more income from their maize farm in the previous season than those who had little income. This could partly be attributed to the fact that farmers who had more revenue in the last season might have diversified their income into other farm or non-farm business. Farmers participating in demonstration farms or on-farm trials have a higher probability of allocating a more significant proportion of their maize farmland to IMV compared to those who did not participate as indicated in the empirical findings. Through demonstration farms, farmers become aware of the attributes of IMV and acquire sufficient knowledge to make adoption decisions. Farmers learn more and become more sensitize through visuals and hands-on than hearing, hence the importance of demonstration fields. These results complement those of Mmbando and Baiyegunhi (Citation2016) and Gecho and Punjabi (Citation2011). Finally, farmer’s membership of FBO variable is significant and positively related to the intensity of IMV adoption, implying that farmers belonging to FBOs adopt IMV more than the non-members of FBOs. Similar results were reported by Mmbando and Baiyegunhi (Citation2016) in Tanzania, Ojo and Ogunyemi (Citation2014) and Ugwumba and Okechukwu (Citation2014) in Nigeria.
4. Conclusions
The study estimated the determinants of adoption and the intensity of adoption of IMVs in the Tolon district of Ghana using farm-level data collected from 200 maize farm households. The empirical results indicated that adoption was influenced by factors such as the age of the household head, household size, experience, workshop attendance, education, membership of FBO, amount of labor, and extension contacts. Further, factors such as formal education, household size, distance from home to farm plot, participation in farm demonstrations, membership of FBO, farm size and previous income from maize crop significantly influence farmer’s adoption intensity of IMV in the study area.
5. Policy implications
Some significant lessons have emerged from this study which needed to be tackled comprehensively. First, the investigation revealed that education of farm households is key to enhancing adoption. Formal education of young people through aggressive human and infrastructural development is required as the youth are encouraged to consider agriculture as a business and not “a way of life.” Formal adult education where numeracy is thought can be integrated into already existing intervention programmes, FBOs and extension service delivery, and these have the potential to shape the decision-making process of the farmers. Public education on farm management practices (including IMV adoption) can also be intensified through radio, mobile phone services, and any available platform by MoFA to re-enforce farmers’ knowledge on adoption of agricultural technologies. Intensifying extension services in the rural areas where most agricultural production occurs will promote adoption of farm technologies. More attention should be given to the organization of on-farm trials with the farmers to enhance their technical and managerial abilities, hence, boost IMV adoption. Finally, the formation of FBOs should be encouraged to promote farmer-farmer extension services and knowledge sharing.
Funding
The authors received no direct funding for this research.
Additional information
Notes on contributors
Gideon Danso-Abbeam
Gideon Danso-Abbeam is a lecturer and a researcher at the Department of Agricultural and Resource Economics, University for Development Studies, Ghana, where he teaches courses in applied economics at the undergraduate level. Gideon is currently a PhD student with the Discipline of Agricultural Economics, University of KwaZulu-Natal, South Africa. His research interest includes adoption, efficiency and impact evaluation studies.
Joshua Antwi Bosiako
Joshua Antwi Bosiako holds Bsc in Agribusiness (Resource Economics option) and currently a technical officer with the socioeconomic department of Savannah Agricultural Research Institute (SARI), Tamale, Ghana. His main research interests include adoption and impact studies.
Dennis Sedem Ehiakpor
Dennis Sedem Ehiakpor is a lecturer and a PhD candidate at the Department of Agricultural and Resource Economics, University for Development Studies, Ghana. His main research activities are in the area of agricultural marketing and finance, adoption as well as impact studies.
Franklin Nantui Mabe
Franklin Nantui Mabe is a lecturer and a PhD candidate at the Department of Agricultural and Resource Economics, University for Development Studies, Ghana. Franklin has researched and published on various themes relating to adoption, efficiency and welfare economics.
Notes
1. The Ghana Poverty and Inequality study was conducted by Cooke, Hague, and McKay (Citation2016), using the Ghana Living Standard Survey round six.
2. The five maize cultivated agro-ecological zones are; (1) Coastal Savannah zone that runs along the coast of Ghana (Central, Greater Accra and the southern part of Volta regions); (2) The High rainforest zone which covers most part of the Western region and a small portion of the central region; (3) The semi-deciduous forest zone stretches from Ashanti, Eastern, Southern part of the Brong-Ahafo region and some parts of the Western region; (4) The forest savannah lies in the middle portion of Brong-Ahafo, the northern part of both Ashanti and Eastern regions and the Western portion of Volta region, and (5) the Guinea Savannah which covers the whole of Northern and Upper West regions. However, in the Sudan Savannah zone within the Upper East region, maize is cultivated in areas within some parts of Navrongo and Bawku.
References
- Adeoti, A. I. (2009). Factors influencing irrigation technology adoption and its impact on household poverty in Ghana. Journal of Agriculture and Rural Development Tropics and Subtropics, 109, 51–63.
- Adesina, A. A. (1996). Factors affecting the adoption of fertilizers by rice farmers in Cote D’Ivoire in nutrient cycling. Nutrient Cycling in Agroecosystems, 46(1), 29–39.10.1007/BF00210222
- Afolami, C. A., Obayelu, A. E., & Vaughan, I. I. (2015). Welfare Impact of adoption of improved cassava varieties by rural households in South Western Nigeria. Agricultural and Food Economics, 3(1), 1–7.
- Ahmed, M. H. (2015). Adoption of multiple agricultural technologies in maize production of the Central Rift Valley of Ethiopia. Studies in Agricultural Economics, 117(3), 162–168.10.7896/issn.2063-0476
- Alene, A. D., Menkir, A., Ajala, S. O., Badu-Apraku, B., & Olanrewaju, A. S. (2009). The economic and poverty impacts of maize research in West and Central Africa. Agricultural Economics, 40(5), 535–550.10.1111/agec.2009.40.issue-5
- Alexandratos, N., & Bruinsma, J. (2012). World agriculture towards 2030/2050: The 2012 Revision ( ESA Working Paper No. 12-03). Rome: Food and Agriculture Organization of the United Nations (FAO), Agricultural Development Economics Division.
- Amanor-Boadu, V. (2012). Maize price trend in Ghana (2007–2011): METSS—Ghana Research and Issue ( Paper Series, No. 01-2012).
- Awotide, B. A., Abdoulaye, T., Alene, A., & Manyong, V. M. (2014). Assessing the extent and determinant of adoption of improved cassava varieties in South-western Nigeria. Journal of Development and Agricultural Economics, 6(9), 376–385.
- Awotide, B. A., Aziz, A. K., & Diagne, A. (2016). Agricultural technology adoption, commercialization and smallholder rice farmers’ welfare in Rural Nigeria. Agricultural and Food Economics, 4, 3.
- Baltas, G., & Doyle, P. (2001). Random utility models in marketing research: A survey. Journal of Business Research, 51(2), 115–125.10.1016/S0148-2963(99)00058-2
- Borooah, V. K. (2002). Logit and probit ordered and multinomial models. CA: Sage.
- Bruins, M. (2009). The evolution and contribution of plant breeding to global agriculture, In: UPOV, responding to the challenges of a changing world: The role of new plant varieties and high quality seed in agriculture. In Proceedings of the Second World Seed Conference held at the FAO Headquarters, Rome (pp. 18–29). September 8-10, 2009. Geneva: UPOV.
- CGIAR. (2011). forty findings on the impacts of CGIAR research, 1971–2011. Washington, DC: Consultative Group on International Agricultural Research (CGIAR) Fund Office, World Bank.
- Cooke, E., Hague, S., & McKay, A. (2016). Ghana poverty and inequality report: Using the 6th Ghana living standard survey. Retrieved from https://www.unicef.org/ghana/Ghana_Poverty_and_Inequality_Analysis_FINAL
- Deepa, R., Bandyopadhyay, A. K., & Mandal, M. (2015). Factors related to adoption of maize production technology in cooch behar district of West Bengal. Agriculture, 5(2), 775–777.
- Denkyirah, E. K., Okoffo, E. D., Adu, D. T., Aziz, A. A., Ofori, A., & Denkyirah, E. K. (2016). Modeling Ghanaian cocoa farmers’ decision to use pesticide and frequency of application: The case of Brong-Ahafo region. SpringerPlus, 5(1), 1–13.
- Diiro, G. (2013). Impact of off-farm income on agricultural technology adoption intensity and productivity: Evidence from rural maize farmers in Uganda, Uganda Support Programme IFPRI Working Papers (vol. 11).
- Diiro, M. G., Ker, A. P., & Sam, A. G. (2015). The role of gender in fertilizer adoption in Uganda. African Journal of Agricultural and Resource Economics, 10(2), 117–130.
- Etoundi, S. M. N., & Dia, B. K. (2008). Determinants of the adoption of improved varieties of maize in Cameroon: Case of CMS 8704. Proceedings of the African Economic Conference, 2008, 397–413.
- FAO. (2015). Food and Agriculture Organization of the United Nations, Country Fact Sheets on Food and Agriculture Policy Trends. Ghana: Food and Agriculture Policy Development Analysis.
- Fisher, M., & Carr, E. R. (2015). The influence of gendered roles and responsibilities on the adoption of technologies that mitigate drought risk: The case of drought-tolerant maize seed in eastern Uganda. Global Environmental Change, 35, 82–92.10.1016/j.gloenvcha.2015.08.009
- Gebresilassie, L., & Bekele, A. (2015). Factors determining allocation of land for improved wheat variety by smallholder farmers of Northern Ethiopia. Journal of Development and Agricultural Economics, 7(3), 105–112.
- Gecho, Y., & Punjabi, N. K. (2011). Determinants of adoption of improved maize technology in Damot Gale, Wolaita, Ethiopia. Raj Journal of Extension Education, 19, 1–9.
- Ghana Statistical Service. (2014a). Ghana Living Standard Survey 6: Poverty Profile Report. Ghana: Government of Ghana.
- Ghana Statistical Service. (2014b). 2010 Housing and population census, district analytical report – Tolon District. Ghana: Ghana Statisitcal Service.
- Ghana Statistical Services. (2016). Revised 2015 annual gross domestic product (GDP). Ghana: Ghana Statisitcal Service.
- Grigoriou, C., Guillaumont, P., & Yang, W. (2005). Child mortality under Chinese reforms. China Economic Review, 16, 441–464.10.1016/j.chieco.2005.03.004
- Gujarati, D. N., & Sangeetha, P. (2009). Basic econometrics (4th ed.). New Delhi: Tata McGraw Hill Education Private Limited.
- Guris, S., Metin, N., & Caglayan, E. (2006). The brand choice model of wine consumers: A multinomial logit model. Quality and Quantity, 41, 447–460.
- Hanemann, W. M. (1984). Discrete/continuous models of consumer demand. Econometrica, 52, 541–561.10.2307/1913464
- Hardin, J., & Hilbe, J. (2001). Generalized linear models and extensions. College Station, TX: Stata Press.
- IFC. (2013). Working with Smallholders: A hand for Firms Building Sustainable Supply Chains. Washington D.C: International Finance Corporation, World Bank Group.
- Islam, K. Z., Sumelius, J., & Bäckman, S. (2012). Do differences in technical efficiency explain the adoption rate of HYV rice: Evidence from Bangladesh. Agricultural Economics Review, 13(1), 93–104.
- Kaliba, A., Verkuijl, H., & Mwangi, W. (2000). Factors affecting adoption of improved maize seeds and use of inorganic fertilizer for maize production in the intermediate and 39 Lowland Zones of Tanzania. Journal of Agricultural and Applied Economics, 32(1), 35–47.10.1017/S1074070800027802
- Kassie, M., Jaleta, M., Shiferaw, B., Mmbando, F., & Mekuria, M. (2013). Adoption of interrelated sustainable agricultural practices in smallholder systems: Evidence from rural Tanzania. Technological Forecasting and Social Change, 80(3), 525.10.1016/j.techfore.2012.08.007
- Kebede, W. M., & Tadesse, D. (2015). Factors affecting adoption of malt-barley technology: Evidence from North Gondar Ethiopia. Journal of Food Security, 3(3), 75–81.
- Krishna, V. V., & Qaim, M. (2008). Potential impacts of Bt eggplant on economic surplus and farmers’ health in India. Agricultural Economics, 38(2), 167–180.10.1111/agec.2008.38.issue-2
- Lunduka, R., Fisher, M., & Snapp, S. (2012). Could farmer interest in a diversity of seed attributes explain adoption plateaus for modern maize varieties in Malawi? Food Policy, 37, 504–510.10.1016/j.foodpol.2012.05.001
- Madala, G. S. (2005). Introduction to Econometrics (3rd ed.). Chichester: Wiley.
- Mendola, M. (2007). Agricultural technology adoption and poverty reduction: A propensity-score matching analysis for rural Bangladesh. Food Policy, 32, 372–393.10.1016/j.foodpol.2006.07.003
- Mmbando, F. E., & Baiyegunhi, L. J. (2016). Socio-economic and institutional factors influencing adoption of improved maize varieties in Hai District, Tanzania. Journal of Human Ecology, 53(1), 49–56.10.1080/09709274.2016.11906955
- Ministry of Food and Agriculture. (2013). Statistical, research and information directorate. Accra: Author.
- Nmadu, J. N., Sallawu, H., & Omojeso, B. V. (2015). Socio-economic factors affecting adoption of innovations by cocoa farmers in Ondo State, Nigeria. European Journal of Business, Economics and Accountancy, 3(2), 58–66.
- Ojo, S. O., & Ogunyemi, A. I. (2014). Analysis of factors influencing the adoption of improved cassava production technology in Ekiti State, Nigeria. International Journal of Agricultural Sciences and Natural Resources, 1(3), 40–44.
- Ouma, J. O., & De Groote, H. (2011). Determinants of improved maize seed and fertilizer adoption in Kenya. Journal of Development and Agricultural Economics, 3(11), 529–536.
- Papke, L. E., & Wooldridge, J. M. (1996). Econometric methods for fractional response variables with an application to 401(k) plan participation rates. Journal of Applied Econometrics, 11, 619–632.10.1002/(ISSN)1099-1255
- Pryce, G., & Mason, P. (2006). Controlling for transactions bias in regional house price indices. In Paper presented at the Conference in Honour of Patric Hendershott (July), Columbus Ohio.
- Rogers, E. (1962). Diffusion of innovations (1st ed.). New York, NY: Free Press of Glencoe.
- Simtowe, F., Kassie, M., Diagne, A., Silim, S., Muange, E., Asfaw, S., & Shiferaw, B. (2011). Determinants of agricultural technology adoption: The case of improved Pigeonpea varieties in Tanzania. Quarterly Journal of International Agriculture, 50(4), 325–345.
- Sisay, D., Jema, H., Degye, G., & Abdi-Khalil, E. (2015). Speed of improved maize seed adoption by smallholder farmers in Southwestern Ethiopia: Analysis using the count data models. Journal of Agricultural Economics, Extension and Rural Development, 3(5), 276–282.
- Smale, M., Byerlee, D., & Jayne, T. (2011). Maize revolutions in Sub-Saharan Africa Policy Research Working Paper 5659. Washington, DC. The World Bank, Development Research Group, Agriculture and Rural Development Team.10.1596/prwp
- Sodjinou, E., Glin, C. L., Nicolay, G., Tovignan, L., & Hinvi, J. (2015). Socioeconomic determinants of organic cotton adoption in Benin, West Africa. Agricultural and Food Economics, 3(1), 12.
- Taha, M. (2007). Determinants of the adoption of improved onion production package in Dugda Bora District, East Shoa, Ethiopia, M.Sc. Thesis (Unpublished). Presented to School of Graduate Studies of Haramaya University.
- Teklewold, H., Kassie, M., & Shiferaw, B. (2013). Adoption of multiple sustainable agricultural practices in rural Ethiopia. Journal of Agricultural Economics, 64(3), 597–623.10.1111/1477-9552.12011
- Thomson, K., Gelson, T., & Elias, K. (2014). Adoption of improved maize varieties in Southern Zambia. Asian Journal of Agricultural Sciences, 6(1), 33–39.
- Tobin, J. (1958). Estimation of relationships for limited dependent variables. Econometrica, 26(1), 24–36.10.2307/1907382
- Train, K. E. (2003). Discrete choice methods with simulation. New York, NY: Cambridge University Press.10.1017/CBO9780511753930
- Ugwumba, C. O. A., & Okechukwu, E. O. (2014). Adoption of improved maize production technologies in Enugu State, Nigeria. International Journal of Agriculture Innovations and Research, 3(1), 259–261.
- Waithaka, M. M., Thornton, P. K., Shepherd, K. D., & Ndiwa, N. N. (2007). Factors affecting the use of fertilizers and manure by smallholders: The case of Vihiga, western Kenya. Nutrient Cycling in Agroecosystems, 78(3), 211–224.10.1007/s10705-006-9087-x
- Wale, E. (2010). How do farmers allocate land for coffee trees? Implications for On-farm conservation and seed technology adoption. Journal of Sustainable Agriculture, 34(3), 270–291.10.1080/10440041003613313
- Walker, T., Alene, A., Ndjeunga, J., Labarta, R., Yigezu, Y., Diagne, A., … Sushil, P. (2014). Measuring the effectiveness of crop improvement research in sub-Saharan Africa from the perspectives of varietal output, adoption, and change: 20 crops, 30 countries, and 1150 cultivars in farmers’ fields ( Report of the Standing Panel on Impact Assessment (SPIA). Rome, Italy: CGIAR Independent Science and Partnership Council (ISPC) Secretariat.
- World Bank (2007). World development report 2008: Agriculture for development. Washington, DC: Author.